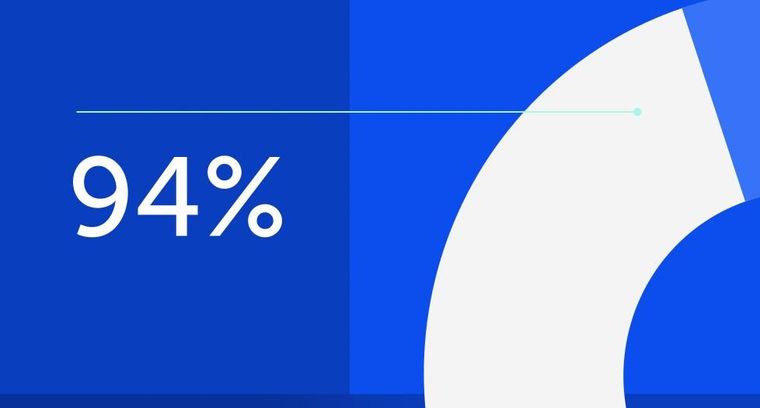
94% of researchers rate our articles as excellent or good
Learn more about the work of our research integrity team to safeguard the quality of each article we publish.
Find out more
ORIGINAL RESEARCH article
Front. Educ., 24 April 2023
Sec. STEM Education
Volume 8 - 2023 | https://doi.org/10.3389/feduc.2023.1062053
This article is part of the Research TopicEye Tracking for STEM Education Research: New PerspectivesView all 12 articles
This secondary analysis of an earlier eye-tracking experiment investigated how triangulating changes in pupil dilation with student-self reports can be used as a measure of cognitive load during instructional videos with complex chemical representations. We incorporated three signaling conditions, dynamic, static and no signals, into instructional videos to purposefully alter cognitive load. Our results indicate that self-reported extraneous cognitive load decreased for dynamic signals compared to static or no signals, while intrinsic cognitive load was not affected by the signaling condition. Analysis of pupil dilation show significantly larger pupils for dynamic signals as compared to the other two conditions, suggesting that when extraneous cognitive load decreased, students still engaged cognitively with the task. Correlation analyses between measures were only significant for pupil dilation and extraneous cognitive load, but not pupil dilation and intrinsic cognitive load. We argue that beneficial design choices such as dynamic signals lead to more working memory capacity that can be leveraged toward learning. These findings extend previous research by demonstrating the utility of triangulating self-report and psychophysiological measures of cognitive load and effort.
To develop learning materials that align with insights from cognitive science, theories of human cognitive architecture are used to shape instructional approaches. Particularly in STEM, where the subject matter gets increasingly abstract and complex, effective learning materials are indispensable. One consensus framework guiding instructional design is Cognitive Load Theory (CLT) which proposes that learning occurs when information is initially processed in working memory and subsequently stored in long-term memory (Sweller et al., 1998, 2019). The mental effort expended in working memory is referred to as cognitive load, and during learning this load can be induced by the difficulty of the task (referred to as intrinsic cognitive load, or ICL) or by its design (referred to as extraneous cognitive load, or ECL; Sweller et al., 2019). Because working memory is limited in capacity and duration, learning is impeded when working memory capacity is exceeded, i.e., when one experiences excessive cognitive load. One goal of CLT-informed instructional design is to minimize ECL in order to keep enough working memory resources free for managing ICL of the material to be learned.
Following STEM researchers’ and teachers’ interest in supporting student learning by altering and optimizing instructional design, we investigated the impact of several design choices on students’ cognitive load. We designed instructional videos on organic chemistry reaction mechanisms, because (1) small alterations can be made to videos to detect differences while keeping the overall instruction constant, and (2) reaction mechanisms are known to be visually and conceptually demanding and thus difficult to learn for students (for reviews, see Gilbert, 2005; Graulich, 2015; Daniel et al., 2018). One main student challenge in organic chemistry involves understanding the domain-specific representations and linking them to the underlying chemical concepts. Students often struggle to identify the relevant entities (Rodemer et al., 2020), which induces cognitive load (Rodemer et al., 2022). Since these chemical representations are intrinsically complex, unnecessary cognitive load might be counteracted with reducing extraneous load by optimized instructional design. To examine the impact of design on load, we chose different signaling techniques derived from multimedia learning principles (de Koning et al., 2009; Mayer, 2014; van Gog, 2014). By guiding students’ attention to relevant parts of the learning material, signals facilitate comprehension and reduce cognitive load. Specifically, we compared how cognitive load is influenced by three signaling conditions: sequential signaling (dynamic), permanent signaling (static), and no signaling (control).
Cognitive load can be assessed by using psychophysiological and self-reported measures. A well-known and reliable indicator for cognitive load is pupil dilation, which can be measured with an eye tracker. Pupillometry has been used extensively to investigate cognitive load in different learning scenarios (for reviews, see Beatty and Lucerno-Wagoner, 2000; Just et al., 2003; van der Wel and van Steenbergen, 2018). However, little work has examined the influence of different types of load on pupil dilation. The present study is a secondary analysis from our prior eye-tracking experiment focusing on the impact of signals on learning outcomes, cognitive load and attention (Rodemer et al., 2022). In this report, we present the first analysis of pupil diameter and its relationship to the previously reported cognitive load self-reports.
Cognitive Load Theory describes that learning capability is influenced by human cognitive architecture. More specifically, learning capability is limited by the capacity of human working memory (Sweller et al., 1998, 2011, 2019; van Merriënboer and Sweller, 2005). The amount of information that can be processed simultaneously in working memory restricts the amount of information that can be learned, i.e., information that can be stored in long-term memory. The limitation of working memory accounts especially for novel information that is obtained through sensory systems, since this information must be ordered and integrated (van Merriënboer and Sweller, 2005). The acquisition of expertise, or in other words, learning, is hindered when working memory capacity is exceeded (Sweller et al., 2019).
Regarding the two types of cognitive load, ICL is determined by the expertise of the learner and their interaction with the given nature of the learning material (van Merriënboer and Sweller, 2005). It is caused by the amount of information that must be processed simultaneously in working memory, i.e., ICL depends on the extent of element interactivity of the learning material. The larger the number of interacting elements, the more difficult the given content is understood. In order to facilitate understanding, these interacting elements need to be incorporated into cognitive schemata, which are acquired over time through experience with subject material. Thus, ICL of a task or material decreases with expertise in a specific domain (van Merriënboer and Sweller, 2005). With a specific learning goal and learning task at hand, ICL cannot be altered purposefully by instructional interventions (van Merriënboer and Sweller, 2005). In contrast, ECL does not contribute to load necessary for understanding the material at hand (van Merriënboer and Sweller, 2005). ECL is induced by sub-optimal design choices, where a learner has to search for relevant information, or by triggering weak problem-solving methods (van Merriënboer and Sweller, 2005). Hence, ECL can be altered purposefully by instructional interventions.
Intrinsic cognitive load and ECL have an additive relationship to each other. If one load is exceeded, working memory capacity is exceeded in total, resulting in impeded learning (Paas et al., 2003; Cowan, 2010). If a task is perceived as easy, i.e., ICL is low, then a high ECL might be manageable for a learner, since the overall working memory capacity is kept within its limits. However, if ICL is high, ECL must be decreased in order for a learner to work through a task without cognitive overload (Kalyuga, 2011; Sweller et al., 2019). Hence, the goal of well-designed instructional material is to reduce ECL so that available cognitive resources can be fully devoted to the actual learning process (Mayer, 2005, 2021).
Based on CLT, the Cognitive Theory of Multimedia Learning proposes several design principles in order to manage cognitive load effectively (Mayer, 2005, 2021). Multimedia formats such as instructional videos utilize both the auditory and visual sensory channels, which has specific implications for designing these learning materials. Building upon this dual-channel assumption (Clark and Paivio, 1991), the CTML puts forward that auditory and visual information must first be integrated in working memory before they can be stored in long-term memory (Mayer, 2021). In line with CLT and CTML, attention that is available for each of these two separate information processing channels is limited (limited-capacity assumption; Mayer, 2014). Multimedia learning material is considered effective when each channel is addressed in its natural form, i.e., when images or representations are seen and when sounds are heard (Mayer, 2014). To leverage learning, the modality principle suggests verbal explanations better complement visual stimuli as opposed to displaying text on screen (Low and Sweller, 2014). Other well-researched principles for reducing ECL are summarized as follows (Mayer and Fiorella, 2014): The coherence principle declares that task-irrelevant details, such as additional texts or decorative pictures, should be excluded. The redundancy principle emphasizes that information that is simultaneously provided through multiple sensory channels places additional cognitive load on the learner, e.g., by providing a verbal narration and printed text. The spatial and temporal contiguity principle suggests that corresponding words and pictures should be presented near to each other or simultaneously rather than separately or successively.
Beyond these guidelines for reducing ECL, a great body of research is concerned with the signaling principle (for meta-analyses; see Richter et al., 2016; Xie et al., 2017; Schneider et al., 2018; Alpizar et al., 2020). The signaling principle states that a visual cue or highlight that emphasizes relevant parts of the learning material reduces ECL by guiding attention, particularly when the amount of information is difficult to change. Signals can appear as a circle, arrow, or by coloring specific parts. A visual signal is known to support a learner to focus on relevant features of a display. The underlying mechanism is that cognitive resources that might otherwise be directed toward visual search are freed up (de Koning et al., 2009).
Several approaches to measuring cognitive load have been proposed. These approaches are based either on subjective judgments or on objective measurements, and thus address load either directly, e.g., by asking learners to rate their perceived mental load, or indirectly, e.g., by using indicators that are thought to reflect learners’ mental load, such as performance (Klepsch et al., 2017). Generally, the different approaches all show strengths and weaknesses (see Brünken et al., 2010). In educational research, subjective ratings of cognitive load are the most frequently used approach (e.g., Schmeck et al., 2015; Krieglstein et al., 2022). In these approaches, the learner is asked, in most cases retrospectively, to rate the perceived amount of cognitive load on a Likert scale while working on a task. Generally, this approach is considered beneficial due to its economy and flexibility. In addition, the retrospective rating does not disturb the learning process and impose load by itself, which may be the case in other approaches such as dual-task measures (Brünken et al., 2010). A recent meta-analysis concluded that self-reports of perceived cognitive load also are a valid and reliable measure (Krieglstein et al., 2022). However, there is empirical evidence that the rating of cognitive load depends on certain personal and situational aspects, such as the timing of the measurement (Brünken et al., 2010) and subjective internal standards for evaluating current load state (Klepsch et al., 2017). Furthermore, multidimensional measures of cognitive load often show significant correlations between different types of cognitive load (e.g., ICL and ECL), which seems inconsistent with the additivity hypothesis of the cognitive load theory (Krieglstein et al., 2022).
Another stream of research is concerned with small changes in pupil diameter that are attributed to reflect changes in brain activity, or, more specifically, human cognition (Beatty and Lucerno-Wagoner, 2000; Just et al., 2003; van der Wel and van Steenbergen, 2018). In this stream, pupil dilation has been used as a proxy measure for many cognitive processes, including arousal, attention, and cognitive load (Stanners et al., 1979; Klingner et al., 2010; Kang et al., 2014; Miller and Unsworth, 2020). Although this relationship between pupil dilation and cognitive effort was first reported over 100 years ago (e.g., Löwenstein, 1920), it was popularized as a systematic course of study with seminal studies in the mid-1960s, which demonstrated that an increase in pupil size compared to baseline, up to 0.5 mm, could be discretely correlated with mental effort exerted in increasingly complex numerical recall tasks (Hess and Polt, 1964; Kahneman and Beatty, 1966). Recent neurobiological studies suggest that this effect is due to activation of the noradrenergic system’s locus coeruleus, which is activated by stress, and may also play a role in memory consolidation (Beatty and Lucerno-Wagoner, 2000; Laeng et al., 2012; van der Wel and van Steenbergen, 2018).
Studies on task-evoked pupillary responses (TEPR) focus on how changes in attention or cognitive effort during a task can be measured through changes to pupil size compared to baseline. This response can be isolated through careful control of the environment, e.g., controlling external stimuli such as change in brightness or excessive movement that can induce a change in pupil size, and careful design of the experiment to reduce the number of conflicting cognitive signals (Beatty and Lucerno-Wagoner, 2000; Karch, 2018).
The relationship between experimental design and the nature of what is being assessed through pupil dilation is not straightforward. Many studies correlate pupil dilation with task demand, e.g., cognitive load, particularly for simple tasks such as arithmetic, repeating back an increasingly long stream of numbers or letters, or entering a difficult password (Hess and Polt, 1964; Kahneman and Beatty, 1966; Klingner, 2010; Krejtz et al., 2018; Abdrabou et al., 2021). However, a recent meta-review of TEPR studies suggests that this relationship is more complicated, and that pupil dilation can better be understood as cognitive effort rather than task demand (van der Wel and van Steenbergen, 2018). Thus, it is crucial to understand how a participant may be experiencing a task in order to interpret their pupil dilation, because more novice performers in a task may have higher pupil dilations to reflect that they need to put in more effort to grapple with the task, and more expert performers may have smaller dilations due to the fact that they need to exert less effort (Ahern and Beatty, 1979; Szulewski et al., 2017; van der Wel and van Steenbergen, 2018; Zhou et al., 2022).
There have been several promising studies that it is possible to ascribe meaning to pupil dilations collected in situ, e.g., while one is engaged in a task, to understand how engaging with the task involves cognitive effort (e.g., Palinko et al., 2010; Krejtz et al., 2020; da Silva Castanheira et al., 2021; Shechter and Share, 2021). However, few have tried to make claims about the nature of the cognitive load that induces this effort, in part because of the difficulty associated with interpreting psychophysiological signals (Cacioppo and Tassinary, 1990). Some have done so through deliberate experimental design. For example, Foroughi et al. (2017) found that pupil size decreased as participants completed multiple trials of an experiment, suggesting they automatized the process. Shechter and Share (2021) conducted word recognition experiments, finding significantly larger relative changes in pupil size for stimuli associated with higher cognitive effort. Another way to investigate pupil dilations may be to triangulate other sources of data, such as gaze data (e.g., Klingner, 2010; Karch et al., 2019; Miller and Unsworth, 2020), spatio-temporal sensory cues (e.g., Sharma et al., 2021), interviews (e.g., Pomerleau-Turcotte et al., 2021), motivational manipulation by task-switching (da Silva Castanheira et al., 2021), microsaccadic responses (Krejtz et al., 2020), and through probes mid-task (Franklin et al., 2013) to try to understand the underlying cognitive process reflected in the pupil dilation.
The goal of the present experiment was twofold. The first was a conceptual goal. We wanted to understand how different design choices for signaling during instructional videos impacted the cognitive load students experienced while watching these videos (RQ1). While there is a large body of research supporting the cognitive benefit of signals, most of this evidence is based on learning outcomes. Additionally, many studies make use of rather simple tasks that require rapid mental operations in working memory (e.g., arithmetic or memory scanning) and/or that can be solved without substantial prior knowledge but on basis of the given instruction. The first research question of this study is:
RQ1: Under which condition is either students’ ICL or ECL reduced while watching instructional videos with either dynamic, static, or no signals?
Based on the literature, we expect to reduce ECL by providing signals in a descending order from control to static to dynamic signaling, e.g., that tasks with the control signal will result in the highest ECL, whereas tasks with dynamic signaling will have the lowest ECL. Furthermore, we hypothesize that based on our task design, ICL will be kept constant across signaling conditions (Richter et al., 2016; Xie et al., 2017; Schneider et al., 2018; Alpizar et al., 2020).
The second was a methodological goal. Although pupillometry can potentially offer an in situ method to examine how cognitive load changes over time, few studies have looked at change in pupil dilation while watching instructional videos (Huh et al., 2019), in part because pupil signals can be challenging to isolate and interpret. Additionally, the relationship between pupil dilation and different types of cognitive load is unclear. Traditional TEPR studies focus on the relationship between pupil dilation and task difficulty, e.g., ICL (e.g., Hess and Polt, 1964; Kahneman and Beatty, 1966; Szulewski et al., 2017). Some studies have started to look at how altering the design of a task to provide visual supports impacts cognitive load, e.g., focusing on the relationship between pupil dilation and ECL (Zheng and Cook, 2012; Kruger et al., 2013). However, neither of these studies conducted a targeted study on the relationship between ECL and pupil size, but rather looked at the effect on cognitive load as a whole. Mitra et al. (2017) used CLT to show that it is possible to use pupillary responses to infer the extent to which students experience different types of cognitive load, but their study was conducted using fairly straightforward tasks such as question comprehension or mental math. Thus, we wanted to understand how pupil dilations change when the ECL of an authentic instructional task is altered, due to modifying the signaling condition but not the difficulty of the tasks (RQ2). By triangulating self-report measures and psychophysiological measures of cognitive load, our goal is to contribute to making pupillometry a more useful and interpretable measure for educational research. Thus, the second research question is:
RQ2: Does pupillometry indicate differences in pupil diameter when altering extraneous load across experimental conditions?
Our hypothesis is that pupil diameter is affected by different extraneous load conditions. We predict that as extraneous load goes down across the three signaling conditions, we will see a corresponding decrease in pupil dilation.
The study presented here is a re-analysis of prior work from the first and last author, which focused primarily on how the signaling conditions in the instructional videos impacted students’ attention, self-reported cognitive load, and learning outcomes (Rodemer et al., 2022). In this study, 28 undergraduate chemistry students (50% female, 50% male; 0% nonbinary) from a German university participated on a voluntary base in winter semester 2019. Participants were currently enrolled in an introductory general chemistry course to ensure that they had sufficient prior knowledge to potentially understand the rather complex chemical reactions that were presented in our instructional videos. All participants had normal or corrected-to-normal vision. None of the participants reported on either color vision deficiency or specific learning disabilities (e.g., dyslexia) that might have impacted their processing of the videos or their cognitive load.
A 1 × 3 within-subject design was employed in which the instructional videos were manipulated according to three different signaling conditions (i.e., no signaling vs. static signaling vs. dynamic signaling). Each participant watched three videos in a constant video order but received each video including one of the three signaling conditions. To control for potential sequencing effects, each of the three signaling conditions were presented according to a counterbalanced 3 × 3 Latin Square design to evaluate potential effects of treatment position and video content on the dependent variables (Tabachnick and Fidell, 2007; see also Figure 1). Participants were randomly assigned to one of the three treatment sequences, which were implemented as a between-subject factor.
Figure 1. Experimental 3 × 3 Latin Square design showing signaling condition, instructional video and treatment sequence. The boxes display a screenshot from the chemical representations that were explained in the videos and the design of the signaling conditions. All participants received the instructional videos in the same order with regards to content, while the order of the signaling condition differed according to their treatment sequence.
Three instructional videos covering introductory organic reaction mechanisms at the university level were developed (Eckhard et al., 2022). Each video focuses on one of three chemical factors that influence reaction speed, namely leaving group ability (video 1), substrate effects (video 2), and nucleophilic strength (video 3). Overall, the difficulty of each video was comparable since the chemical factors chosen for each example can be understood independently from each other and do not built upon each other. To keep the design of the videos constant, representations on the display were arranged the same way and verbal explanations that accompanied the task followed the same structure. Videos had a length of approximately 5 min each (for German (original) and English (translated) videos, see: https://osf.io/r4sx3/).
Each video was presented as a case comparisons of nucleophilic substitution reactions. This task format is common in chemistry entailing complex representations, such as structural formulas and electron-pushing arrows (Caspari et al., 2018; Graulich and Schween, 2018; Bodé et al., 2019). Students needed to compare commonalities and differences between the representations, connect these features to chemical factors from the verbal explanation, and critically weigh the factors in terms of their influence on the reaction speed. The corresponding verbal explanations were narrated in line with recommendations based on the modality principle (Ayres and Sweller, 2014). The explanations followed a step-by-step structure that are commonly used in worked examples (Renkl, 2014). The only aspect in which the videos differed from each other were the example reactions that were chosen to highlight different factors that influence reaction speed. The structure of the explanation was kept comparable across instructional videos.
Concerning the experimental factor signaling condition, either no signals (i.e., control condition), static signals (i.e., permanent coloring of specific representational features), or dynamic signals (i.e., a sequential red dot) were added to the videos. The dynamic signal was embedded when the narration mentioned specific relevant features of the representations, lasting anywhere from single words to several consecutive sentences. For each instructional video (i.e., Videos 1, 2, and 3), the narrated explanation was identical in all signaling conditions but differed between instructional videos based on the content they present.
The instructional videos were presented on a 24-inch screen with a 1920 × 1080 pixel resolution using the software Tobii Pro Lab. Participants sat in front of the screen at approximately 60-cm distance with headsets to follow the verbal explanation of the videos without distractions. Participants’ pupil diameters were recorded using a Tobii Pro Spectrum, an eye tracking device with a 200 Hz sampling rate which estimates true pupil size based on the participants’ distance from the eye tracker and shape of their cornea (Karch, 2018). The system was calibrated using 9-point calibration and subsequent validation. The calibration accuracy was below 0.5° for all participants (M = 0.30°, SD = 0.21°).
To prepare pupillometric data for analysis, raw data with Tobii I-VT Fixation Filter with a threshold of 30°/s were exported from Tobii Pro Lab. Raw data were uploaded to and processed in RStudio. Following Mathôt’s (2018) guidelines on pre-processing pupil data and adapting code from the second author (Karch, 2018), blinks were removed by calculating a velocity profile to identify when there were rapid changes in pupil size, indicating that the eyes closed, and removing points that fell outside of the threshold of three standard deviations from the median velocity (Leys et al., 2013; Kret and Sjak-Shie, 2019). Then data were smoothed using a rolling average over three data points (a window of 15 ms) to remove potential noise at very high frequencies from instrument error. Finally, for each video, baseline values calculated based on the median of the first ten samples were subtracted from all pupil size values to give dilation data. These baseline-subtracted dilation values were then used for all statistical analyses described below (processing code can be accessed online at: https://osf.io/r4sx3/).
We used the established self-report scales by Klepsch et al. (2017) to measure intrinsic and extraneous cognitive load. Participants rated their perceived cognitive load on a 7-point rating scale (1 = low, 7 = high) immediately after each video. The cognitive load items were presented on the computer screen and were read aloud by the test supervisor. To adapt the measure according to the context of the study, the wording in the items were changed from “task” to “video.” Cronbach’s α indicated a sufficiently high reliability of the two scales (αICL = 0.91; αECL = 0.85).
The study followed ethical standards recommended by the German Research Foundation: Upon arrival, participants were fully informed about the voluntary nature, goals, process, and data handling of this study. All participants signed a written informed consent and were aware that they could withdraw their consent at any time.
The study was performed in single sessions of 1.5 h in a light-controlled environment. After completing a pen and paper questionnaire about demographics, participants were familiarized with the eye tracker and the calibration procedure. Calibration was repeated until high accuracy was reached. Then, participants were instructed to watch the three instructional videos carefully and that they could not pause or rewind. In between each video, the cognitive load items were asked. Once the instruction was completed, participants received a monetary compensation. The procedure was kept constant for all participants.
Our analyses of variance were focused on the effects of the experimental signaling conditions (within-subject measure), potential differences across the three instructional videos, and also included the sequence of experimental conditions (see Figure 1) as a between-subject factor. To answer our research questions, we were most interested in the main effects of the three signaling conditions. Position and sequence effects were investigated to control for potential content or carryover effects across conditions in our within-subject design. Post-hoc pairwise comparisons were performed using Benjamini-Hochberg adjustment. As measure for the effect size (partial) η2 and the correlation coefficient r are reported, where values are interpreted according to Cohen (1988). Statistical analyses were performed using R Version 4.0.4 and several packages, notably ‘tidyverse’, ‘ExpDes’, ‘rmcorr’, and ‘lme4’ (Bates et al., 2015; Bakdash and Marusich, 2017; Wickham et al., 2019; Batista Ferreira et al., 2021; R Core Team, 2021).
As expected, our analysis of ICL showed no main effect for the experimental factor signaling condition (F(2,83) = 0.26, p = 0.769, η2 = 0.05; Figure 2, left). Further, we found no significant effect for the instructional video (F(2,83) = 2.24, p = 0.113, η2 < 0.01), whereas a significant effect was present for the factor treatment sequence (F(2,83) = 5.69, p = 0.005, η2 = 0.13). Regarding the treatment sequence, pairwise comparisons indicated that Sequence 2 (dynamic/static/control) showed significantly lower ICL compared to both Sequence 1 (control/dynamic/static, p = 0.003) and Sequence 3 (static/control/dynamic, p = 0.048). Sequences 1 and 3 did not differ significantly (p = 0.319).
Figure 2. Means for intrinsic cognitive load (left), extraneous cognitive load (center), and pupil dilation (right) by signaling condition. Points indicate mean-values, error bars 95% confidence intervals. Results of pairwise comparisons are indicated by significance levels (NS. p > 0.05; *p < 0.05; **p < 0.01; ***p < 0.001).
As predicted, the analysis of ECL showed a significant main effect for the experimental factor signaling condition (F(2,83) = 8.89, p < 0.001, η2 = 0.19; Figure 2, center). Pairwise comparisons indicated a significant lower ECL for the condition with dynamic signals compared to the condition with static signals or no signals in the control condition (both p < 0.001). There was no difference between the static and control condition (p = 1.00). Furthermore, we found no significant effects for the factor instructional video (F(2,83) = 1.54, p = 0.221, η2 = 0.04) or the factor treatment sequence (F(2,83) = 2.88, p = 0.062, η2 = 0.07).
With regard to mean pupil dilation values, the analysis showed a significant main effect for the experimental factor signaling condition (F(2,83) = 3.24, p = 0.045, η2 = 0.08; Figure 2, right), no significant effect for the instructional video (F(2,83) = 1.72, p = 0.186, η2 = 0.04), and no significant effect for the factor treatment sequence (F(2,83) = 1.04, p = 0.355, η2 = 0.03). Although the average measures for all pupil data suggest that during task participants’ eyes were constricted compared to baseline, pairwise comparisons indicated a significant larger relative dilation for the condition with dynamic signals compared to the condition with no signals in the control condition (p = 0.001). There was no significant difference between the static and control condition (p = 0.065) and between the static and the dynamic condition (p = 0.195).
To gain more fine-grained insights into the processes of the video consumption, students’ pupil dilation has been analyzed across time for each of the videos. Figure 3 illustrates the time course of the average pupil dilation across participants for each of the three videos and separated by treatment condition. Peaks and valleys represent changes in pupil dilation over time, where peaks represent instances of higher cognitive load. These graphs show that the dilatory response to videos 1 and 3 were consistently higher in the dynamic condition compared to the control and static condition, and that the control condition was the lowest, while the mean dilations across time for video 2 tended to be more similar. Additionally, the shapes of the graphs, i.e., where there tended to be peaks and valleys, were relatively similar across all three conditions, suggesting that students may have experienced stimuli that induced cognitive load at similar points. This is what we would anticipate, as the scripts and video were identical across all three conditions. These time course graphs provide additional qualitative evidence that the mean pupil dilations shown in Figure 2 (right) reflected differences that were maintained across the entire course of each instructional video.
Repeated measures correlation coefficients for pairwise correlations between self-reported cognitive load scales (ICL and ECL) and pupil dilation were calculated to analyze the relationship between these measures. Findings indicate a negative association between ECL and pupil dilation, rrm (55) = −0.25, 95% CI [−0.48, −0.02], p = 0.06 (Figure 4, left), i.e., when students report higher extraneous cognitive load after watching the video, their mean pupil dilation is more negative, indicating smaller pupil size. The association between ICL and pupil dilation is also negative, but smaller and not significant (rrm (55) = −0.10, 95% CI [−0.30, 0.17], p = 0.46; Figure 4, center). The association between ICL and ECL is positive (rrm (55) = 0.53, 95% CI [0.33, 0.72], p < 0.01; Figure 4, right).
Figure 4. Repeated measures correlation plots for the association between pupil dilation and extraneous cognitive load (ECL; Left) and intrinsic cognitive load (ICL; Center), respectively, as well as between ECL and ICL (Right). Each participant provides three data points (one per video) that are shown in a different color. The colored lines show repeated measurement correlation fits for each participant. The black line indicates the overall regression line.
This secondary analysis followed a conceptual and a methodological goal. The first goal was to investigate how different types of signaling impacted students’ cognitive load while watching instructional videos containing complex chemical representations. The second goal was to examine the relationship between pupil dilation and self-reports while altering different types of cognitive load. To approach these goals we implemented dynamic, static, or no signaling in instructional videos and recorded pupil dilations with an eye-tracker as well as collected self-reports on intrinsic and extraneous cognitive load with an established questionnaire.
The analysis of ICL self-reports showed no main effect for the experimental factor signaling condition. This result was expected since the difficulty of each instructional video was kept comparable. A main effect was found for the treatment sequence, indicating that participants perceived the instructional videos to be easier when they received them in the order dynamic–static–control. This finding may possibly be attributed to fading-out support over time—an instructional principle that is well-known in research concerning worked examples (Renkl, 2014). In such a fading procedure, full support is provided in the first example. Then, in the following examples, the amount of support decreases until only the problem that is to be solved is left.
Results of ECL self-reports showed a significant reduction for dynamic signals as compared to static or no signals. Consistent with CLT and CTML (Sweller et al., 2019; Mayer, 2021), the reduction of ECL through dynamic signals can be attributed to a reduction of search space. Showing a dynamic signal facilitated information selection from the visual representations. Furthermore, the dynamic signal supported integrating the audible explanation and the visual representation. Based on the dual-coding assumption and the CTML, we argue that the dynamic signal from our instructional video supports the integration of the auditory and visual information in working memory by increasing attention to relevant entities, and, thus freeing up working memory capacity that otherwise would be attributed to searching the relevant representations that are mentioned in the explanation. Considering the intrinsic complexity of the chemical representations, reducing unnecessary load might support students in overcoming their difficulty in connecting these representations with the underlying concepts (Graulich, 2015).
Given this finding, we would have expected there to be a corresponding decrease in cognitive load as measured by pupil dilation. However, results showed significantly larger pupils in the dynamic signaling condition as compared to the control condition without signals, but not significantly different for the comparisons dynamic—static and static—control. This result is surprising because we anticipated that a dynamic signal would decrease cognitive load, and thus lead to smaller pupil dilation (Hess and Polt, 1964; Klingner et al., 2010). A possible explanation might be that dynamic signaling increased cognitive processing, e.g., working memory allocated to productive mental effort, as opposed to cognitive load, e.g., working memory allocated to deal with a task (Krejtz et al., 2020; Shechter and Share, 2021). Comparing both results, the reduction of ECL by self-reports and the increase of pupil dilation, supports the interpretation of increased cognitive processing. When ECL is reduced and ICL stays constant, more working memory capacity can be directed toward (productive) mental effort, such as cognitive schemata formation, which is in line with findings described in TERP-literature (Mitra et al., 2017; van der Wel and van Steenbergen, 2018; da Silva Castanheira et al., 2021; Shechter and Share, 2021; Zhou et al., 2022). Another explanation might be that dynamic signals increased curiosity because of their movement, which would also be reflected in pupil diameter changes (van der Wel and van Steenbergen, 2018). Although we applied a tight research design varying only one factor, we cannot rule out this explanation because we did not collect additional affective variables. Consequently, further research is needed to inform a valid interpretation of findings based on changes in pupil diameter in the context of multimedia learning and complex, domain-specific representations.
One limitation of studies with pupillary data is that they cannot be interpreted in isolation, because pupillary signals may have many confounding sources. However, secondary sources of evidence can be used to support interpretation of pupillary data (e.g., Franklin et al., 2013; Krejtz et al., 2020; Miller and Unsworth, 2020; da Silva Castanheira et al., 2021). Our first secondary source of evidence are the self-reports of cognitive load, as discussed above. Our second source is evidence from two earlier studies from our research group that found gains in overall learning performance and retention moderated through dynamic signals in instructional videos (Rodemer et al., 2021, 2022). In the case of the control condition without signaling, ECL was higher and pupil dilation was lower, indicating that cognitive resources might be occupied by a visuospatial searching process. Without appropriate support, cognitive resources may have been overloaded, leading to participants’ disengagement with the instruction, which is reflected in smaller pupil size and thus less mental effort (Peavler, 1974; Krejtz et al., 2018; van der Wel and van Steenbergen, 2018). In the case of the dynamic signaling, ECL was lower and pupil dilation was higher, and learning gains were increased, suggesting that the additional cognitive effort indicated by pupil dilation was a result of productive mental effort that led to these increased learning gains (Mitra et al., 2017; van der Wel and van Steenbergen, 2018).
Repeated measures correlation analyses show a significant correlation between pupil dilation and ECL but not ICL. This suggests the perceived inherent difficulty of the videos to be unrelated to the extent of cognitive processing, while the video design, e.g., the signaling condition, seems to be the more important factor, at least in the present study. Mitra et al. (2017) showed that pupils dilated to different types of cognitive load. In their study, they altered the intrinsic difficulty (ICL) of the tasks while keeping the extraneous difficulty (ECL) constant. However, their study used very simple tasks that are hardly comparable to the rather complex instructional videos we used in our experiment, since the chemical representations presented require specific domain-specific understanding which is not the case for the graphs used in the study by Mitra and colleagues. Although our results indicate a crucial role of the instructional design that takes the extraneous difficulty into account, more systematic research is needed to further investigate the relations between different types of cognitive load and pupil dilation, particularly during domain-specific learning tasks.
When designing this experiment, we argued that instructional design should be modified with the goal to reduce extraneous cognitive load, e.g., by implementing dynamic signals. The results from this study suggests that not only do dynamic signals reduce ECL, this reduction may free up enough mental resources that students have a larger capacity to grapple with the task itself or with learning processes. This is suggested by the presence of larger pupil sizes during tasks with lower reported ECL, suggesting that students were still cognitively engaged and putting mental effort into the task. This has several implications for practice. First, implementing dynamic signaling in instructional videos may support student learning in the class. Second, although the videos in our study were designed to all have the same relative level of difficulty, it is possible that the resources freed up by reducing ECL may free up space for higher levels of ICL. That is, dynamic signaling may be a useful scaffold when instructors introduce more intrinsically difficult tasks. Third, our study provides support for a transfer of the fading principle to the application of signaling in instructional videos. The fading principle describes gradually fading support over time which was originally described in Renkl’s (2014) theory of example-based learning. Finally, although our study focuses on the use of dynamic signaling in organic chemistry instructional videos, the theoretical foundation of the work is not drawn from chemistry but rather CMTL, thus it may be possible that our findings on the effect of dynamic signaling in instructional videos may be applicable to other domains.
With regards to research, we demonstrated the utility of triangulating findings from self-report cognitive load measures and pupillometric data. In particular, we showed that combining these two streams of data facilitated a more nuanced analysis of the possible effect of reducing ECL, e.g., freeing up resources for students to engage and provide effort in other ways when working with the task. Our research demonstrates promising potential that in combination with secondary data sources (self-reports and student outcomes), pupillary data can be meaningfully interpreted in more naturalistic and complex educational tasks, such as the case comparisons reported here. Future research should investigate pupil dilation by systematically varying stimuli with different levels of difficulty to induce different amounts of intrinsic cognitive load.
This study found that dynamic signals as compared to static or no signals reduced students’ self-reported extraneous cognitive load without impacting intrinsic cognitive load during the consumption of instructional videos containing complex chemical representations. Furthermore, significant correlations were only found between pupil dilation and self-reported extraneous cognitive load, but not intrinsic cognitive load. Our results call for a stronger emphasis on instructional design to manage cognitive load. Based on the assumption that pupil dilation indicates mental effort, more systematic research is needed that investigates different types of cognitive load across tasks and instructions that vary in context and complexity.
The raw data supporting the conclusions of this article will be made available by the authors, without undue reservation.
Ethical review and approval was not required for the study on human participants in accordance with the local legislation and institutional requirements. The patients/participants provided their written informed consent to participate in this study.
MR: conceptualization, methodology, formal analysis, investigation, resources, data curation, writing—original draft, and visualization. JK: conceptualization, methodology, formal analysis, writing—original draft. SB: conceptualization, methodology, formal analysis, writing—review and editing, supervision, project administration, and funding acquisition. MR and JK contributed equally to the writing of the original draft. All authors contributed to the article and approved the submitted version.
This work was supported by the German Research Foundation DFG (Deutsche Forschungsgemeinschaft) under Grant 329801962.
We thank all the students who participated in our study. We also thank Julia Eckhard, Nicole Graulich, Gyde Asmussen, and Svea Hinrichsen.
The authors declare that the research was conducted in the absence of any commercial or financial relationships that could be construed as a potential conflict of interest.
All claims expressed in this article are solely those of the authors and do not necessarily represent those of their affiliated organizations, or those of the publisher, the editors and the reviewers. Any product that may be evaluated in this article, or claim that may be made by its manufacturer, is not guaranteed or endorsed by the publisher.
Abdrabou, Y., Abdelrahman, Y., Khamis, M., and Alt, F. (2021). Think harder! Investigating the effect of Passwort strength on cognitive load during password creation. In Extended Abstracts of the 2021 CHI Conference on Human Factors in Computing Systems. New York: Association for Computing Machinery, 1–7.
Ahern, S., and Beatty, J. (1979, 4412). Pupillary responses during information processing vary with scholastic aptitude test scores. Science (New York, N.Y.) 205, 1289–1292. doi: 10.1126/science.472746
Alpizar, D., Adesope, O. O., and Wong, R. M. (2020). A meta-analysis of signaling principle in multimedia learning environments. Educ. Technol. Res. Dev. 68, 2095–2119. doi: 10.1007/s11423-020-09748-7
Ayres, P., and Sweller, J. (2014). “The Split attention principle in Mutlimedia learning,” in Cambridge Handbooks in Psychology. The Cambridge Handbook of Multimedia Learning. ed. R. E. Mayer (Cambridge: Cambridge University Press), 206–226.
Bakdash, J. Z., and Marusich, L. R. (2017). Repeated measures correlation. Front. Psychol. 8:456. doi: 10.3389/fpsyg.2017.00456
Bates, D., Mächler, M., Bolker, B., and Walker, S. (2015). Fitting linear mixed-effects models using lme4. J. Stat. Softw. 67, 1–48. doi: 10.18637/jss.v067.i01
Batista Ferreira, E., Piscitelli Cavalcanti, P., and Alves Nogueira, D. (2021). ExpDes: Experimental designs package [R package]. Version 1.2.2. Available at: https://CRAN.R-project.org/package=ExpDes (Accessed September 09, 2022).
Beatty, J., and Lucerno-Wagoner, B. (2000). “The pupillary system,” in Handbook of Psychophysiology. eds. J. T. Cacioppo, L. G. Tassinary, and G. G. Berntson 2nd ed (Cambridge: Cambridge University Press), 142–162.
Bodé, N. E., Deng, J. M., and Flynn, A. B. (2019). Getting past the rules and to the WHY: causal mechanistic arguments when judging the plausibility of organic reaction mechanisms. J. Chem. Educ. 96, 1068–1082. doi: 10.1021/acs.jchemed.8b00719
Brünken, R., Seufert, T., and Paas, F. G. (2010). “Measuring cognitive load,” in Cognitive Load Theory. eds. J. L. Plass, R. Moreno, and R. Brunken (Cambridge: Cambridge University Press), 181–202.
Cacioppo, J. T., and Tassinary, L. G. (1990). Inferring psychological significance from physiological signals. Am. Psychol. 45, 16–28. doi: 10.1037//0003-066x.45.1.16
Caspari, I., Kranz, D., and Graulich, N. (2018). Resolving the complexity of organic chemistry students’ reasoning through the lens of a mechanistic framework. Chem. Educ. Res. Pract. 19, 1117–1141. doi: 10.1039/C8RP00131F
Clark, J. M., and Paivio, A. (1991). Dual coding theory and education. Educ. Psychol. Rev. 3, 149–210. doi: 10.1007/BF01320076
Cohen, J. (1988). Statistical Power Analysis For the Behavioral Sciences. 2nd edn. Hillsdale, NJ: L. Erlbaum Associates.
Cowan, N. (2010). The magical mystery four: how is working memory capacity limited, and why? Curr. Dir. Psychol. Sci. 19, 51–57. doi: 10.1177/0963721409359277
da Silva Castanheira, K., LoParco, S., and Otto, A. R. (2021). Task-evoked pupillary responses track effort exertion: evidence from task-switching. Cogn. Affect. Behav. Neurosci. 21, 592–606. doi: 10.3758/s13415-020-00843-z
Daniel, K. L., Bucklin, C. J., Leone, E. A., and Idema, J. (2018). “Towards a definition of representational competence,” in Towards a Framework for Representational Competence in Science Education. ed. K. L. Daniel (Cham: Springer International Publishing), 3–11.
Koning, B. B.De, Tabbers, H. K., Rikers, R. M. J. P., and Paas, F. (2009). Towards a framework for attention cueing in instructional animations: guidelines for research and design. Educ. Psychol. Rev., 21, 113–140. doi: 10.1007/s10648-009-9098-7
Eckhard, J., Rodemer, M., Bernholt, S., and Graulich, N. (2022). What do University students truly learn when watching tutorial videos in organic chemistry? An exploratory study focusing on mechanistic reasoning. J. Chem. Educ. 99, 2231–2244. doi: 10.1021/acs.jchemed.2c00076
Foroughi, C. K., Sibley, C., and Coyne, J. T. (2017). Pupil size as a measure of within-task learning. Psychophysiology 54, 1436–1443. doi: 10.1111/psyp.12896
Franklin, M. S., Broadway, J. M., Mrazek, M. D., Smallwood, J., and Schooler, J. W. (2013). Window to the wandering mind: Pupillometry of spontaneous thought while reading. Q. J. Exp. Psychol. 66, 2289–2294. doi: 10.1080/17470218.2013.858170
Gilbert, J. K. (2005). “Visualization: a metacognitive skill in science and science education,” in Models and Modeling in Science Education: Vol. 1. Visualization in Science Education. ed. J. K. Gilbert (Dordrecht: Springer), 9–27.
Graulich, N. (2015). The tip of the iceberg in organic chemistry classes: how do students deal with the invisible? Chem. Educ. Res. Pract. 16, 9–21. doi: 10.1039/C4RP00165F
Graulich, N., and Schween, M. (2018). Concept-oriented task design: making purposeful case comparisons in organic chemistry. J. Chem. Educ. 95, 376–383. doi: 10.1021/acs.jchemed.7b00672
Hess, E. H., and Polt, J. M. (1964). Pupil size in relation to mental activity during simple problem-solving. Science (New York, N.Y.) 143, 1190–1192. doi: 10.1126/science.143.3611.1190
Huh, D., Kim, J.-H., and Jo, I.-H. (2019). A novel method to monitoring changes in cognitive load in video-based learning. J. Comput. Assist. Learn. 35, 721–730. doi: 10.1111/jcal.12378
Just, M. A., Carpenter, P. A., and Miyake, A. (2003). Neuroindices of cognitive workload: neuroimaging, pupillometric and event-related potential studies of brain work. Theor. Issues Ergon. Sci. 4, 56–88. doi: 10.1080/14639220210159735
Kahneman, D., and Beatty, J. (1966). Pupil diameter and load on memory. Science (New York, N.Y.) 154, 1583–1585. doi: 10.1126/science.154.3756.1583
Kalyuga, S. (2011). Cognitive load theory: how many types of load does it really need? Educ. Psychol. Rev. 23, 1–19. doi: 10.1007/s10648-010-9150-7
Kang, O., Huffer, K. E., and Wheatley, T. (2014). Pupil dilation dynamics track attention to high-level information. PLoS One 9, 1–6. doi: 10.1371/journal.pone.0102463.g001
Karch, J. M. (2018). “Beyond gaze data: Pupillometry as an additional data source in eye tracking,” in Eye tracking for the Chemistry Education Researcher. eds. J. R. VandenPlas, S. J. R. Hansen, and S. Cullipher 1st ed (Washington, DC: American Chemical Society), 145–163.
Karch, J. M., García Valles, J. C., and Sevian, H. (2019). Looking into the black box: using gaze and Pupillometric data to probe how cognitive load changes with mental tasks. J. Chem. Educ. 96, 830–840. doi: 10.1021/acs.jchemed.9b00014
Klepsch, M., Schmitz, F., and Seufert, T. (2017). Development and validation of two instruments measuring intrinsic, extraneous, and germane cognitive load. Front. Psychol. 8:1997. doi: 10.3389/fpsyg.2017.01997
Klingner, J. (2010). Measuring cognitive load during visual tasks by combining pupillometry and eye tracking. Dissertation. Stanford University, Stanford.
Klingner, J., Tversky, B., and Hanrahan, P. (2010). Effects of visual and verbal presentation on cognitive load in vigilance, memory, and arithmetic tasks. Psychophysiology 48, 323–332. doi: 10.1111/j.1469-8986.2010.01069.x
Krejtz, K., Duchowski, A. T., Niedzielska, A., Biele, C., and Krejtz, I. (2018). Eye tracking cognitive load using pupil diameter and microsaccades with fixed gaze. PLoS One 13:e0203629. doi: 10.1371/journal.pone.0203629
Krejtz, K., Duchowski, A. T., Zurawska, J., and Wichary, S. (2020). Pupillary and microsaccadic responses to cognitive effort and emotional arousal during complex decision making. J. Eye Mov. Res. 13:2. doi: 10.16910/jemr.13.5.2
Kret, M. E., and Sjak-Shie, E. E. (2019). Preprocessing pupil size data: Guidelines and code. Behav. Res. Methods 51, 1336–1342. doi: 10.3758/s13428-018-1075-y
Krieglstein, F., Beege, M., Rey, G. D., Ginns, P., Krell, M., and Schneider, S. (2022). A systematic meta-analysis of the reliability and validity of subjective cognitive load questionnaires in experimental multimedia learning research. Educ. Psychol. Rev. 34, 2485–2541. doi: 10.1007/s10648-022-09683-4
Kruger, J.-L., Hefer, E., and Matthew, G. (2013). Measuring the impact of subtitles on cognitive load. In ETSA ‘13 (Ed.), Proceedings of the 2013 Conference on Eye Tracking South Africa (pp. 62–66). Association for Computing Machinery.
Laeng, B., Sirois, S., and Gredebäck, G. (2012). Pupillometry: a window to the preconscious? Pers. Psychol. Sci. J. Assoc. Psychol. Sci. 7, 18–27. doi: 10.1177/1745691611427305
Leys, C., Ley, C., Klein, O., Bernard, P., and Licata, L. (2013). Detecting outliers: do not use standard deviation around the mean, use absolute deviation around the median. J. Exp. Soc. Psychol. 49, 764–766. doi: 10.1016/j.jesp.2013.03.013
Low, R., and Sweller, J. (2014). “The modality principle in multimedia learning,” in Cambridge Handbooks in Psychology. The Cambridge Handbook of Multimedia Learning. ed. R. E. Mayer (Cambridge: Cambridge University Press), 227–246.
Löwenstein, O. (1920). Experimentelle Beiträge zur Lehre von den katatonischen Pupillenveränderungen. Eur. Neurol. 47, 194–215. doi: 10.1159/000190690
Mathôt, S. (2018). Pupillometry: psychology, physiology, and function. J. Cogn. 1:16. doi: 10.5334/joc.18
Mayer, R. E. (2005). “A cognitive theory of multimedia learning,” in The Cambridge Handbook of Multimedia Learning. ed. R. E. Mayer (Cambridge: Cambridge University Press), 41–61.
Mayer, R. E. (2014). “Cognitive theory of multimedia learning,” in Cambridge Handbooks in Psychology. The Cambridge Handbook of Multimedia Learning. ed. R. E. Mayer (Cambridge: Cambridge University Press), 43–71.
Mayer, R. E., and Fiorella, L. (2014). “Principles for reducing extraneous processing in multimedia learning: coherence, signaling, redundancy, spatial contiguity, and temporal contiguity principles,” in Cambridge Handbooks in Psychology. The Cambridge Handbook of Multimedia Learning. ed. R. E. Mayer (Cambridge: Cambridge University Press), 279–315.
Miller, A. L., and Unsworth, N. (2020). Variation in attention at encoding: insights from pupillometry and eye gaze fixations. J. Exp. Psychol. Learn. Mem. Cogn. 46, 2277–2294. doi: 10.1037/xlm0000797
Mitra, R., McNeal, K. S., and Bondell, H. D. (2017). Pupillary response to complex interdependent tasks: a cognitive-load theory perspective. Behav. Res. Methods 49, 1905–1919. doi: 10.3758/s13428-016-0833-y
Paas, F., Renkl, A., and Sweller, J. (2003). Cognitive load theory and instructional design: recent developments. Educ. Psychol. 38, 1–4. doi: 10.1207/S15326985EP3801_1
Palinko, O., Kun, A. L., Shyrokov, A., and Heeman, P. (2010). Estimating cognitive load using remote eye tracking in a driving simulator. ETRA, 141–144. doi: 10.1145/1743666.1743701
Peavler, W. S. (1974). Pupil size, information overload, and performance differences. Psychophysiology 11, 559–566. doi: 10.1111/j.1469-8986.1974.tb01114.x
Pomerleau-Turcotte, J., Moreno Sala, M. T., Dubé, F., and Vachon, F. (2021). Does cognitive load differ among sight-singers? An exploratory study using pupillometry and interviews. In Proceedings of the Future Directions of Music Cognition International Conference March 6-7, Virtual Conference. Columbus: Ohio State University Libraries, pp. 158–162.
R Core Team. R: a language and environment for statistical computing (version 4.0.4) [computer software]. (2021). R Foundation for Statistical Computing. Vienna, Austria. Available at: https://www.R-project.org/
Renkl, A. (2014). Toward an instructionally oriented theory of example-based learning. Cogn. Sci. 38, 1–37. doi: 10.1111/cogs.12086
Richter, J., Scheiter, K., and Eitel, A. (2016). Signaling text-picture relations in multimedia learning: a comprehensive meta-analysis. Educ. Res. Rev. 17, 19–36. doi: 10.1016/j.edurev.2015.12.003
Rodemer, M., Eckhard, J., Graulich, N., and Bernholt, S. (2020). Decoding case comparisons in organic chemistry. Eye-tracking students’ visual behavior. J. Chem. Educ. 97, 3530–3539. doi: 10.1021/acs.jchemed.0c00418
Rodemer, M., Eckhard, J., Graulich, N., and Bernholt, S. (2021). Connecting explanations to representations: benefits of highlighting techniques in tutorial videos on students’ learning in organic chemistry. Int. J. Sci. Educ. 43, 2707–2728. doi: 10.1080/09500693.2021.1985743
Rodemer, M., Lindner, M. A., Eckhard, J., Graulich, N., and Bernholt, S. (2022). Dynamic signals in instructional videos support students to navigate through complex representations: an eye-tracking study. Appl. Cogn. Psychol. 36, 852–863. doi: 10.1002/acp.3973
Schmeck, A., Opfermann, M., van Gog, T., Paas, F., and Leutner, D. (2015). Measuring cognitive load with subjective rating scales during problem solving: differences between immediate and delayed ratings. Instr. Sci. 43, 93–114. doi: 10.1007/s11251-014-9328-3
Schneider, S., Beege, M., Nebel, S., and Rey, G. D. (2018). A meta-analysis of how signaling affects learning with media. Educ. Res. Rev. 23, 1–24. doi: 10.1016/j.edurev.2017.11.001
Sharma, H., Drukker, L., Papageorghiou, A. T., and Alison Noble, J. (2021). Multi-modal learning from video, eye tracking, and Pupillometry for operator skill characterization in clinical fetal ultrasound. In Proceedings of the 2021 IEEE 18th International Symposium on Biomedical Imaging April 13-16, Nice, Piscataway: Institute of Electrical and Electronics Engineers, Inc., 1646–1649.
Shechter, A., and Share, D. L. (2021). Keeping an eye on effort: a Pupillometric investigation of effort and effortlessness in visual word recognition. Psychol. Sci. 32, 80–95. doi: 10.1177/0956797620958638
Stanners, R. F., Coulter, M., Sweet, A. W., and Murphy, P. (1979). The pupillary response as an indicator of arousal and cognition. Motiv. Emot. 3, 319–340. doi: 10.1007/BF00994048
Sweller, J., van Merriënboer, J. J. G., and Paas, F. G. W. C. (1998). Cognitive architecture and instructional design. Educ. Psychol. Rev. 10, 251–296. doi: 10.1023/A:1022193728205
Sweller, J., van Merriënboer, J. J. G., and Paas, F. G. W. C. (2019). Cognitive architecture and instructional design: 20 years later. Educ. Psychol. Rev. 31, 261–292. doi: 10.1007/s10648-019-09465-5
Szulewski, A., Kelton, D., and Howes, D. (2017). Pupillometry as a tool to study expertise in medicine. Frontline Learn. Res. 5, 55–65. doi: 10.14786/flr.v5i3.256
Tabachnick, B. G., and Fidell, L. S. (2007). Experimental Designs Using ANOVA. Belmont, CA: Thomson/Brooks/Cole.
van der Wel, P., and van Steenbergen, H. (2018). Pupil dilation as an index of effort in cognitive control tasks: a review. Psychon. Bull. Rev. 25, 2005–2015. doi: 10.3758/s13423-018-1432-y
van Gog, T. (2014). “The signaling (or cueing) principle in multimedia learning,” in Cambridge Handbooks in Psychology. The Cambridge Handbook of Multimedia Learning. ed. R. E. Mayer (Cambridge: Cambridge University Press), 263–278.
van Merriënboer, J. J. G., and Sweller, J. (2005). Cognitive load theory and complex learning: recent developments and future directions. Educ. Psychol. Rev. 17, 147–177. doi: 10.1007/s10648-005-3951-0
Wickham, H., Averick, M., Bryan, J., Chang, W., McGowan, L., François, R., et al. (2019). Welcome to the Tidyverse. J. Open Source Softw. 4, 1686–1691. doi: 10.21105/joss.01686
Xie, H., Wang, F., Hao, Y., Chen, J., An, J., Wang, Y., et al. (2017). The more total cognitive load is reduced by cues, the better retention and transfer of multimedia learning: a meta-analysis and two meta-regression analyses. PLoS One 12:e0183884. doi: 10.1371/journal.pone.0183884
Zheng, R., and Cook, A. (2012). Solving complex problems: a convergent approach to cognitive load measurement. Br. J. Educ. Technol. 43, 233–246. doi: 10.1111/j.1467-8535.2010.01169.x
Keywords: eye-tracking, pupillometry, cognitive load, instructional videos, chemistry, representations
Citation: Rodemer M, Karch J and Bernholt S (2023) Pupil dilation as cognitive load measure in instructional videos on complex chemical representations. Front. Educ. 8:1062053. doi: 10.3389/feduc.2023.1062053
Received: 05 October 2022; Accepted: 06 April 2023;
Published: 24 April 2023.
Edited by:
Maike Schindler, University of Cologne, GermanyReviewed by:
Sarah Hansen, Columbia University, United StatesCopyright © 2023 Rodemer, Karch and Bernholt. This is an open-access article distributed under the terms of the Creative Commons Attribution License (CC BY). The use, distribution or reproduction in other forums is permitted, provided the original author(s) and the copyright owner(s) are credited and that the original publication in this journal is cited, in accordance with accepted academic practice. No use, distribution or reproduction is permitted which does not comply with these terms.
*Correspondence: Marc Rodemer, bWFyYy5yb2RlbWVyQHVuaS1kdWUuZGU=
Disclaimer: All claims expressed in this article are solely those of the authors and do not necessarily represent those of their affiliated organizations, or those of the publisher, the editors and the reviewers. Any product that may be evaluated in this article or claim that may be made by its manufacturer is not guaranteed or endorsed by the publisher.
Research integrity at Frontiers
Learn more about the work of our research integrity team to safeguard the quality of each article we publish.