- 1Education and Educational Psychology, Department of Psychology, Ludwig-Maximilians-Universität in Munich, Munich, Germany
- 2Institute for Medical Education, University Hospital, Ludwig-Maximilians-Universität in Munich, Munich, Germany
Research on diagnosing in teacher education has primarily emphasized the accuracy of diagnostic judgments and has explained it in terms of factors such as diagnostic knowledge. However, approaches to scientific argumentation and information processing suggest differentiating between diagnostic judgment and diagnostic argumentation: When making accurate diagnostic judgments, the underlying reasoning can remain intuitive, whereas diagnostic argumentation requires controlled and explicable reasoning about a diagnostic problem to explain the reasoning in a comprehensible and persuasive manner. We suggest three facets of argumentation for conceptualizing diagnostic argumentation, which are yet to be addressed in teacher education research: justification of a diagnosis with evidence, disconfirmation of differential diagnoses, and transparency regarding the processes of evidence generation. Therefore, we explored whether preservice teachers’ diagnostic argumentation and diagnostic judgment might represent different diagnostic skills. We also explored whether justification, disconfirmation, and transparency should be considered distinct subskills of preservice teachers’ diagnostic argumentation. We reanalyzed data of 118 preservice teachers who learned about students’ learning difficulties with simulated cases. For each student case, the preservice teachers had to indicate a diagnostic judgment and provide a diagnostic argumentation. We found that preservice teachers’ diagnostic argumentation seldom involved all three facets, suggesting a need for more specific training. Moreover, the correlational results suggested that making accurate diagnostic judgments and formulating diagnostic argumentation may represent different diagnostic skills and that justification, disconfirmation, and transparency may be considered distinct subskills of diagnostic argumentation. The introduced concepts of justification, disconfirmation, and transparency may provide a starting point for developing standards in diagnostic argumentation in teacher education.
Introduction
Diagnostic skills are relevant in many fields, one of which is teacher education (Heitzmann et al., 2019). Teachers’ diagnosing is a prototypical practice scenario for evidence-oriented practice, and as such, it is crucial for teachers’ professionalism (Fischer, 2021). Previous research on teachers’ diagnosing has primarily investigated diagnostic accuracy—i.e., the correctness of diagnostic judgments—because inaccurate judgments can easily disadvantage students by, for example, leading to unsuitable or insufficient educational interventions (e.g., Loibl et al., 2020; Urhahne and Wijnia, 2020; Kramer et al., 2021a). Besides making accurate diagnostic judgments, communicating diagnostic considerations is another vital aspect of diagnostic skills, for example, for purposes such as reporting diagnostic findings (Bauer et al., 2020) or collaborative diagnosing (Kiesewetter et al., 2017). However, thus far, there is no clear conceptualization of diagnostic argumentation, which we define as explaining a diagnostic judgment and the underlying reasoning comprehensibly and persuasively (see Walton, 1990; Berland and Reiser, 2009). It is also unclear whether professionals (e.g., teachers) who can make accurate diagnostic judgments are capable of offering sufficient diagnostic argumentation. This raises the question of whether accurate diagnostic judgment and diagnostic argumentation are fully based on the same knowledge—reflecting one overarching diagnostic skill—or whether they need to be considered different subskills of diagnosing. This differentiation might have implications for teaching diagnostic skills, such as the definition of learning objectives and the design and implementation of learning environments (see Grossman et al., 2009).
To our knowledge, no systematic research has differentiated between the concepts of diagnostic argumentation and diagnostic judgment. Therefore, we propose a conceptualization of diagnostic argumentation that consists of three facets: justification of a diagnosis with evidence, disconfirmation of differential diagnoses, and transparency regarding the processes of evidence generation. We explore diagnostic argumentation in terms of these three facets and investigate whether they indicate one joint underlying skill or different aspects of diagnostic skills by analyzing their interrelations with one another and with a potentially joint knowledge base. We also explore how justification, disconfirmation, and transparency in diagnostic argumentation are related to the accuracy of diagnostic judgments in the context of teacher education.
Diagnosing in teacher education
Teacher education is one of the fields in which learning diagnostic skills is an important matter of professionalization (Grossman, 2021). In particular, teachers have to diagnose students’ performance, progress, and learning prerequisites (e.g., Praetorius et al., 2013; Südkamp et al., 2018). However, these aspects also include the initial identification of clinical problems, such as learning difficulties (e.g., dyslexia) and behavioral disorders (e.g., attention deficit hyperactivity disorder, i.e., ADHD; e.g., Poznanski et al., 2021). In all these contexts, we broadly define diagnosing as a “goal-oriented collection and interpretation of case-specific or problem-specific information to reduce uncertainty in order to make […] educational decisions” (Heitzmann et al., 2019, p. 4). Other associated terms are used for diagnosing in teacher education as well, such as assessment (e.g., Herppich et al., 2018). As part of teachers’ professional activities, diagnosing is crucially related to the discussion around teachers’ evidence-oriented practice (Stark, 2017) and is possibly a prototypical practice scenario (Fischer, 2021). Teachers are expected to use knowledge on theories, methods, procedures, and findings from educational research (e.g., Kiemer and Kollar, 2021) to reflect their experiences, possibly overcome dysfunctional intuitive approaches and—at least partially—guide their diagnostic activities and interventions. Teacher education programs are increasingly acknowledging the relevance of facilitating diagnostic skills, and research in teacher education has also addressed the issue of how diagnostic skills are learned (e.g., Chernikova et al., 2020; Loibl et al., 2020; Sailer et al., 2022).
Teachers’ diagnostic judgments
Previous research on teachers’ diagnosing has focused on how teachers make diagnostic judgments (e.g., Loibl et al., 2020; Urhahne and Wijnia, 2020; Kramer et al., 2021a). Loibl et al. (2020) suggested distinguishing between the processes and products of teachers’ diagnostic judgments In terms of product indicators, research on teachers’ diagnostic judgments has focused on diagnostic accuracy—i.e., the correctness of diagnostic judgments—because inaccurate judgments can lead to unsuitable or insufficient educational interventions that easily disadvantage students (e.g., Urhahne and Wijnia, 2020). There is also an increasing amount of research investigating teachers’ judgment processes, for example, in terms of diagnostic activities such as generating hypotheses, generating and evaluating evidence, and drawing conclusions (e.g., Wildgans-Lang et al., 2020; Codreanu et al., 2021; Kramer et al., 2021a). In addition, research has begun to focus more on the role of information processing in teachers’ judgment processes (e.g., Loibl et al., 2020). Teachers’ diagnostic judgment processes can involve intuitive information processing—i.e., fast recognition of patterns of information—which facilitates flexible and adaptive acting in the classroom; teachers can also engage in controlled information processing when spending time and effort on consciously evaluating evidence and its causal relations (Kahneman, 2003; Evans, 2008). Teachers’ information processing in making diagnostic judgments depends on situational characteristics (Loibl et al., 2020), such as the available time for making a judgment (Rieu et al., 2022), the consistency and conclusiveness of the available evidence, and teachers’ perceptions of their situational accountability (Pit-ten Cate et al., 2020). In classrooms with multiple students, teachers often need to make intuitive judgments, prioritize tasks, and decide where to invest their time and cognitive resources (Feldon, 2007; Vanlommel et al., 2017). With respect to achieving diagnostic accuracy, research suggests regarding judgment processes (e.g., in terms of information processing) as processes that interact with teachers’ characteristics, especially their diagnostic knowledge (e.g., Loibl et al., 2020; Kramer et al., 2021a).
The role of diagnostic knowledge
Diagnostic knowledge is generally considered an important basis of diagnostic skills (Heitzmann et al., 2019). Having a sufficient base of specific diagnostic knowledge seems to be a necessary condition for achieving accurate diagnostic judgments (Kolovou et al., 2021). In addition, advanced diagnosticians’ well-organized knowledge structures enable them to recognize patterns of critical case information correctly, without necessarily conducting a controlled analysis of the underlying causal relations (see Kahneman, 2003; Evans, 2008; Boshuizen et al., 2020). Research has suggested that performing complex cognitive tasks requires not only knowledge about relevant concepts but also knowledge about how to systematically approach the task (e.g., Van Gog et al., 2004). In the context of teacher education, Shulman (1986) suggested that, besides domain-specific content, distinguishing between different types of knowledge—such as conceptual and strategic knowledge—is relevant to capturing different functionalities of knowledge, such as acting adaptively in response to various problems and situations. In the course of developing strategic knowledge, basic aspects of conceptual knowledge are abstracted and integrated with episodic knowledge into cognitive scripts about approaching certain problems or situations (e.g., Shulman, 1986; Schmidmaier et al., 2013; Boshuizen et al., 2020). This means that conceptual and strategic knowledge about the same specific content are likely related but address different aspects of solving a task. Conceptual and strategic knowledge have been adapted and empirically investigated in the context of diagnosing in medical education (e.g., Stark et al., 2011; Schmidmaier et al., 2013): conceptual diagnostic knowledge (CDK) consists of concepts, such as diagnoses and their relations with each other and with evidence, whereas strategic diagnostic knowledge (SDK) refers to how to proceed in diagnosing a specific problem (i.e., how to reject or confirm differential diagnoses and which informational sources provide critical evidence for doing so). Researchers addressing diagnosing in teacher education have also suggested distinguishing between CDK and SDK (e.g., Förtsch et al., 2018). Therefore, CDK and SDK seem crucial for correctly processing relevant case information and making accurate diagnostic judgments.
Diagnostic argumentation
Beyond making accurate diagnostic judgments, there are instances in which teachers or other diagnosticians need to explain their reasoning and the resulting diagnostic judgment in a comprehensible and persuasive manner, which we suggest to designate as diagnostic argumentation (see Walton, 1990; Berland and Reiser, 2009). Diagnostic argumentation is required in situations in which explanations are directed toward a recipient, such as a collaborating teacher or school psychologist (e.g., Kiesewetter et al., 2017; Csanadi et al., 2020; Radkowitsch et al., 2021). The context of identifying students’ clinical problems is one example in which diagnostic argumentation is particularly relevant for teachers, as in many educational systems, final judgments about clinical diagnoses are made by clinical professionals (e.g., school psychologists), with whom teachers might need to collaborate (Albritton et al., 2021). However, also in other contexts, diagnostic argumentation facilitates a collaborative process of considering and reconciling competing explanations and thus, if necessary, can help improve the diagnosing (see Berland and Reiser, 2009; Csanadi et al., 2020). There are also nonimmediate dialogical situations (see Walton, 1990), such as writing a report about diagnostic findings (Bauer et al., 2020), in which information may need to be comprehensible and persuasive to potential recipients at a later point in time.
Especially when engaging in a face-to-face critical exchange of arguments in collaborative or otherwise dialogical diagnosing, teachers might involve in argumentation processes and a controlled analysis of the available evidence and potential explanations before making a diagnostic judgment. Collaborative generation and evaluation of evidence and a critical evaluation of others’ arguments can improve the quality of argumentative outcomes (Mercier and Sperber, 2017; Csanadi et al., 2020). In other contexts, teachers might make intuitive judgments without a controlled analysis of all the available evidence and causal relations. If the information processing for a diagnostic judgment mainly involves intuitive pattern recognition, parts of the reasoning can remain implicit (Evans, 2008). However, comprehensively explaining a judgment and its underlying reasoning initially requires that the reasoning be explicable or at least constructible in retrospect. In terms of nondialogical situations, such as writing reports, initial evidence suggests that compared to medical education, there seems to be a lower standardization in teacher education (Bauer et al., 2020), which could facilitate constructing persuasive explanations in retrospect. For these reasons, it might not necessarily be a given that teachers who make accurate judgments in nondialogical diagnostic situations are capable of subsequently providing comprehensible and persuasive explanations of their reasoning. This open question has yet to be explored by research.
Justification, disconfirmation, and transparency in diagnostic argumentation
To explore how diagnostic judgment and diagnostic argumentation are related, it is first necessary to define what kind of information is expected to be provided in the context of diagnostic argumentation. We argue that besides providing comprehensible explanations, diagnostic argumentation also aims to persuade potential recipients of the presented reasoning (Berland and Reiser, 2009) and, thus, requires providing information that enables a recipient’s understanding and evaluation of the efforts made during diagnosing (see Chinn and Duncan, 2018). Therefore, to further define the concept of diagnostic argumentation, we suggest three facets that might facilitate recipients’ understanding of the presented reasoning: justification, disconfirmation, and transparency. We propose that these three facets of diagnostic argumentation resemble approaches in scientific argumentation (see Sampson and Clark, 2008; Mercier and Heintz, 2014), namely justifying one’s reasoning with evidence (e.g., Toulmin, 1958), considering and disconfirming alternative explanations (e.g., Lawson, 2003), and emphasizing the credibility of informational sources with methodological transparency (e.g., Chinn et al., 2014). In what follows, we explain the three facets in further detail.
Justification denotes the provision of evidence in support of a claim (e.g., Toulmin, 1958; Hitchcock, 2005), which allows recipients to raise potential issues about the reasoning that was presented. In the context of diagnostic argumentation, diagnostic judgments are claims that need to be justified by providing evidence derived from the case information. Therefore, justifications evaluate relevant case information as evidence from which to draw conclusions concerning a judgment (see Fischer et al., 2014).
Disconfirmation emphasizes discussing differential diagnoses that may have been hypothesized when diagnosing a given case. As a process of uncertainty reduction (Heitzmann et al., 2019), diagnosing involves generating and evaluating different hypotheses (Klahr and Dunbar, 1988; Fischer et al., 2014) that resemble competing claims in argumentation. Similar to the scientific approach of disconfirmation (e.g., Gorman et al., 1984), a rebuttal of competing claims supports the persuasiveness of the final claim (e.g., Toulmin, 1958; Lawson, 2003). In diagnostic argumentation, differential diagnoses are competing claims that should be explicated and discussed to facilitate the persuasiveness of the final judgment by demonstrating that alternative explanations have been considered. Recipients can build on this information to evaluate and criticize whether relevant differential diagnoses have been missed or mistakenly rejected.
Transparency regarding the processes of evidence generation provides information about the reliability of the methodology for generating evidence from informational sources (Chinn et al., 2014; Fischer et al., 2014). In diagnostic argumentation, transparency is achieved by describing the processes underlying evidence generation, thus allowing recipients to evaluate the presented evidence and diagnostic conclusions. Explicating how evidence was generated facilitates a recipient’s understanding and ability to criticize the quality of the evidence and, ultimately, the validity of the conclusions (Vazire, 2017).
Analogously to approaches involved in scientific argumentation (see Sampson and Clark, 2008; Mercier and Heintz, 2014), we suggest that justification, disconfirmation, and transparency in diagnostic argumentation facilitate a recipient’s understanding and evaluation of the efforts made during diagnosing. We are unaware of any research in teacher education that has conceptualized or investigated a skill similar to what we have defined as diagnostic argumentation, including the facets of justification, disconfirmation, and transparency. Therefore, in this study, we aimed to explore the interrelations between justification, disconfirmation, and transparency in diagnostic argumentation, as well as their relations with making accurate diagnostic judgments, and the explanatory roles of CDK and SDK (see Figure 1).
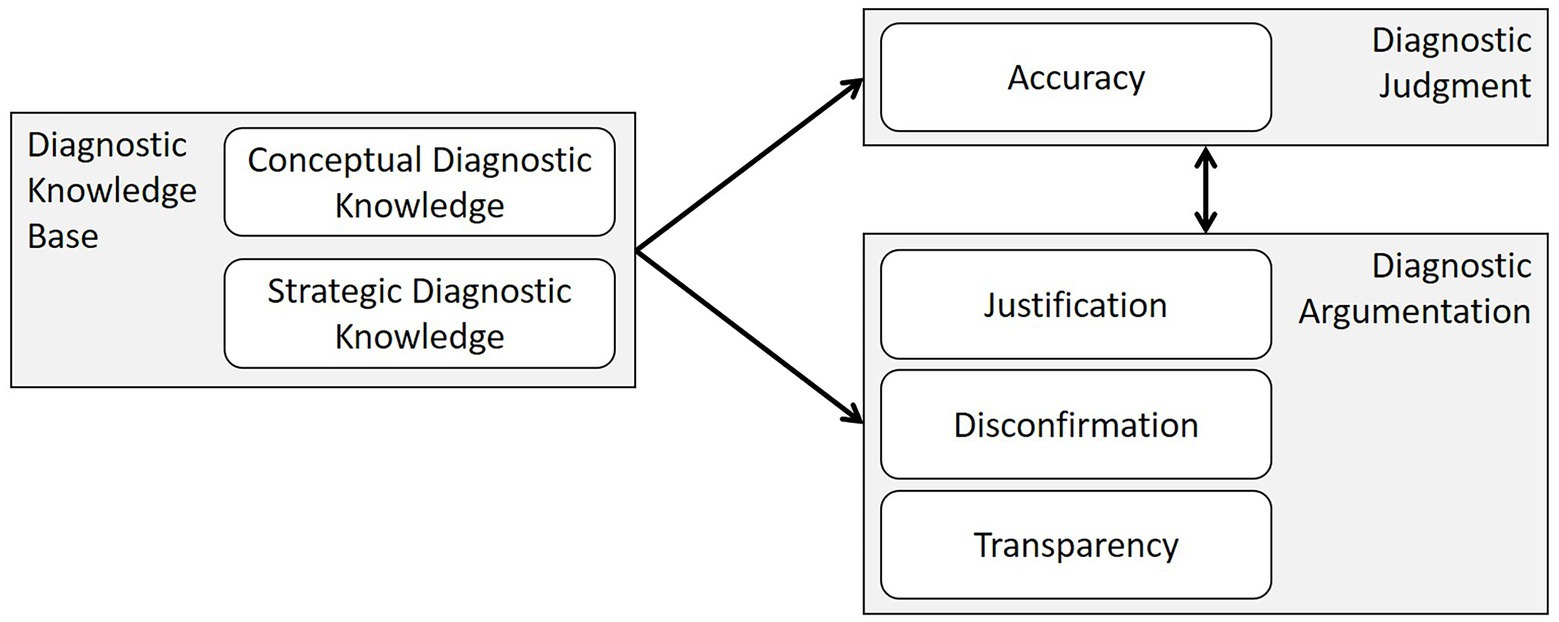
Figure 1. Theoretical model of the potential relationships between diagnostic knowledge and the skills of diagnostic judgment and diagnostic argumentation.
Research questions
We propose that justification, disconfirmation, and transparency are three relevant facets of diagnostic argumentation and that diagnostic argumentation and diagnostic judgment might represent two distinct diagnostic skills that may, however, both be partially explained by CDK and SDK. Understanding the interrelations between these skills and knowledge might provide relevant information for teacher educators and the field of teacher education.
In investigating the proposed concept of diagnostic argumentation, it is also important to explore whether justification, disconfirmation, and transparency might represent distinct subskills or indicators of one joint underlying diagnostic skill (RQ1). To approach this question, we investigated how the individual facets (1a) and different combinations of the facets (1b) occur within preservice teachers’ diagnostic argumentation and analyzed the facets’ relations (1c) in preservice teachers’ diagnostic argumentation. We assumed that finding close relationships would indicate a joint basis of knowledge and skills; by contrast, small relationships or a lack thereof would indicate that the three facets represent different subskills of diagnostic argumentation.
In terms of distinguishing between the three facets as different subskills, a related question is to what extent justification, disconfirmation, and transparency are based on conceptual diagnostic knowledge and strategic diagnostic knowledge (RQ2). Because CDK and SDK are thought to be a major basis for the reasoning presented in diagnostic argumentation (Heitzmann et al., 2019), we assumed that they also partially explain justification, disconfirmation, and transparency; that is, CDK and SDK may be needed to generate evidence from informational sources (explicated in transparency) and to make a warranted connection between the evidence and a diagnosis (explicated in justification) or several differential diagnoses (explicated in disconfirmation). Exploring the degree to which CDK and SDK explain justification, disconfirmation, and transparency in diagnostic argumentation can provide an initial basis for future research on teachers’ prerequisites for diagnostic argumentation. Given that diagnostic argumentation additionally aims to be persuasive instead of solely verbalizing the reasoning made while processing information, further knowledge and skills beyond CDK and SDK may contribute to justification, disconfirmation, and transparency in diagnostic argumentation.
For the same reason, we assumed that, despite a presumably joint basis of CDK and SDK, diagnostic accuracy might not necessarily be related to justification, disconfirmation, and transparency. Therefore, we explored whether diagnostic judgment (indicated by diagnostic accuracy) and diagnostic argumentation (indicated by justification, disconfirmation, and transparency) might represent different diagnostic skills (RQ3). In doing so, we assumed that identifying close relationships would indicate a joint underlying diagnostic skill; by contrast, small relationships or a lack thereof would indicate that diagnostic argumentation and diagnostic judgment might represent different diagnostic skills.
Materials and methods
Participants
In this study, we reanalyzed data that were originally collected to train an AI-based adaptive feedback component for a simulation-based learning environment (see Pfeiffer et al., 2019). A total of 118 preservice teachers participated in the data collection and processed simulated cases pertaining to students’ clinical problems. Participants were M = 22.96 years old (SD = 4.10), the majority were women (102 women, 15 men, and 1 nonbinary), and they were in their first to 13th semester (M = 4.62, SD = 3.40) of a teacher education program. We recruited preservice teachers in all semesters because relevant courses about students’ clinical problems were not compulsory or bound to a specific semester but could be taken in any semester. Participants subjectively rated their prior knowledge of students’ clinical problems prior to receiving any instruction about the content of the study. On average, they indicated a medium rating of their own prior knowledge (on a rating scale ranging from 1 to 5 points: prior knowledge about ADHD, M = 2.78, SD = 0.81; prior knowledge about dyslexia, M = 2.47, SD = 0.76). We assumed that this sample mirrors the diverse population of preservice teachers.
Research design
We chose a quantitative and correlational research design to determine the relationships between the following variables: justification, disconfirmation, and transparency in diagnostic argumentation; CDK and SDK; and the accuracy of diagnostic judgment.
Simulation and tasks
We asked participants to take on the role of a teacher and process eight cases of primary and secondary students with performance-related or behavioral problems that might or might not indicate a clinical diagnosis in the range of ADHD or dyslexia. Two independent domain experts, one school psychologist and one psychotherapist for children and adolescents, validated the case materials before they were implemented in CASUS, a case-based online learning environment.1 Participants solved the cases consecutively. The cases included several informational sources, such as samples of the students’ written exercises and school certificates, reports of observations from inside and outside the classroom, and conversations with the respective students, their parents, and other teachers (the German-language case materials can be accessed at https://osf.io/hn7wm/). Participants could freely choose how many and which informational sources to consult and in which order they wanted to do so (see Figure 2).
One example is the case of a secondary school student named Anna, who is showing symptoms of attention-deficit disorder (ADD). An initial problem statement describes Anna as a fifth-grade student, 11 years old, who constantly needs to be pushed to finish her tasks and who has poor grades in many subjects, especially the core subjects, such as math and the language subjects. The learners could examine written observations of Anna’s in-class and out-of-class behavior, read recordings of conversations with Anna or with her parents and several teachers, or look at Anna’s last annual report and an example of a written exercise. Her behavior is described as very calm and distracted. She reads very slowly, and it is difficult for her to answer questions about a text that she has just read. She often fails to follow the exact instructions for tasks or fails to complete them fully. Moreover, she often does not bring all the required school supplies or arrives late in the morning. At a parent–teacher conference, Anna’s mother backs up the impression of disorganized and slow learning behavior when talking about Anna’s homework. Anna’s last annual report and the conversations with the other teachers show that her grades are also affected by her inattentiveness, except artistic subjects and gym class. She mostly interacts with one friend and tends to remain distant from the other students. Anna herself points out that it is hard for her to concentrate because she feels easily distracted. However, at home, where there are fewer ambient noises, she can focus on and enjoy reading, drawing, and painting. Overall, the case information is designed in such a way that the diagnosis of ADD is the most likely diagnosis, despite the fact that several differential diagnoses may be relevant. The other cases included the same kinds of informational sources as Anna’s case.
To complete a case and move on to the next case, participants had to complete two tasks. First, they had to make a diagnostic judgment, answering the question of whether the simulated student has issues that warrant further diagnosing of a clinical problem and, if so, which diagnosis may apply. Second, we asked participants to write an argumentation text about their conclusions and their reasoning about the case. For the purpose of this study, participants received no further guidance or support regarding how to write their diagnostic argumentation.
Procedure
The data were collected on computers in a laboratory setting, with three to 20 participants simultaneously joining the study. They worked individually at separate desks and were not permitted to speak to each other. We introduced the participants to the aims and procedure of the study and familiarized them with the learning environment. After giving informed consent to participate in the study, participants received randomly assigned codes to log on to the CASUS learning environment to anonymize the data. When entering the online learning environment, participants first received a 25 min theoretical input concerning the topic of diagnosing in general and the diagnosing of ADHD and dyslexia in particular to activate existing knowledge and ensure the minimum amount of knowledge required for solving the cases. Participants were not allowed to take any notes or go back to the input part at a later point to avoid biases in subsequent testing and learning. Following the theoretical input, participants spent around 25 min on a pretest that assessed their CDK and SDK. Subsequently, participants entered the learning phase consisting of the eight simulated cases, with a break of 10 min after four cases. They had to finish one case at a time to gain access to the next case. All participants received the cases in the same sequence. The time on task for all cases was around 1 h. Subsequently, participants spent around 25 min on a posttest. Generally, participants were allowed to work at their own pace. Overall, participants spent around 3 h from login to logout. During the study, researchers were available to help with technical issues or questions about navigation but did not answer any content-related questions. Participants received monetary compensation of 35 euros.
Data sources and measurements
The data sources used for the presented analyses are the CDK and SDK scores from the pretest as well as the written diagnostic judgments and diagnostic argumentation texts from six of the eight cases. We decided to exclude two cases from the analysis because their case information turned out to be more ambiguous and inconclusive compared to the other cases.
Diagnostic knowledge
Conceptual diagnostic knowledge
CDK was assessed in the pretest after participants received the theoretical input. We used 14 single-choice items about diagnosing ADHD and dyslexia with four answer options each (one correct answer and three distractors). The CDK questionnaire was developed prior to the study to assess participants’ CDK, which was considered relevant for processing the simulated cases. Two independent domain experts, one school psychologist and one psychotherapist for children and adolescents, validated the CDK questionnaire. One example item is “Which of the following is not one of the cardinal symptoms of ADHD?” with the answer options (a) Inattentiveness, (b) Hyperactivity, (c) Impulsivity, and (d) Impatience. Participants received one point per correct answer. The points were aggregated into a total score, ranging from 0 to 14 points, for CDK.
As suggested by Stadler et al. (2021; see also Diamantopoulos and Siguaw, 2006; Taber, 2018), we calculated variance inflation factors (VIFs) for all items to avoid having redundant items representing the formative knowledge construct. The maximum VIF was VIFmax = 1.30, which is well below the recommended cut-off of 3.3.
Strategic diagnostic knowledge
Subsequent to assessing CDK, we measured SDK using four key-feature cases (two key-feature cases about ADHD and two about dyslexia) with two multiple-choice questions each (see Page et al., 1995). Key-feature cases present a brief description consisting of a few sentences before asking about the strategic approaches used to diagnose the case. The key-feature cases were developed prior to the study to assess participants’ SDK, which was considered relevant for processing the simulated cases. Two independent domain experts, one school psychologist and one psychotherapist for children and adolescents, validated the key-feature cases. One example key-feature case introduced the fourth grader Luis, who has always been a rather poor reader but has begun to fall farther behind his classmates over the last few months and just recently again received the lowest grade in the class on a reading test. He cannot summarize the contents of a short text even immediately after reading it and can only read aloud very slowly. Apart from his performance issues, he has a chronic disease due to which he cannot regularly attend school for stretches of several weeks. After reading this brief case description, two multiple-choice questions were asked.
The first of the two multiple-choice questions per key-feature case asked participants to choose all relevant differential diagnoses out of a list of clinical as well as non-clinical differential diagnoses (one to three correct options out of seven to nine answer options). Participants received points for correctly choosing relevant options and not choosing irrelevant options. We calculated one mean score across all options per key-feature case, resulting in a diagnosis score of 0 to 1 for the first question for each key-feature case.
The second of the two multiple-choice questions per key-feature case asked participants to choose from a list of further approaches and resources relevant to confirm or disconfirm a given set of differential diagnoses (three to six correct options out of seven to 10 answer options). Participants received points for correctly choosing relevant options and not choosing irrelevant options. We calculated one mean score across all options per key-feature case, resulting in a resource score of 0 to 1 for the second question for each key-feature case.
The four diagnosis scores and four resources scores were accumulated into a total score of 0 to 8 points for SDK on the pretest. There were no redundant items (VIFmax = 1.09).
Accuracy of diagnostic judgment
To measure diagnostic accuracy, we coded all the written diagnoses as accurate (1 point), partially accurate (0.5 points), or inaccurate (0 points). We coded written diagnoses as accurate if indicating a diagnosis that was considered the correct solution when designing the cases (e.g., ADD for the case Anna). The written diagnoses were coded as partially accurate if correctly indicating the higher-level class of diagnoses for the accurate diagnosis (e.g., if the correct diagnosis was ADD and the participants indicated ADHD). A total of 12.5% of the diagnoses were double-coded, resulting in an interrater reliability (IRR) of Cohen’s κ = 0.80 (Cohen, 1960). The internal consistency across the six cases was McDonald’s ω = 0.37 (McDonald, 1999). For further analyses, we calculated a total score from the points achieved for diagnostic accuracy with a possible range of 0 to 6 points.
Justification, disconfirmation, and transparency in diagnostic argumentation
We operationalized justification, disconfirmation, and transparency based on a coding of the six cases’ diagnostic argumentation texts.
Justification
We operationalized the presence or absence of justification in diagnostic argumentation as evaluating evidence co-occurring with drawing conclusions within the temporal context of two sentences, resulting in 1 or 0 points per diagnostic argumentation. In this study, we reanalyzed data that were originally used to train an AI-based adaptive feedback algorithm for a simulation-based learning environment (see Pfeiffer et al., 2019). Four expert raters coded the diagnostic argumentation texts segmented by sentences regarding the categories evaluating evidence and drawing conclusions. They initially read the complete diagnostic argumentation before coding evaluating evidence and drawing conclusions for the individual sentences. Evaluating evidence was defined as explicitly presenting or interpreting case information (e.g., “Markus behaves aggressively and gets offended very easily”). Drawing conclusions was defined as explicitly accepting or rejecting at least one diagnosis (e.g., “I think most likely the diagnosis is ADHD”). The raters simultaneously coded 15% of the data before dividing the rest of the data because of substantial agreement (IRRs: Fleiss’ κ = 0.71 for drawing conclusions; Fleiss’ κ = 0.75 for evaluating evidence; Fleiss, 1971; Landis and Koch, 1977). The internal consistency across six cases was sufficient (McDonald’s ω = 0.60; McDonald, 1999). We calculated a total justification score for each participant, with a possible range of 0 to 6 points.
Disconfirmation
We operationalized disconfirmation as present if two or more differential diagnoses were addressed, resulting in 1 or 0 points per diagnostic argumentation. This round of coding was done separately from the coding of justification and transparency for the purpose of our reanalysis. Two expert raters coded the diagnostic argumentation texts of six cases regarding a set of differential diagnoses. The coding scheme consisted of 27 differential diagnoses, which included non-clinical (e.g., insufficient schooling, emotional stress, and problematic home environment) and clinical differential diagnoses (e.g., ADHD, ADD, dyslexia, and autism). The raters considered the facet of disconfirmation as being included in the diagnostic argumentation if two or more of these differential diagnoses were discussed in one diagnostic argumentation, independent of which diagnosis the participant indicated as the final diagnosis. The raters simultaneously coded 15% of the data before dividing the rest of the data (overall IRR: Cohen’s κ = 0.92; Cohen, 1960). The internal consistency was sufficient (McDonald’s ω = 0.60; McDonald, 1999). We calculated a total disconfirmation score for each participant, with a possible range of 0 to 6 points.
Transparency
We operationalized transparency in diagnostic argumentation as at least one explication of generating evidence, resulting in 1 or 0 points per diagnostic argumentation. The coding for transparency was done in in the same round as the coding for justification. Four expert raters coded the diagnostic argumentation texts regarding generating evidence, which was defined as an explicit description of accessing informational sources (i.e., tests or observations; e.g., “I observed Anna’s school-related behavior and achievement”). The raters simultaneously coded 15% of the data before dividing the rest of the data because of substantial agreement (IRR: Fleiss’ κ = 0.70; Landis and Koch, 1977). The internal consistency was sufficient (McDonald’s ω = 0.71; McDonald, 1999). We calculated a total transparency score for each participant, with a possible range of 0 to 6 points.
Statistical analyses
For RQ1, we explored the descriptive statistics of justification, disconfirmation, and transparency in preservice teachers’ diagnostic argumentation texts in terms of both individual facets (1a) and facet combinations (1b). We considered facet combinations as types of argumentation texts and depicted them in relation to the individual facets using Epistemic Network Analysis (ENA; Shaffer, 2017). The ENA algorithm analyzes and accumulates co-occurrences of elements in coded data, such as the three facets of argumentation within individual argumentation texts, to create a multidimensional network model, which is depicted as a dynamic network graph. To determine the types of argumentation texts, we grouped the argumentation texts according to the presence or absence of each argumentation facet in each argumentation text. The ENA algorithm then accumulated co-occurrences of the three facets across the argumentation texts to create a network model. We depicted this model as a two-dimensional network graph that showed the relative location of the argumentation types within the resulting two-dimensional space. We used the ENA online tool to create the network graphs.2 In addition to the descriptive analyses, we calculated Pearson correlations with participants’ overall justification, disconfirmation, and transparency scores (1c). To investigate RQ2, we calculated a multivariate multiple linear regression with the predictors CDK and SDK and the dependent variables justification, disconfirmation, and transparency. For RQ3, we first created two separate ENA networks by grouping the diagnostic argumentation texts that addressed either accurate or inaccurate diagnostic judgments; we tested the difference between the group means’ locations in the network space using a t-test. To facilitate the statistical testing of the groups’ network differences, we used the option of means rotation, which aligns the two group means on the X-axis of the network, thus, depicting systematic variance in only one dimension in the two-dimensional space (Shaffer, 2017). Moreover, we again calculated Pearson correlations, including the participants’ overall scores for diagnostic accuracy, justification, disconfirmation, and transparency. We also explored partial correlations, controlling for CDK and SDK. For RQ1c and RQ3, including multiple comparisons (three Pearson correlations each), the significance level was Bonferroni-adjusted to α = 0.0167 (α = 0.05/3). For the other analyses, the significance level was set to α = 0.05.
Results
RQ1: Justification, disconfirmation, and transparency
To investigate whether justification, disconfirmation, and transparency represent distinct subskills or one joint underlying diagnostic skill (RQ1), we analyzed the prevalence of the individual facets (1a) and the combinations of the facets (1b) in preservice teachers’ individual argumentation texts. Moreover, we analyzed the relationships between justification, disconfirmation, and transparency in preservice teachers’ diagnostic argumentation (1c). We considered findings of close relations to indicate a joint basis of knowledge and skills, and small or no relations to indicate that the three facets represent different aspects of diagnostic skills.
RQ1a: Prevalence of the facets in preservice teachers’ argumentation texts
Analyzing the descriptive statistics of the prevalence of justification, disconfirmation, and transparency in preservice teachers’ individual argumentation texts, we found that justification was the most common of the three facets in all diagnostic argumentation texts (see Table 1): Participants explicitly stated conclusions and justified them by evaluating evidence alongside the conclusion in 66% (M = 0.66; SD = 0.47) of all argumentation texts. Disconfirmation was found in 26% (M = 0.26; SD = 0.44) of all diagnostic argumentation texts, indicating that the majority of diagnostic argumentation texts did not involve differential diagnoses but tended to focus on one final diagnosis. Moreover, we found transparency concerning the processes of evidence generation in 46% (M = 0.46; SD = 0.50) of all argumentation texts, indicating that approximately half of the diagnostic argumentation texts explained the processes of evidence generation.

Table 1. Prevalence of the individual facets justification, disconfirmation, and transparency in the 709 diagnostic argumentation texts.
RQ1b: Combinations of the facets in preservice teachers’ argumentation texts
Descriptive statistics of the combinations of justification, disconfirmation, and transparency are outlined in Table 2. The combinations of the three facets can be considered different types of diagnostic argumentation texts, which we distinguished using the following abbreviations: J indicates the presence of justification, D indicates the presence of disconfirmation, T indicates the presence of transparency, and O indicates the absence of a facet (e.g., JOT indicates justification and transparency without disconfirmation; see Table 2 for all argumentation types and their prevalence). A notable pattern was that argumentation texts addressing more than one diagnosis usually discussed the different diagnoses by evaluating evidence to make and justify conclusions (JDT and JDO), whereas hardly any argumentation texts addressed differential diagnoses without making and justifying related conclusions (ODT and ODO). However, diagnostic argumentation texts frequently presented a confirmatory justification of a single diagnosis without discussing alternative explanations (JOT and JOO). Consequently, including disconfirmation in diagnostic argumentation was dependent on including justification, but justification in diagnostic argumentation was not dependent on including the facet of disconfirmation, suggesting a relationship of unidirectional dependency.
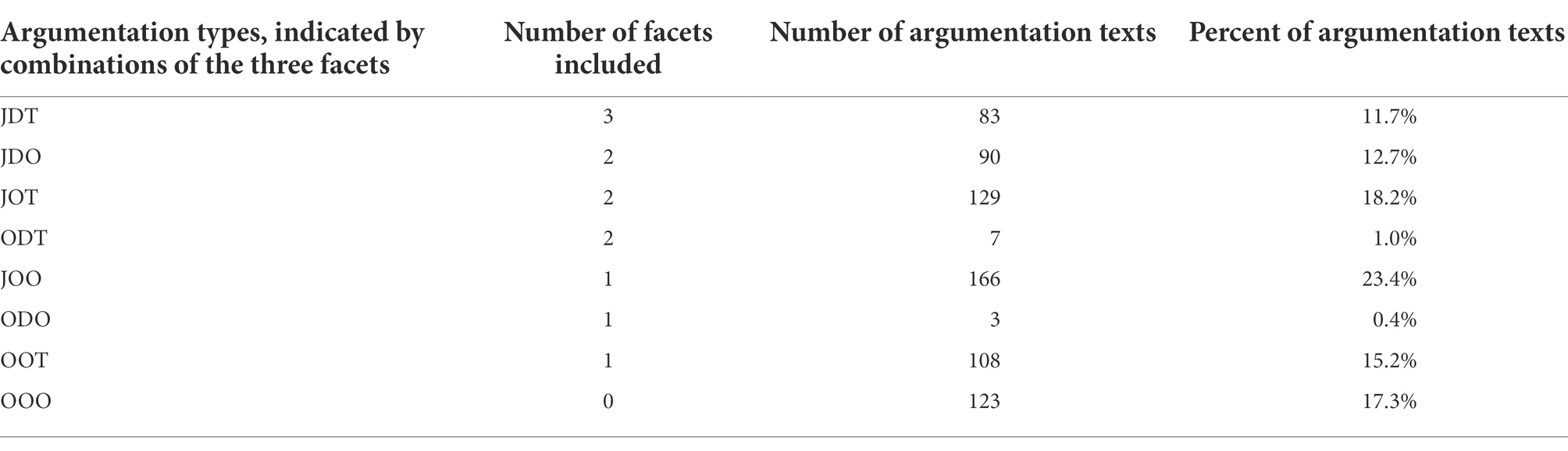
Table 2. Prevalence of the argumentation types, indicated by combinations of the facets Justification (J), Disconfirmation (D), and Transparency (T), in the 709 argumentation texts.
To illustrate the types of argumentation texts and their relationships with the individual facets, we used ENA to plot both the argumentation types (indicated by colored squares) and the individual facets (indicated by gray nodes) in a two-dimensional space (see Figure 3). The two-dimensional space was built based on the co-occurrences of two argumentation facets each, which are indicated by the blue lines. The thickness of the blue lines represents the relative frequency of the co-occurrences (e.g., the thick line between justification and transparency relates to the 212 co-occurrences of justification and transparency in JDT and JOT). The positioning of argumentation types (indicated by the colored squares) along the X-axis is relative to the facets’ co-occurrences, which is why JOT is located toward the right-sided node of transparency and JDO is located toward the left-sided node of disconfirmation. The central positioning of justification is due to its high overall prevalence (see Table 1). The positioning of argumentation types along the Y-axis indicates the argumentation texts’ comprehensiveness regarding the three facets, with the extremes of JDT (all facets are present) and OOO (all facets are missing).
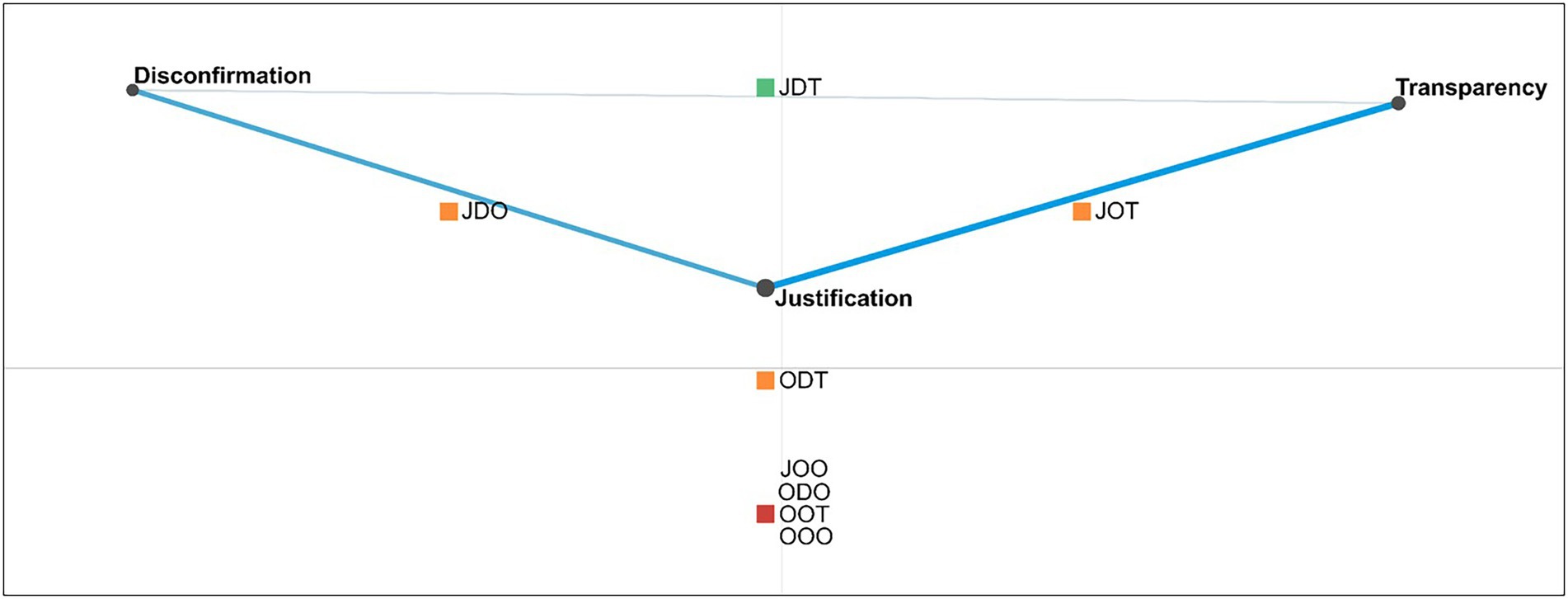
Figure 3. Argumentation types (indicated by colored squares) plotted in a two-dimensional space to indicate the relationship between the argumentation types and the individual facets (indicated by gray nodes) and the co-occurrences of the facets (indicated by blue lines). argumentation types are characterized by: J, Justification; D, Disconfirmation; T, Transparency; O, Absence of a Facet. For example: JOT, Justification and transparency without disconfirmation.
Overall, the findings indicate that preservice teachers tend to primarily provide justification in their diagnostic argumentation as an antecedent to including disconfirmation, transparency, or both. Moreover, the results suggest that there may be a relationship of unidirectional dependency of disconfirmation on justification.
RQ1c: Relations of justification, disconfirmation, and transparency
Beyond exploring the three facets in the individual argumentation texts, we also analyzed the descriptive statistics and correlations of preservice teachers’ justification, disconfirmation, and transparency across the cases. The descriptive results of the facets’ total scores (see Table 3) were consistent with the pattern found in the individual argumentation texts (see Table 1). Participants mostly focused on justification (M = 3.83, SD = 1.58), rarely used disconfirmation (M = 1.53, SD = 1.41), and put a medium emphasis on transparency (M = 2.67, SD = 1.81). The correlational analysis (see Table 3) indicated that justification and disconfirmation were significantly correlated, with a large effect (r = 0.568, p < 0.001). By contrast, transparency was not significantly correlated with justification (r = 0. 055, p = 0.554) or disconfirmation (r = 0.025, p = 0.787). Considering the unidirectional dependency of disconfirmation on justification (see the results of RQ1b), we interpreted the overall result pattern as suggesting that justification, disconfirmation, and transparency are distinct facets of diagnostic argumentation rather than indicators of a uniform skill.
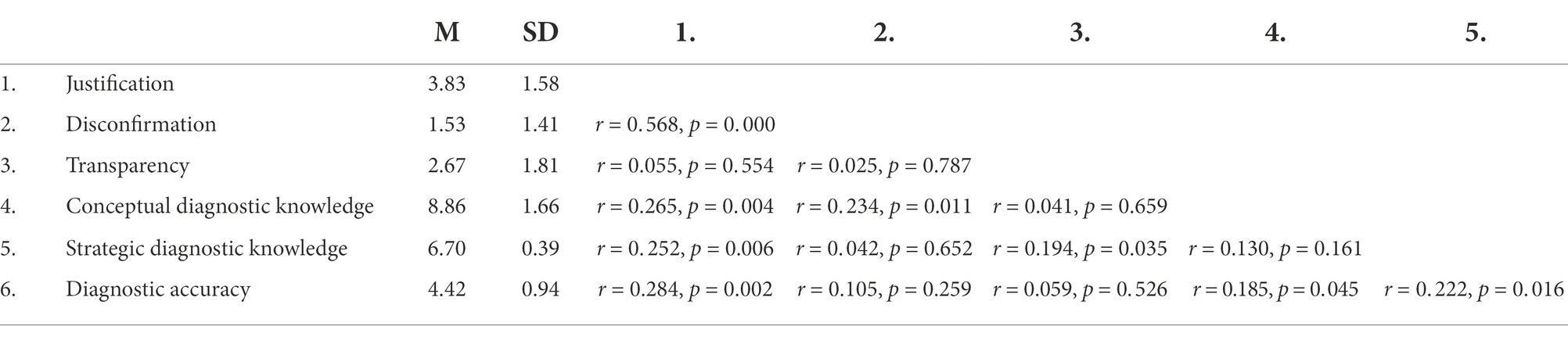
Table 3. Descriptive results and Pearson correlations of preservice teachers’ scores for the three argumentation facets justification, disconfirmation, and transparency, as well as conceptual and strategic diagnostic knowledge and diagnostic accuracy.
RQ2: Relations of conceptual and strategic diagnostic knowledge with justification, disconfirmation, and transparency
To explore the extent to which CDK and SDK predicted the dependent variables of justification, disconfirmation, and transparency, we calculated a multivariate multiple linear regression. Participants achieved M = 8.86 points (SD = 1.66) out of a maximum of 14 points on the CDK test and M = 6.70 points (SD = 0.39) out of a maximum of eight points on the SDK test (see Table 3). The Pearson correlations of the three argumentation facets with the variables CDK and SDK are reported in Table 3. The overall regression model with the predictors CDK and SDK significantly predicted justification—F(2, 115) = 7.725, p = 0.001—and explained 11.8% of the variance. Both CDK (β = 0.236, p = 0.009) and SDK (β = 0.222, p = 0.013) contributed significantly to the model. Similarly, disconfirmation was significantly predicted by the overall regression model, with the predictors CDK and SDK—F(2, 115) = 3.331, p = 0.039—explaining 5.5% of the variance. Whereas CDK (β = 0.232, p = 0.012) contributed significantly to the model, SDK did not (β = 0.012, p = 0.898). By contrast, transparency was not significantly predicted by the overall regression model, including both predictors, CDK and SDK—F(2, 115) = 2.264, p = 0.109—which explained 3.8% of the variance. CDK (β = 0.016, p = 0.861) was not a significant predictor of transparency; however, SDK (β = 0.192, p = 0.040) was a significant predictor of transparency in diagnostic argumentation.
Overall, justification, disconfirmation, and transparency were each partially explained by CDK, SDK, or both, with small effect sizes. Across the three facets, there were considerable differences in the amounts of variance explained by CDK and SDK. Moreover, the pattern in which CDK and SDK predicted justification, disconfirmation, and transparency differed considerably.
RQ3: Relationship between diagnostic judgment and diagnostic argumentation
To explore whether diagnostic judgment and diagnostic argumentation represent different diagnostic skills, we started again by plotting argumentation texts in ENA. First, we grouped argumentation texts according to diagnostic accuracy to compare argumentation concerning inaccurate versus accurate judgments. Second, we explored preservice teachers’ total scores to investigate whether diagnostic accuracy correlated with justification, disconfirmation, and transparency in diagnostic argumentation.
To explore whether the argumentation texts differed if concerning an accurate vs. an inaccurate judgment, we grouped the individual argumentation texts by diagnostic accuracy and created one overall ENA network per group. We descriptively compared the networks of the groups of argumentation texts concerning accurate judgments (see Figure 4A) and inaccurate judgments (see Figure 4C), which we found to be highly similar (see also the comparison plot in Figure 4B, which shows the other two networks’ differences). To determine whether the two groups of argumentation texts differed significantly, we centered the networks, resulting in the two group means (indicated by colored squares, with confidence intervals indicated by colored dashed boxes) depicted in Figure 4B.
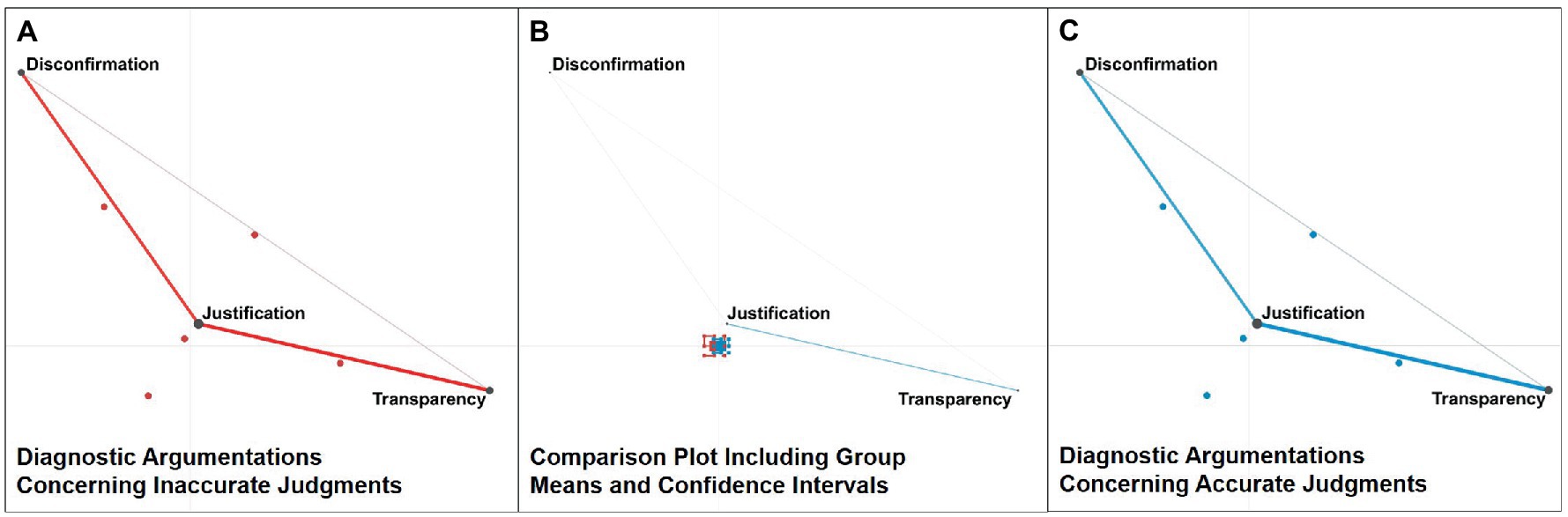
Figure 4. Networks across diagnostic argumentation texts, grouped by diagnostic accuracy and rotated by group means: (A) shows the network of diagnostic argumentation texts concerning inaccurate judgments. (C) shows the network of diagnostic argumentation texts concerning accurate judgments. (B) shows the comparison plot, which depicts the differences between the other two networks, as well as the group means (indicated by colored squares) and confidence intervals (indicated by colored dashed boxes) of the other two networks.
All networks in Figure 4 were rotated to align both group means to the X-axis, which enabled statistical testing of group differences in a single dimension (Shaffer, 2017). The positioning of the group mean of argumentation texts concerning inaccurate judgments (M = −0.01, SD = 0.38, n = 100) was not statistically significantly different from the positioning of the group mean of argumentation texts concerning accurate judgments (M = 0. 01, SD = 0.41, n = 457; t(153.53) = 0.56, p = 0.58, Cohen’s d = 0.06). The analysis suggests that, overall, argumentation texts did not differ if addressing an accurate versus an inaccurate judgment.
We proceeded with a correlational analysis of preservice teachers’ total scores to investigate whether their overall diagnostic accuracy was correlated with justification, disconfirmation, and transparency (see Table 3). On average, participants achieved a diagnostic accuracy of M = 4.42 points (SD = 0.94) out of a maximum of six achievable points. We found that participants’ diagnostic accuracy and justification were significantly correlated, with a small effect (r = 0.284, p = 0.002). By contrast, diagnostic accuracy was not significantly correlated with either disconfirmation (r = 0.105, p = 0. 259) or transparency (r = 0.059, p = 0.526).
To determine the role of CDK and SDK in explaining the relationship between diagnostic accuracy and justification, we calculated a partial correlation between diagnostic accuracy and justification, statistically controlling for CDK and SDK (see Table 3 for the Pearson correlations of CDK and SDK with the argumentation facets and diagnostic accuracy). We found that the resulting partial correlation between diagnostic accuracy and justification in diagnostic argumentation remained significant, with a small effect (r = 0.211, p = 0.023). Thus, controlling for CDK and SDK hardly decreased the effect size of the correlation between diagnostic accuracy and justification. Consequently, our results suggest that CDK and SDK are not the variables that primarily explain the relationship between diagnostic accuracy and justification.
Overall, the results only indicate a weak relationship between the accuracy of preservice teachers’ diagnostic judgments on the one hand, and justification, disconfirmation, and transparency in their diagnostic argumentation on the other. CDK and SDK did not explain the small correlation between diagnostic accuracy and justification. Moreover, groups of argumentation texts concerning inaccurate versus accurate judgments did not show a statistically significant difference. These findings suggest that diagnostic judgment and diagnostic argumentation can be considered different diagnostic skills.
Discussion
In exploring whether justification, disconfirmation, and transparency represent distinct subskills or one joint underlying diagnostic skill (RQ1), we found that preservice teachers primarily provide justification in their diagnostic argumentation as an antecedent to including disconfirmation or transparency in their diagnostic argumentation. Furthermore, we found a unidirectional dependency of disconfirmation on justification; diagnostic argumentation texts presenting more than one diagnosis usually discussed the differential diagnoses by evaluating evidence to make conclusions; however, preservice teachers often only argued for their final diagnosis without discussing competing explanations. Concerning the interrelations between justification, disconfirmation, and transparency, we found that they were distinguishable facets of diagnostic argumentation. Determining the extent to which justification, disconfirmation, and transparency were explained by CDK and SDK (RQ2), we found that justification was predicted by CDK about diagnoses and evidence as well as SDK about diagnostic approaches and activities. Disconfirmation of different diagnoses was only predicted by CDK of diagnoses. By contrast, transparency about the diagnostic approaches for generating evidence was only predicted by SDK of diagnostic proceedings for generating evidence. However, the variance explained by CDK and SDK was low. Furthermore, the accuracy of diagnostic judgments and justification, disconfirmation, and transparency in diagnostic argumentation did not necessarily seem to be related (RQ3). Overall, groups of argumentation texts addressing either accurate or inaccurate diagnostic judgments did not show a statistically significant difference. However, in contrast to disconfirmation and transparency, we found that justification in diagnostic argumentation was significantly correlated with the accuracy of diagnostic judgments. Despite statistically controlling for CDK and SDK, the relationship between the accuracy of diagnostic judgments and justification in diagnostic argumentation remained significant, suggesting that other variables may be important in explaining the relationship.
Overall, we interpreted the results as suggesting that diagnostic judgment and diagnostic argumentation might be different diagnostic skills. Finding a relationship between the accuracy of diagnostic judgments and justification in diagnostic argumentation supports the relevance and validity of the construct of diagnostic argumentation. Yet, the argumentation facets seemed to be sufficiently distinguishable from one another and from diagnostic accuracy. Finding differences regarding the predictive patterns of CDK and SDK (see Förtsch et al., 2018) supports the notion that justification, disconfirmation, and transparency are distinct subskills of diagnostic argumentation. Justification involves explicitly evaluating evidence as the basis for concluding a diagnosis (see Fischer et al., 2014; Heitzmann et al., 2019). Therefore, justification requires CDK about relevant concepts (e.g., diagnoses, evidence, and their interrelations; see Förtsch et al., 2018). Moreover, justification requires making warranted connections between evidence and diagnoses (e.g., Toulmin, 1958) to conclude or reject diagnoses, which seems to be facilitated by SDK (see Förtsch et al., 2018). Disconfirmation involves addressing differential diagnoses to demonstrate that alternative explanations have been considered (e.g., Toulmin, 1958; Lawson, 2003), which seems to primarily require CDK about differential diagnoses. By contrast, transparency, which involves describing the processes behind evidence generation (see Chinn et al., 2014; Vazire, 2017), seems to rely on SDK when it comes to the process of diagnosing a specific problem (e.g., which informational sources can deliver critical evidence).
Large amounts of variance in justification, disconfirmation, and transparency remained unexplained by CDK and SDK. Our findings raise the question of which additional kinds of knowledge and skills may be used when formulating justified, disconfirming, and transparent diagnostic argumentation. Beyond CDK and SDK, we propose two additional variables that might play a role in explaining justification, disconfirmation, and transparency within diagnostic argumentation: (1) knowledge about standards in diagnosing and diagnostic argumentation (see Chinn et al., 2014; Bauer et al., 2020) and (2) argumentation skills that are transferrable across domains (Hetmanek et al., 2018). In teacher education, there seems to be limited agreement about standards in diagnostic practices compared with other fields, such as medical education (Bauer et al., 2020). Teacher education programs do not yet systematically teach agreed-upon standards for communicating in situations that require what we defined as diagnostic argumentation. Consequently, preservice teachers likely do not have much knowledge about standards in diagnostic argumentation. There might also be differences between teacher and medical education in what are considered suitable standards for diagnostic argumentation (Bauer et al., 2020). Moreover, teachers and teacher educators might vary in their views regarding the role of scientific standards in diagnostic argumentation. Therefore, it is important to continue to discuss such standards in teacher education. We suggest using justification, disconfirmation, and transparency as a starting point from which to further discuss, systematize, and teach standards for diagnostic argumentation in teacher education.
The performance differences and higher prevalence of justification observed in the current study may be explained by argumentation skills that are transferrable across domains. It has been suggested that cross-domain transferable skills can, to some extent, compensate for a lack of more specifically relevant knowledge (e.g., knowledge about standards in diagnostic argumentation; Hetmanek et al., 2018). Accordingly, knowledge about standards in diagnostic argumentation, as well as cross-domain transferable argumentation skills, may be relevant for explaining justification, disconfirmation, and transparency in preservice teachers’ diagnostic argumentation beyond their CDK and SDK. Other possible sources of variance are additional kinds of knowledge used in diagnosing that were not considered in this study, such as scientific knowledge that is not pertinent to the context (e.g., Hetmanek et al., 2015) or subjective theories, beliefs, and epistemic goals (Stark, 2017).
CDK and SDK also did not explain the relationship found between the accuracy of diagnostic judgments and justification in diagnostic argumentation. Beyond a joint knowledge base, another variable that could potentially explain the relationship between accuracy and justification may be the different types of information processing that occur during the judgment process (see Loibl et al., 2020). The literature on dual-process theories (see Kahneman, 2003; Evans, 2008) suggests that controlled information processing results in more conscious and explicable reasoning compared to intuitive information processing (e.g., pattern recognition; see Evans, 2008). Thus, a controlled analysis of evidence during the judgment process could affect the accuracy of diagnostic judgments (see Coderre et al., 2010; Norman et al., 2017) and at the same time facilitate justification in diagnostic argumentation.
Limitations and future research
One methodological limitation that needs to be discussed is the low internal consistency of diagnostic accuracy across diagnostic judgments, which may hide further correlations that were not observed in the results. Low internal consistency values are a common issue in measurement instruments with small numbers of items (e.g., Monteiro et al., 2020). However, we did not assume that low internal consistency was a major issue for our interpretations because we still found the theoretically expected relations of diagnostic accuracy with the variables CDK and SDK.
The operationalization of the judgment process in the simulation-based learning environment might be considered to limit generalizability to real-life practice situations, in which teachers’ judgment processes might take place over several days or weeks and involve higher degrees of complexity and ambiguity compared to our simulated cases. However, in our simulation, preservice teachers could decide by themselves how much evidence they wanted to collect, and in which order they would access which informational sources (e.g., conversation protocols). Therefore, we argue that, for the purpose of our research goals, the simulation provided a sufficient representation of a real-world diagnostic situation.
Descriptive results of the participants’ performance in all three argumentation facets across the measurement points of the different cases suggest that participants’ performance generally decreased throughout the data collection. The long duration of the study might have exhausted the participants or decreased their motivation. In addition, some participants might have concluded from the order of the tasks in the simulated cases that they would not need to include their initially indicated diagnostic judgments as a conclusion in their subsequently written diagnostic argumentation texts. Given that the operationalization of justification required participants not only to evaluate evidence but also to explicate conclusions in their argumentation texts, their argumentation skills in terms of justification might have been underestimated in our study. Therefore, generalizing to teachers in authentic classroom situations based on our participants’ performance should be done with caution.
There are areas other than students’ clinical problems in which teachers’ diagnosing is relevant (e.g., assessing a student’s level of skill). Our choice of topic might limit the generalizability of the findings to other areas of diagnosing in teacher education. However, we consider the conceptualization of diagnostic argumentation (i.e., justification, disconfirmation, and transparency) presented in this article nonspecific to the content area of clinical problems. Thus, we expect the result pattern to be replicable in other areas of teachers’ diagnosing, which could be investigated in further research.
To explore the research questions addressed in this paper, we reanalyzed the data collected in a prior cross-sectional study. The sample was too small to employ structural equation modeling, which would have been preferable to analyzing the data with correlation and regression analyses. Although our results provide initial evidence of the potential relationships between the investigated constructs, they must be replicated in future research using larger samples and advanced methods.
Future research is necessary to further validate the findings that diagnostic argumentation is a diagnostic skill that is distinct from diagnostic judgment. For this purpose, we recommend the approach to investigate preservice teachers’ performance based on both qualitative and quantitative data as illustrated in our study. In particular, possible joint predictors of accurate diagnostic judgments and justified diagnostic argumentation, such as controlled information processing during the judgment process, require further clarification because CDK and SDK did not seem to explain the relation between accuracy and justification. Additionally, further research in teacher education should investigate the knowledge and skills that underlie justification, disconfirmation, and transparency beyond CDK and SDK, such as knowledge about standards in diagnosing, cross-domain transferrable argumentation skills, as well as subjective theories, beliefs, and experiential knowledge regarding evidence-oriented practice.
In our study, we did not specify a particular recipient to whom preservice teachers should direct their diagnostic argumentation. However, diagnostic argumentation might vary considerably depending on the recipient (e.g., a teacher colleague, a school psychologist, or a parent) and the argumentative situation (during a collaborative judgment process or subsequent to making a judgment). For example, prior research in collaborative diagnosing has emphasized the potential role of meta-knowledge about the collaborating professional’s role and responsibilities (Radkowitsch et al., 2021). Therefore, future studies might systematically investigate the role of different recipients in teachers’ diagnostic argumentation.
Research may also validate whether professionals in teacher education perceive justification, disconfirmation, and transparency as facilitating comprehensibility and persuasiveness in diagnostic argumentation or whether our suggested conception of argumentation facets needs to be further specified for the area of teacher education. One interesting and potentially relevant direction in which to further develop our conception might be found in the literature on professional vision, which distinguishes between describing and interpreting evidence as two different forms of how evidence is reported and evaluated in the context of teachers’ diagnosing (Seidel and Stürmer, 2014; Kramer et al., 2021b). Moreover, researchers could explore the potential of different learning opportunities and support measures for fostering preservice teachers’ diagnostic argumentation. Similarly, researchers could investigate whether diagnostic judgment and diagnostic argumentation have similar or different developmental trajectories and might benefit from similar or different forms of instruction.
Conclusion
In this article, we presented evidence suggesting that diagnostic judgment and diagnostic argumentation might represent different diagnostic skills. Preservice teachers do not necessarily seem to be equally capable of making accurate diagnostic judgments on the one hand, and formulating justified, disconfirming, and transparent diagnostic argumentation on the other. We suggest that justification, disconfirmation, and transparency can be considered relevant facets and distinct subskills of diagnostic argumentation, as our results appear to indicate differences in the underlying knowledge bases. Despite the fact that CDK and SDK explain some variance in justification, disconfirmation, and transparency, the portion of variance they explain might be rather small. Thus, additional variables may be relevant predictors of justification, disconfirmation, and transparency in diagnostic argumentation, such as knowledge of diagnostic standards or cross-domain transferable argumentation skills. Including these additional constructs in further investigations would be a promising direction for future research on diagnostic argumentation. In addition, it seems particularly important that researchers and educators in the field of teacher education, as well as in-service teachers as practitioners in the field, further reflect on standards in diagnosing and diagnostic argumentation. Justification, disconfirmation, and transparency may serve as a productive set of constructs for establishing standards for teachers’ diagnostic argumentation in the future.
Data availability statement
The data presented in this article will be made available by the authors upon request. Requests to access the data should be directed to elisabeth.bauer@psy.lmu.de.
Ethics statement
The studies involving human participants were reviewed and approved by the Ethics Committee of the Medical Faculty of LMU Munich (no. 17-249). The participants provided their written informed consent to participate in this study.
Author contributions
EB, MS, JK, MF, and FF developed the study concept and contributed to the study design. EB performed the data analysis. EB, MS, and FF interpreted the data. EB drafted the manuscript. MS, JK, MF, and FF provided critical revisions. All authors contributed to the article and approved the submitted version.
Funding
This research was funded by the German Federal Ministry of Research and Education (FAMULUS-Project 16DHL1040) and the Elite Network of Bavaria (K-GS-2012-209).
Conflict of interest
The authors declare that the research was conducted in the absence of any commercial or financial relationships that could be construed as a potential conflict of interest.
Publisher’s note
All claims expressed in this article are solely those of the authors and do not necessarily represent those of their affiliated organizations, or those of the publisher, the editors and the reviewers. Any product that may be evaluated in this article, or claim that may be made by its manufacturer, is not guaranteed or endorsed by the publisher.
Footnotes
References
Albritton, K., Chen, C.-I., Bauer, S. G., Johnson, A., and Mathews, R. E. (2021). Collaborating with school psychologists: moving beyond traditional assessment practices. Young Except. Children 24, 28–38. doi: 10.1177/1096250619871951
Bauer, E., Fischer, F., Kiesewetter, J., Shaffer, D. W., Fischer, M. R., Zottmann, J. M., et al. (2020). Diagnostic activities and diagnostic practices in medical education and teacher education: an interdisciplinary comparison. Front. Psychol. 11, 1–9. doi: 10.3389/fpsyg.2020.562665
Berland, L. K., and Reiser, B. J. (2009). Making sense of argumentation and explanation. Sci. Educ. 93, 26–55. doi: 10.1002/sce.20286
Boshuizen, H. P., Gruber, H., and Strasser, J. (2020). Knowledge restructuring through case processing: the key to generalise expertise development theory across domains? Educ. Res. Rev. 29:100310. doi: 10.1016/j.edurev.2020.100310
Chernikova, O., Heitzmann, N., Fink, M. C., Timothy, V., Seidel, T., and Fischer, F. (2020). Facilitating diagnostic competences in higher education - a meta-analysis in medical and teacher education. Educ. Psychol. Rev. 32, 157–196. doi: 10.1007/s10648-019-09492-2
Chinn, C. A., and Duncan, R. G. (2018). “What is the value of general knowledge of scientific reasoning? Scientific reasoning and argumentation: the roles of domain-specific and domain-general knowledge,” in Scientific reasoning and argumentation. eds. F. Fischer, A. C. Clark, K. Engelmann, and J. Osborne (New York: Routledge), 77–101.
Chinn, C. A., Rinehart, R. W., and Buckland, L. A. (2014). “Epistemic cognition and evaluating information: applying the AIR model of epistemic cognition,” in Processing inaccurate information: Theoretical and applied perspectives from cognitive science and the educational sciences. eds. D. Rapp and J. Braasch (Cambridge, MA: MIT Press), 425–453.
Coderre, S., Wright, B., and McLaughlin, K. (2010). To think is good: querying an initial hypothesis reduces diagnostic error in medical students. Acad. Med. 85, 1125–1129. doi: 10.1097/ACM.0b013e3181e1b229
Codreanu, E., Sommerhoff, D., Huber, S., Ufer, S., and Seidel, T. (2021). Exploring the process of preservice teachers’ diagnostic activities in a video-based simulation. Front. Educ. 6:626666. doi: 10.3389/feduc.2021.626666
Cohen, J. (1960). A coefficient of agreement for nominal scales. Educ. Psychol. Meas. 20, 37–46. doi: 10.1177/001316446002000104
Csanadi, A., Kollar, I., and Fischer, F. (2020). Pre-service teachers’ evidence-based reasoning during pedagogical problem-solving: better together? Eur. J. Psychol. Educ. 36, 147–168. doi: 10.1007/s10212-020-00467-4
Diamantopoulos, A., and Siguaw, J. A. (2006). Formative versus reflective indicators in organizational measure development: a comparison and empirical illustration. Br. J. Manag. 17, 263–282. doi: 10.1111/j.1467-8551.2006.00500.x
Evans, J. S. B. T. (2008). Dual-processing accounts of reasoning, judgment, and social cognition. Annu. Rev. Psychol. 59, 255–278. doi: 10.1146/annurev.psych.59.103006.093629
Feldon, D. F. (2007). Cognitive load and classroom teaching: the double-edged sword of automaticity. Educ. Psychol. 42, 123–137. doi: 10.1080/00461520701416173
Fischer, F. (2021). Some reasons why evidence from educational research is not particularly popular among (pre-service) teachers: a discussion. Zeitschrift für Pädagogische Psychologie 35, 209–214. doi: 10.1024/1010-0652/a000311
Fischer, F., Kollar, I., Ufer, S., Sodian, B., Hussmann, H., Pekrun, R., et al. (2014). Scientific reasoning and argumentation: advancing an interdisciplinary research agenda in education. Frontline Learn. Res. 2, 28–45. doi: 10.14786/flr.v2i2.96
Fleiss, J. L. (1971). Measuring nominal scale agreement among many raters. Psychol. Bull. 76:378, –382. doi: 10.1037/h0031619
Förtsch, C., Sommerhoff, D., Fischer, F., Fischer, M. R., Girwidz, R., Obersteiner, A., et al. (2018). Systematizing professional knowledge of medical doctors and teachers: development of an interdisciplinary framework in the context of diagnostic competences. Educ. Sci. 8:207. doi: 10.3390/educsci8040207
Gorman, M. E., Gorman, M. E., Latta, R. M., and Cunningham, G. (1984). How disconfirmatory, confirmatory and combined strategies affect group problem solving. Br. J. Psychol. 75, 65–79. doi: 10.1111/j.2044-8295.1984.tb02790.x
Grossman, P. (2021). Teaching core practices in teacher education, Cambridge: Harvard Education Press.
Grossman, P., Compton, C., Igra, D., Ronfeldt, M., Shahan, E., and Williamson, P. (2009). Teaching practice: a cross-professional perspective. Teach. Coll. Rec. 111, 2055–2100. doi: 10.1177/016146810911100905
Heitzmann, N., Seidel, T., Opitz, A., Hetmanek, A., Wecker, C., Fischer, M., et al. (2019). Facilitating diagnostic competences in simulations: a conceptual framework and a research agenda for medical and teacher education. Frontline Learn. Res. 7, 1–24. doi: 10.14786/flr.v7i4.384
Herppich, S., Praetorius, A.-K., Förster, N., Glogger-Frey, I., Karst, K., Leutner, D., et al. (2018). Teachers' assessment competence: integrating knowledge-, process-, and product-oriented approaches into a competence-oriented conceptual model. Teach. Teach. Educ. 76, 181–193. doi: 10.1016/j.tate.2017.12.001
Hetmanek, A., Engelmann, K., Opitz, A., and Fischer, F. (2018). “Beyond intelligence and domain knowledge: scientific reasoning and argumentation as a set of cross-domain skills. Scientific reasoning and argumentation: the roles of domain-specific and domain-general knowledge,” in Scientific reasoning and argumentation. eds. F. Fischer, A. C. Clark, K. Engelmann, and J. Osborne (New York: Routledge), 203–226. doi: 10.4324/9780203731826
Hetmanek, A., Wecker, C., Kiesewetter, J., Trempler, K., Fischer, M. R., Gräsel, C., et al. (2015). Wozu nutzen Lehrkräfte welche Ressourcen? Eine Interviewstudie zur Schnittstelle zwischen bildungswissenschaftlicher Forschung und professionellem Handeln im Bildungsbereich [for which purposes do teachers use which resources? An interview study on the relation between educational research and professional educational practice]. Unterrichtswissenschaft 43, 194–210.
Hitchcock, D. (2005). Good reasoning on the Toulmin model. Argumentation 19, 373–391. doi: 10.1007/s10503-005-4422-y
Kahneman, D. (2003). A perspective on judgment and choice: mapping bounded rationality. Am. Psychol. 58, 697–720. doi: 10.1037/0003-066X.58.9.697
Kiemer, K., and Kollar, I. (2021). Source selection and source use as a basis for evidence-informed teaching. Zeitschrift für Pädagogische Psychologie 35, 127–141. doi: 10.1024/1010-0652/a000302
Kiesewetter, J., Fischer, F., and Fischer, M. R. (2017). Collaborative clinical reasoning—a systematic review of empirical studies. J. Contin. Educ. Health Prof. 37, 123–128. doi: 10.1097/CEH.0000000000000158
Klahr, D., and Dunbar, K. (1988). Dual space search during scientific reasoning. Cognit. Sci. 12, 1–48. doi: 10.1207/s15516709cog1201_1
Kolovou, D., Naumann, A., Hochweber, J., and Praetorius, A.-K. (2021). Content-specificity of teachers’ judgment accuracy regarding students’ academic achievement. Teach. Teach. Educ. 100:103298. doi: 10.1016/j.tate.2021.103298
Kramer, M., Förtsch, C., Boone, W. J., Seidel, T., and Neuhaus, B. J. (2021a). Investigating pre-service biology teachers’ diagnostic competences: relationships between professional knowledge, diagnostic activities, and diagnostic accuracy. Educ. Sci. 11:89. doi: 10.3390/educsci11030089
Kramer, M., Förtsch, C., Seidel, T., and Neuhaus, B. J. (2021b). Comparing two constructs for describing and analyzing teachers’ diagnostic processes. Stud. Educ. Eval. 68:100973. doi: 10.1016/j.stueduc.2020.100973
Landis, J. R., and Koch, G. G. (1977). The measurement of observer agreement for categorical data. Biometrics 33, 159–174. doi: 10.2307/2529310
Lawson, A. (2003). The nature and development of hypothetico-predictive argumentation with implications for science teaching. Int. J. Sci. Educ. 25, 1387–1408. doi: 10.1080/0950069032000052117
Loibl, K., Leuders, T., and Dörfler, T. (2020). A framework for explaining teachers’ diagnostic judgements by cognitive modeling (DiaCoM). Teach. Teach. Educ. 91:103059. doi: 10.1016/j.tate.2020.103059
Mercier, H., and Heintz, C. (2014). Scientists’ argumentative reasoning. Topoi 33, 513–524. doi: 10.1007/s11245-013-9217-4
Mercier, H., and Sperber, D. (2017). The enigma of reason. Cambridge, Massachusetts: Harvard University Press.
Monteiro, S. D., Sherbino, J., Schmidt, H., Mamede, S., Ilgen, J., and Norman, G. (2020). It’s the destination: diagnostic accuracy and reasoning. Adv. Health Sci. Educ. 25, 19–29. doi: 10.1007/s10459-019-09903-7
Norman, G. R., Monteiro, S. D., Sherbino, J., Ilgen, J. S., Schmidt, H. G., and Mamede, S. (2017). The causes of errors in clinical reasoning: cognitive biases, knowledge deficits, and dual process thinking. Acad. Med. 92, 23–30. doi: 10.1097/ACM.0000000000001421
Page, G., Bordage, G., and Allen, T. (1995). Developing key-feature problems and examinations to assess clinical decision-making skills. Acad. Med. 70, 194–201. doi: 10.1097/00001888-199503000-00009
Pfeiffer, J., Meyer, C. M., Schulz, C., Kiesewetter, J., Zottmann, J., Sailer, M., et al. (2019). “FAMULUS: Interactive Annotation and Feedback Generation for Teaching Diagnostic Reasoning,” in Proceedings of the 2019 Conference on Empirical Methods in Natural Language Processing and the 9th International Joint Conference on Natural Language Processing (EMNLP-IJCNLP): System Demonstrations,. eds. S. Padó and R. Huang Stroudsburg, PA, USA: Association for Computational Linguistics., 73–78
Pit-ten Cate, I. M., Hörstermann, T., Krolak-Schwerdt, S., Gräsel, C., Böhmer, I., and Glock, S. (2020). Teachers’ information processing and judgement accuracy: effects of information consistency and accountability. Eur. J. Psychol. Educ. 35, 675–702. doi: 10.1007/s10212-019-00436-6
Poznanski, B., Hart, K. C., and Graziano, P. A. (2021). What do preschool teachers know about attention-deficit/hyperactivity disorder (ADHD) and does it impact ratings of child impairment? Sch. Ment. Heal. 13, 114–128. doi: 10.1007/s12310-020-09395-6
Praetorius, A. K., Berner, V. D., Zeinz, H., Scheunpflug, A., and Dresel, M. (2013). Judgment confidence and judgment accuracy of teachers in judging self-concepts of students. J. Educ. Res. 106, 64–76. doi: 10.1080/00220671.2012.667010
Radkowitsch, A., Sailer, M., Schmidmaier, R., Fischer, M. R., and Fischer, F. (2021). Learning to diagnose collaboratively–effects of adaptive collaboration scripts in agent-based medical simulations. Learn. Instr. 75:101487. doi: 10.1016/j.learninstruc.2021.101487
Rieu, A., Leuders, T., and Loibl, K. (2022). Teachers’ diagnostic judgments on tasks as information processing–the role of pedagogical content knowledge for task diagnosis. Teach. Teach. Educ. 111:103621. doi: 10.1016/j.tate.2021.103621
Sailer, M., Bauer, E., Hofmann, R., Kiesewetter, J., Glas, J., Gurevych, I., et al. (2022). Adaptive feedback from artificial neural networks facilitates pre-service teachers’ diagnostic reasoning in simulation-based learning. Learn. Instr. 101620, 1–10. doi: 10.1016/j.learninstruc.2022.101620
Sampson, V., and Clark, D. B. (2008). Assessment of the ways students generate arguments in science education: current perspectives and recommendations for future directions. Sci. Educ. 92, 447–472. doi: 10.1002/sce.20276
Schmidmaier, R., Eiber, S., Ebersbach, R., Schiller, M., Hege, I., Holzer, M., et al. (2013). Learning the facts in medical school is not enough: which factors predict successful application of procedural knowledge in a laboratory setting? BMC Med. Educ. 13, 1–9. doi: 10.1186/1472-6920-13-28
Seidel, T., and Stürmer, K. (2014). Modeling and measuring the structure of professional vision in preservice teachers. Am. Educ. Res. J. 51, 739–771. doi: 10.3102/0002831214531321
Shulman, L. S. (1986). Those who understand: knowledge growth in teaching. Educ. Res. 15, 4–14. doi: 10.3102/0013189X015002004
Stadler, M., Sailer, M., and Fischer, F. (2021). Knowledge as a formative construct: a good alpha is not always better. New Ideas Psychol. 60:100832. doi: 10.1016/j.newideapsych.2020.100832
Stark, R. (2017). Probleme evidenzbasierter bzw. -orientierter pädagogischer praxis [problems of evidence-based or rather evidence-oriented educational practice]. Zeitschrift für Pädagogische Psychologie 31, 99–110. doi: 10.1024/1010-0652/a000201
Stark, R., Kopp, V., and Fischer, M. R. (2011). Case-based learning with worked examples in complex domains: two experimental studies in undergraduate medical education. Learn. Instr. 21, 22–33. doi: 10.1016/j.learninstruc.2009.10.001
Südkamp, A., Praetorius, A.-K., and Spinath, B. (2018). Teachers’ judgment accuracy concerning consistent and inconsistent student profiles. Teach. Teach. Educ. 76, 204–213. doi: 10.1016/j.tate.2017.09.016
Taber, K. S. (2018). The use of Cronbach’s alpha when developing and reporting research instruments in science education. Res. Sci. Educ. 48, 1273–1296. doi: 10.1007/s11165-016-9602-2
Urhahne, D., and Wijnia, L. (2020). A review on the accuracy of teacher judgments. Educ. Res. Rev. 32:100374. doi: 10.1016/j.edurev.2020.100374
Van Gog, T., Paas, F., and Van Merriënboer, J. J. (2004). Process-oriented worked examples: improving transfer performance through enhanced understanding. Instr. Sci. 32, 83–98. doi: 10.1023/B:TRUC.0000021810.70784.b0
Vanlommel, K., Van Gasse, R., Vanhoof, J., and Van Petegem, P. (2017). Teachers’ decision-making: data based or intuition driven? Int. J. Educ. Res. 83, 75–83. doi: 10.1016/j.ijer.2017.02.013
Vazire, S. (2017). Quality uncertainty erodes trust in science. Collabra. Psychology 3. doi: 10.1525/collabra.74
Walton, D. N. (1990). What is reasoning? What is an argument? J. Philos. 87, 399–419. doi: 10.2307/2026735
Keywords: teacher education, diagnostic argumentation, scientific argumentation, diagnostic accuracy, diagnostic judgment, diagnostic knowledge
Citation: Bauer E, Sailer M, Kiesewetter J, Fischer MR and Fischer F (2022) Diagnostic argumentation in teacher education: Making the case for justification, disconfirmation, and transparency. Front. Educ. 7:977631. doi: 10.3389/feduc.2022.977631
Edited by:
Robin Stark, Saarland University, GermanyReviewed by:
Timothy Fukawa-Connelly, Temple University, United StatesTom Rosman, Leibniz-Institute for Psychology (ZPID), Germany
Hendrik Lohse-Bossenz, University of Education Heidelberg, Germany
Copyright © 2022 Bauer, Sailer, Kiesewetter, Fischer and Fischer. This is an open-access article distributed under the terms of the Creative Commons Attribution License (CC BY). The use, distribution or reproduction in other forums is permitted, provided the original author(s) and the copyright owner(s) are credited and that the original publication in this journal is cited, in accordance with accepted academic practice. No use, distribution or reproduction is permitted which does not comply with these terms.
*Correspondence: Elisabeth Bauer, elisabeth.bauer@psy.lmu.de