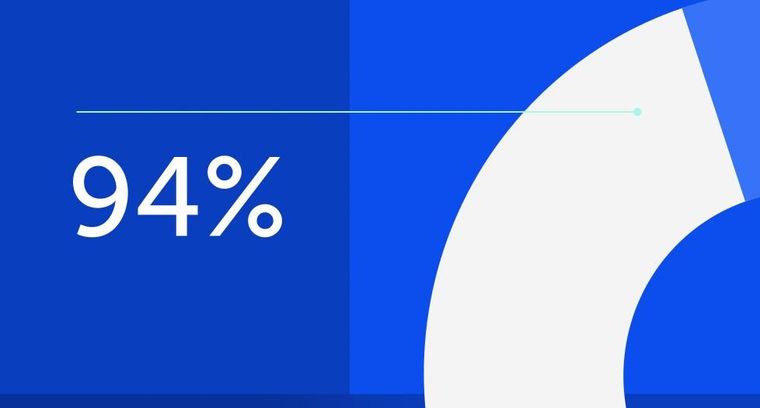
94% of researchers rate our articles as excellent or good
Learn more about the work of our research integrity team to safeguard the quality of each article we publish.
Find out more
ORIGINAL RESEARCH article
Front. Educ., 21 September 2022
Sec. Digital Education
Volume 7 - 2022 | https://doi.org/10.3389/feduc.2022.993265
The objective of the current study is to test the impact of low-tech solutions during COVID-19 school closures on maths, Urdu, and English scores for students in the underdeveloped district of Bahawalnagar, which is situated in the southern part of Punjab in Pakistan. The target population for this study is Grade 8 students attending private schools. Simple random sampling technique is adopted for the selection of participants in the study. We have tested the impact of three interventions, namely: Teaching at the right level (TaRL), Fortnightly assessments (FAS), and Digital teacher training sessions (DTS). Our findings show a significant and positive ‘Intention to Treat’ (ITT) impact on Urdu and English scores of the students in the TaRL treatment group. The students increased their English and Urdu scores by 0.56 SD. However, we found no significant impact of the intervention on maths scores in the TaRL treatment group. Fortnightly assessments and digital teacher training sessions were also found to contribute to higher English scores of the students. However, we found no ITT impact on the maths and Urdu scores for these treatment groups. The Local Average Treatment Effect (LATE) analysis revealed positive and significant improvement in Urdu and English scores of the students in the TaRL treatment group. Key stakeholders whom we interviewed suggested that redesigning the curriculum and incorporating TaRL within this approach could facilitate enhancement in learning outcomes in students in deprived areas. Our findings are important to help inform policymakers on the importance of designing and implementing cost-effective, low-tech solutions to help reduce learning gaps.
Education plays a significant role in the socio-economic development of a country. It builds the capacity of individuals to increase productivity and efficiency, which in turn can put the economy on a path to sustainable development (Ahmad et al., 2014). Within the context of Pakistan, the education system currently experiences two main challenges. The first relates to access, with Pakistan currently hosting the second largest number of out-of-school children. The second relates to poor learning for children even when they are in school (Ahmad et al., 2013).
According to the most recent Annual Status of Education Report (ASER), Pakistan has the second largest number of out-of-school children in the world, with around 22 million learners of school-going age (ages 5–6) out of school. ASER (2019) also illustrates the problem of low learning outcomes, with many students at the primary education level being unable to read a story comprehensively in Urdu (the national language) and Sindhi or Pashto (provincial local languages).
The outbreak of COVID-19 has further aggravated issues relating to access, learning, and gender inequalities. In March 2020, in an attempt to contain the spread of the virus, the Government of Pakistan implemented a nationwide lockdown. This resulted in schools remaining closed from March 2020 to September 2020. Thereafter, intermittent school closures came into effect between November 2020 and August 2021. The lockdown meant that 46 million school-going children in Pakistan were required to stay at home, with learners from impoverished and underdeveloped areas of Pakistan particularly hard-hit by the school closures.
The ‘learning crisis’ in Pakistan calls for an urgent need for the Government of Pakistan to consider how children can learn more effectively. One way to do this is to fundamentally shift how education systems are organized. This would mean organizing children according to their learning levels, rather than age or grade—in other words, teaching at the right level. Using technology to do this, which is the focus of the study, is one approach government officials could consider.
In doing so, governments would need to consider the type of technology that would be appropriate for the context within which this is operationalized in Pakistan. The vastly different economic, social, and cultural barriers that exist when it comes to the use of technology are crucial for success, and these would need to be considered for students, parents, and teachers. Another important implication for policy in terms of organizing education systems in this way is the training that teachers would need in order to execute these changes, as opposed to continuing with a ‘business as usual’ approach. Specifically, given that this incorporates the use of technology by both students and teachers, as proposed by Hennessey et al. (2021) this would mean: using technology to train teachers, including the assessment of their students; and teachers being able to support students in the use of technology for their learning.
There is dearth of literature regarding impact of education technologies in remote areas with a grim socio-economic landscape. During our search for literature on technology and personalized learning in Pakistan, we found limited rigorous evidence. This is supported by Zubairi et al. (2021) who conclude that studies focusing specifically on personalized learning and technology integration in Pakistan are limited, and where they exist they are of poor quality. The study is designed to not only provide rigorous evidence on the use of education technologies in such settings in context of COVID-19 but also provides a real time evidence on the challenges faced at household, school and government level to adopt such measures.
The evidence base on teachers’ use of technology in the classroom in Pakistan is still developing. While a number of studies argue that technology should be provided to students, less attention is paid to how EdTech will be used by instructors. Providing technology to instructors rather than students, on the other hand, was not only more cost-effective but also resulted in better learning results. The outcomes of two RCTs in the Punjab region were compared by Beg et al. (2019) to see how different methods to improving student achievement were. The first method bypassed teachers by giving eLearn tablets to youngsters instead of teachers. In the second, teachers were supported and trained using eLearn classrooms in the second method. The authors concluded that the eLearn tablets reduced student achievement by roughly 0.4 standard deviations, a finding they ascribed to the tablets diverting children’s attention away from more important educational activities. In just 4 months, the eLearn classroom technology increased student achievement by approximately 0.3 standard deviations, or 60%, above the control group. This was partially due to the use of EdTech in conjunction with current pedagogies.
The purpose of the study was to explore what effect a set of EdTech interventions had on improving the learning outcomes of students. It specifically focused on learners living in the economically disadvantaged district of Punjab (in Pakistan). The study was conducted over the period when intermittent school closures occurred due to the COVID-19 pandemic. This study’s core research focus had three stated objectives. These were: (i) Explore the types of technologies being used in the disadvantaged area where this study took place, and understand the constraints faced by the education system in using technology in such settings; (ii) Investigate what role technology-based solutions could have in aiding the objective of ‘personalized learning’ during school closures and (iii) Assess the impact of the selected interventions on learning outcomes for English, maths, and Urdu for learners in Grade 8 of secondary school.
Ed-Tech essentially has three vital areas that need consideration for effective teaching and learning methodologies (Stošić and Stošić, 2015). They are (i) Technology as a tutor (tech-based instruction); (ii) Technology as a teaching tool; and (iii) Technology as a learning tool. The subject study encapsulates all three vital areas and investigates them holistically through the three interventions of Teaching at the Right Level (TaRL), Student motivation, and Teacher effectiveness under RCT.
Our study took place in the District of Bahawalnagar which is located in the southern part of the province of Punjab in Pakistan. Within this district, we selected 12 private schools across five administrative units (known as tehsils) of Bahawalnagar district. From each of the schools we worked in, we selected approximately 20 students to take part in the study. The students who were selected came from economically disadvantaged backgrounds where household incomes were low.
The three interventions implemented and tested were: (i) Computer-assisted teaching at the right level (TaRL) interventions, (ii) Fortnightly assessments (FAS), and (iii) Digital teacher training sessions (DTS).
This research provides insights into what effect technology can have on learning outcomes, especially in context of Pakistan. This study also highlights the particular barriers students from economically disadvantaged backgrounds face and considers these through the lens of gender. The inferences from the study can assist policymakers when it comes to forward-looking policy reforms which look specifically at the role of EdTech within education systems.
Our results show a significant and positive ‘Intention to Treat’ (ITT) impact on the Urdu and English scores of the students who were part of the computer-assisted TaRL treatment group. These students increased their English and Urdu scores by 0.56 SD. However, we found no significant impact on maths scores in the TaRL treatment group. We also found a positive impact of the fortnightly assessment and digital teacher training session interventions on students’ English scores. However, we found no ITT impact on the maths and Urdu scores for these treatment groups. The Local Average Treatment Effects on Treated (LATE) analysis revealed positive and significant improvement in the Urdu and English scores of the students in the TaRL treatment group.
The study found a positive and significant impact of attending online classes due to school closures on students’ maths and English scores. However, we didn’t find any significant impact of online classes on the Urdu scores of students. When differentiating for income, we found that students from relatively higher income brackets scored 0.18 and 0.14 SD points higher in English and maths tests but 0.2 SD lower in Urdu tests. Students from high-income households also scored significantly higher scores in maths and English than students from low-income households.
Key stakeholders whom we interviewed for this study recommended redesigning the curriculum to incorporate approaches such as TaRL to help alleviate the current learning crisis defining the education system in Pakistan. Moreover, parental involvement with students’ education and parental cooperation with teachers and school administrations is also vital.
The rest of the study consists of 4 sections. Section 2 describes the research methodology, including the sampling procedure and sample size, data collection, and analysis. Section 3 presents the main findings. Section 4 discusses the policy implications of this study and outlines a set of recommendations that relate to technology and learning. Section 5 concludes the study.
In this section, we provide a brief review of the literature to gain a better understanding of existing studies on the use of technology in low- and middle-income countries (LMICs) to promote personalized learning for better learning outcomes and cognition. The review is made up of 41 academic articles, together with 13 studies that fell under gray literature or non-academic criteria. This section is divided into two main sub-sections on technology-enhanced personalized learning and teaching at the right level (TaRL).
In a recent review undertaken on technology-supported personalized learning, Major and Francis (2020) found that this can’t only enhance learning outcomes but also support TaRL. The key findings from the review appear to indicate that technology-supported personalized learning:
■ Can lead to a significant improvement in learning outcomes.
■ Is adaptive in nature, allowing students to learn at their own pace.
■ Can potentially help to close educational gaps for lower-attaining students.
■ Would enhance rather than decrease the importance of teachers.
Major and Francis’ (2020) findings are supported by studies undertaken by Koomar and Jull (2020) and Lee et al. (2018).
Technology has revolutionized personalized learning (Andra, 2016). Students’ one-to-one interactions with technologies such as tablets, netbooks, and mobile devices have provided more opportunities for personalized learning both in and outside of the traditional school environment. Students’ interactions with learning platforms can generate data, which may then be used to learn about their knowledge, interests, and preferences. In turn, based on this information, instructional content for students can be tailored to their needs depending on the data generated. Data generated by student use of technology, for example, may be used to compare the learning behaviors they engage in, as well as whether such actions are similar to those of previous successful or failed students (Penuel and Johnson, 2016).
Henrie et al.’s (2015) study of personalized learning in schools, discovered that rather than simply adding devices and software onto existing instructional programs, an integrated and coherent set of technological tools could be used to transform instruction and learning. The schools in their study utilized information management and productivity tools, computer-adaptive curricula and assessments, as well as digital media spaces to aid students in creative work. McHugh et al. (2020) similarly describe the process school leaders developed to produce an idiosyncratic technology ecosystem which is seen as critical to the implementation of personalized learning. Such a system would encompass the use of student-facing digital management systems and computer-adaptive technologies for instruction and assessment. There is a need for a transition from traditional in-class teaching and learning to technology-integrated in-class learning, especially in Pakistan, where resistance to using technology has to be addressed by vigorous research and training.
McCarthy et al. (2020) compared the performance of students in the District of California in the United States who used EdTech with that of a virtual control group. They measured the impact of the EdTech intervention on maths, reading and language. Over the 3-year period that the intervention was administered, the 1,911 students who were in the treatment group consistently outperformed their cohorts. The study also examined the ways personalization of reading tasks influences learning outcomes. It found that learners who received adaptive text selection1 achieved greater gains in their comprehension skills compared to students receiving random text selection, but only if they were less-skilled readers. They found no significant overall differences in performance and no differences in self-reported motivation or engagement. This suggests that there are heterogeneous impacts of the use of technology in education.
Tauson and Stannard (2018) also describe utilizing technology as either an addition to or a replacement for, traditional methods. The results of their thematic analysis, which are organized into four topics, offer further information (ibid. p. 33):
This looks at how technology-assisted personalized learning makes good educational resources more accessible, adjusts to learners’ requirements by teaching at the correct level, extends learning, and potentially eliminates educational disparities for the most disadvantaged.
This investigates the central role of teachers and teacher professional development in enabling technology-supported personalized learning.
This gives an insight into the pedagogical benefits of technology-assisted personalized learning and how it affects student motivation.
This deals with the financial and infrastructure consequences, as well as scalability and sustainability concerns.
Lessons learnt through EdTech are particularly important in the contemporary environment because EdTech has the ability to adjust to learners’ requirements by teaching at the right level. Although there are few examples of research addressing the development of non-cognitive skills, the majority of studies focus on maths and science teaching.
The evidence base on teachers’ use of technology in the classroom in Pakistan is still developing. While a number of studies argue that technology should be provided to students, less attention is paid to how EdTech will be used by teachers. Providing technology to teachers rather than students, on the other hand, is not only more cost-effective but also results in better learning outcomes. The results of two randomized controlled trials (RCTs) in the Punjab region were compared by Beg et al. (2019) to identify the differences between two methods of improving student achievement. The first method bypassed teachers by giving eLearn Tablets2 to youngsters instead of teachers. In the second, teachers were supported and trained using eLearn Classrooms in the second method.
The authors concluded that the e-Learn Tablets reduced student achievement by roughly 0.4 SDs, a finding they attributed to the tablets diverting children’s attention away from more important educational activities. In just 4 months, the eLearn classroom technology increased student achievement by approximately 0.3 SDs or 60% above the control group. This was partially due to the use of EdTech in conjunction with current pedagogies.
Finally, the authors (ibid) highlighted the relative cost-effectiveness and scalability of EdTech interventions at the teacher level, arguing that such programs must function via the government school system, which teaches over 65% of Pakistani students, in order to be effective at scale. Teacher professional development (or training) is at the heart of nearly any pedagogical intervention (Waqar and Bokhari, 2019).
Teaching at the Right Level is an evidence-based educational strategy that assists children at the primary and secondary levels to develop fundamental reading and arithmetic abilities, resulting in improved learning outcomes. Pratham, a renowned Indian organization committed to increasing the quality of education, pioneered this technique in 2007, and it has since been adopted by students all around the world (Lakhsman, 2019).
Teaching at the Right Level was created using a combination of hands-on experience, internal assessments, and research-based RCT evaluations. It has been tested in six randomized studies in India, with positive results (Banerjee et al., 2007, 2010). Since then, this intervention has been employed in countries across the world for students studying between Grades 3 and 10 to improve their reading and numeracy skills. Figure 1 explains the Steps involved in TaRL methodology.
Teaching at the Right Level is an augmented learning method that attempts to improve the quality of education by separating pupils into learning levels rather than grades and ages. It was created by the Pratham Education Foundation (Jagannathan, 2001) and encompasses the following key components for its teaching practice:
There are two different models used in TaRL now, following decades of assessments and refinement:
1. The Directly Implemented Learning Camp Model. In this model, children are grouped according to their learning levels after pre-assessments; they study for 2–3 h after school, for 1–2 months.
2. The Government Partnership Model, which engages teachers after training.
Teaching at the Right Level can be implemented in any of three ways (see Figure 2).
Thus, personalized learning technology-based TaRL is an approach that uses technology to facilitate the TaRL pedagogical approach. An example of where such an approach has been applied comes from India. A study undertaken by Muralidharan et al. (2017) is one example of this approach. Here, the intervention in question focused on 215 students in Marathi-medium schools in India. The study found that technology-assisted TaRL is effective for learning and teaching. Students scored 100% in one of the eight phonic skills and learning abilities increased approximately tenfold.
Perry and Steck (2015) tested the impact of an online TaRL intervention on student learning outcomes in the United States. Students underwent a pre-assessment and were segregated into groups. The treatment group was then given access to iPads, while the control group were administered traditional in-classroom teaching pedagogical approaches, which involved direct instruction, pen-and-paper and drill and practice methods of learning. The content used to teach both groups was the same. Post-intervention, the treatment group demonstrated greater student engagement, improved test scores, and increased self-efficacy compared to the control group. However, a number of studies conclude that merely integrating technology into teaching and learning is not enough to achieve desired outcomes. Training teachers in the use of technology is equally important to motivate teachers (Rosas and Campbell, 2010; Parkay et al., 2010).
The literature reviewed above recommends a shift toward personalized learning. During our search for literature on technology and personalized learning in Pakistan, we found limited rigorous evidence. This is supported by Zubairi et al. (2021) who conclude that studies focusing specifically on personalized learning and technology integration in Pakistan are limited, and where they exist they are of poor quality. Furthermore, the findings from the studies that do exist must be treated with caution given their unreliable research designs (Rodriguez-Segura, 2021). This dearth in research is part of the motivation for our study, the detail of which is presented in the following section.
This section details the methodological approach used in this study, which primarily focuses on an RCT approach that measures the effect of technological interventions on students learning outcomes.
We administered the interventions to 12 randomly selected private sector schools3 in the Bahawalnagar district which is one of the districts situated in the Pakistani province of Punjab. Random sampling technique is adopted due to the reason that (i) there were only few schools in the district that offered online education during school closure and (ii) those schools were not uniformly scattered in the district. Therefore, a random sample was chosen from the set of available schools offering online education.
Within the district of Bahawalnagar, there are 118 union councils within which there are 390 private schools. Since Bahawalnagar is a remote and underdeveloped district, the majority of the private schools were unable to deliver online education due to the unavailability of technological devices such as smartphones, tablets, and laptops, etc. This was further compounded by internet costs being prohibitive for the majority of the schools in this district. Hence, only 25% of the private schools in the district delivered online instruction during school closures.
The 12 schools selected for this study were selected on the basis of:
1. Their ability to deliver online education.
2. The schools offering education to Grade 8 students.
3. Both boys and girls attending the schools as this study was interested in measuring the intervention’s differential effect by gender.
All available students from Grade 8 were selected and, as far as possible, an equal number of boys and girls were selected from each school.
The 12 schools were selected from 12 different union councils to avoid spillover effects. Eight of these schools were used as treatment schools, while four schools were randomly selected to act as a control group. Table 1, below, shows the distribution of samples into different treatment groups. Parents of the 258 students who were chosen to be part of the treatment and control groups were then selected to collect information intended to capture socio-economic dynamics and the possible impact of COVID-19 on the students’ learning outcomes.
We collected quantitative data on the relevant socio-economic characteristics by conducting structured surveys with 246 parents (father/mother), 258 students, 36 teachers (class teachers and subject teachers), and 12 school principals. The aim was to collect the data required for the baseline. The dimensions covered in the surveys were as follows:
1. Socio-economic profiles of households, including household composition, monthly income, information on other assets, employment status, type of house, monthly internet expenses, number and type of available devices to access online education.
2. Access and barriers to EdTech and its use.
3. Perception of respondents regarding the effectiveness of educational technologies and online delivery of instruction.
The study employed two types of tests to assess student learning. The first were standard tools that have been developed for the ASER4 Survey in Pakistan. Language and maths tests administered for the ASER Survey are the primary performance measures ASER uses to test children’s performance. Since ASER tests measure basic numeracy and language skills to Grade 3 level, we observed a ceiling effect in the results of the majority of the Grade 8 students. Grade-appropriate tests were designed to match the grade-level competency of the students to avoid such ceiling effects. The tests were designed from the Grade 8 Punjab textbook board syllabus for maths, English, and Urdu. The Urdu and English tests covered vocabulary, grammar, and reading comprehension and maths tests measured grade-level skills such as number system, sets, LCM, HCF, ratios, etc. Tests were administered twice, once in baseline and then in endline surveys. These tests, which took between 30 and 35 min to administer, tested the grade-appropriate competencies of the students.
The preliminary observation from our baseline survey of 12 schools located in Bahawalnagar district illustrated that the majority of the sampled students would only benefit from online education through the use of smartphones (WhatsApp messages) or basic phones (messages). This observation was based on the very limited ownership by households in our sample of either a laptop/PC or tablet.
The majority (approximately 90%) of the sample population used mobile data to access the internet. The speed and bandwidth of the mobile data were very low in the majority of the locations that we were conducting this study, thereby making the use of Zoom to conduct online teaching a fundamental challenge. Of the sample of schools that were worked with, only two schools—situated in relatively more developed regions of Bahawalnagar district—used Zoom to conduct online teaching. Seven out of our sample 12 schools continued online education through the use of WhatsApp. With these factors in mind, we used WhatsApp5 to deliver online instruction of TaRL.
After selecting the four schools where the TaRL intervention would be implemented, we identified weak students who scored less than 50% in the grade-appropriate test that they took for the baseline survey. The students from all four schools were then grouped into English, maths, and Urdu groups. Hence, we formed three separate WhatsApp groups (one for each subject) consisting of 30 to 35 students in each group. The grouping was done separately for each subject, and a separate instructor/volunteer (with specialization and relevant experience in that subject) was assigned to each subject group.
The instructors sent personalized learning material to each group that matched the learning level of that specific group every week. The learning material was related to the grade-appropriate skills tested at baseline. Students received a learning problem, a personalized recorded video for solving that problem, and a short quiz through WhatsApp. The students had to solve the problem during the week and send a picture of the solved problem to the instructor via WhatsApp. Online material on IXL6 and Khan Academy for maths and English were also utilized and shared with the students where feasible. However, the instructor for Urdu language used personalized videos7 for instruction, practice material, and quizzes due to the unavailability of online material on IXL and Khan Academy. After 3 months of instruction, the students were re-assessed, and an endline survey was administered to evaluate the impact of the TaRL intervention on their learning levels.
The digital training session intervention was designed to provide teacher training in four schools on using EdTech for instruction in and out of school. The majority of the teachers in the selected schools had not received any training to deliver technology-assisted instruction before our proposed intervention. The training was intended to help teachers to use technology more competently to better understand whether this could positively affect student learning outcomes. Three teachers per school (teaching maths, Urdu, and English, respectively, in Grade 8) were selected from the four schools where the digital training session treatment was administered. In one school, where there were two Grade 8 classes, 6 teachers were trained in the use of technology. In total, 15 teachers from the 4 schools received training from an expert trainer. These sessions took place over Zoom on a fortnightly basis, with a total of 6 sessions delivered (see Table 2).
Student engagement is a concept that is approximately three-quarters of a century old and refers to how engrossed or attentive students seem to be in their learning (Axelson and Flick, 2011).
Formative assessment is a tool to enhance student motivation and engagement in the learning process. Previous research shows that formative assessment conducted by teachers in the classroom has a positive impact on students’ learning and motivation and may increase students’ achievements, their understanding of how to learn, and control over their learning (Black and Wiliam, 1998; Brookhart, 2009).
The intervention was designed to affect learning from the demand side of education by enhancing student involvement in the learning process. For this intervention, teachers of maths, Urdu, and English from selected schools were given two training sessions before the intervention. The sessions imparted skills to make and assign online assessments to students using the CK12 app and WhatsApp. These instructors assigned six online assessments/quizzes to their class on a fortnightly basis using CK-12 and WhatsApp as per the availability of devices such as smartphones, tablets, etc., and internet facilities for their students. Each quiz was created using the content taught in the 2 weeks prior to the delivery of the assessment in respective schools. Reassessment of students at the end of the intervention measured the impact of this intervention on student learning levels.
To help answer the third and final research question, key informant interviews and focus group discussions were held.
The key informant interviews were held with institutions/individuals with deep insight into the existing landscape of education during the COVID-19 pandemic and the spectrum of education technology in use, especially in impoverished and deprived areas of Pakistan.
Our sampling approach for stakeholder selection was based on identifying respondents from different domains.
■ A stakeholder familiar with the local landscape of secondary education where the study was being conducted, i.e., Bahawalnagar district.
■ A stakeholder from the research and policy domain with professional expertise in education and EdTech for policy insights.
■ Stakeholders from the international development network, operating in Pakistan and facilitating the role of EdTech in education.
In this context, stakeholders from these areas were identified and semi-structured interviews of three key stakeholders were carried out. Given the sort of information we were interested in collecting, our key informant interviews were with stakeholders from:
1. Foreign, Commonwealth and Development Office (FCDO).
2. Institute of Development and Economic Alternatives (IDEAS), Pakistan.
3. A member of the Private School Association/Private school owner in the Bahawalnagar district.
Apart from the face-to-face interview, which we held with the member of the Private School Association, the remaining interviews were conducted online. The interview schedule was divided into the following areas.
1. The landscape of education in Pakistan especially during the COVID-19 pandemic.
2. The adaptability of EdTech by the target group (students, teachers, and parents).
3. Barriers in the use of EdTech, especially in deprived areas.
4. The impact of COVID on learning levels.
5. The role and response of the government and key players in relation to EdTech.
6. Future priorities for EdTech.
Focus group discussions were held with the teachers of the selected schools in the sample. These were held in person, and each focus group included 6–8 teachers teaching in Grades 7 and 8.
Exploratory data analysis (EDA) attempts to examine and display observed data in a relatively straightforward way. As a technique, EDA was considered an appropriate technique for obtaining thorough information on student motivation and teachers’ skills.
This study suffers from non-compliance in two of its interventions. The students included in TaRL were added to WhatsApp groups. However, around 15% of the original 78 students selected for the study left the groups and did not enroll and sign the consent form to participate in the study again. A further 20% of students left the groups in the middle of the intervention. This meant that only 65% (or 51 out of the 78 original students selected for the study) remained in the group till the end of the intervention. Similarly, for fortnightly assessments, a total of six tests were administered on a fortnightly basis during the 3 months of the intervention to 83 students. However, 37% of students (or 31 students) did not attempt any test in this intervention.
Hence, the estimated impact in the absence of full compliance in the treatment group is called ITT. Here, we compare the groups that were randomly assigned to the treatment with the comparison group regardless of the lack of full compliance of the treatment group. The ITT is a weighted average of the outcomes of participants and non-participants in the treatment group compared with the average outcome of the comparison group (Khandker et al., 2010).
The following specification was used for estimating the impact of interventions on test scores (ITT) in the first step:
Here, Yist1 is the normalized test score (normalized at μ = 0 and SD = 1) of the student i in school s at time t1 (endline). At the same time, yist0 is the student’s test score in school s at time t0 (baseline). xis is a vector of student- and household-level factors, including gender, online class status, and wealth index. ist is the error term. Treatment is the variable of interest in this equation. Treatment is a categorical variable showing random assignment of sample units into different treatment groups or the control group. Treatment is assigned the value “1” if the student is in the ‘combined treatment’ group, “2” if the student is in a ‘TaRL treatment’ group, “3” for the ‘fortnightly assessment’ group, “4” for the ‘digital training session’ group, and “0” for the control group.
We ran two specifications, one without clustering and one with clustering standard errors at the level of schools. Further, all the specifications were controlled for baseline test scores. In addition, we also controlled for gender, online class status (a dummy assigned the value “1” if the school continued online education during school closure and “0” otherwise), and for the wealth index of the household. The wealth index is calculated by using the first factors from the polychoric principal component analysis. Household monthly income and different household assets are used for the construction of the wealth index.8
Next, we also needed to measure the impact of our program on the group of individuals who were offered the program and who participated. This estimated impact is called the ‘Treatment on the Treated’ (Khandker et al., 2010). In case of incomplete compliance, we needed to estimate the Local Average Treatment Effect (LATE). To measure LATE, i.e., the impact of treatment on those who attended the online instruction (TaRL) or assessments (FAS), we specify the instrumental variable (IV) equation as follows:
Here Yist1, Yist0, Xisθ, and εist are defined as earlier. ‘TaRL attendance days’ are the number of days a student remained in the WhatsApp group formed for TaRL instruction. Fortnightly assessments (FAS Tests) are the number of tests a student attempted out of six tests administered during the 3-month intervention period. The Local Average Treatment Effect estimated the dose-response relationship between attendance days, tests attempted, and value added.
Since the participation of the students in TaRL groups and FAS tests may be endogenous to the expected performance on test scores, we instrumented attendance days and FAS tests with the random allocation of a student to particular treatment groups following Muralidharan et al. (2017).
To test the endogeneity of the instrumental variable, we applied the Durbin and Wu–Hausman tests. The null hypothesis of both the tests is that the variable under consideration can be treated as exogenous.
Next, we applied Sargan and Basmann tests of overidentifying restrictions. The null hypothesis of these tests is that one or more instruments are invalid, or that the structural model is incorrectly specified.
We ran two specifications, one without clustering and one with clustering standard errors at the level of schools. Further, all the specifications were controlled for the baseline test scores. In addition, we also controlled for gender, online class status (a dummy assigned the value “1” if the school continued online education during school closures and “0” otherwise), and the wealth index of the household. The wealth index is calculated by using the first factors from the polychoric principal component analysis (PCA) (see Table 1). Household monthly income and different household assets are used for the construction of the wealth index.
Since this research focused on students who were under the age of 18, we took a number of measures to preclude any unintentional adverse effects on the participants and to ensure their participation was truly voluntary. We obtained permission from a parent or legal guardian in the household to acquire permission for the student to take part in the study.
The participants were also assured that no identifiable personal data would be shared with any other party and that their responses would not be used for any purpose other than the aims and objectives of this research. This guarantee of anonymity and the confidentiality of the respondents’ data was provided and respected.
To ensure the cultural appropriateness of the survey, female enumerators surveyed females/girls and male enumerators surveyed males/boys. Surveys were then translated into Urdu and local enumerators were assigned to conduct interviews in Urdu.
The primary challenge for this study related to the uncertainty caused by COVID-19, with the frequent school openings and closures leading to coordination challenges. As soon as our intervention started, a nationwide lockdown due to the third wave of COVID-19 sweeping the country meant that schools were closed. This negatively affected our interaction with students and teachers and coordination with them became more challenging.
A further challenge negatively affecting the study was the unwillingness of teachers and school administrations to take part in the study unless financial incentives were forthcoming. Moreover, school administrators were reluctant to run the interventions introduced for this study in their schools, arguing that this would be an extra burden both for teachers and students.
Another challenge we faced was that a number of the teachers and students who were part of the study frequently left the WhatsApp groups which had been specifically created to administer the interventions. This links to a major limitation of this study—its small sample size. Budget and time constraints meant that we were only able to focus this intervention on 12 schools and 258 students. The attrition of students leaving the intervention or else changing schools meant the original number of students who were part of the intervention fell further to 208 when we administered the endline survey.
Lastly, the study design was constrained in the sort of technological solutions we could employ, due to the lack of appropriate infrastructure to support these in the remote area of Pakistan where we were working. The digital training session intervention, for example, was constrained due to the lack of computer laboratories in schools sampled in this study, and teachers’ unreadiness to use this technology. In addition, some teachers did not even own smartphones. This limited our capacity to go for high-tech instruction methods, which we believe could have contributed to more effective and interactive delivery of instruction.
Our intervention targeted 258 students who took the baseline tests pre-intervention. Of these, 139 (54%) were female and 119 (46%) were male. The control group included 43 males and 44 females, while the treatment group included a total of 96 females and 75 males. Ultimately, 81% (or 208) of the original sample took the endline test (see Table 3).
While there was 100% compliance for the digital training session intervention, the issue of non-compliance arose when it came to the TaRL and fortnightly assessment interventions. For the TaRL intervention, for instance, the mean attendance days in WhatsApp groups was 25 days. The ‘complier’s’9 mean attendance rate was 28% (25 days out of 90 maximum possible days) in TaRL groups. For the fortnightly assessments, a mean of 4.9 Urdu tests out of the maximum of 6 tests was attempted by complying students. The equivalents for English and maths were 5.2 and 4.9, respectively.
We found no significant difference between treatment and control groups in mean student characteristics (age, gender, wealth index, or baseline test scores) of those who attended both baseline and endline tests and who comprised our main study sample (Table 4).
The exploratory data analysis (EDA) was intended to capture issues relating to the access, use, and barriers students faced in using EdTech during school closures.
The main mechanisms through which students accessed learning content during school closures were WhatsApp, SMS, and textbooks. Nearly 93% of students used WhatsApp and SMS as a mechanism with which to continue their learning during the COVID-19 pandemic, while 88% used textbooks to do the same. Comparatively fewer students accessed learning through smartphone applications and web platforms (37%), and recorded videos (35%). When it came to online classes, less than 15% of students reported learning through this mechanism during school closures. On the other hand, 50% of students resorted to in-person tuition, which became a dominant source of learning.
In terms of access and type of technology, the majority (98%) had access to some form of technology in their homes. Mobile phones turned out to be the most prevalent type of technology (96%) in households, followed by TV (72%). By contrast, laptop ownership was comparatively lower (31%). Most mobile phones owned by households were smartphones (74%), while 19% owned basic phones. The high level of smartphone ownership means there is potential to explore the access of educational apps by students through the use of smartphones. Our survey found that nearly 56% of students had access to mobile phones during school closures, while nearly half had limited access or no access at all (Figure 3, below).
The majority of the students (71%) confirmed that online lessons took place during school closures (Figure 4). The main mechanisms for the delivery of these online lessons were WhatsApp or SMS messages. Online lessons using Zoom, Teams, and Google Classroom, on the other hand, were reported to be less well-utilized with less than one-eighth of the sample indicating these mechanisms were used.
When it came to online assessments, close to 62% of students surveyed reported taking these during the period of school closures. The type of online assessments varied depending on the school. Multiple-choice questions (32%) and short-answer questions (35%) were the main formats of online assessments. A substantial percentage of students (42%) used WhatsApp for taking online assessments during school closures.
Students who faced barriers in accessing online education during school closures reported the unavailability of devices (laptops/tablets/smartphones) as the main reason for this (47%), while 19% reported the unavailability of the internet as a barrier to accessing online education. A total of 34% of students could not access online education because their school either did not offer any online options or access to devices or the internet (Figure 5).
Students had mixed views about the effectiveness of EdTech in terms of learning. While the majority of students (48%) indicated that EdTech proved to be effective and that they had learned new concepts through EdTech, a significant number of students disagreed (30%), while 21% remained neutral. The consensus among students was that nothing could replace the in-class learning experience.
When questioned about monthly internet costs over the period schools were closed, 37% of the parents surveyed reported that they spent less than Rs 1,000 per month (this is equivalent to USD 5.8). For 35% of parents surveyed, the cost was the equivalent of between Rs. 1,000–2,000 (between USD 5.8 and USD 11.7). For 6.5% of parents surveyed, the cost exceeded Rs 2,000 (USD 11.7).
When asked about the time parents spent supporting their children with online education, the majority of the parents (87%) reported allocating 1–2 h per day to facilitate their children’s access to online education. Nine per cent spent 2–3 h per day helping their children with online learning during school closures.
Of the 246 parents asked whether preferential access was granted to children based on their gender, 130 parents (or 53% of the total) indicated there was no gender bias. In contrast, 54 parents (or 22% of the total) indicated that they gave boys preferential treatment over girls when it came to accessing devices needed for education, while 29 (or 12% of the total) parents gave preference to girls over boys (Figure 6). Similarly, while most parents (67% of the total surveyed) permitted their daughters to access and use various devices to attain online education, 72 parents (29%) did not permit girls to access devices needed to access education during the school closures (Figure 7). Among households where girls were prohibited from accessing devices, the reasons ranged from cultural or religious to financial ones. Lower motivation to learn and decrease in literacy were reported to be the major outcomes of COVID-19 (Figure 8).
Figure 6. Were either boys or girls given a preference in accessing technology during school closures?
The grade-appropriate tests administered at baseline and endline provide us data for the assessment of grade-level competence of the students and improvement in their scores as a result of interventions administered in our sample. Before discussing the regression estimates, a simple visualization of the baseline and endline scores through box plots (Figures 9–11) depicts an improvement in the student’s endline scores in the TaRL and combined treatment groups for all three subjects in contrast to the control group. However, we observed no positive change in students’ endline scores in the fortnightly assessment and digital training session treatment groups at this stage. Section 4.3.1 and 4.3.2 below present regression results to provide greater detail on the exact impact of the interventions on students’ performance.
Table 5, below, shows the impact of our different interventions on the standardized scores achieved by the students. We found that the students randomly assigned to the TaRL group increased their English and Urdu scores by 0.56 SD compared to the control group following the 3 months of the intervention (see columns 1, 2, 4, and 5, row 2). However, our results found no significant impact of TaRL on maths scores. This is in contrast to what other studies evaluating the impact of computer-assisted learning in under-resourced contexts have found where observable significant and positive effect sizes on student academic achievement of between 0.10 and 0.35 SD have been noted (Banerjee et al., 2007; Mo et al., 2015). However, our data on Urdu and English scores are consistent with these studies. Our findings show that the combined treatment effect led to a 0.32 SD higher score in English compared to the control group (column 2 and 5, row 1).
The interventions relating to fortnightly assessments and the digital training sessions also demonstrate an improvement in the English scores of the groups receiving this. Fortnightly assessments resulted in a 0.33 SD higher score while digital training sessions increased the English score of the treatment group by 0.58 SD. These interventions, however, had no impact on Urdu and maths scores (Columns 2 and 5, Row 3 and 4).
The first covariate is the wealth index of the households constructed by using the first factors of polychoric principal component analysis (see Table 1). The second covariate is a dummy for gender, and the third is the dummy for showing if the school continued online education during school closures due to COVID-19. These additional covariates did not change our previous findings, with the TaRL intervention contributing to a positive and significant improvement on the Urdu and English scores of students.
Table 1 presents the impact of the wealth index on students’ standardized endline scores. We found that students with a higher score on the wealth index scored 0.18 and 0.14 SD points higher in English and maths tests while 0.2 SD lower in the Urdu tests after 3 months.
These findings may be supported by the fact that the students from well-off families usually go to English-medium schools where greater emphasis is given to English and maths subjects. The students from higher-income brackets usually have good English language skills compared to Urdu. We can confirm it from the fact that the wealth index and baseline scores of students were positively associated with maths (Correlation coefficient = 0.16) and English scores (Correlation coefficient = 0.27) while negatively correlated with the Urdu scores (Correlation coefficient = −0.05).
Gender did not significantly affect student scores (Table 2). However, for students who continued to receive online education over the course of the school closures, standardized maths and English scores improved at the endline (Table 3). At the same time, however, we found no impact of online classes on the Urdu scores of the students.
Again, in all specifications, TaRL significantly improved Urdu and English scores with no impact on maths scores. Recent research on the effectiveness of computer-assisted learning has shown that insignificant or small impacts may be linked to a lack of compliance with the intervention (Tang et al., 2018; Mo et al., 2020). The next section takes non-compliance into account and estimates the local average treatment effect on the treated.
Using the 2SLS technique, IV estimates appear to indicate that an extra day of attendance within the WhatsApp groups formed for TaRL instruction increased Urdu scores by 0.03 SD and English scores by 0.02 SD. See columns 1, 2, 4, and 5 in Table 6, below.
However, the number of tests attempted under fortnightly assessments did not increase student scores significantly in any of the subjects. Since there was 100% compliance for the digital training sessions treatment, we only tested the dose-response value-added impact for TaRL and fortnightly assessment interventions.
Rows 8, 9, 10, and 11 in Table 6 present the test statistics for overidentifying restrictions and endogeneity. The p-value of both Sargan and Basmann test statistics is greater than 0.1; hence we reject the null hypothesis of overidentification and misspecification of our structural model. Durbin and Wu-Hausman test statistics are highly significant, so we reject the null of exogeneity; we must continue to treat endline test scores as endogenous.
Again a day’s increase in attendance in the WhatsApp TaRL group led to a 0.04 SD increase in Urdu and a 0.03 SD increase in English scores. Hence, attendance in TaRL groups turned out to be consistent and significant in this specification as well. Online classes increased students’ scores by 0.49 SD in English and 0.51 SD in maths. We found a significant improvement in the English and Urdu standardized scores in the TaRL treatment group when using the ITT and LATE approaches. However, the fortnightly assessment intervention did not show any improvement in test scores at the endline. However, we found no significant impact of TaRL on maths scores.
An important aspect of computer-assisted learning at the right level is the differential role of teachers in school- or home-based learning. Straub (2009) suggested that adoption and compliance of computer-assisted learning interventions are significantly dependent on the teacher, even if the end-user is the student. Since greater teacher–student interaction is required for effective maths understanding, our intervention of TaRL, where video lectures were shared through WhatsApp messages when the schools were closed, could not have a significant impact.
One piece of evidence done in the less-resourced regions consistent with our finding is from De Witte et al. (2015). They found significant improvement in standardized math scores of the 9898 secondary school students in the Netherlands’ low-performing schools after the use of CAL software. In another evidence is from United States African American Children. Access to and use of computers and software had a positive impact on the academic achievement of the students (Judge, 2005).
Research conducted by Leu et al. (2011) stated that students living in the deprived areas rarely use the internet for learning purposes. Thus, it is important to introduce contemporary technical equipment as a learning tool from an early age (Gutnick et al., 2011; Rideout, 2014).
Ma et al. (2020) conducted an RCT for measuring the impact of CAL intervention on Math performance and academic attitudes of rural students in Taiwan. They found no significant ITT impact on math performance but LATE showed significant improvement in the performance of the 30 most active students in the treatment group.
An assessment from 215 students from 20 sessions in Marathi schools in India suggested that computer-assisted TaRL is effective for learning and teaching. Results from the study revealed that students scored 100% in one of the eight phonic skills and learning abilities increased approximately ten times. Research evidence from another experimental study revealed positive impacts of using technology within the education system. Using technology in the TaRL approach enhances productivity in the delivery of education (Muralidharan et al., 2017).
Muralidharan and Sheth (2016) have conducted a study based on the Mindspark program. In their study, they have offered 45 min of CAL software access to students and 45 min for instructors. Students were able to open this program, 6 days a week. Study results were analyzed in two forms; independently collected test scores and school tested scores. Results from both scores showed a significant positive impact of the intervention on students.
A randomized control trial (RCT) conducted on computer-assisted learning by the Gujrat government in India suggested that CAL significantly improved the math scores of students (Banerjee et al., 2007). A peer-reviewed study suggests that computer-assisted instructions improve reading comprehension levels for students with learning disabilities (Kim et al., 2017). Researchers have also reported that this program is cost-effective and can be implemented in school classrooms, in after-school programs, or through self-guided study.
Online education requires the cooperation of parents but, as the feedback from the focus groups and key informant interviews illustrated, this was not forthcoming. A number of reasons for the lack of parental cooperation were identified.
The low literacy and educational attainment levels of the parents of the student population we were working with meant that few parents were technologically literate. This translated into two specific barriers. First, parents’ technological illiteracy prevented them from supporting their children when accessing online education. Second, it led to parents’ reluctance in allowing their children access to technological devices. Rather than seeing technology as a medium to access educational content, technology was instead perceived by parents to be a mechanism through which children were idly spending their time surfing the internet.
In terms of accessing education online, gender dimensions also reflected some of the restrictions imposed in households, specifically when it came to girls’ use of technology. In the EDA analysis, 80% of respondents indicated that they gave preference to boys over girls for using mobile phones. The focus group discussions with teachers indicated that often girls were not allowed to use mobile phones, or else indicated that the uneven distribution of household chores contributed to girls’ lack of participation in online classes.
Aside from barriers relating to households, the lack of teacher training in how to use technology to conduct online classes was identified as a barrier. In the context of the study, teachers were ill-prepared to teach remotely using technology and were not given the tools needed to do so. While teachers required time to acclimatize to this new normal, the reality was that several stakeholders identified that teachers were not given the support they needed to switch from face-to-face to online teaching.
This study has revealed a number of important findings. Some of these relate specifically to the use of technology-assisted TaRL, while other insights are more generally related to the use of technology to support education. Specifically, TaRL is a promising approach to teaching, which has demonstrable positive effects on learning outcomes. However, there are general challenges relating to technology adoption that must be overcome before technology-enabled TaRL can be incorporated more widely. This section presents some of the main issues that policymakers need to take into account when designing a technology-assisted TaRL program in Pakistan. 1. Actively involving school actors in the design of a technology-assisted TaRL intervention is important for the long-term buy-in of teachers and students. One of the challenges that this study faced was the resistance of school actors in implementing this intervention. By extension, this led to challenges of non-compliance among students selected to take part in the program. Where school teachers were supportive of our intervention, students were more proactive in the WhatsApp groups that were being administered by us. The opposite was also true with a lack of teacher support resulting in less student compliance. Part of the resistance stemmed from school officials believing the intervention created more work for them and the students. Therefore, any TaRL intervention should be integrated into existing processes. It must also avoid overburdening both students and teachers in order to get adequate buy-in. 2. Understanding the complexities involved when it comes to implementing technology-assisted TaRL interventions and how this can impact their level of success is key. For the purposes of this study, the design was heavily reliant on hiring specialist volunteers to administrate the WhatsApp groups through which interventions were administered. Similarly, due to the school closures, the intervention was largely implemented out of school and at home. Lastly, the interventions were made in addition to the instructional time being offered by schools. Each of these factors affected student and teacher compliance. For example, interventions were administered at the household level, despite our interviews appearing to conclude that the households in the context we were working in appeared to harbor largely negative attitudes toward technology. Policymakers would therefore need to Investigating the Impact on Learning Outcomes of EdTech in Punjab, Pakistan 45 EdTech Hub consider the best mix of approaches when designing a technology-assisted TaRL intervention. While the interventions that we administered for this study did not provide support to any of the households, given the importance of such support we would also recommend that any program carefully look at what support households could be given to facilitate students’ learning. 3. Considering factors relating to access and use of devices beyond device ownership alone is essential. The design of our study was to a large extent influenced by the types of devices households in the poor rural contexts we were working in had access to, namely, mobile phones. We found, however, that even where household ownership of mobile phones was high, internet costs associated with accessing WhatsApp—the main medium through which our intervention was rolled out—was a big challenge in terms of engaging students within our treatment groups. This supports Muralidharan et al. (2017), who found that any technology-aided instruction involving even a small cost limits the ability of low-income students to benefit from the program. In such contexts, policymakers must consider costs relating to hardware, alongside costs that end-users may face. Policymakers may wish to consider whether and how some of these costs can be subsidized. 4. Community perceptions of technology must be factored into the planning of any technology-supported education programs. Lack of implementation fidelity was a significant challenge in delivering this research. There were significant levels of non-compliance among participants. Our research identified that this non-compliance largely stemmed from limited pre-existing exposure to technology among the participants’ families and teachers. Where technology use was more common it was generally used for entertainment purposes, rather than education. These kinds of cultural norms and reluctance to use technology to support learning must be addressed before EdTech can support learning among the most marginalized.
The objective of this study was to test the impact of a technology-assisted TaRL intervention on student learning outcomes during school closures in the low-income district of Bahawalnagar in Pakistan. This was undertaken using low-tech solutions. Our findings showed a significant and positive impact on the Urdu and English scores of students who were part of the TaRL treatment group. However, no significant impact of the TaRL intervention was found for maths scores. The digital training session and fortnightly assessment treatment groups were found to have positive and significant effects only on English scores. The impact of the intervention was found to be linked with both the fidelity of user uptake, as well as cultural norms surrounding the use of technology among the beneficiary communities. Interestingly, the study found that gender did not seem to be a determining factor in the learning outcomes generated. However, it was observed that students from higher-income households generally had higher levels of engagement with the intervention, and therefore, income was linked to better learning outcomes of students.
This study concluded with a set of key policy suggestions based on the findings emerging from the study. This includes policy suggestions relevant to the deployment of technology-enabled TaRL solutions, as well as the deployment of technology-facilitated learning interventions more generally. These recommendations include ensuring that school actors (i.e., teachers and students) are involved in the design of technology-assisted TaRL solutions to foster buy-in, applying due consideration to how the implementation modalities may influence the uptake of the TaRL tools, expanding access considerations well beyond device ownership and considering cultural attitudes to technology during program design. Considering these important areas during the design and implementation of both technology-enabled TaRL programs and EdTech interventions more broadly, are likely to significantly improve the success of future similar initiatives.
Identifying the most effective modes of delivery for the program at larger scale is an important area for future research (Muralidharan et al., 2017). Further our findings on Digital training sessions should not be deemed as there is no impact of teacher training on student performance. Since the schools remained closed during most of the intervention period and some of the schools were not even delivering online instruction, the intervention showed no significant improvement in test scores of students in Urdu and Math subjects. Similarly, no significant LATE for FAS may also be seen from the same lens.
The datasets presented in this article are not readily available because the funder edtechhub.org have a data secrecy policy. The data may be made available by making an appropriate request to edtechhub.org.
Ethical review and approval was not required for the study on human participants in accordance with the local legislation and institutional requirements. Written informed consent to participate in this study was provided by the participants’ legal guardian/next of kin.
FA contributed to write-up, survey design, survey conduct, stakeholder interviews, and FGD. RN contributed to intervention and study design, intervention conduct, quantitative analysis, and write-up. MA contributed to coordination for survey and intervention conduct, supervision of interventions, and write-up. All authors contributed to the article and approved the submitted version.
This study was funded by the edtechhub.org and they have a data secrecy policy. Research reported in this publication was supported by Ed-Tech Hub.org that receives funding from United Kingdom Aid and World Bank, licensed under https://creativecommons.org/licenses/by/4.0/.
The authors declare that the research was conducted in the absence of any commercial or financial relationships that could be construed as a potential conflict of interest.
All claims expressed in this article are solely those of the authors and do not necessarily represent those of their affiliated organizations, or those of the publisher, the editors and the reviewers. Any product that may be evaluated in this article, or claim that may be made by its manufacturer, is not guaranteed or endorsed by the publisher.
Ahmad, I., Rauf, M., and Rashid, A. (2013). Analysis of the problems of primary education system in Pakistan: Critical review of literature. Acad. Res. Int. 4, 324–331.
Ahmad, I., Ur Rehman, K., Ali, A., Khan, I., and Khan, F. A. (2014). Critical analysis of the problems of education in Pakistan: Possible solutions. Int. J. Eval. Res. Educ. 3, 79–84. doi: 10.11591/ijere.v3i2.1805
Andra, N. (2016). The role of technology in addressing personalized learning. MA thesis. Austin, TX: The University of Texas.
ASER (2019). Annual status of education report. ASER. Available online at: http://aserpakistan.org/document/aser/2019/reports/national/ASER_National_2019.pdf (accessed March 4, 2021).
Banerjee, A. V., Banerji, R., Duflo, E., Glennerster, R., and Khemani, S. (2010). Pitfalls of participatory programs: Evidence from a randomized evaluation in education in India. Am. Econ. J. 2, 1–30. doi: 10.1257/pol.2.1.1
Banerjee, A. V., Cole, S., Duflo, E., and Linden, L. (2007). Remedying education: Evidence from two randomized experiments in India. J. Econ. Econ. Educ. Res. 122, 1235–1264. doi: 10.1162/qjec.122.3.1235
Beg, S. A., Lucas, A. M., Halim, W., and Saif, U. (2019). Engaging teachers with technology increased achievement, bypassing teachers did not. (No. 25704; NBER Working Papers). Cambridge, MA: National Bureau of Economic Research, Inc.
Black, P., and Wiliam, D. (1998). Assessment and classroom learning. Assess. Educ. 5, 7–74. doi: 10.1080/0969595980050102
De Witte, K., Haelermans, C., and Rogge, N. (2015). The effectiveness of a computer-assisted math learning program. J. Comput. Assist. Learn. 31, 314–329. doi: 10.1111/jcal.12090
Gutnick, A. L., Robb, M., Takeuchi, L., Kotler, J., Bernstein, L., and Levine, M. H. (2011). Always connected. Available online at: http://www.joanganzcooneycenter.org/wpcontent/uploads/2011/03/jgcc_alwaysconnected.pdf
Hennessey, S., D’Angelo, S., McIntyre, N., Koomar, S., Kreimeia, A., Cao, L., et al. (2021). Technology, teacher professional development and low- and middle-income countries: Technical report on systematic mapping review. London: EdTech Hub. doi: 10.53832/edtechhub.0047
Henrie, C. R., Halverson, L. R., and Graham, C. R. (2015). Measuring student engagement in technology-mediated learning: A review. Comput. Educ. 90, 36–53. doi: 10.1016/j.compedu.2015.09.005
HundrED.org (2019). Teaching at the right level (TaRL). Available online at: https://hundred.org/en/innovations/teaching-at-the-right-level-tarl#4d14df26 (accessed June 27, 2019).
Jagannathan, S. (2001). The role of nongovernmental organizations in primary education: A study of six NGOs in India (Policy Research Working Paper No. 2530). Washington, DC: Robert McNamara Fellowships Program, World Bank Institute.
Judge, S. (2005). The impact of computer technology on academic achievement of young African American children. J. Res. Child. Educ. 20, 91–101. doi: 10.1080/02568540509594554
Khandker, S. R., Koolwal, G. B., and Samad, H. A. (2010). Handbook on impact evaluation: Quantitative methods and practices. Washington, DC: World Bank. doi: 10.1596/978-0-8213-8028-4
Kim, J. S., Hemphill, L., Troyer, M., Thomson, J. M., Jones, S. M., LaRusso, M. D., et al. (2017). Engaging struggling adolescent readers to improve reading skills. Read. Res. Q. 52, 357–382. doi: 10.1002/rrq.171
Koomar, S., and Jull, S. (2020). Open educational resources in Africa: A curated resource list (Helpdesk Response No. 20). London: EdTech Hub.
Lakhsman, S. (2019). Improving reading and arithmetic outcomes at scale: Teaching at the right level (TaRL), Pratham’s approach to teaching and learning. Rev. Int. Éduc. Sèvres. Available online at: http://journals.openedition.org/ries/7470 (accessed February 15, 2021).
Lee, D., Huh, Y., Lin, C.-Y., and Reigeluth, C. M. (2018). Technology functions for personalized learning in learner-centered schools. Educ. Technol. Res. Dev. 66, 1269–1302. doi: 10.1007/s11423-018-9615-9
Leu, D. J., Gregory McVerry, J., Ian O’Byrne, W., Kiili, C., Zawilinski, L., Everett-Cacopardo, H., et al. (2011). The new literacies of online reading comprehension: Expanding the literacy and learning curriculum. J. Adolesc. Adult Lit. 55, 5–14. doi: 10.1598/JAAL.55.1.1
Ma, Y., Fairlie, R. W., Loyalka, P., and Rozelle, S. (2020). Isolating the “Tech” from edtech: Experimental evidence on computer assisted learning in China (No. w26953). Cambridge, MA: National Bureau of Economic Research. doi: 10.3386/w26953
Major, L., and Francis, G. A. (2020). Technology-supported personalised learning: A rapid evidence review (Rapid Evidence Review No. 1). London: EdTech Hub. doi: 10.5281/zenodo.4556925
McCarthy, E. M., Liu, Y., and Schauer, K. L. (2020). Strengths-based blended personalized learning: An impact study using virtual comparison group. J. Res. Technol. Educ. 52, 353–370. doi: 10.1080/15391523.2020.1716202
McHugh, D., Shaw, S., Moore, T., Ye, L., Romero-Masters, P., and Halverson, R. (2020). Uncovering themes in personalized learning: Using natural language processing to analyze school interviews. J. Res. Technol. Educ. 52, 391–402. doi: 10.1080/15391523.2020.1752337
Mo, D., Bai, Y., Shi, Y., Abbey, C., Zhang, L., Rozelle, S., et al. (2020). Institutions, implementation, and program effectiveness: Evidence from a randomized evaluation of computer-assisted learning in rural China. J. Dev. Econ. 146:102487. doi: 10.1016/j.jdeveco.2020.102487
Mo, D., Huang, W., Shi, Y., Zhang, L., Boswell, M., and Rozelle, S. (2015). Computer technology in education: Evidence from a pooled study of computer assisted learning programs among rural students in China. China Econ. Rev. 36, 131–145. doi: 10.1016/j.chieco.2015.09.001
Muralidharan, K., Niehaus, P., and Sukhtankar, S. (2017). General equilibrium effects of (improving) public employment programs: Experimental evidence from India (Working Paper No. 23838). Cambridge, MA: National Bureau of Economic Research. doi: 10.3386/w23838
Muralidharan, K., and Sheth, K. (2016). Bridging education gender gaps in developing countries: The role of female teachers. J. Hum. Resour. 51, 269–297. doi: 10.3368/jhr.51.2.0813-5901R1
Parkay, F. W., Stanford, B. H., and Gougeon, T. D. (2010). Becoming a teacher. Carmel, IND: Pearson/Merrill, 432–462.
Penuel, W. R., and Johnson, R. (2016). Review of “Continued progress: Promising evidence on personalized learning”. Boulder, CO: National Education Policy Center.
Perry, D. R., and Steck, A. K. (2015). Increasing student engagement, self-efficacy, and meta-cognitive self-regulation in the high school geometry classroom: Do iPads help? Comput. Sch. 32, 122–143. doi: 10.1080/07380569.2015.1036650
Rideout, V. (2014). “Learning at home: Families’ educational media use in America,” in Proceedings of the joan ganz cooney center at sesame workshop (New York, NY: Joan Ganz Cooney Center).
Rodriguez-Segura, D. (2021). EdTech in developing countries: A review of the evidence. World Bank Res. Obs. 37, 171–203. doi: 10.1093/wbro/lkab011
Rosas, C., and Campbell, L. (2010). Who’s teaching math to our most needy students? A descriptive study. Teach. Educ. Spec. Educ. 33, 102–113. doi: 10.1177/0888406409357537
Stošić, L., and Stošić, I. (2015). Perceptions of teachers regarding the implementation of the internet in education. Comput. Hum. Behav. 53, 462–468. doi: 10.1016/j.chb.2015.07.027
Straub, E. T. (2009). Understanding technology adoption: Theory and future directions for informal learning. Rev. Educ. Res. 79, 625–649. doi: 10.3102/0034654308325896
Tang, M., Gao, H., Zhang, Y., Liu, Y., Zhang, P., and Wang, P. (2018). Research on deep learning techniques in breaking text-based Captchas and designing image-based Captcha. IEEE Trans. Inf. Forensics Secur. 13, 2522–2537. doi: 10.1109/TIFS.2018.2821096
Tauson, M., and Stannard, L. (2018). EdTech for learning in emergencies and displaced settings: A rigorous review and narrative synthesis. London: Save the Children UK.
Waqar, Y., and Bokhari, T. B. (2019). Redesigning the design: A review of education technology interventions in Pakistan. Pan-Commonwealth Forum 9 (PCF9). Available online at: http://oasis.col.org/bitstream/handle/11599/3273/PCF9_Papers_paper_163.pdf?sequence=1&isAllowed=y
Keywords: education technology, COVID-19 school closures, teaching at the right level, randomized control trial (RCT), online education
Citation: Adil F, Nazir R and Akhtar M (2022) Investigating the impact on learning outcomes through the use of EdTech during COVID-19: Evidence from an RCT in the Punjab province of Pakistan. Front. Educ. 7:993265. doi: 10.3389/feduc.2022.993265
Received: 13 July 2022; Accepted: 19 August 2022;
Published: 21 September 2022.
Edited by:
Sajid Iqbal, Bahauddin Zakariya University, PakistanReviewed by:
Nabila Khurshid, COMSATS University Islamabad, PakistanCopyright © 2022 Adil, Nazir and Akhtar. This is an open-access article distributed under the terms of the Creative Commons Attribution License (CC BY). The use, distribution or reproduction in other forums is permitted, provided the original author(s) and the copyright owner(s) are credited and that the original publication in this journal is cited, in accordance with accepted academic practice. No use, distribution or reproduction is permitted which does not comply with these terms.
*Correspondence: Rabia Nazir, cmFiaWEubmF6aXJAaXViLmVkdS5waw==
Disclaimer: All claims expressed in this article are solely those of the authors and do not necessarily represent those of their affiliated organizations, or those of the publisher, the editors and the reviewers. Any product that may be evaluated in this article or claim that may be made by its manufacturer is not guaranteed or endorsed by the publisher.
Research integrity at Frontiers
Learn more about the work of our research integrity team to safeguard the quality of each article we publish.