- 1Department of Psychology, University of Houston, Houston, TX, United States
- 2Harris County Juvenile Probation Department, Houston, TX, United States
- 3Department of Molecular and Human Genetics, Baylor College of Medicine, Houston, TX, United States
Introduction: This study aimed to investigate (a) the immediate and long-term changes in youth offending rates among 138 neighborhoods within a large metropolitan area in the context of COVID-19 and (b) the extent to which the socioeconomic composition of the neighborhoods accounted for variations of the changes.
Methods: Discontinuous growth models were applied to demonstrate the changes in offenses against a person, property offenses, and drug-related offenses one-year prior to, at (March 2020), and one-year following the pandemic.
Results: At the onset of the pandemic, we registered an immediate reduction in offenses against a person and property offenses but not in drug-related offenses. There was a steeper declining trend for property offenses one-year following the pandemic as compared with that one-year prior to the pandemic. The neighborhood concentration of affluence and poverty was not related to the immediate reduction in any type of delinquency.
Discussion: We conclude that the COVID-19 pandemic not only had an abrupt but also an enduring impact on youth delinquency.
Introduction
In response to the COVID-19 pandemic, national and local authorities around the world implemented a variety of social restriction policies in order to curb the spread of the virus and keep their populations safe (Hodgkinson et al., 2022). Collectively referred to as “containment measures,” various social restrictions were designed to limit the transmission of the virus between individuals, usually involving some combination of stay-at-home orders, social distancing, travel bans, closures of schools, non-essential businesses, restaurants, and theaters, and barring public and private gatherings (Nivette et al., 2021; Payne et al., 2022). This unprecedented implementation of social restrictions has been considered “the largest criminological experiment in history” (Stickle and Felson, 2020, p. 525), which has led to a burgeoning body of research on the relationship between the COVID-19 pandemic and crime (e.g., Felson et al., 2020; Mohler et al., 2020; Nivette et al., 2021).
Nevertheless, existing studies are limited in several ways despite their high significance. First, the vast majority of studies have focused on the change in recorded crime in the context of the COVID-19 pandemic among adults. Less is known about the association between the pandemic and delinquency among youth (for exceptions, see McCarthy et al., 2021; Revital and Haviv, 2022). School closures and remote instruction that are unique containment measures for youth disrupted their routine activities. In turn, this disruption exerted a profound impact on youth delinquency.
Second, given the acute nature of the COVID-19 pandemic, nearly all existing research has been concentrated on the abrupt change of offending following the pandemic (i.e., whether the observed offending rates deviated from what one would have expected in the absence of the COVID-19 pandemic). From a developmental/life course criminology perspective, however, turning points, such as the pandemic, are not only presumed to stimulate an immediate but also a long-term change (Laub and Sampson, 1993). Yet, the enduring impact of the COVID-19 pandemic on offending has not drawn sufficient attention in the existing literature.
Third, despite the recognition of the heterogeneity of the relationship between COVID-19 and offending across communities (Campedelli et al., 2020; McCarthy et al., 2021; Andresen and Hodgkinson, 2022; Hodgkinson et al., 2022), contextual factors associated with such variation are understudied. This is because the units of analyses in existing research have overwhelmingly been cities (e.g., Mohler et al., 2020; Nivette et al., 2021) or countries (e.g., Gerell et al., 2020; Halford et al., 2020). However, focusing on crime change at the macro level precludes identifying “local” contextual factors that may exacerbate or mitigate the impact of the pandemic on crime and delinquent behavior.
The present study aimed to (a) evaluate the abrupt (the immediate change of offending in the month when containment measures were implemented) and long-term (developmental trajectory of offending 1 year following the implementation of containment measures relative to that 1 year prior to the pandemic) changes in youth offending rates in the context of the COVID-19 pandemic and (b) investigate the extent to which the socioeconomic composition of neighborhoods accounted for variations in the immediate and long-term changes in youth offending rates. Specifically, we employed discontinuous growth models (DGM) to evaluate changes of youth offending rates in offenses against a person, property offenses, and drug-related offenses among 138 neighborhoods in Harris County, Houston, Texas, from March 2019 to February 2021 and explored the extent to which the concentration of affluence and poverty accounted for neighborhood variations of youth offending changes prior to, at, and following the implementation of containment measures in response to the pandemic.
The abrupt change of offending following the COVID-19 pandemic
Implementing various containment measures, such as stay-at-home orders, drastically disrupted routine activities, which in turn had a spillover effect on the structural opportunity for crime and delinquency. The routine activity theory has been the dominant theory in understanding the relationship between the COVID-19 pandemic and offending. In their theory development, Cohen and Felson (1979) posited that structural changes in routine activity patterns could impact crime rate trends by affecting the concurrence in space and time of three elements—motivated offenders, suitable targets, and the absence of guardians against a violation. They further argued that the lack of any one of these elements is sufficient to prevent the successful completion of committing a crime.
An array of recent research has demonstrated that the impact of the structural change of routine activities on the change of offending in the context of COVID-19 varies as a function of crime types, locations, and time frames. In general, while a significant reduction of offending counts in shoplifting, theft, and theft from a vehicle following the pandemic has consistently been observed across different regions (Ashby, 2020; Halford et al., 2020; Hodgkinson and Andresen, 2020; Campedelli et al., 2021; Langton et al., 2021; Nivette et al., 2021; Payne et al., 2021; Koppel et al., 2022), findings for other types of crime are mixed. Specifically, (a) some studies have reported no change in assault (Ashby, 2020; Hodgkinson and Andresen, 2020; Mohler et al., 2020; Campedelli et al., 2021; Payne et al., 2022), whereas other studies have reported a significant reduction in assault (Gerell et al., 2020; Halford et al., 2020; Langton et al., 2021; Nivette et al., 2021; Koppel et al., 2022); (b) some studies have reported a significant reduction in residential burglary (Ashby, 2020; Halford et al., 2020; Payne et al., 2021; Koppel et al., 2022), whereas other studies have reported an increase in commercial burglary (Hodgkinson and Andresen, 2020; Koppel et al., 2022); and (c) some studies have reported no change in robbery (Gerell et al., 2020; Mohler et al., 2020), whereas other studies have reported a significant reduction in robbery (Campedelli et al., 2021; Langton et al., 2021; Nivette et al., 2021; Koppel et al., 2022).
Despite noticeable variations, existing findings largely supported the notion that the controlled closure (e.g., stay-at-home restrictions) and limited social interactions (e.g., cancelations of private and public gatherings) influenced criminal opportunities by increasing the guardianship and reducing the exposure of victims to motivated offenders, which in turn resulted in the abrupt change of offending (Stickle and Felson, 2020). It is important to note that the aforementioned studies all revolve around recorded crime data for adults. Research on the situational explanation of individual deviant behavior suggests that containment measures, particularly school closures, and remote instruction, may have had a profound effect on the developmental patterns of delinquency for youth (Buchanan et al., 2020; McCarthy et al., 2021). This assumption has been evidenced by two studies.
McCarthy et al. (2021) examined changes in reported offending by youth aged 10–17 years old in Queensland, Australia, between periods prior to (January 1, 2018–March 31, 2020) and following (April 1, 2020–June 30, 2020) the introduction of containment measures. Results of Poisson panel analysis with random intercept showed significant decreases in rates of offenses against a person, property offenses, and public order offenses, but not drug-related offenses, following restrictions to movement and gathering as compared to the pre-COVID-19 period. Revital and Haviv (2022) used data on weekly juvenile crime files obtained from Israel Police between January 2019 and December 2020 to explore whether the crime time series changed significantly following the two lockdown periods (March 25–May 4 of 2020 and September 25–October 17 of 2020). Results of autoregressive integrated moving average (ARIMA) models showed a significant reduction in assaults during lockdowns and in drug-related offenses during the COVID-19 pandemic but no significant alteration in robbery and property offenses. The two studies presented inconsistent findings, challenging the generalizability regarding the impact of COVID-19 on youth offending. Thus, additional research is needed to provide a better understanding of this issue. Moreover, the statistical approaches employed by the two aforementioned studies pertaining to youth, and the vast majority of studies for adults, successfully reflected the overall discrepancy between prior to and following the pandemic but failed to represent the dynamic nature of change of offending during the post-transition period. In other words, there is sufficient knowledge about the abrupt impact of the COVID-19 pandemic on offending, but little is known about its enduring impact.
The long-term change of offending following the COVID-19 pandemic
Routine activity theory provides a situational explanation in terms of when and where crime is more likely to occur (Cohen and Felson, 1979), emphasizing the immediate change of crime and delinquent behavior at the onset of the COVID-19 pandemic. Importantly, such an event as the pandemic is not only discrete but also “bounded in time and space” (Morgeson et al., 2015, p. 516). According to the events system theory (EST; Morgeson et al., 2015), COVID-19 stimulated larger chains of subsequent events that affected individual and collective behavior across time. For example, COVID-19 led to school closures and then remote instruction, which in turn caused more interactions between parents and children. These growing interactions could have either strengthened or weakened the bonds of parents with their children (Prime et al., 2020). The strengthened bonds could prohibit delinquency, whereas the weakened bonds could increase delinquency (Hirschi, 1969). This process is presumed to occur in an incremental and gradual manner. Toward this end, the pandemic not only exerted an abrupt but also an enduring impact on offending. In fact, recognizing the abrupt and enduring impact of an event on the change of criminal trajectories is not new (Bersani and Doherty, 2013). From a developmental/life course criminology perspective, for example, turning points such as marriage are so critical for some individuals that they immediately redirect their criminal trajectories, but less so for many others, who instead decrease their offenses gradually through an incremental process (Laub and Sampson, 1993). The long-term change in crime and delinquency in the context of the COVID-19 pandemic has been understudied. In other words, little is known about the developmental trajectory of delinquency following the implementation of containment measures.
Contextual factors and offending during the COVID-19 pandemic
Beyond knowing the extent to which implemented containment measures related to COVID-19 has had effects on the change of offending, a better understanding of contextual factors that may exacerbate or mitigate the disparity of crime trajectories has practical implications. Social disorganization theory serves as a well-accepted theoretical perspective in understanding neighborhood crime (Shaw and McKay, 1942; Kornhauser, 1978; Bursik, 1988; Sampson and Groves, 1989). The theory posits that structural characteristics of neighborhoods—poverty, racial/ethnic heterogeneity, and residential mobility—lead to decreased formal and informal social control, which in turn increases the risk of offending and delinquency.
A growing number of studies have examined the extent to which various structural characteristics contribute to the change of different crime types following the implementation of containment measures (Campedelli et al., 2020; McCarthy et al., 2021; Andresen and Hodgkinson, 2022; Hodgkinson et al., 2022). In general, prior research suggests that the impact of a specific contextual feature on the change of offending depends on offending types. Yet, contradictory findings have also emerged in prior research. In a study based on 77 communities in Chicago, Campedelli et al. (2020) demonstrated that a higher level of poverty was related to a lower likelihood of reduction of burglary as compared to no change or increase, but it had no effect on assault, narcotics, and robbery reduction. In another study based on 362 dissemination areas (i.e., small, relatively stable geographic units composed of one or more dissemination blocks; each block includes 400–700 persons and represents the smallest standard geographic area for which all census data are disseminated) in Saskatoon, Canada, Hodgkinson et al. (2022) registered that areas with higher median family income were more likely to experience no change in commercial burglary, residential burglary, theft of a vehicle, violence, and mischief than experiencing decreases in those crime types during the COVID-19 lockdown. McCarthy et al. (2021) focused on youth offending rates in Queensland, Australia, and observed that containment measures stimulated notably steeper declines in property and public order offenses (but not in offenses against a person or drug-related offenses) in communities with lower than higher socioeconomic status (SES).
Notwithstanding the variations of the three studies in design, units of analysis, and analytical methods, the findings of Campedelli et al. (2020) showed that a higher level of community SES was associated with a higher likelihood of a reduction in crime. In contrast, the findings of Hodgkinson et al. (2022) and McCarthy et al. (2021) showed that a lower level of community SES was related to a higher likelihood of a reduction in crime and delinquency. These inconsistent findings warrant additional research into the effect of community characteristics on crime trajectories during the COVID-19 pandemic.
The current study
Despite substantial efforts devoted to understanding the relationship between the COVID-19 pandemic and offending for adults, less is known about such a phenomenon for youth as there are only limited relevant studies (McCarthy et al., 2021; Revital and Haviv, 2022). The present study aimed to fill in the gaps by (a) investigating the immediate and long-term change in youth offending rates for three types of offenses—offenses against a person, property offenses, and drug-related offenses—among 138 neighborhoods in Harris County, Houston, Texas in the context of COVID-19 and (b) evaluating the extent to which the socioeconomic composition of neighborhoods as indexed by concentrated affluence and poverty accounted for variations of the change of youth offending rates.
Methodologically, the current study features a novel application of the discontinuous growth models, DGM, under the multilevel modeling (MLM) framework to capture the dynamic change of youth offending rates prior to (March 2019–February 2020), at (March 2020), and following (March 2020–February 2021) the implementation of containment measures. Time series analysis and its extensions, the method dominantly employed in existing research related to COVID-19 and crime, address the abrupt change due to the pandemic. However, DGM allows for (a) an evaluation of the trajectory in offending rates prior to the pandemic (i.e., pre-transition slope), (b) determining the extent to which the pandemic produces an immediate change in the offending rates relative to what would have been expected from the pre-transition trajectory (i.e., transition), and (c) comparing the pre-transition trajectory with post-transition trajectory (Bliese et al., 2017). Akin to a one-group pretest-posttest design, modeling the pre-transition slope is essential to establish the baseline and characterize the pretest effect. The transition parameter captures the abrupt impact of the pandemic on offending rates. A contrast between the pre-transition slope and the post-transition slope allows for the evaluation of the enduring impact of the COVID-19 pandemic on youth offending trajectories. The MLM framework takes the dependency (i.e., occasions are nested within neighborhoods) into account while modeling the changes and allows for the investigation of the contextual factors associated with the changes.
Past research has suggested that pleasant temperatures tend to prompt individuals to spend more time outside the home, which increases opportunities for victimization (Hipp et al., 2004). Thus, seasonality is a confounder that needs to be considered while investigating delinquency trends over time (McCarthy et al., 2021). After taking seasonal variations into account, we hypothesized that (a) there would be no systematic change in offenses against a person and property offenses across the 12 months prior to the pandemic, whereas a decreasing trend would be expected for drug-related offenses due to a new policy that Harris County had stopped prosecuting some marijuana possession cases since July 2019 (HPM Digital Team, 2019); (b) in line with McCarthy et al. (2021) there would be an immediate reduction in offenses against a person and property offenses, but no change for drug-related offenses in March 2020; (c) given the lack of empirical evidence regarding the developmental trajectory of offending following the pandemic, we did not offer specific hypotheses for the post-transition slope; yet, we completed a relative explorative study; and (d) the concentration of affluence and poverty would account for neighborhood variations of these pandemic-related changes.
Methods
Data sources
Two data sources were exploited to perform the analyses. Youth delinquency data were provided by Harris County Juvenile Probation Department (HCJPD), Houston, Texas, including all reported delinquent incidents by youth aged 10–17 years that occurred in Harris County from January 2006 to March 2021. For the present study, a subset of offending types was selected, including offenses against a person, property offenses, and drug-related offenses that occurred between March 1, 2019, and February 28, 2021. Offenses against a person include assault, aggravated robbery, robbery, and murder. Property offenses include unauthorized use of a motor vehicle, theft, and burglary. Drug-related offenses include possession or use of illicit drugs. Selecting such a time frame allows the proposed models to capture the dynamic change 1 year prior to and 1 year following the implementation of containment measures.
Harris County is located in greater Houston, Texas, the fourth-largest metropolitan area in the United States (U.S.). The county’s population was 4.73 million, according to the 2021 census (U.S. Census Bureau, 2021). Similar to many other counties in the state, Harris County initially implemented strict containment measures pertaining to schools and non-essential businesses in response to the pandemic and gradually loosened the social restrictions, with schools being to open among the last entities. Governor of Texas, Greg Abbott, issued a disaster proclamation on March 13, 2020, in response to the imminent threat of COVID-19 to all counties in the state of Texas (Exec. Order GA 08, 2020). Schools in the state were ordered to close on March 19, 2020, and remained temporarily closed for the 2019–2020 school year (Exec. Order GA 16, 2020). Students in the Houston Independent School District (HISD), including nearly all youth involved in the current study, began the 2020–2021 school year virtually on September 8, 2020, and continued with remote learning for 6 weeks through October 16, 2020. Starting October 19, 2020, parents had the option to choose remote instruction for the fall semester (through January 29, 2021) or the entire school year (through June 11, 2021) or face-to-face instructions for the entire school year (HISD, 2021). Therefore, youth involved in the current study were influenced by school closures and remote instruction during the 1 year follow-up since the proclamation of the pandemic.
The second data source was the American Community Survey (2015–2019 estimates). This survey provides socioeconomic data from stable geographic units defined by the U.S. Census Bureau designed to reflect U.S. Postal Service zip code boundaries.
Measurement
The dependent variable was the monthly count of youth delinquency recorded by HCJPD within each zip code during the study period. Zip codes were the smallest geographic unit provided in the data. Table 1 presents descriptive statistics of the aggregate offending counts over the 2-year follow-up period for offenses against a person, property offenses, and drug-related offenses based on the 138 neighborhoods involved in the analyses. Figure 1 shows the change of the average observed counts of delinquency across 138 neighborhoods. Youth population is a measure that taps into neighborhood variation in the number of youths between the ages of 10 and 19 (mdn = 4,906, min = 607, max = 22,508).
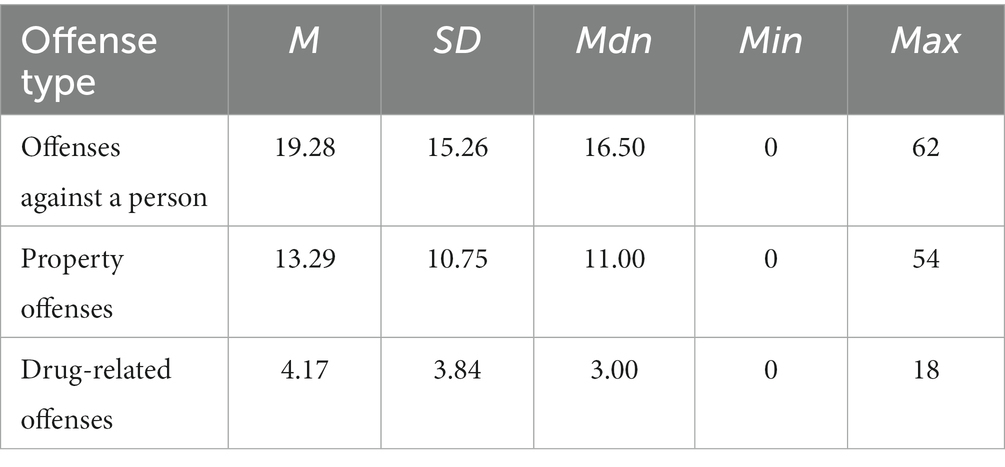
Table 1. Descriptive statistics of monthly offending counts for offenses against a person, property offenses, and drug-related offenses across 138 neighborhoods.
We used the Index of Concentration at the Extremes (ICE) to measure the concentration of affluence and poverty (Krieger et al., 2016). We used the formula ICE = (A – P)/T, where A, P, and T reflect the number of people in each neighborhood belonging to the most privileged group, the least privileged group, and the total population, respectively. Theoretically, ICE scores can range from −1.0 (absolute concentrated poverty) to 1.0 (absolute concentrated affluence), with a 0 indicating that a given neighborhood comprised equally affluent and disadvantaged families. We computed ICE as follows: White, non-Hispanic households with incomes of $100,000 or greater (most privileged) compared to non-White or Hispanic households with incomes of $24,999 or less (least privileged). This race-income measure avoids collinearity problems that arise from including separate measures for each separate component in a single model. The ICE scores ranged from −0.351 to 0.538 (M = 0.045, SD = 0.224) in our sample of 138 neighborhoods.
In order to account for the seasonal effects on the likelihood of youth offending, the average monthly temperature was included as a time-varying covariate (Hipp et al., 2004). Historical temperature data were extracted from Weather Underground (n.d.). To facilitate interpretation, the average monthly temperature was centered at the grand mean (M = 71.79, SD = 10.98).
Analyses
DGM is an extension of the linear growth model where change is described using a discontinuous trajectory with separate slopes through distinct phases of time (Hoffman, 2015). The discontinuity can be achieved through recoding time variables (Bliese et al., 2017). Table 2 shows the coding for time variables involved in the DGM. The first time-varying predictor (TIME) represented the linear change across the 24 months and was centered in March 2019, which was 1 year prior to the occurrence of COVID-19. The second time-varying predictor captures the intercept discontinuity (INTDIS), which was coded 0 prior to the onset of the pandemic (i.e., March 2019–February 2020) and 1 after the onset of the pandemic (i.e., March 2020–February 2021). The third time-varying variable represents the slope discontinuity (SLOPEDIS), which was coded 0 prior to or during the pandemic and coded using consecutive integer values from 0 to 11 for TIMEs 12–23. The three aforementioned time-varying variables, along with seasonality, were identical for all neighborhoods involved in the analyses.
Given the number of aggregate neighborhood delinquency naturally occurring as frequency counts, DGM was estimated under the multilevel generalized linear mixed models (ML-GLMM) framework. The selection of ML-GLMM for a count variable depends on the assumptions of the count response distribution (i.e., underdispersion, equidispersion, and overdispersion). Peugh et al. (2021) recommended identifying the best-fitting ML-GLMM by comparing six different models: Poisson, random intercept Poisson (RIP), negative binomial (NB), zero-inflated Poisson (ZIP), zero-inflated random intercept Poisson (ZI-RIP), and zero-inflated negative binomial (ZI-NB) models. Equations 1–3 represent models sampling count response values for Poisson, RIP, and NB, respectively. Adding Equation 4, a binary logistic regression, to the aforementioned count models (Equations 1–3) led to ZIP, ZI-RIP, and ZI-NB, respectively:
where t is the month in the study, and i is the index of the neighborhood. We noted that where individuals had different exposures (i.e., the youth population size varies across the 138 neighborhoods), an offset (log(Pi), where Pi represents the youth population size for neighborhood i) was added to the count model to account for the expected variation that population size would produce in the observed counts. The fixed effect included indicating the initial intercept in March 2019, representing the estimation of the average linear change per month over the 12 months prior to the onset of the pandemic (March 2020) in the rate of delinquency over time, representing the extent to which the onset of the pandemic produced an immediate change in youth offending rate relative to what would have been expected from the pre-transition trend, specifying the average shift in the monthly change in the rate following the pandemic relative to the pre-change slope ( ), and capturing the seasonal effect. Equations 1–3 also include different numbers of random variances ( , , , , and ) that are used to meet different assumptions for a count response distribution. The inflation model (Equation 4) is essentially a binary logistic model in which models the probability of observing a structural value of zero versus the probability of observing at least one offending count, and the probability is estimated as a logit intercept ( ) controlling for seasonal effect .
Poisson, RIP, NB, ZIP, ZI-RIP, and ZI-NB were fitted to each type of offense data (offenses against a person, property offenses, and drug-related offenses) separately. For each outcome variable, the model with the smallest Bayesian information criterion (BIC) evidenced the best-fitting model and would be retained for interpretation (Peugh et al., 2021). Building upon the unconditional DGM, we investigated the extent to which ICE accounted for variances of , , , , and when possible, . All ML-GLMM were conducted using “glmmTMB” (Brooks et al., 2017) package embedded in the R environment (R Core Team, 2021).
Results
Selecting the best-fitting longitudinal count model
Table 3 presents BIC for the six ML-GLMM. When the proposed models with freely estimated random effects on , , and (the top panel of Table 3), only Poisson and ZIP successfully converged for offenses against a person and property offenses, and all models failed to converge for drug-related offenses. Despite the convergence problems, estimates for the unconditional models with the random-effect variances on , , and were provided in Supplementary Tables S1–S3.
The convergence problem made the estimated parameters unreliable. A common reason behind the convergence problem is model overfitting (Hoffman, 2015), such that redundant random effects are specified. A closer inspection of the results suggests that model convergence problems may be due to the small random-effect variance for (ranging from 0.000 to 0.009 for offenses against a person, from 0.000 to 0.011 for property offenses, and from 0.000 to 0.005 for drug-related offenses) and (ranging from 0.001 to 0.014 for offenses against a person, from 0.000 to 0.016 for property offenses, and from 0.000 to 0.003 for drug-related offenses) across the six models. These trivial variances in the slopes indicate that the developmental trajectories for the three types of delinquency operate in a relatively homogenous way across the neighborhoods.
To that end, we reran the six models separately for each type of delinquency by constraining and to be 0. The bottom panel of Table 3 presents BIC for each estimated DGM model. For offenses against a person, ZINB was the best fitting model (BIC = 7,286). Regarding property offenses, RI-ZIP was the best fitting model (BIC = 6,119). For drug-related offenses, RI-ZIP was the best fitting model (BIC = 2,608). Taken together, results from ZINB for offenses against a person and RI-ZIP for property offenses and drug-related offenses adequately reflected the interrupted effect of the COVID-19 pandemic on youth offending rate trend over the 2 years. Full estimates for the unconditional models with random-effect variance on are presented in Supplementary Tables S4–S6.
The interrupted developmental trajectories of delinquency among youth
Table 4 provides the fixed effects, random effects, and model fit for the best-fitting models for the three types of offenses. The expected count of offenses against a person for a typical neighborhood (the number of population for youth between 10 and 19 years of age is 4,906) in a typical season ( = 0) in March 2019 was 0.933 [ ]. The relative change in the rate of offenses against a person decreased over time during the 1 year period prior to the pandemic by 1.6% [ ]. While holding the seasonal effect constant, the instantaneous decrease in the count of offenses against a person at the onset of the pandemic (i.e., March 2020) when social regulations first came into effect for a typical neighborhood was 0.553 [ ]. A proportional incidence rate ratio (IRR) comparison showed an 28.3% instantaneous decrease in generated IRR of offenses against a person in March 2020 compared to the expected IRR. The expected change in the rate of offenses against a person following the implementation of containment measures did not significantly differ from the change in the rate prior to the COVID-19 pandemic (γ30 = −0.004, p = 0.773), suggesting an absence of long-term change following the pandemic relative to prior to the pandemic.
Figure 2 shows the model predicted count for offenses against a person prior to, at, and following the implementation of containment measures for a typical neighborhood after taking seasonal effect into account. The model predicted count for offenses against a person was 0.870, 0.862, 0.456, 0.444, and 0.414 in January, February, March, April, and May of 2020, indicating that the onset of the COVID-19 pandemic dramatically reduced the count of offenses against a person. The figure also emphasized the seasonal effect on offenses against a person such that individuals were more likely to commit offenses against a person during spring and winter when the temperature is moderate and pleasant rather than summer and fall in Houston when the temperature is high and unpleasant.
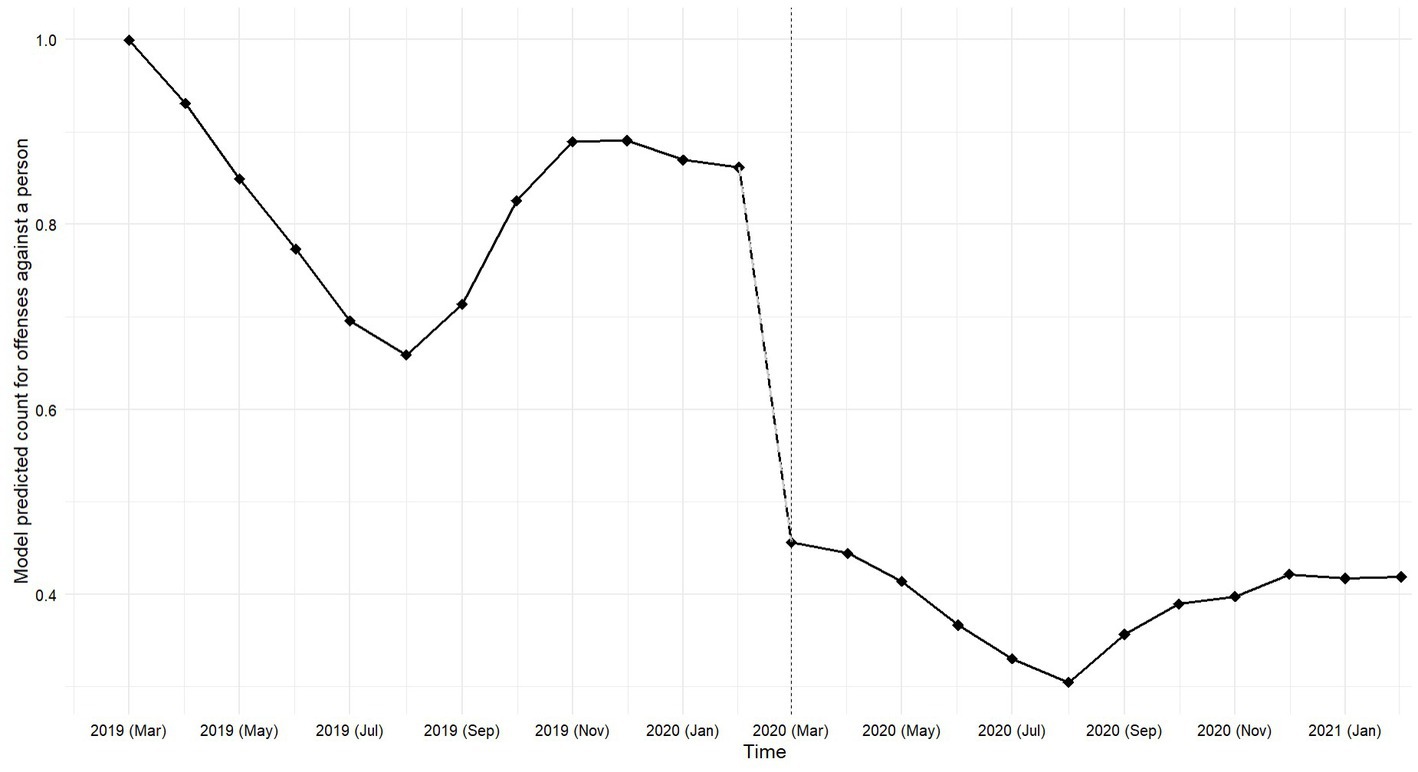
Figure 2. Model predicted counts for offenses against a person prior to, at, and following the COVID-19 pandemic by taking seasonality into account. The X-axis represents months prior to (March 2019 to February 2020), at (March 2020, the vertical dashed line), and following (April 2020 to February 2021) the school closures. Y-axis represents the model predicted mean count. The dashed vertical line indicates the month when containment measures started to be implemented. The dashed oblique line highlights the abrupt jump in offense count between 1 month before the implementation of the containment measures and the month after implementing these containment measures.
The expected count for property offenses for a typical neighborhood in a typical season in March 2019, was 0.600 [ ]. The pre-pandemic change in the rate of property offenses was effectively 0 (b = −0.009, p = 0.349), indicating that the rate of property offenses did not change across the 12 months prior to the onset of the pandemic. The expected count of property offenses at the onset of the pandemic (i.e., March 2020) dropped to 0.421 [ , suggesting an immediate reduction. A proportional IRR comparison showed a 21.9% [ ] instantaneous decrease in the generated rate of property offenses than the expected rate. Following the onset of the pandemic, the expected property offenses rate decreased by 6.0% ] per month, on average. Compared to the fluctuation of property offenses 1 year prior to the pandemic, the systematic and long-term decrease of property offenses 1 year following the pandemic indicates the enduring impact of the pandemic on property offenses.
Figure 3 shows the model predicted count of property offenses prior to, at, and following the COVID-19 pandemic for a typical neighborhood after taking seasonal effect into account. A statistically significant downfall in property offenses occurred between February and March 2020. In addition, the count of property offenses fluctuated prior to the onset of the pandemic, whereas there was a decreasing trend over time following the pandemic. Interestingly, there was no pattern in terms of the change echoing the insignificant seasonal effect for property offenses.
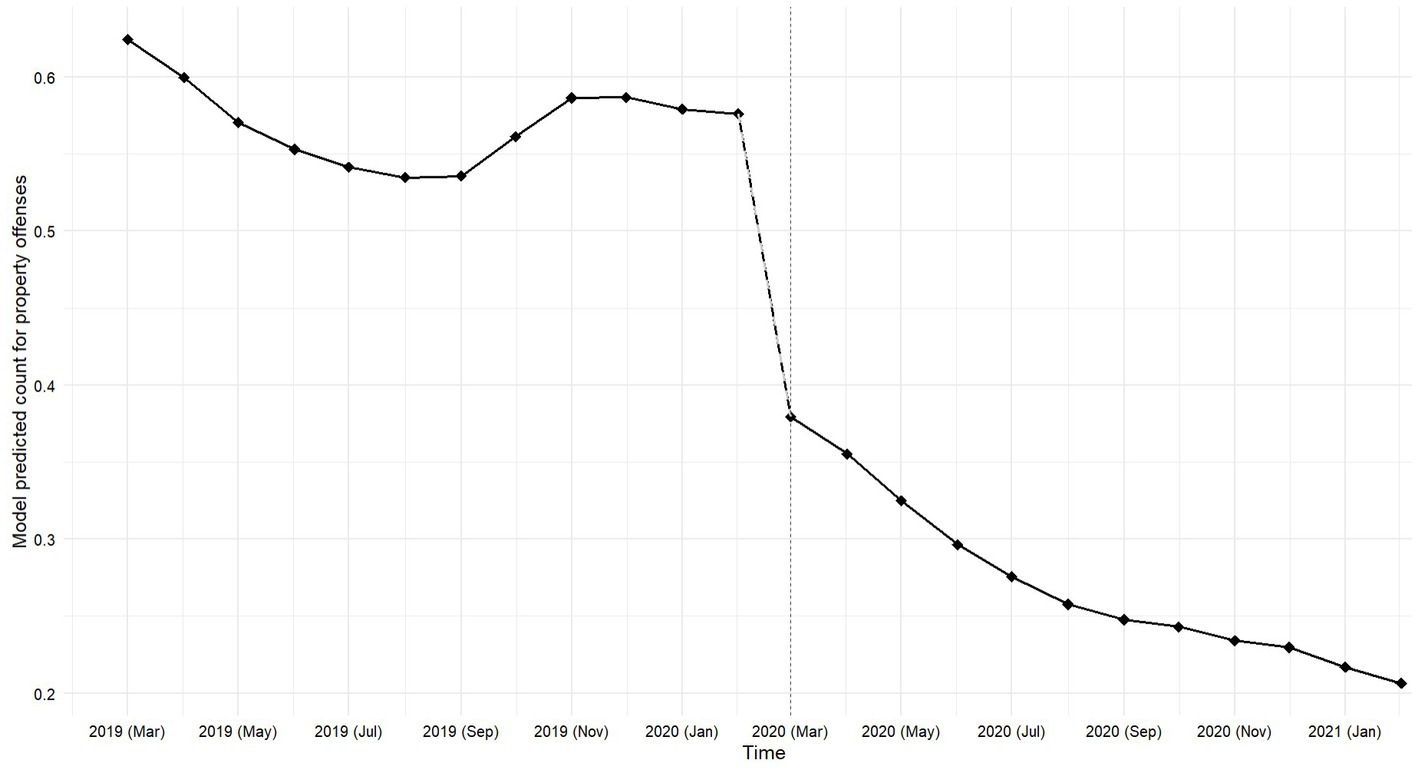
Figure 3. Model predicted count for property offenses prior to, at, and following the COVID-19 pandemic by taking seasonality into account. The X-axis represents months prior to (April 2019 to February 2020), at (March 2020, the vertical dashed line), and following (April 2020 to February 2021) the school closures. Y-axis represents the model predicted mean count. The dashed vertical line indicates the month when containment measures started to be implemented. The dashed oblique line highlights the abrupt jump in offense count between 1 month before the implementation of the containment measures and the month after implementing these containment measures.
Regarding drug-related offenses, the expected count of drug-related offenses for a typical neighborhood in a typical season in March 2019 was 0.810 [ ]. The expected rate of drug-related offenses decreased over the 12-month period prior to the onset of the pandemic by 21.3% [ ] per month, on average. When the pandemic hit, the generated rate of drug-related offenses was not significantly different from the expected rate (γ30 = 0.404, p = 0.102), suggesting a lack of immediate change. Following the COVID-19 pandemic, the expected property offenses rate decreased by 5.6% ] per month, on average, suggesting that the decreasing trend following the pandemic became much less steep compared to the declining trend prior to the pandemic.
Figure 4 clearly shows the noticeable decline in the average count of drug-related offenses 1 year prior to the onset of the pandemic, whereas the decreasing change became significantly less steep following the pandemic as compared to prior to the pandemic. Importantly, there was no significant shift to the count in March 2020 when the pandemic hit. It should be noted that although the season had no effect on the count of drug-related offenses, it was associated with the likelihood of having no drug-related offenses versus more than one drug-related offense in any month (π10 = 0.345, p < 0.001).
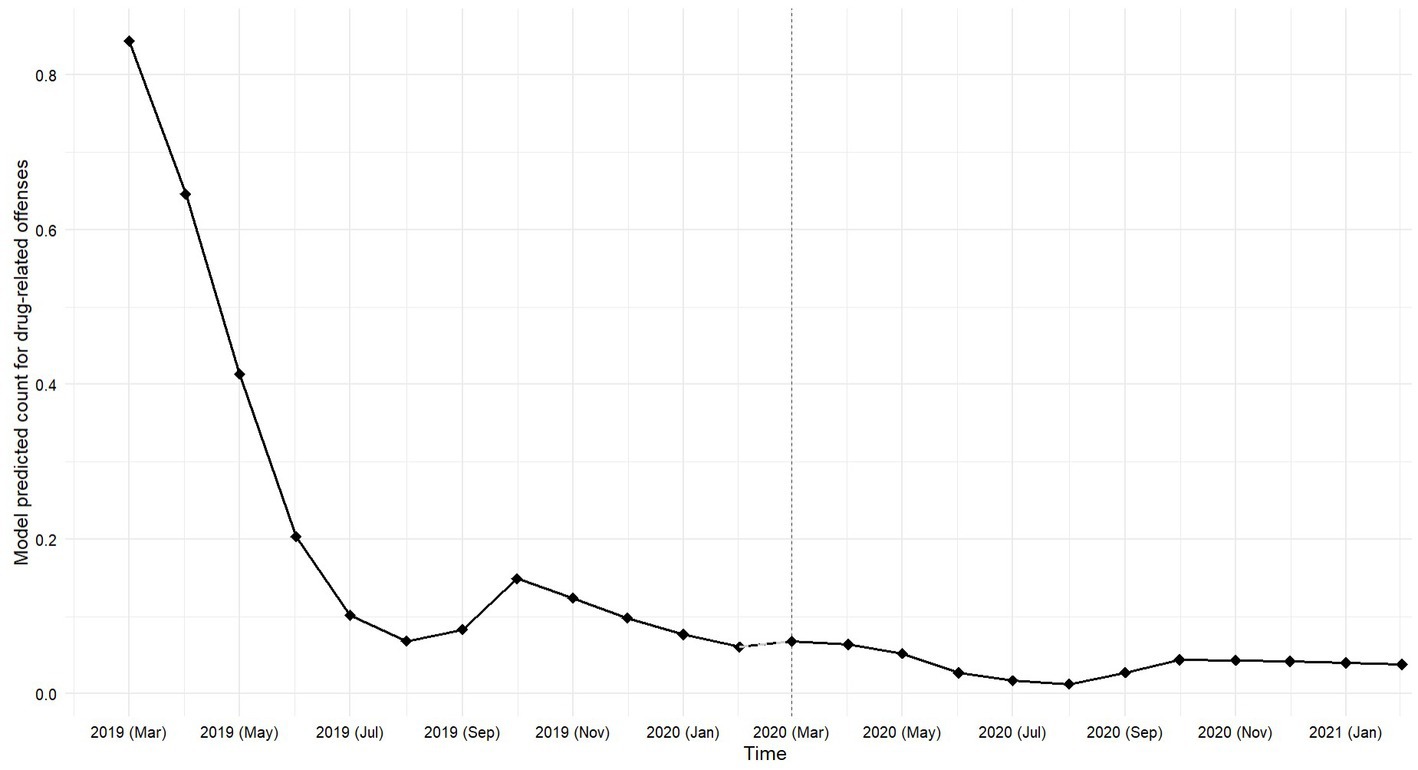
Figure 4. Model predicted count for drug-related offenses prior to, at, and following the COVID-19 pandemic by taking seasonality into account. The X-axis represents months prior to (April 2019 to February 2020), at (March 2020, the vertical dashed line), and following (April 2020 to February 2021) the school closures. Y-axis represents the model predicted mean count. The dashed vertical line indicates the month when containment measures started to be implemented. The dashed oblique line highlights the abrupt jump in offense count between 1 month before the implementation of the containment measures and the month after implementing these containment measures.
The effect of concentrated affluence and poverty on the variation of the discontinuity of delinquency
After establishing the interrupted developmental trajectories for the three types of delinquency (ZINB for offenses against a person and RI-ZIP for property offenses and drug-related offenses), we proceeded to evaluating the extent to which ICE accounted for the random-effect variance on (i.e., ). Note that the associations of ICE with and were not evaluated, because they were constrained to be 0 as illustrated above.
The conditional count model for offenses against a person was as follows:
The conditional count model for property offenses and drug-related offenses was
The inflation model was
Table 5 presents results pertaining to the effect of ICE on the variation of the initial intercept and the discontinuity of the intercept. Yet, the three conditional models had convergence problems, suggesting that estimates may not be reliable. Sensitivity analyses reveal that the convergence problems trivially distort the key estimates— and Despite the heterogeneity in terms of BIC among the six unconditional models for each type of delinquency (Supplementary Tables S4–S6), we developed corresponding conditional models for sensitivity analyses. Supplementary Tables S7–S9 present the results. It appears that the direction of the estimates of interest— and —was all the same and differences of estimates occur at the first decimal place. If making a dichotomous reject-nonrejected decision, the six conditional models for each type of delinquency would lead to the same conclusion: a higher ICE was related to a lower initial intercept, and ICE was not related to discontinuity. Thus, to be consistent with the unconditional models presented in Table 4, we presented estimates derived from the corresponding conditional models in Table 5, though with convergence problems.
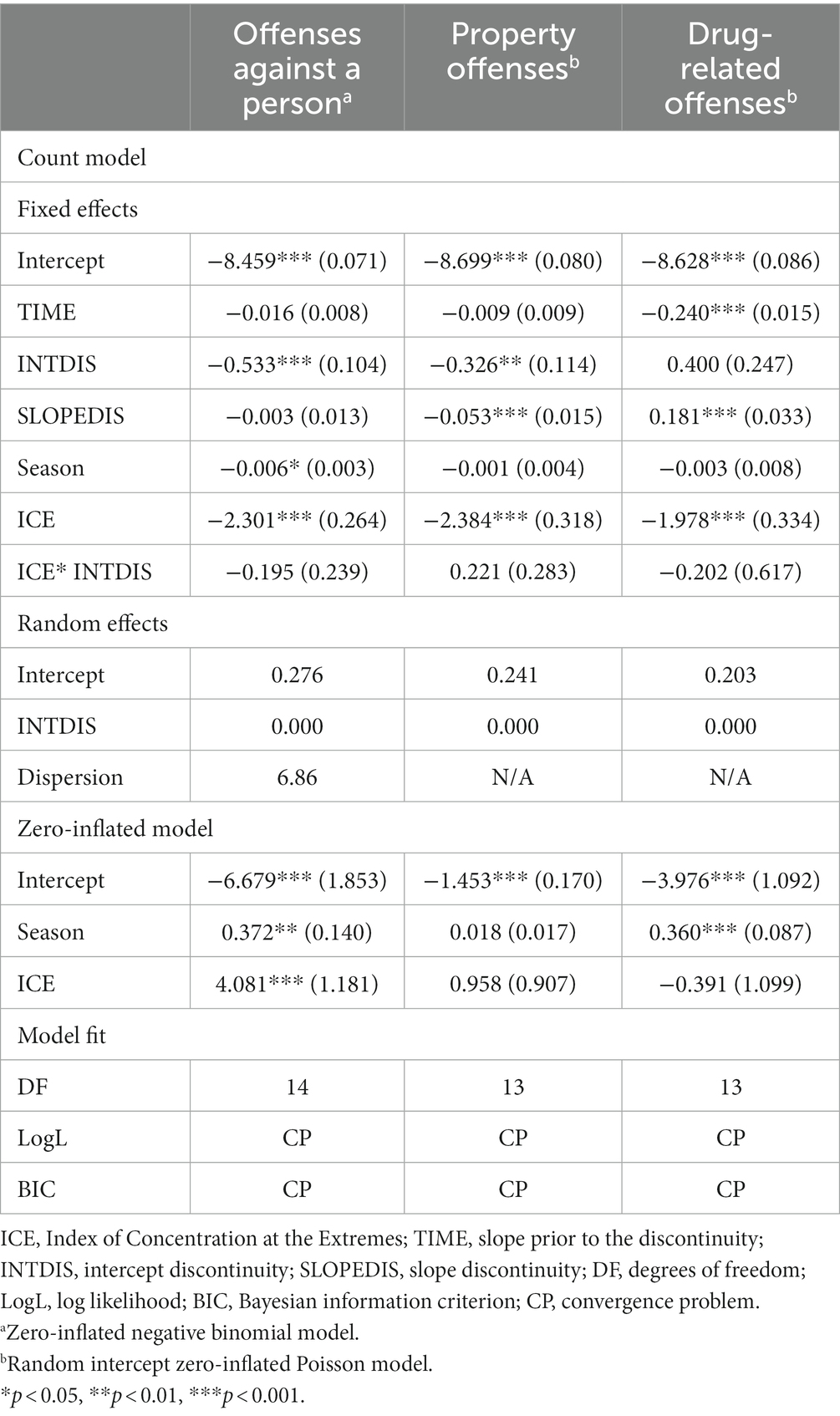
Table 5. Conditional discontinuous growth models for accounting for variations in the discontinuity by concentrated affluence and poverty.
For the three types of offenses, a higher ICE was related to a lower initial intercept (γ01 = −2.301, p < 0.001 for offenses against a person; γ01 = −2.384, p < 0.001 for property offenses; γ01 = −1.978, p < 0.001 for drug-related offenses). In March 2019 (1 year prior to the pandemic), the expected count of offenses against a person for a typical neighborhood, for example, was 0.110 [ ], 1.098 [ ], and 10.959 [ ] for neighborhoods with absolute concentrated affluence (ICE = 1), with equally affluent and disadvantaged families (ICE = 0), and absolute concentrated disadvantaged families (ICE = −1), respectively. Neighborhoods with absolute concentrated disadvantage had a rate of offense against a person 8.982 ( ) and 98.627 ( ) times greater than neighborhoods with equally affluent and disadvantaged families and absolute concentrated affluence, respectively, 1 year prior to the onset of the COVID-19 pandemic. However, ICE was not significantly associated with the shift of the intercept for offenses against a person (γ21 = −0.195, p = 0.415), property offenses (γ21 = 0.221, p = 0.434), and drug-related offenses (γ21 = −0.202, p = 0.744).
Discussion
The present study advances the literature by providing insight into (a) the immediate and long-term change of youth delinquency in offenses against a person, property offenses, and drug-related offenses in the context of COVID-19 and (b) the effects of concentrated affluence and poverty on the interrupted developmental trajectories of delinquency. By applying the rigorous DGM under the ML-GLMM framework, we found that (1) the onset of the COVID-19 pandemic was associated with an immediate reduction in offenses against a person and property offenses but not in drug-related offenses; (2) the post-transition trends for property offenses and drug-related offenses were significantly different from their corresponding pre-transition trends; (3) the pre-transition trends and the relative post-transition trends for the three types of delinquency were relatively homogenous across the neighborhoods; and (4) ICE did not account for variations of the immediate change for the three offending types.
The abrupt impact of the COVID-19 pandemic on youth delinquency
In line with prior research based on a sample of youth in Australia (McCarthy et al., 2021), we found an immediate decrease in offenses against a person and property offenses, but not drug-related offenses. Revital and Haviv (2022), however, reported a significant reduction in assaults during lockdowns and in drug-related offenses during the pandemic, whereas property offenses remained unchanged. The inconsistent findings are difficult to reconcile due to substantial variations in operationalizing crime types, jurisdictions, and follow-up periods. Nevertheless, in combination with existing research for youth (McCarthy et al., 2021; Revital and Haviv, 2022) and adults (e.g., Felson et al., 2020; Mohler et al., 2020; Nivette et al., 2021), the present study demonstrates that the altered routine activities caused by the COVID-19 pandemic exerted an abrupt impact on crime and delinquent behavior.
Stay-at-home restrictions, social distancing, school closures, remote instruction, and barring gatherings, among others, largely reshaped the patterns of social connection and movement for youth. Parents had to structure the ordinary activities of everyday life for their children across all school years. For example, parents had to ensure their children attended virtual classes on time. Parents also had to consider and support their children’s activities in their spare time. According to the U.S. Census Bureau’s 2020 Survey of Income and Program Participation, for example, 69% of parents reported reading to young children five or more times per week, compared with 65% in 2018 and 64% in 2019 (Mayol-Garcia, 2022). Importantly, the presence of parents at home per se has been claimed to be a primary factor in shrinking delinquency opportunities for youth due to increased social control and censorship (Osgood et al., 1996; Hawdon, 1999).
Moreover, the conventional face-to-face interactions between youth that commonly happen in school buildings and other elements of the built environment were largely replaced with virtual communications following the implementation of containment measures. Juvenile delinquency is primarily a group phenomenon, as being accompanied by adolescent peers makes deviance easier and more rewarding (Sampson and Groves, 1989; Osgood et al., 1996). In the context of COVID-19, however, the reduced in-person social interactions between youth decreased the situational potential for deviance by making delinquency more challenging and devaluing the symbolic and tangible rewards of committing shared delinquent behavior (Osgood et al., 1996). Moreover, the prohibition of private and public gatherings and closures of non-essential businesses and entertainment significantly reduced the number of potential victims.
Thus, the immediate reduction in offenses against a person and property offenses can be partially attributable to (a) the presence of guardianship, (b) the inhibited motivation to conduct delinquency due to the drastic reduction of face-to-face interactions with peers, and (c) the lack of access to victims (i.e., less opportunity for victimization) caused by remote instructions. It should be noted that the impact of various social restrictions on the immediate change of the youth offending count is likely to involve more complicated mechanisms than the ones we proposed here. For instance, it is unclear whether the absence of peers, the reduced exposure to potential victims, the presence of authoritative figures, and structured activities contribute to the change in a linear or nonlinear manner.
The enduring impact of the COVID-19 pandemic on youth delinquency
While existing research exploiting time series analysis and its extensions have emphasized the abrupt change of offenses during the pandemic, the DGM approach used in the current study offered insight into the enduring impact of the COVID-19 pandemic on youth delinquency. This was achieved by comparing the post-transition slope (the change following the pandemic) with the pre-transition slope (the change prior to the pandemic). As a baseline, youth delinquency rates were assumed to fluctuate over time without demonstrating any systematic change after controlling for the seasonal variants prior to the acute event occurring (i.e., March 2020). This assumption was supported for property offenses. Despite the declining pre-transition slope for offenses against a person, its changing rate was trivial (1.6%). Regarding drug-related offenses, Harris County initiated a policy that the District Attorney’s office did not prosecute some possession of marijuana cases (personal use—less than 2 oz) in July 2019, and since then, those cases have no longer been tracked by HCJPD. The systematic declining trend for drug-related offenses prior to the pandemic (pre-transition slope) confounded this drug-related policy, so it failed to establish a plausible baseline to be compared with the post-transition slope. Thus, interpreting the relative change of the post-transition slope compared to the pre-transition slope for drug-related offenses is not as robust as for offenses against a person and property offenses.
We observed a steeper decline in property offenses following the pandemic as compared to the trend 1 year prior to the pandemic, implying, perhaps, an enduring impact of the COVID-19 pandemic on youth delinquency. In the context of COVID-19, parents or caregivers faced unprecedented financial stressors resulting from unemployment and recession, which drained their mental and emotional resources. In order to negotiate such an unprecedented challenge, the disruption caused by the pandemic might trigger the resilience mechanisms in family dynamics, including clear information, enhanced emotional sharing, collaborative problem-solving, and heightened hope (Prime et al., 2020). It is presumable that such family resilience in response to the pandemic was formulated in an accumulating and gradual manner rather than an acute manner. The intact family dynamics have the potential to enhance child attachment to their parents, which is hypothesized to impede delinquent behavior (Hirschi, 1969). The enduring impact of the pandemic on the long-term decline of property offenses highlighted the process-oriented nature of the pandemic. According to EST (Morgeson et al., 2015), COVID-19 presumably triggered multilayer paths of subsequent events, whereby some would result in a positive change of behavior (e.g., family resilience), whereas others would result in a negative change of behavior (e.g., coercive and harsh parenting), depending on the features of these subsequent events. The steeper declining trend for property offenses may reveal that the positive consequences outweigh the negative consequences stimulated by the event in determining this type of delinquency.
Regarding offenses against a person, the pre-transition and post-transition slopes paralleled, suggesting that the pandemic did not exert an extra and enduring impact on offenses against a person. Following the reasoning elaborated above, the lack of long-term change in offenses against a person may be interpreted as the result of a combined effect of strengthened bonds (e.g., family resilience), on the one hand, and worsened bonds (e.g., unhealthy parent–child relationship caused by problematic forms of parenting while parents faced the unprecedented strain) on the other, within the family. A competing interpretation is that the COVID-19 pandemic may not stimulate chains of subsequent events that are strong enough to influence violent behavior. Indeed, violent behavior, including offenses against a person, typically has a strong continuity between childhood, adolescence, and adult life (Rappaport and Thomas, 2004). To that end, criminal propensities such as early initiation of delinquency, low self-control, parental criminality, mental disorders, and child maltreatment (Hawkins et al., 1998) are presumably more relevant to youth violence than the cumulative yet gradual change of individual and collective behavior triggered by the pandemic. Considering the heterogeneity regarding the enduring impact of the COVID-19 pandemic on offenses against a person and property offenses suggests that the mechanisms through which the pandemic affected youth offending depended on the type of delinquency.
The concentration of affluence and poverty and youth delinquency during the COVID-19 pandemic
We found that the concentration of affluence and poverty, as indexed by ICE, was associated with lower initial delinquency rates (counts in March 2019, 1 year prior to the pandemic) for offenses against a person, property offenses, and drug-related offenses. This finding is partially consistent with McCarthy et al. (2021), whereby they found that community SES (a composite of employment, education, welfare payments, and housing) was associated with lower initial intercept for offenses against a person and property offenses but not illicit drug offenses. These findings together imply that low SES communities are characterized by a relatively poor ability to supervise and control teenage peer groups, which in turn may increase the opportunities for committing crime and delinquency (Sampson and Groves, 1989).
Consistent with McCarthy et al. (2021), we observed that ICE was not associated with the immediate reduction in offenses against a person and drug-related offenses. Although McCarthy et al. (2021) reported that the pandemic mitigated the rate of property offending such that the disparity of property offenses between low and high SES communities narrowed down following the pandemic, our findings showed that the pandemic was not associated with the immediate reduction in property offenses. One explanation of the discrepant findings centers around the power and sample size. Moderation for ML-GLMM is notoriously underpowered to detect significant effects (Peugh et al., 2021). There were 138 neighborhoods included in the current study as compared to more than 500 communities involved in McCarthy et al. (2021). Another explanation of the discrepancy pertains to the conceptualization of reduction. For McCarthy et al. (2021), the reduction in property offenses referred to the difference between the average offending count from April and June 2020 and the average offending count from January 2018 to March 2020. However, we focused on the immediate reduction in March 2020. Thus, the different ways of quantifying the reduction make comparable interpretations challenging.
Beyond the methodological and conceptual considerations, the findings that ICE did not account for variations in the immediate reduction in the three types of delinquency may be a consequence of the nature of ICE. According to the social disorganization theory (Sampson and Groves, 1989), neighborhood contexts, including poverty, racial/ethnic heterogeneity, and residential mobility, influence crime and delinquency indirectly through informal and formal social control. In other words, ICE is hypothesized to play a role in delinquency through social control. To that end, the influence of ICE on youth delinquency is arguably weaker than the influence of social control. It is possible that neighborhood social control contributes to the variation instead of the manifest concentration of affluence and poverty. Thus, it is worthwhile for the future to consider social control while investigating the extent to which community characteristics influence the abrupt reduction of delinquency during the pandemic.
Limitations and future directions
There are several limitations of the current study that need to be noted. First, the results reported here are specific to the jurisdiction in Harris County, so it may not generalize to other jurisdictions in the U.S. In fact, a consistent finding derived from existing research is that the impact of the pandemic on crime and delinquency varies as a function of jurisdictions and regions (e.g., Ashby, 2020). Second, given the related lack of precision and suppressed reflection of variability within and between the county’s neighborhoods, using zip codes as indicators of SES is another limitation.
Third, it is important to note that delinquency rates are susceptible to police activity and enforcement. Nielson et al. (2022) examined the impact of COVID-19 on police reactive and proactive activities in Houston, Texas. For police reactive activities, police response to violent crime calls significantly increased after COVID-19. Police response to traffic-related calls, property crime, and service-related calls all decreased significantly in the weeks after the announcement of the COVID-19 pandemic. Officers were engaged in more frequent self-initiated patrol activities compared to the pre-pandemic data. Thus, future research needs to take police activity into consideration while evaluating the association between the pandemic and offending in order to obtain robust estimates.
Fourth, given its easy accessibility for scholars, official records have been the dominant data sources when studying the interrupted effect of the pandemic on crime and delinquency; however, such records have been stated to reflect the tip of the iceberg for delinquent behavior because most of such behaviors are unregistered by law enforcement (Moffitt, 1993). Future research utilizing self-report data can complement existing evidence about the pandemic and offending based on recorded crime and delinquency.
Conclusion
We conclude that the COVID-19 pandemic not only had an abrupt but also an enduring impact on juvenile delinquency. The sudden alternation of routine activities was related to the immediate reduction of delinquency in offenses against a person and property offenses at the onset of the pandemic. Meanwhile, subsequent events triggered by the pandemic were associated with the systematic declining trend in property offenses. Although the concentration of affluence and poverty did not mitigate the disparity regarding the immediate reduction of offenses against a person and property offenses across neighborhoods, there was no evidence showing that it exacerbated the disparity. Beyond that, we call for future research to redirect the focus from whether the COVID-19 pandemic had influenced criminal and delinquent behavior to how the impact had occurred. Without gaining an insight into the mechanisms behind the change in offending during the pandemic, all interpretations and implications accumulated from the large body of existing research, as well as the current study, are deducted based on criminological theories. Such a deduction, without further data-based verification, undermines the validity of the implications. Thus, it is imperative for future research to collect retrospective data characterizing the social, emotional, and psychological characteristics of youth, parents, peers, and teachers and investigate how the changes in conventional activities contributed to the change in criminal and delinquent behavior. A better understanding of the mechanisms behind the abrupt and enduring effects of the pandemic on offending would solidify existing studies and improve the precision of designing programs to inhibit criminal and delinquent involvement.
Data availability statement
The raw data supporting the conclusions of this article will be made available by the authors, without undue reservation.
Ethics statement
The studies involving human participants were reviewed and approved by the University of Houston Committee for the Protection of Human Subjects Harris County Juvenile Probation Department. Written informed consent from the participants’ legal guardian/next of kin was not required to participate in this study in accordance with the national legislation and the institutional requirements.
Author contributions
NL and EG contributed to the conception and design of the study and drafted the manuscript. DQ and MS provided the data. NL analyzed the data. All authors have made a substantial, direct, and intellectual contribution to the work and approved it for publication.
Funding
This research was supported by the Eunice Kennedy Shriver National Institute of Child Health and Development (P20HD091005; PI: EG).
Acknowledgments
We thank Ioannis Konstantinidis and Dan Price for their contributions in extracting relevant data from the American Community Survey, and Lauren Elderton for her editorial assistance with this manuscript.
Conflict of interest
The authors declare that the research was conducted in the absence of any commercial or financial relationships that could be construed as a potential conflict of interest.
Publisher’s note
All claims expressed in this article are solely those of the authors and do not necessarily represent those of their affiliated organizations, or those of the publisher, the editors and the reviewers. Any product that may be evaluated in this article, or claim that may be made by its manufacturer, is not guaranteed or endorsed by the publisher.
Supplementary material
The Supplementary material for this article can be found online at: https://www.frontiersin.org/articles/10.3389/feduc.2023.1007807/full#supplementary-material
References
Andresen, M. A., and Hodgkinson, T. (2022). In a world called catastrophe: The impact of COVID-19 on neighbourhood level crime in Vancouver, Canada. J. Exp. Criminol. Advance online publication. doi: 10.1007/s11292-021-09495-6
Ashby, M. P. J. (2020). Initial evidence on the relationship between the coronavirus pandemic and crime in the United States. Crime Sci. 9, 1–16. doi: 10.1186/s40163-020-00117-6
Bersani, B. E., and Doherty, E. E. (2013). When the ties that bind unwind: Examining the enduring and situational processes of change behind the marriage effect. Criminology 51, 399–433. doi: 10.1111/1745-9125.12008
Bliese, P. D., Adler, A. B., and Flynn, P. J. (2017). Transition processes: A review and synthesis integrating methods and theory. Annu. Rev. Organ. Psych. Organ. Behav. 4, 263–286. doi: 10.1146/annurev-orgpsych-032516-113213
Brooks, M. E., Kristensen, K., Van Benthem, K. J., Magnusson, A., Berg, C. W., Nielsen, A., et al. (2017). GlmmTMB balances speed and flexibility among packages for zero-inflated generalized linear mixed modeling. R J. 9, 378–400. doi: 10.3929/ethz-b-000240890
Buchanan, M., Castro, E. D., Kushner, M., and Krohn, M. D. (2020). It’s F** ing Chaos: COVID-19’s impact on juvenile delinquency and juvenile justice. Am. J. Crim. Jus. 45, 578–600. doi: 10.1007/s12103-020-09549-x
Bursik, R. J. (1988). Social disorganization and theories of crime and delinquency: Problems and prospects. Criminology 26, 519–552. doi: 10.1111/j.1745-9125.1988.tb00854.x
Campedelli, G. M., Aziani, A., and Favarin, S. (2021). Exploring the immediate effects of COVID-19 containment policies on crime: An empirical analysis of the short-term aftermath in Los Angeles. Am. J. Crim. Just. 46, 704–727. doi: 10.1007/s12103-020-09578-6
Campedelli, G. M., Favarin, S., Aziani, A., and Piquero, A. R. (2020). Disentangling community-level changes in crime trends during the COVID-19 pandemic in Chicago. Crime Sci. 9:21. doi: 10.1186/s40163-020-00131-8
Cohen, L. E., and Felson, M. (1979). Social change and crime rate trends: A routine activity approach. Am. Sociol. Rev. 44, 588–608. doi: 10.2307/2094589
Felson, M., Jiang, S., and Xu, Y. (2020). Routine activity effects of the Covid-19 pandemic on burglary in Detroit, March, 2020. Crime Sci. 9:10. doi: 10.1186/s40163-020-00120-x
Gerell, M., Kardell, J., and Kindgren, J. (2020). Minor covid-19 association with crime in Sweden. Crime Sci. 9:19. doi: 10.1186/s40163-020-00128-3
Halford, E., Dixon, A., Farrell, G., Malleson, N., and Tilley, N. (2020). Crime and coronavirus: Social distancing, lockdown, and the mobility elasticity of crime. Crime Sci. 9:11. doi: 10.1186/s40163-020-00121-w
Hawdon, J. E. (1999). Daily routines and crime: Using routine activities as measures of Hirschi’s involvement. Youth Soc. 30, 395–415. doi: 10.1177/0044118X99030004001
Hawkins, J. D., Herrenkohl, T., Farrington, D. P., Brewer, D., Catalano, R. F., and Harachi, T. W. (1998). “A review of predictors of youth violence” in Serious and violent juvenile offenders: Risk factors and successful interventions. eds. R. Loeber and D. P. Farrington (Thousand Oaks, California: Sage Publications), 106–146.
Hipp, J. R., Curran, P. J., Bollen, K. A., and Bauer, D. J. (2004). Crimes of opportunity or crimes of emotion? Testing two explanations of seasonal change in crime. Soc. Forces 82, 1333–1372. doi: 10.1353/sof.2004.0074
HISD (2021). HISD to reconnect safely, return strong for 2020–2021 school year. Available at: https://blogs.houstonisd.org/news/2020/07/15/hisd-to-reconnect-safely-return-strong-for-2020-2021-school-year/
Hodgkinson, T., and Andresen, M. A. (2020). Show me a man or a woman alone and I’ll show you a saint: Changes in the frequency of criminal incidents during the COVID-19 pandemic. J. Crim. Just. 69:101706. doi: 10.1016/j.jcrimjus.2020.101706
Hodgkinson, T., Andresen, M. A., Frank, R., and Pringle, D. (2022). Crime down in the Paris of the prairies: Spatial effects of COVID-19 and crime during lockdown in Saskatoon, Canada. J. Crim. Just. 78:101881. doi: 10.1016/j.jcrimjus.2022.101881
Hoffman, L. (2015). Longitudinal analysis: Modeling within-person fluctuation and change. New York, NY: Routledge.
HPM Digital Team . (2019). Harris County will stop prosecuting some marijuana possession cases. Available at: https://www.houstonpublicmedia.org/articles/news/2019/07/02/338502/da-kim-ogg-announces-change-on-charges-for-misdemeanor-possession-of-marijuana/
Koppel, S., Capellan, J. A., and Sharp, J. (2022). Disentangling the impact of Covid-19: An interrupted time series analysis of crime in New York City. Am. J. Crim. Just. Advance online publication. doi: 10.1007/s12103-021-09666-1
Krieger, N., Waterman, P. D., Spasojevic, J., Li, W., Maduro, G., and Van Wye, G. (2016). Public health monitoring of privilege and deprivation with the index of concentration at the extremes. Am. J. Public Health 106, 256–263. doi: 10.2105/AJPH.2015.302955
Langton, S., Dixon, A., and Farrell, G. (2021). Six months in: Pandemic crime trends in England and Wales. Crime Sci. 10:6. doi: 10.1186/s40163-021-00142-z
Laub, J. H., and Sampson, R. J. (1993). Turning points in the life course: Why change matters to the study of crime. Criminology 31, 301–325. doi: 10.1111/j.1745-9125.1993.tb01132.x
Mayol-Garcia, Y. (2022). Pandemic brought parents and children closer: More family dinners, more reading to young children. Available at: https://www.census.gov/library/stories/2022/01/parents-and-children-interacted-more-during-covid-19.html
McCarthy, M., Homel, J., Ogilvie, J., and Allard, T. (2021). Initial impacts of COVID-19 on youth offending: An exploration of differences across communities. J. Criminol. 54, 323–343. doi: 10.1177/00048658211005816
Moffitt, T. E. (1993). Adolescence-limited and life-course-persistent antisocial behavior: A developmental taxonomy. Psychol. Rev. 100, 674–701. doi: 10.1037/0033-295X.100.4.674
Mohler, G., Bertozzi, A. L., Carter, J., Short, M. B., Sledge, D., Tita, G. E., et al. (2020). Impact of social distancing during COVID-19 pandemic on crime in Los Angeles and Indianapolis. J. Crim. Just. 68:101692. doi: 10.1016/j.jcrimjus.2020.101692
Morgeson, F. P., Mitchell, T. R., and Liu, D. (2015). Event system theory: An event-oriented approach to the organizational sciences. Acad. Manag. Rev. 40, 515–537. doi: 10.5465/amr.2012.0099
Nielson, K. R., Zhang, Y., and Ingram, J. R. (2022). The impact of COVID-19 on police officer activities. J. Crim. Just. 82:101943. doi: 10.1016/j.jcrimjus.2022.101943
Nivette, A. E., Zahnow, R., Aguilar, R., Ahven, A., Amram, S., and Ariel, B. (2021). A global analysis of the impact of COVID-19 stay-at-home restrictions on crime. Nat. Hum. Behav. 5, 868–877. doi: 10.1038/s41562-021-01139-z
Osgood, D. W., Wilson, J. K., Omalley, P. M., Bachman, J. G., and Johnston, L. D. (1996). Routine activities and individual deviant behavior. Am. Sociol. Rev. 61, 635–655. doi: 10.2307/2096397
Payne, J. L., Morgan, A., and Piquero, A. R. (2021). Exploring regional variability in the short-term impact of COVID-19 on property crime in Queensland, Australia. Crime Sci. 10:7. doi: 10.1186/s40163-020-00136-3
Payne, J. L., Morgan, A., and Piquero, A. R. (2022). COVID-19 and social distancing measures in Queensland, Australia, are associated with short-term decreases in recorded violent crime. J. Exp. Criminol. 18, 89–113. doi: 10.1007/s11292-020-09441-y
Peugh, J. L., Beal, S. J., McGrady, M. E., Toland, M. D., and Mara, C. (2021). Analyzing discontinuities in longitudinal count data: A multilevel generalized linear mixed model. Psychol. Methods 26, 375–397. doi: 10.1037/met0000347
Prime, H., Wade, M., and Browne, D. T. (2020). Risk and resilience in family well-being during the COVID-19 pandemic. Am. Psychol. 75, 631–643. doi: 10.1037/amp0000660
R Core Team (2021). R: A language and environment for statistical computing. In R foundation for statistical computing. Available at: https://www.R-project.org/
Rappaport, N., and Thomas, C. (2004). Recent research findings on aggressive and violent behavior in youth: Implications for clinical assessment and intervention. J. Adolesc. Health 35, 260–277. doi: 10.1016/j.jadohealth.2003.10.009
Revital, S. S., and Haviv, N. (2022). Juvenile delinquency and COVID-19: The effect of social distancing restrictions on juvenile crime rates in Israel. J. Exp. Criminol. Advance online publication. doi: 10.1007/s11292-022-09509-x
Sampson, R. J., and Groves, W. B. (1989). Community structure and crime: Testing social-disorganization theory. Am. J. Sociol. 94, 774–802. doi: 10.1086/229068
Shaw, C. R., and McKay, H. D. (1942). Juvenile delinquency in urban areas. Chicago, IL: University of Chicago Press.
Stickle, B., and Felson, M. (2020). Crime rates in a pandemic: The largest criminological experiment in history. Am. J. Crim. Just. 45, 525–536. doi: 10.1007/s12103-020-09546-0
U.S. Census Bureau (2021). QuickFacts: Harris County, Texas. Available at: https://www.census.gov/quickfacts/harriscountytexas
Weather Underground (n.d.). Houston, TX weather history: William P. hobby airport station. Available at: https://www.wunderground.com/history/monthly/us/tx/houston/KHOU/date/2021-2
Keywords: COVID-19, school closures, discontinuous growth model, delinquency, SES
Citation: Li N, Quintana D, Shelton M and Grigorenko EL (2023) Impact of the COVID-19 pandemic on youth delinquency: A discontinuous growth analysis. Front. Educ. 8:1007807. doi: 10.3389/feduc.2023.1007807
Edited by:
Brett Miller, National Institutes of Health (NIH), United StatesReviewed by:
Molly McCarthy, Monash University, AustraliaYanhua Xu, Jiangxi Normal University, China
Caitlin Canfield, Grossman School of Medicine, New York University, United States
Elizabeth Connors, Yale University, United States
Paige Greenwood, Columbia University Irving Medical Center, United States
Copyright © 2023 Li, Quintana, Shelton and Grigorenko. This is an open-access article distributed under the terms of the Creative Commons Attribution License (CC BY). The use, distribution or reproduction in other forums is permitted, provided the original author(s) and the copyright owner(s) are credited and that the original publication in this journal is cited, in accordance with accepted academic practice. No use, distribution or reproduction is permitted which does not comply with these terms.
*Correspondence: Elena L. Grigorenko, elena.grigorenko@times.uh.edu