- 1Department of Psychology, Nanjing Normal University, Nanjing, China
- 2Department of Education, Nanjing Normal University, Nanjing, China
The conceptual level is an index of personality development. In the field of teaching, the conceptual level is seen as a dynamic learning style. It has important implications for student learning and individual growth, as well as guidance for teaching. However, the lack of a measurement tool with a clear internal structure for the conceptual level of students has slowed the development of the theory and made it difficult to implement the teaching. To address these issues, this study describes the initial development and validation of the Student Conceptual Level Scale (SCLS) with four samples of students (n = 1,321) drawn from eight secondary schools in China. We constructed a second-order three-factor model of the SCLS consisting of three factors—learning awareness level, autonomous input level, and environmental coping level—each with its own independent set of items. This study validated the use of full-scale and subscale scores and examined their relationship with different validity criteria: autonomous learning, mental effort, and academic scores. This updated measure reflects the value and role of the conceptual level in the learning and individual development of students and also provides a more complete frame of reference for the use of the conceptual level in teaching and learning.
Introduction
The conceptual level (CL) is a personality development index, originally established in the social domain, which refers to an individual’s cognitive complexity in response to evolving and changing information (Harvey et al., 1962; Hunt, 1971). CL has been widely used in the field of teaching and learning to represent the learning style of students, which is an indicator of the maturity of students’ learning and individual development (Hunt, 1977, 1979; Hunt et al., 1978; Miller, 1981). In general, students perform better across a range of cognitive, emotional, and behavioral domains the higher their CL—for example, showing more complex levels of cognition (Harvey et al., 1962; Hunt, 1971), stronger emotion management skills (Doyle and Rutherford, 1984), more positive attitudes toward learning and more congenial interpersonal relationships (Hunt et al., 1974), less problematic behavior (McLachlan, 1974; Brill, 1978), better environmental adaptability (Hunt, 1977), and increased autonomy (Phillips and Sinclair, 1973). Studies related to the relationship between student CL and learning outcomes have shown that learning is better when students’ CL is matched with teacher instruction (Hunt et al., 1974, 1978; Doyle and Rutherford, 1984; Tan, 1995). Matching means that low-CL students are matched with high-structured instruction and high CL students are matched with low-structured instruction. Structure refers to the degree of teacher direction and control over student learning and classroom behavior (Hunt et al., 1974; Miller, 1981).
The concept of CL is derived from conceptual system theory (CST; Harvey et al., 1962; Harold et al., 1967). CST is a theory of personality, and CL is a core element of CST (Hunt, 1975b; Hunt et al., 1978). Influenced by Lewin’s (1936) behavioral theory, CST suggests that behavioral outcomes are a function of the interaction between an individual’s conceptual level and the environmental conditions he or she is exposed to: When the environmental conditions promote the “cognitive activities” necessary to increase the conceptual level, the individual achieves optimal “growth” (Harvey et al., 1962). Because this viewpoint of CST is based on the personality domain, the CL of personality orientation describes changes in personality development only through the lens of cognitive dispositions and does not focus on behavioral characteristics (Harvey et al., 1962; Miller, 1981). When Hunt extended the ideas of CST to the field of teaching and learning (Hunt, 1970), the CL of learning style orientation described changes in student learning and individual development beyond learning in terms of both cognitive dispositions and behavioral characteristics. CL reflects not only the cognitive complexity of students’ perceptions of learning information and information in general but also cognitive projections to behavioral characteristics such as independence in processing information and flexibility in responding to complex situations (Hunt, 1977; Hunt et al., 1978; Miller, 1981). In fact, regardless of the orientation, cognitive and behavioral characteristics cannot be separated diametrically when discussing individual characteristics. Hunt (1975b, 1977) described CL in terms of cognitive complexity, independence, and environmental adaptability, which relate to both cognitive dispositions and behavioral characteristics. Cognitive complexity refers to the ability to process information and reflects the extent to which students’ cognition develops on the concrete–abstract dimension; independency refers to the ability to learn independently and reflects the extent to which students develop on the dependence–independence dimension; environmental adaptability refers to the adaptation to the learning environment and reflects the extent to which students encounter difficult or contradictory situations and the degree of development on the conflict–balance dimension (Hunt, 1977; Miller, 1981). From these three perspectives, high-CL students are characterized by a high level of cognitive complexity and the ability to solve more abstract problems; the ability to think and learn independently, with less reliance on outside help; and a high level of environmental adaptability, which allows them to better achieve a balance between internal and external environments when they encounter frustration or conflict. Students with low CL are characterized by one-sided cognition, dependence on the outside world, and difficulty balancing their relationship with the outside world; they need more help from the outside world to learn and grow more effectively (Hunt et al., 1978; Miller, 1981). CL theory suggests that instruction should be matched to the student’s CL to facilitate further improvement in learning and CL and to move closer to the developmental goal of “autonomy” (Hunt et al., 1978), which is especially important for students at low conceptual levels (Tomlinson and Hunt, 1971; McLachlan and Hunt, 1973).
CL has potential educational value: (1) It has universal significance for individual student development—it specifies the maturity level of students at this stage of learning and individual development. (2) It provides the basis for the implementation of teaching—teaching based on students’ conceptual level can better facilitate students’ learning and personal development and promote students’ growth while optimizing the teaching and learning process and preventing under-resourcing or waste (Brophy and Good, 1974; Hunt, et al., 1974; Hunt, 1977). Hunt (1975c, 1976b), even found that CL had an impact on teachers’ teaching levels: Students at high conceptual levels had a “student pull” on teachers, which allowed teachers to unconsciously adapt their teaching to students’ needs, and teachers increased their “adaptive level.”
For the measurement of CL, there are currently only three measurement instruments, all of which are projective tests: TIB (This I Believe; Harvey et al., 1962; Greaves, 1971; Stoppard and Miller, 1985), ITI (Interpersonal Topical Inventory; Tuckman, 1965), and, adapted from the PCT (Paragraph Completion Test), PCM (Paragraph Completion Method; Hunt et al., 1978). Among them, the TIB and ITI, both of which are biased by their scale structure and theoretical content, have low correlations, and they are not used to measure conceptual level as a student learning style (Miller, 1978). For example, the TIB is used to measure the teacher’s CL (Murphy and Brown, 1970) or the CL of individuals outside of the teaching context (Goldberg, 1974). The topics, formats, and scoring criteria are the same for both PCM and PCT, except for the scoring range: 1–7 for PCT and 0–3 for PCM (Miller, 1981). The only widely used measurement tool for student CL is the PCM. The PCM is a semi-projective measure that consists of six themes, with each item giving only the first part of the question and requiring the respondent to complete the rest of the question. These themes are designed to understand how students respond to conflict or ambivalent situations (e.g., “criticized,” “unsure,” or “disagree”) and their perceptions of rules and authority complexity (“rules,” “parents,” “being told”). The PCM has a number of limitations: (1) In terms of instrument characteristics, the scoring results of projective tests are difficult to objectify, the administration procedures are complex and inefficient, and they are suitable for case studies (Dai et al., 1999, pp. 241, 242). (2) The PCM was originally used to assess personality, and when used to assess learning styles, it was not possible to demonstrate the generalizability of the instrument across contexts, especially on the “authority, rules” theme (Miller, 1981). (3) The reliability and validity are not satisfactory (Gardiner, and Schroder, 1972; Miller, 1978). (4) Different researchers disagree in the analysis of the data. For example, when grouping scores high and low, some scholars have used the median as a basis for classifying high and low levels of CL, showing that the mean score of the high-CL group was instead lower than the mean score of the low-CL group. Such is a false negative result (Miller, 1981). Other researchers refer to both the range of the score distribution (large enough) and the absolute level (Hunt et al., 1974; McLachlan, 1974). Therefore, as an unstructured measurement tool, it is difficult for the PCM reflect the internal structure of CL. This inconsistency between the theoretical connotation and the measurement tool has created a situation where the conceptual level is difficult to generalize. This is also a great challenge for CL to exert its value. It can be seen that constructing a measurement tool with good measurement properties is the foundation of subsequent CL research.
The present study was based on the theoretical connotations of CL as a student learning style (Hunt et al., 1974, 1978; Hunt, 1975a) to develop a measurement instrument and used secondary school students as the study population. This was for the following reasons. (1) From the perspective of cognitive development theory (Piaget, 1953), secondary school students are in the formal operational stage of cognitive development. This stage reflects the transformation from concrete to abstract thinking: secondary school students process information cognitively in a more profound and abstract way. (2) According to Erikson’s (1959) self-concept theory, secondary school students are in a period of self-identity formation and identity confusion and are acquiring self-identity, during which individuals face more internal confusion and conflict and struggle to find a sense of self-continuity and coherence. During this period, adolescents struggle to construct a balance between the self and the external environment (Nie and Ding, 2009). In this way, the cognitive development of secondary school students is markedly complex and conflictual, and they vary more markedly in three aspects of CL (conceptual complexity, independence, and environmental adaptation; Hunt, 1975a; Hunt et al., 1978). Therefore, the development of a measurement instrument for secondary school students’ CL has both theoretical and practical implications: Theoretically, it helps to clarify the internal structure of students’ CL; practically, it helps to gain insight into students’ cognitive and behavioral development and thus provide targeted educational responses.
Based on the connotation of learning style of CL, this study proposed the concept and dimensions of student CL through qualitative research around the characteristics of three aspects of CL (conceptual complexity, independence, and environmental adaptability), developed the Student Conceptual Level Scale (SCLS), and conducted a large-sample reliability test on the scale to confirm the necessity and value of distinguishing CL into more factors.
Study 1
The aim of Study 1 was to generate a pool of items that comprehensively structure and measure the dimensions of SCLS through qualitative research and to examine the content of the items.
Methods
Participants
Personal interviews and open-ended questionnaires were used for initial project construction. A total of seven secondary school students in three provinces and cities were interviewed personally (five from Shanghai, one from Inner Mongolia, and one from Hunan; aged 12–14 years). An open-ended questionnaire was administered to 32 secondary school students in 3 provinces and cities (10 in Shandong, 20 in Ningxia, and 2 in Hainan; ages 12–15 years, grades 7–9, 32 valid questionnaires). All studies reported in this article were approved by the ethics committee.
The expert panel was recruited to assess the content and face validity of the generated items. The expert panel consisted of 10 experts, including 2 professors of psychology and 8 PhDs in psychology.
The pretest and recheck items were designed to determine the official initial version of the scale for use in the exploratory factor analysis (EFA). The pre-test consisted of 20 secondary school students recruited online (age 12–15 years, grades 7–9, 20 valid questionnaires). Re-checking of the initial project consisted of six experts (two PhDs in Psychology, two graduate students in Chinese, and two secondary school psychology teachers).
Procedure
The qualitative study used a combination of personal interviews and open-ended questionnaires. (a) Based on the results of personal interviews and open-ended questionnaires, the detailed records of interview and open-ended questionnaire results were compiled, their contents were analyzed, and representative terms were extracted for classification and summarization. (b) The themes were further refined and compared and analyzed with literature collation, items with high overlap and relevance were retained, the empirical structure of CL was established, and self-edited question items were generated. (c) By extracting scales related to CL learning connotations, similar meaningful questions were adapted to generate adapted items. (d) After generating an initial pool of questions consisting of both self-edited items and adapted items, the content and face validity of the items were examined by a panel of experts. Ten experts iteratively revised all items, modifying the expression of some entry statements to ensure that there were no biases in the subjects’ understanding of the questions. (e) To ensure that the question items could be accurately understood by the respondents without bias, 20 secondary school students were recruited through the Internet to make small-scale predictions. (f) After the end of the prediction, six experts were asked to revise the content and language of the questionnaire for items with quality issues (e.g., not easily understood, ambiguously expressed, and sensitive) and then separately asked to review the clarity and appropriateness of the revised questions.
Measures
Interview outline: (1) How do you understand the concept of learning? (2) What are your expectations of learning? (3) How do you think about the rules of school? (4) How do you view yourself in relation to others? (5) How do you face the temptations in learning? (6) How do you feel about what others say about you? (7) Which teaching style is conducive to improving your academic performance? (8) Please give a comprehensive evaluation of yourself.
Open-ended questionnaire: (1) What do you consider to be independent, and please summarize it in one or two sentences. (2) How do you understand “independence in learning”? In what aspects of learning are you independent? In what ways are you dependent on others (teachers, parents)? (3) What factors might affect your independence in learning? (4) Which of the following two teaching styles do you think is more helpful to you in your learning: (a) a teaching style in which the teacher talks a lot and strictly manages the classes, or (b) a teaching style in which the teacher talks less and is loosely managed? What do you think are the benefits of the teaching style you have chosen? (5) How would you assess your own performance in learning? If you could change your learning, what would you like to change (e.g., try to control negative emotions, learn more consciously, try to understand different perspectives, be brave enough to ask questions, etc.)? (6) Do you ignore rules (including school rules and regulations, classroom discipline) when they differ from your personal views? Why? (7) How do you think good or bad relationships with others (parents, teachers, classmates) affect your learning? Please give your opinion based on this. (8) Do you study hard? Why? (9) Please combine the above questions and give a comprehensive evaluation of your value, and please talk about your ideas in as much detail as possible.
Analysis and results
Based on the connotation of CL learning style, all contents related to the three aspects of CL (cognitive complexity, independence, and environmental adaptability) were included in the individual interviews to avoid bias caused by the researcher’s subjective screening. In the open-ended questionnaire, the nine questions were organized around six areas: evaluation of one’s own independence; factors influencing learning independence; evaluation of the choice of teaching methods; evaluation of one’s own learning; evaluation of learning environment factors (others, institutions, interpersonal relationships); and learning motivation and self-evaluation. The results of the qualitative analysis showed that the structure of the student CL involves a total of three aspects: learning awareness (cognition), autonomous input (independence), and environmental coping (environmental adaptation). Therefore, this study constructed the initial dimensions of CL from three aspects—the level of learning awareness, the level of autonomous input, and the level of environmental coping—and generated 60 self-edited question items based on these 3 aspects. The adapted items were derived from the Middle School Students’ Self-control Ability Questionnaire (Wang and Lu, 2004), the Personal Goal Orientation Questionnaire (Li and Lin, 2001), the Influences of Middle School Students’ Informal Reasoning Questionnaire (Zhang et al., 2010), and the Adolescent Self-consciousness Scale (Nie and Ding, 2009). Six question items were eventually generated. After 66 initial question items were generated, reviewed, and revised by 10 experts, and after an overall agreement of 90%, the SCLS (initial version) was developed for this study. The SCLS (initial version) resulted in an initial scale of 51 questions corresponding to 3 dimensions: 18 questions for the learning awareness dimension, 14 questions for the autonomous input dimension, and 19 questions for the environmental coping dimension. Twelve of the questions were reverse scored. A six-point Likert scale was used, ranging from “not at all” to “completely.” The total score of the CL is the sum of the scores of each dimension. The higher the total score, the higher the CL.
Study 2
The aims of Study 2 were to explore the factor structure of the SCLS (initial version), including item analysis and EFA, and to assess the factor structure of the SCLS retained from EFA and compared with other models and validity scales, including confirmatory factor analysis (CFA) and scale reliability tests.
Methods
Participants
The questionnaire was administered to students in eight secondary schools in six provinces in China, and the subjects did not include any from the pretest stage. Questionnaires were considered invalid if: a. They included proactive approaches (Dunn et al., 2016) containing self-reported items, such as “I did not answer the above questions seriously” and “I answered the above questions truthfully according to my situation”; if these were answered incorrectly, the responses were deleted (Huang et al., 2012). b. More than half of the answers were blank. c. They had straight-lining (Curran, 2015; Fang et al., 2016), including both the choice of the same number throughout (e.g., 111,111) or a snake arrangement (e.g., 123,321). Cluster sampling and stratified sampling were used to obtain 4 samples, 1,553 questionnaires were distributed, invalid questionnaires were deleted, and finally, 1,321 valid questionnaires were obtained, with an effective rate of 85%. The specific sample composition is shown in Table 1.
Sample 1: A total of 624 secondary school students completed the SCLS (initial version), which was used for EFA. Among them, students in one secondary school in Ningxia Province received a web-based survey, while the rest of the students received an on-site questionnaire.
Sample 2: A total of 385 secondary school students completed the SCLS (formal version) for CFA. All students were administered the on-site questionnaire. Of these students, 150 were additionally required to fill out the Cognitive Load Questionnaire (CLQ), which was used to measure the scale validity.
Sample 3: A total of 226 secondary school students completed the SCLS (formal version) and the Motivated Strategies for Learning Questionnaire (MLSQ, Chinese version) for measuring the scale validity.
Sample 4: A total of 86 secondary school students completed the SCLS (formal version) for retest reliability analysis, all of whom received on-site questionnaires.
The students were recruited in a class setting, with the prior authorization of the teacher. Once written informed consent was obtained from every student, the survey was given in pen and paper format or via online questionnaire on a computer at the end of class.
Measures
Cognitive load
The CLQ uses a six-point Likert scale ranging from 1 to 6 for 15 self-reported items. The CLQ includes three dimensions, i.e., mental engagement, emotional engagement, and time engagement. The three dimensions measure “mental effort” in the cognitive load (Zhao, 2011). Mental effort is the core component of cognitive load and reflects effort and engagement in learning (Gerjets et al., 2009; Leppink et al., 2013; Chang et al., 2017). CL also reflects students’ engagement in and management of learning (Hunt, 1976a, 1977; Miller, 1981). Therefore, the present study hypothesized that CL is related to mental effort. In previous studies, the CLQ had good reliability and validity indicators (Li and Luo, 2014; Sun and Liu, 2016; Sun, 2016), as well as good internal consistency reliability and validity of the validity scales (Cronbach’s α of 0.798 for the total scale and 0.614 to 0.721 for the sub-scale), with good construct validity. In this study, Cronbach’s α of the CLQ was 0.87, and that of mental engagement, emotional engagement, and time engagement was 0.79, 0.81, and 0.84, respectively.
MSLQ (Chinese version)
Two subscales of the MSLQ (Chinese version) that reflect autonomous learning abilities, Strategy Use (13 items) and Self-Regulation (9 items), were selected for this study, with a total of 22 questions and 4 reverse-scored items. The MSLQ (Chinese version) uses a seven-point Likert scale ranging from 1 to 7 (Rao and Sachs, 1999). Both autonomous learning and CL can reflect the extent to which students are self-responsible in their learning (Hunt et al., 1974; Wirth et al., 2020), and both include core characteristics such as independence and self-awareness (Hunt, 1976a; Wong et al., 2018; Carpenter et al., 2020). Therefore, this study hypothesized that CL is associated with autonomous learning. In previous studies, the MSLQ (Chinese version) had good reliability and validity indicators (Cronbach’s α of 0.92; Li, and Yin, 2010; Lin et al., 2013) and also had good construct validity. In this study, the MSLQ (Chinese version) had a Cronbach’s α of 0.89, and Strategy Use and the Self-Regulation had Cronbach’s α of 0.82 and 0.80, respectively.
Academic scores
Academic scores refer to the final exam results of the semester, including three subjects: Chinese, math, and English. CL is an individual characteristic that, in general, has a direct relationship with academic scores (Ghazivakili et al., 2014; Yazici, 2016; İlçin et al., 2018). Therefore, this study hypothesized that CL is related to academic scores.
Data analysis
Using SPSS 25.0 software, item analysis, correlation analysis, and EFA were performed for the data from sample 1, and reliability analysis and CFA were performed for the data from sample 2. First, item–total statistics were used to test whether all the items were consistent with the scale. Inconsistent items were removed based on the results. Second, the Kaiser–Meyer–Olkin (KMO) test and Bartlett’s test of sphericity were used to test whether the data were appropriate for factor analysis. Third, the latent structure of the SCLS item set was explored by combining three principal factor extraction methods: principal component analysis (PCA), varimax, and parallel analysis (PA). The criteria for dimensions and item reduction were as follows (Fan et al., 2003; Wu, 2018): (1) eigenvalues >1; (2) factors containing three or more items; (3) items’ loading onto factors was sufficiently large (>0.40); and (4) items did not cross-load onto other factors (other factors <0.35). For the sample 2 data, Mplus 8.0 software was used for CFA.
Results
EFA
Item analysis
The aim of item analysis is to test the reliability of the items in the scale. The critical ratio (CR) of the sample 1 data and the item–total score correlation coefficients were used for item analysis of the 51 initial items using correlation analysis. First, the CR of the extreme groups was calculated, and the total scores were grouped into high and low groups. The first 27% of the total scores were considered the high group, the last 27% of the total scores were considered the low group, and the significance test of differences was done for the total and mean scores of the two extreme groups. Combining the two criteria with a significance level below 0.05 and CR <3.00, the four question items that did not meet the criteria were removed. Second, 11 question items were removed according to 2 criteria of correlation coefficients >0.3 and significance at the 0.05 level between each item and the total score of the scale, after which the test was conducted again.
Results of item analysis
The results showed that the correlation coefficients of each item with the total score ranged from 0.30 to 0.64. The skewness of all items ranged from −0.953 to 0.426, which did not exceed the critical value of 3. The kurtosis was −1.287 to 0.829, which was not >10, indicating that the scores of all questions conformed to a normal distribution. Meanwhile, the skewness and kurtosis were within the acceptable value range of ±2 (Trochim and Donnelly, 2008; Gravetter and Wallnau, 2014), indicating that none of the items had a substantial ceiling or floor effect.
Factor analysis
Based on the results of item analysis, EFA was performed on the 36 retained items. To ensure the feasibility of EFA, Bartlett’s sphericity test and the KMO test were performed before each EFA was done. The results of the first EFA showed that the KMO = 0.93 and Bartlett’s spherical test chi-square value (χ2(630) = 6868.03, p < 0.001) reached a significant level, indicating that the sample data were suitable for EFA (Hutcheson and Sofroniou, 1999; Field, 2013). PCA and varimax were selected to perform the extraction of common factors (Figure 1) according to the principle of an eigenvalue >1 (Fan et al., 2003), and combined with the gravel plot and PA test (O’connor, 2000; Hayton et al., 2004). Finally, 13 valid items were generated, and a total of 3 factors with eigenvalues >1 were obtained, explaining a total of 51.46% of the total variance. The PA results showed (Figure 1) that the first three factors in the actual data had greater eigenvalues than the average eigenvalue and 95th percentile eigenvalue of the random matrix and had a larger retention value. Meanwhile, the remaining factors were smaller than the average eigenvalue and 95th percentile eigenvalue of the random matrix, with relatively small retention value. The results of the two methods were consistent.
The final determination of the three extracted factors was consistent with theoretical expectations, reflecting three aspects of the CL: cognitive complexity, independence, and environmental adaptability. The scale factor loadings are shown in Table 2. According to the content reflected by the items contained in each factor, factor 1 was named learning awareness, which refers to the degree of conscious awareness of learning goals, plans, and tasks. It reflects the individual’s level of awareness of learning itself and his or her own learning state and contains five items. Factor 2 was named autonomous input, which refers to the individual’s mental ability to consciously inhibit distractions, resist temptations, and think deeply during learning, reflecting the level of independent learning. Containing four items, factor 3 was named environmental coping, which refers to the level of dealing with conflict situations and difficult situations and reflects the degree of individual adaptation to the environment. Based on the connotation of the three factors, this study defines CL as the level of learners’ psychological development in three areas: learning awareness, learning behavior, and processing information from the internal and external environment.
CFA
To verify the structural validity of our scale, the structural equation modeling statistical software Mplus 8.0 was used for CFA on the sample 2 data. To evaluate the CFA models, we considered four fit statistics as primary indicators of model fit: the Tucker Lewis index (TLI; Tucker and Lewis, 1973), the comparative fit index (CFI; Bentler, 1990), the standardized root mean square residual (SRMR; Pavlov et al., 2021), and the root mean square error of approximation (RMSEA; Browne and Cudeck, 1992) due to the sensitivity of the chi-square statistic to sample size.
Results of CFA
The results of CFA are shown in Table 3. Among the three factors, the fitted indicators for each of the learning awareness factors were χ2 = 10.192, df = 5, CFI = 0.979, TLI = 0.958, RMSEA = 0.052, and SRMR = 0.031. The fitted indicators for each of the autonomous input factors were χ2 = 13.443, df = 2, CFI = 0.990, TLI = 0.970, RMSEA = 0.043, and SRMR = 0.020. The fitted indicators for each of the environmental coping factors were χ2 = 32.033, df = 6, CFI = 0.798, TLI = 0.663, RMSEA = 0.10, and SRMR = 0.089. The scale was validated, and the first-order three-factor model underperformed on all indicators: χ2 = 253.582, df = 65, CFI = 0.721, TLI = 0.665, RMSEA = 0.087, SRMR = 0.075. The second-order three-factor model fit was excellent, and the indicators were χ2 = 100.461, df = 62, CFI = 0.943, TLI = 0.928, RMSEA = 0.040, and SRMR = 0.046. The data statistics showed that the second-order three-factor model fit better than the first-order three-factor model and was more consistent with theoretical expectations, indicating that the SCLS had good structural validity, and the later analysis was based on this model.
Reliability and validity assessment
Partial correlations and reliability test
First, sample 2 (n = 385) was analyzed for partial correlation between the total scale and 3 subscales. Second, Cronbach’s α, retest reliability, Spearman–Brown, and CR were tested for the total scale and three subscales. In addition, 95 subjects in sample 4 were randomly selected (valid n = 86), and the interval between the pre-and post-tests was 3 weeks to test the retest reliability of SCLS. The main reliability indexes are shown in Table 4. All the reliability indexes of SCLS met the acceptable standard of the reliability coefficient (Wu, 2018, P244). The square root of the average variance extracted (AVE) of the three subscales was greater than the correlation coefficients of 0.33, 0.40, and 0.31, indicating good discriminant validity. In conclusion, when evaluating the CL of students, it is better to refer to the SCLS total score and the scores of the three high-order factors, rather than explain the scores of the 13 low-order factors alone.
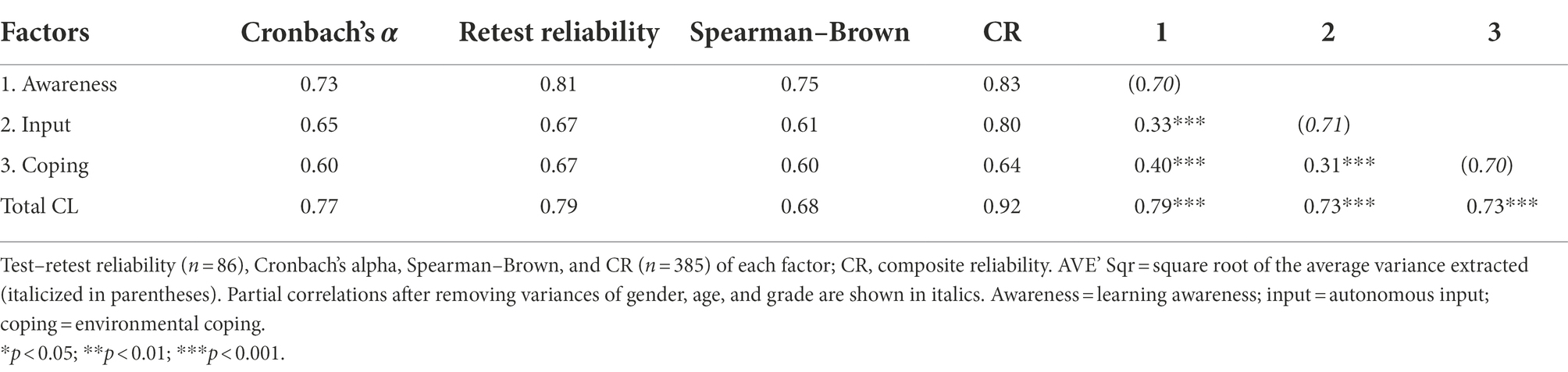
Table 4. Partial Correlations, Cronbach’s Alpha, Retest Reliability, and CR (and AVE’ Sqr for) Between SCLS’ Full-Scale and Subscale Scores.
Criterion validity
Since the method used to collect data in this study was self-reporting, there was a possibility of common method variance (CMV). Therefore, the Harman single-factor test was used for both sample data. The results showed that there were 11 factors with eigenvalues >1 in sample 2, and the variance explained by the first factor was 17.43%, which was less than the critical criterion of 40%. In sample 3, there were eight factors with eigenvalues >1, and the first factor explained 28.94% of the variance. Both samples were <40% of the critical threshold, indicating that there was no serious CMV in this study (Zhou and Long, 2004). In the absence of severe CMV, the study then tested the correlations of the three factors of the SCLS with Chinese, math, and English scores, respectively, and the results are shown in Table 5. The calibration validity between CLQ, MSLQ, and SCLS was analyzed based on a valid sample size of 376. In CLQ, the SCLS total score was correlated with 16 mental effort items, of which 15 items were significantly correlated (0.22 ≤ r ≤ 0.66, p < 0.01); there was no significant correlation between autonomous input of SCLS and emotion engagement of mental effort. In the MLSQ (Chinese version), SCLS has 12 correlations with autonomous input, all of which are significantly correlated (0.27 ≤ r ≤ 0.83, p < 0.001). In academic scores, the SCLS total score was significantly positively correlated with the academic total score and English score (0.10 ≤ r ≤ 0.13, p < 0.05) and had no significant correlation with math or Chinese scores. There was no significant correlation between the environmental coping and the autonomous input with the scores of all subjects.
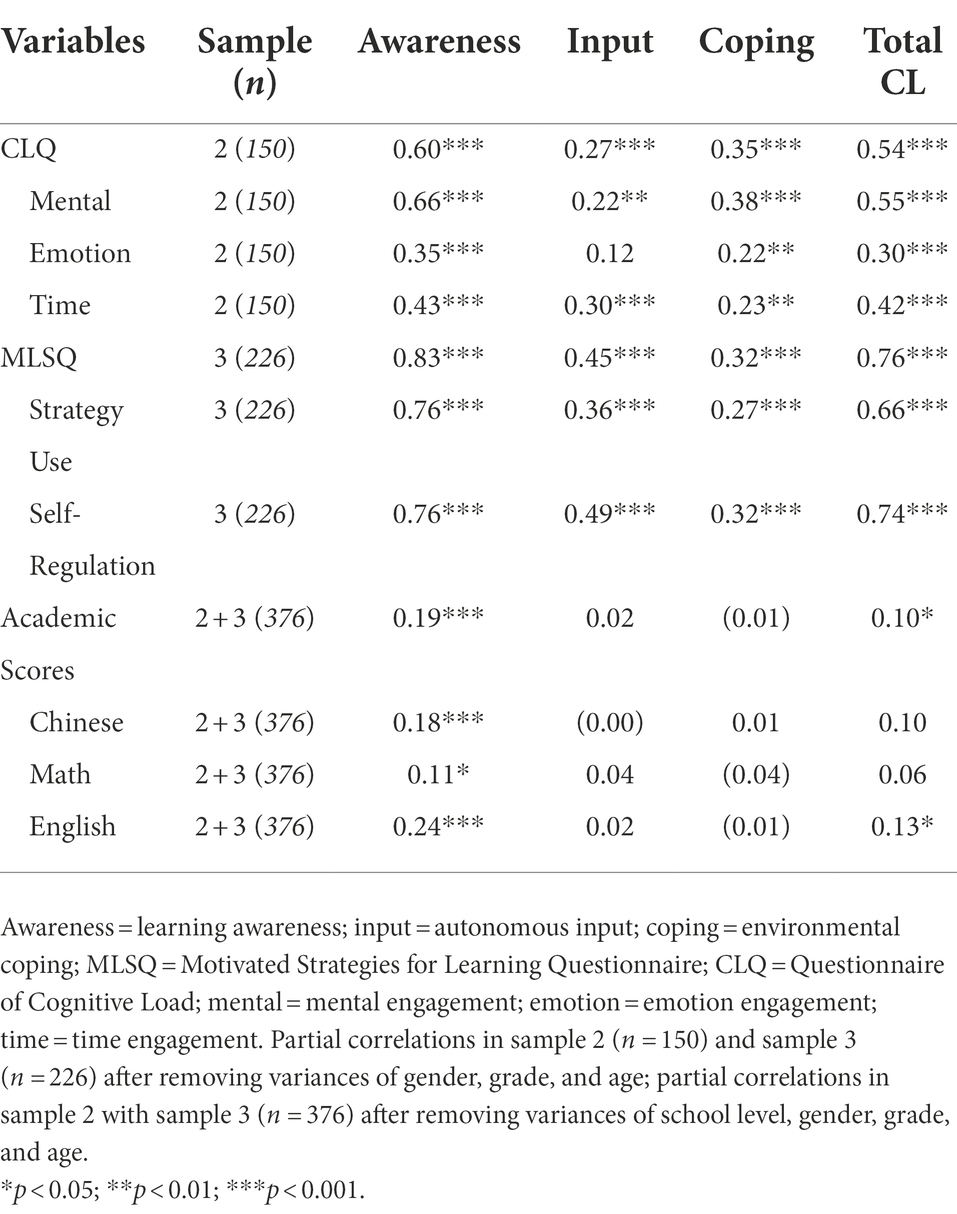
Table 5. Partial correlations between SCLS (and its three subscales), CLQ, MLSQ, and academic scores.
Discussion
The purpose of this research was to create and validate a survey measuring student conceptual level, specifically for secondary school students. The EFA of SCLS showed that the statistical results were consistent with the constructed theoretical framework of conceptual level and that the three factors both independently represented the significance of their respective scores and combined to reflect the overall conceptual level. The three factors contributed 51.46% of the total variance. The results of the CFA test showed that the model had high structural validity and met the assumptions of the empirical construction of students’ conceptual level. Reliability and validity analyses showed high correlations between the dimensions of the scale and the variables. The Cronbach’s α, Spearman–Brown, CR, and retest reliability of both the SCLS total and subscales exceeded the 0.6 critical standard, indicating good overall internal consistency and retest reliability. The results of this study provide sufficient validity evidence for the scores, generalizations, and extrapolations of the SCLS’ full scale and subscales, as well as for the significance and utility of the subscales, indicating that the scale can assess student CL.
Empirical construction of conceptual level
In the social domain, CL is defined as a personality trait. In the teaching field, CL is defined as learning style. Both define CL as a developmental index that has value for personal growth (Harvey et al., 1962; Hunt et al., 1978). In addition to affirming the value of CL, there is some disagreement and confusion between the two fields as to the exact meaning and internal structure of CL. First, the CL for personality orientation describes only cognitive dispositions, whereas the CL for learning style orientation describes both cognitive dispositions and behavioral characteristics. Second, the CL for personality orientation is how individuals behave in general, whereas the CL for learning style orientation is how students behave in learning situations. Finally, the initial measurement scale for this study adapted the PCM themes about rules, authority, and parenting but did not show good statistical properties and was solidly removed. The above three points show that the theoretical connotation and internal structure of the CL of learning style orientation have changed; not only the connotation is different from the personality orientation, but also the measurement instrument proves the inapplicability of PCM. This study explores the changes in the theoretical connotation and inner structure of CL with learning style orientation, not only theoretically deepening the learning style connotation of CL but also addressing the reality of measurement tools.
In quantitative research, a clear definition of a concept is the basis for operationalizing and quantifying that concept. Based on the connotations of learning in CL, this study is empirically constructed using a realist perspective around the characteristics of the three aspects proposed by Hunt (cognitive complexity, independence, and environmental adaptability) and analyzes which characteristics students have that reflect these three aspects and which specific factors these three aspects focus on. Based on the theoretical constructs, this study used logical methods such as generalization and induction to gradually construct the empirical dimensions of students’ CL.
Item screening was a key component in the development of the SCLS. To improve the reliability of the scale, this study analyzed each item in the scale through item analysis to find differences between high and low subgroups and to screen out high-quality items to constitute the scale. The correlation coefficients between the scale items and the full scale reached statistically significant levels, except for the expert evaluations. The correlation between each item and the total score was significant, with coefficients ranging from 0.30 to 0.64. The skewness of all items ranged from −0.953 to 0.426, which did not exceed the critical value of 3. The kurtosis ranged from −1.287 to 0.829, which was not >10, indicating that the scores of all items conformed to a normal distribution and had a high degree of discrimination.
Dimensions of conceptual level
The student conceptual level consists of three factors—learning awareness, autonomous input, and environmental coping—reflecting the three aspects proposed by Hunt: cognitive complexity, independence, and environmental adaptability. These three relatively independent but interrelated components make up the student conceptual level.
Among them, learning awareness reflects the level of development of students on the concrete–abstract cognitive dimension of learning itself, of their own learning state. For example, for students with low learning awareness, teachers should initially set goals and plans for them, organize simpler learning tasks, and pay attention to how students’ cognition evolves on the concrete–abstract dimension. When students’ cognitive abstraction level is improved, teachers should reduce participation and encourage students to plan their own learning. Autonomous input reflects the level of student development on the dependent–independent learning dimension. For example, for students with high levels of dependency, teachers or parents should act as “supervisors” and try to create a learning environment free of distractions and temptations. When students become more independent, teachers or parents should provide a free learning environment, reduce intervention, and encourage students to self-manage. Environmental coping reflects the level of students’ processing of external events on the conflict–balance dimension. For example, when students with a low level of environmental coping encounter conflict or difficult situations (interpersonal and learning situations), teachers should promptly help them construct a good psychological environment and develop interpersonal harmony and psychological resilience.
The above three factors validate the initial theoretical constructs of this study, and the meaning of each is well defined. Therefore, the questionnaire structure of this study is complete.
Reliability and validity of the SCLS
In this study, construct validity, discriminant validity, and criterion validity were used to reflect the overall validity of the scale.
CFA showed that the three-factor structural model of SCLS obtained by EFA had a good fit index. Significant correlations (p < 0.001) were found among the factors and between each factor and the total score, indicating that the scale has good construct validity.
The AVE’ square root for all three factors was greater than the correlation coefficients among the three, indicating good discriminant validity.
In this study, two internal criteria were selected to reflect concurrent validity. Among them, LCQ reflected mental effort. Sixteen of the SCLS items were correlated with the LCQ, 15 of which were significant, confirming a strong relationship between mental effort and individual characteristics (Miller, 1956; Sweller, 2010; Sweller et al., 2019; Paas and Merrinboer, 2020; Brosnan et al., 2021). However, the correlation between autonomous input and emotional engagement (controlling bad emotions and regulating bad moods) was not significant, probably because the level of autonomous input involved cognitive and behavioral dimensions, whereas emotional engagement reflected emotional management, and emotions were not always consistent with cognition and behavior. The MSLQ (Chinese) reflects self-directed learning ability. There were 12 item correlations between SCLS and MSLQ (Chinese), all of which were significant (0.27 ≤ r ≤ 0.83, p < 0.001). Research has found that students who use effective learning strategies and have high levels of self-management are more likely to learn autonomously and have a more internal and deeper understanding of the meaning of learning (Zimmerman, 1986; Pintrich, 2000; Efklides et al., 2018). This supports the theoretical connotation of CL as a developmental goal of “autonomy”: It reflects the degree of autonomy of the learner (Harvey et al., 1962; Hunt, 1971, 1975a, 1976a). The above two validity tests demonstrated the good concurrent validity of the SCLS.
This study also selected students’ academic scores as an external validity marker to reflect predictive validity. Total SCLS scores were significantly positively correlated with total academic scores and English scores, while all other factors were not significantly correlated. This result aptly demonstrates the theoretical connotation of CL: CL is a learning style (Hunt, 1971; Hunt et al., 1974; Tan, 1995; Flowers et al., 2000) that reflects how one learns, not what one learns. Academic scores are a test of accumulated knowledge experience, reflecting what was learned, not how it was learned. CL cannot be linked to academic scores without matching with instruction, and only matched instruction can truly facilitate student learning, which is the core meaning of CL in teaching and learning (Hunt et al., 1974, 1978; Miller, 1981; Tan, 1995). Thus, CL is not simply linearly related to academic scores, and the relationship should be interpreted with caution (Miller, 1978). Specifically, although the total SCLS score was significantly positively correlated with both total academic scores and English scores (0.11 ≤ r ≤ 0.24, p < 0.05), the correlation was low. The total SCLS score was not significantly correlated with math and Chinese scores. Only the learning awareness was significantly positively correlated with scores in all subjects (0.11 ≤ r ≤ 0.24, p < 0.05). It is not difficult to explain that learning awareness reflects students’ in-depth thinking and execution of learning, while strategies and planning predict the achievement of course goals (Kizilcec et al., 2017). Autonomous input was not significantly correlated with all subjects’ scores. This can be explained in two ways: (1) The relationship between input and academic scores is not unidirectional; high input does not mean a high score (Bruin et al., 2020). For example, students can complete academic work and perform well and get good scores without necessarily being invested in it; conversely, there are students who invest a lot of effort in some details and procedures but do not really develop their thinking. This has been classified into two types of effort: objective effort and subjective effort (Dunlosky and Mueller, 2016; Dunlosky et al., 2020). The former is a superficial input, while the latter is a substantive input, and only a substantive input can truly facilitate learning. (2) From the perspective of economics, there is a law of diminishing marginal returns in the learning process: With the increase of learning input, the initial achievement increases, and when the input exceeds a certain limit, this increasing trend gradually decreases and eventually decreases absolutely (Bai, 1999; Shen, 2014), which indicates that the increase of learning input does not necessarily lead to the improvement of learning achievement, and the key is to find the optimal combination between learning inputs and outputs. Environmental coping was not significantly correlated with all subjects’ scores. It reflects how students balance themselves with various elements of their environment and is an unrelated aspect of learning; therefore, it does not directly reflect achievement and may be influenced by other factors. The relationship between each subject score and the total SCLS score needs to be analyzed according to the subject characteristics of the secondary school (grade7-9) curriculum of the Ministry of Education (2011). Chinese and math scores reflect thinking levels and prior knowledge more prominently than English scores and require higher levels of learning ability. Meanwhile, CL is not a learning ability but a cognitive and behavioral characteristic. Secondary school (grade7-9) English does not require a high level of thinking and a priori knowledge and it is still at the primary stage. English learning highlights individual differences in learning characteristics, habits, language communication, social interaction, etc. This is consistent with the connotation of individual differences reflected by CL, so the association between the subject of English and CL is relatively close.
All reliability indicators, such as Cronbach’s α, retest reliability, and Spearman–Brown, met the acceptable criteria for reliability coefficients in this study (Wu, 2018, P244). However, Cronbach’s α for the subscales was only at or near the critical value. This may be related to the length of the test: The number of items on each factor of the SCLS was 3 or 4, which may have led to low reliability. An effective solution would be to increase the length of the test appropriately (Dai et al., 1999).
Limitations and future directions
Owing to the slow research progress of CL theory in the past 20 years, this study focused on the classic literature from the 1960s–1980s in terms of literature acquisition and reference. When we were reconstructing this theory, we lacked empirical comparative studies in the context of the changing times. At the same time, there may be some differences between the grasping of the meaning of the classical theory and the content freely generated by the contemporary subjects. Of course, the theory in these classic texts is not cut off from later generations in the study of personality traits, and its successful application in the field of counseling and therapy is sufficient to show that CL has had a profound influence on personality research. The present study does not further follow the classical theoretical ideas to find a broader developmentally appropriate ground for CL and only explores the significance and internal structure of CL in terms of learning styles, without restoring its significance in personality development and re-exploring the internal structure. Therefore, it is difficult to answer the question, “Has CL also undergone fundamental changes in the assessment of personality based on the social domain?” in this study. In general, the secondary school level is only at a certain stage of learning, with particular temporal and environmental characteristics, while individual learning is permeated by various environments and stages of existence in question. Therefore, it is necessary to explore aspects of CL that may be of value in multiple contexts and stages.
Although the results of this study help to define the connotation of learning styles in CL, there are still some issues to be addressed in terms of validity. Additional follow-up research is needed to address the limitations of this study. (1) Although the characteristics of the sample can be generalized to populations with similar characteristics, they may not apply to populations with different characteristics, lifestyles, or ages in other countries. In future studies, it will be necessary to consider samples from other cultures and test for invariance across groups to extend and support the findings and contributions of this study. (2) It is necessary to combine other forms, such as individual qualitative analysis techniques (e.g., semi-structured interviews, observation, content analysis of autobiographical texts) to deepen the nature of CL authenticity and individual differences among students.
Conclusion
The SCLS developed in this study has good reliability and validity, meets psychometric requirements, and reflects the actual status of the students’ CL. The SCLS has 13 items, including 3 factors: learning awareness, autonomous input, and environmental coping. This study demonstrated that the Student Conceptual Level Scale (SCLS) provides an efficient, reliable, and accurate way to assess learning styles. The SCLS can be used not only to assess student learning and individual development but also to help us understand the causes of differences in CL across students and to reduce this gap through instruction.
Data availability statement
The original contributions presented in the study are included in the article/Supplementary material, further inquiries can be directed to the corresponding author.
Ethics statement
The studies involving human participants were reviewed and approved by Nanjing Normal University. Written informed consent to participate in this study was provided by the participants’ legal guardian/next of kin. Written informed consent was obtained from the individual(s), and minor(s)' legal guardian/next of kin, for the publication of any potentially identifiable images or data included in this article.
Author contributions
XY was responsible for the original conception, data collection, and data analysis and constructed the original concepts. DT and JD critically revised the manuscript. All authors approved the final version of the manuscript for submission.
Acknowledgments
We are grateful for the generous contributions of study participants and staff who assisted with data collection during the study. We thank LetPub (www.letpub.com) for its linguistic assistance during the preparation of this manuscript.
Conflict of interest
The authors declare that the research was conducted in the absence of any commercial or financial relationships that could be construed as a potential conflict of interest.
Publisher’s note
All claims expressed in this article are solely those of the authors and do not necessarily represent those of their affiliated organizations, or those of the publisher, the editors and the reviewers. Any product that may be evaluated in this article, or claim that may be made by its manufacturer, is not guaranteed or endorsed by the publisher.
References
Bai, Y. M. (1999). A review of the relationship between learning time and learning outcome. Stud. Foreign Educ. 6:7.
Bentler, P. M. (1990). Comparative fit indexes in structural models. Psychol. Bull. 107, 238–246. doi: 10.1037/0033-2909.107.2.238
Brill, R. (1978). Implications of the cl matching model for treatment of delinquents. J. Res. Crime Delinq. 15, 229–246. doi: 10.1177/002242787801500208
Brophy, J. E., and Good, T. L. (1974). Teacher-student relationships: causes and consequences. Am. J. Educ. 6, 249–250. Available at: https://eric.ed.gov/?id=ED091495
Brosnan, K., Grün, B., and Dolnicar, S. (2021). Cognitive load reduction strategies in questionnaire design. Int. J. Mark. Res. 63, 125–133. doi: 10.1177/1470785320986797
Browne, M. W., and Cudeck, R. (1992). Alternative ways of assessing model fit. Sociol. Methods Res. 21, 230–258. doi: 10.1177/0049124192021002005
Bruin, A. B. H. D., Roelle, J., Carpenter, S. K., Baars, M., and Trypke, M. (2020). Synthesizing cognitive load and self-regulation theory: a theoretical framework and research agenda. Educ. Psychol. Rev. 32, 903–915. doi: 10.1007/s10648-020-09576-4
Carpenter, S. K., Endres, T., and Hui, L. (2020). Students' use of retrieval in self-regulated learning: implications for monitoring and regulating effortful learning experiences. Educ. Psychol. Rev. 32, 1029–1054. doi: 10.1007/s10648-020-09562-w
Chang, C. C., Liang, C. Y., Chou, P. N., and Lin, G. Y. (2017). Is game-based learning better in flow experience and various types of cognitive load than non-game-based learning? Perspective from multimedia and media richness. Comput. Hum. Behav. 71, 218–227. doi: 10.1016/j.chb.2017.01.031
Curran, P. G. (2015). Methods for the detection of carelessly invalid responses in survey data. J. Exp. Soc. Psychol. 66, 4–19. doi: 10.1016/j.jesp.2015.07.006
Dai, H. Q., Zhang, F., and Chen, X. F. (1999). Psychological and Educational Measurement. (Jinan: Jinan University Press). 241–242.
Doyle, W., and Rutherford, B. (1984). Classroom research on matching learning and teaching styles. Theory Pract. 23, 20–25. doi: 10.2307/1476734
Dunlosky, J., Badali, S., Rivers, M. L., and Rawson, K. A. (2020). The role of effort in understanding educational achievement: objective effort as an explanatory construct versus effort as a student perception. Educ. Psychol. Rev. 32, 1163–1175. doi: 10.1007/s10648-020-09577-3
Dunlosky, J., and Mueller, M. (2016). Recommendations for exploring the disfluency hypothesis toward establishing whether perceptually degrading materials impacts performance. Metacogn. Learn. 11, 123–131. doi: 10.1007/s11409-016-9155-9
Dunn, A. M., Heggestad, E. D., Shanock, L. R., and Theilgard, N. (2016). Intra-individual response variability as an indicator of insufficient effort responding: comparison to other indicators and relationships with individual differences. J. Bus. Psychol. 33, 105–121. doi: 10.1007/s10869-016-9479-0
Efklides, A., Schwartz, B. L., and Brown, V. (2018). “Motivation and affect in self-regulated learning: does metacognition play a role,” in Handbook of Self-regulation of Learning and Performance. 2nd Edn. eds. D. H. Schunk and J. A. Greene
Erikson, E. H. (1959). Identity and the life cycle: selected papers. Psychological Issues. (US: International Universities Press, Inc.), 1–171.
Fan, J. Y., Ye, B., Zhang, Z. Y., and Liu, B. X. (2003). Exploratory factor analysis: a literature review between 1991 and 2000. Adv. Psychol. Sci. 11, 579–585. doi: 10.3969/j.issn.1671-3710.2003.05.018
Fang, J. M., Prybutok, V., and Wen, C. (2016). Shirking behavior and socially desirable responding in online surveys: a cross-cultural study comparing Chinese and American samples. Comput. Hum. Behav. 54, 310–317. doi: 10.1016/j.chb.2015.08.019
Field, A. (2013). Discovering Statistics Using IBM SPSS Statistics (Paperback and Web Assign). 4th Edn. SAGE Publications Ltd.
Flowers, C. P., Hancock, D. R., and Joyner, R. E. (2000). Effects of instructional strategies and cls on students' motivation and achievement in a technology course. J. Res. Dev. Educ. 33, 187–194. Available at: https://eric.ed.gov/?id=EJ612234
Gardiner, G. S., and Schroder, H. M. (1972). Reliability and validity of the paragraph completion test: theoretical and empirical notes. Psychol. Rep. 31, 959–962. doi: 10.2466/pr0.1972.31.3.959
Gerjets, P., Scheiter, K., Opfermann, M., Hesse, F. W., and Eysink, T. (2009). Learning with hypermedia: the influence of representational formats and different levels of learner control on performance and learning behavior. Comput. Hum. Behav. 25, 360–370. doi: 10.1016/j.chb.2008.12.015
Ghazivakili, Z., Nia, R. N., Panahi, F., Karimi, M., and Ahmadi, Z. (2014). The role of critical thinking skills and learning styles of university students in their academic performance. J. Adv. Med. Educ. Prof. 2, 95–102. Available at: https://www.ncbi.nlm.nih.gov/pmc/articles/PMC4235550/pdf/jamp-2-95.pdf.
Goldberg, A. D. (1974). Conceptual system as a predisposition toward therapeutic communication. J. Couns. Psychol. 21, 364–368. doi: 10.1037/h0037108
Gravetter, F. J., and Wallnau, L. B. (2014). Essentials of Statistics for the Behavioral Sciences 8th Edn. Available at: https://xueshu.baidu.com/usercenter/paper/show?paperid=ed7fae97af6284c7ad70df26587704ac
Greaves, G. (1971). Harvey's “this I believe” test: studies of reliability. Psychol. Rep. 28, 387–390. doi: 10.2466/pr0.1971.28.2.387
Harold, M. S., Michael, J. D., and Siegfried, S. (1967). Human information processing: individuals and groups functioning in complex social situations. Am. J. Psychol. 83:136. doi: 10.2307/1420865
Harvey, O. J., Hunt, D. E., and Schroder, H. M. (1962). Conceptual systems and personality organization. Psychosomatics 76:163. doi: 10.2307/1420024
Hayton, J. C., Allen, D. G., and Scarpello, V. (2004). Factor retention decisions in exploratory factor analysis: a tutorial on parallel analysis. Organ. Res. Methods 7, 191–205. doi: 10.1177/1094428104263675
Huang, J. L., Curran, P. G., Keeney, J., Poposki, E. M., and DeShon, R. P. (2012). Detecting and deterring insufficient effort responding to surveys. Journal of Business and Psychology 27, 99–114. doi: 10.2307/41474909
Hunt, D. E. (1970). A CL matching model for coordinating learner characteristics with educational approaches. Interchange 1, 68–82. doi: 10.1007/BF02214677
Hunt, D. E. (1971). Matching Models in Education: The Coordination of Teaching Methods with Student Characteristics, Toronto: Ontario Institute for Studies in Education. Available at: https://psycnet.apa.org/record/1971-27828-001
Hunt, D. E. (1975a). Person-environment interaction: a challenge found wanting before it was tried. Rev. Educ. Res. 45, 209–230. doi: 10.3102/00346543045002209
Hunt, D. E. (1975b). The B-P-E paradigm for theory, research and practice. Can. Psychol. Rev. Psychologie Canadienne 16, 185–197. doi: 10.1037/h0081805
Hunt, D. E. (1975c). Teachers' adaptation to students: implicit and explicit matching. Research and development memorandum no. 139. Adjustment :50. Available at: https://eric.ed.gov/?id=ED117090
Hunt, D. E. (1976a). Teachers are psychologists, too: on the application of psychology to education. ACT Res. Rep. 17, 210–218. doi: 10.1037/h0081840
Hunt, D. E. (1976b). Teachers' adaptation: 'Reading' and 'Flexing' to students. J. Teach. Educ. 27, 268–275. doi: 10.1177/002248717602700323
Hunt, D. E. (1977). Conceptual level theory and research as guides to educational practice. Interchange 8, 78–90. doi: 10.1007/BF01810464
Hunt, D. E. (1979). “Learning style and student needs: an introduction to conceptual level,” in Student Learning Styles: Diagnosing and Prescribing Programs. ed. NASSP (Reston: National Association of High School Principals), 27–38. Available at: https://eric.ed.gov/?id=ED182859
Hunt, D. E., Butler, L. F., Noy, J. E., and Rosser, M. E. (1978). Assessing CL by the Paragraph Completion Method. Toronto: Ontario Institute for Studies in Education. (unpublished online).
Hunt, D. E., Joyce, B. R., Greenwood, J. A., Noy, J. E., Reid, R., and Weil, M. (1974). Student cl and models of teaching: theoretical and empirical coordination of two models. Interchange 5, 19–30. doi: 10.1007/BF02138804
Hutcheson, G. D., and Sofroniou, N. (1999). The Multivariate Social Scientist: Introductory Statistics Using Generalized Linear Models. Sage Publications.
İlçin, N., Tomruk, M., Yeşilyaprak, S. S., Karadibak, D., and Savcı, S. (2018). The relationship between learning styles and academic performance in Turkish physiotherapy students. BMC Med. Educ. 18:291. doi: 10.1186/s12909-018-1400-2
Kizilcec, R. F., Pérez-Sanagustín, M., and Maldonado, J. J. (2017). Self-regulated learning strategies predict learner behavior and goal attainment in massive open online courses. Comput. Educ. 104, 18–33. doi: 10.1016/j.compedu.2016.10.001
Leppink, J., Paas, F., Van der Vleuten, C. P. M., Van Gog, T., and Van Merriënboer, J. J. G. (2013). Development of an instrument for measuring different types of cognitive load. Behav. Res. Methods 45, 1058–1072. doi: 10.3758/s13428-013-0334-1
Lewin, K. (1936). A dynamic theory of personality: selected papers. J. Nerv. Ment. Dis. 33, 548–552. doi: 10.1037/h0050556
Li, X. D., and Lin, C. D. (2001). Academic help-seeking strategies: its relation to personal goal orientation. Classroom Goal Struct. Culture Factors Psychol. Dev. Educ. 17:7. doi: 10.3969/j.issn.1001-4918.2001.02.001
Li, H. M., and Luo, S. Q. (2014). Teaching efficacy logic of academic burden problem solving. Res. Educ. Dev. 10:6. Available at: http://en.cnki.com.cn/Article_en/CJFDTOTAL-SHGJ201410016.htm
Li, Z. J., and Yin, H. B. (2010). The impact of classroom environment on Students' self-regulated learning in Hong Kong: a commentary on the debate of teacher-centeredness and student-centeredness. Peking Univ. Educ. Rev. 1, 70–82. doi: 10.19355/j.cnki.1671-9468.2010.01.008
Lin, T. J., Deng, F., Chai, C. S., and Tsai, C. C. (2013). High school students' scientific epistemological beliefs, motivation in learning science, and their relationships: a comparative study within the Chinese culture. Int. J. Educ. Dev. 33, 37–47. doi: 10.1016/j.ijedudev.2012.01.007
McLachlan, J. F. (1974). Therapy strategies, personality orientation and recovery from alcoholism. Can. Psychiatr. Assoc. J. 19, 25–30. doi: 10.1177/070674377401900106
McLachlan, J. F., and Hunt, D. E. (1973). Differential effects of discovery learning as a function of student CL. Can. J. Behaviouralence Revue Canadienne Desences Du Comportement 5, 152–160. doi: 10.1037/h0082340
Miller, G. A. (1956). The magic number seven plus or minus two: some limits on our capacity for processing information. Psychol. Rev. 101, 343–352. doi: 10.1037/0033-295X.101.2.343
Miller, A. (1978). Conceptual systems theory: a critical review. Genet. Psychol. Monogr. 97, 77–126.
Miller, A. (1981). Conceptual matching models and interactional research in education. Rev. Educ. Res. 51, 33–84. doi: 10.3102/00346543051001033
Ministry of Education (2011). Curriculum Standards for Compulsory Education. Beijing: PRC. Available at: http://www.moe.gov.cn/srcsite/A26/s8001/201112/t20111228_167340.html (Accessed May 30, 2022).
Murphy, P. D., and Brown, M. M. (1970). Conceptual systems and teaching styles. Am. Educ. Res. J. 7, 529–540. doi: 10.2307/1161834
Nie, Y. G., and Ding, L. (2009). The characters of adolescent self-consciousness and its relationship with social adaptive behavior. Psychol. Dev. Educ. 2:8. doi: 10.3969/j.issn.1674-196X.2014.37.043
O’connor, B. P. (2000). SPSS and SAS programs for determining the number of components using parallel analysis and velicer's map test. Behav. Res. Methods Instrum. Comput. 32, 396–402. doi: 10.3758/BF03200807
Paas, F., and Merrinboer, J. J. G. V. (2020). Cognitive-load theory: methods to manage working memory load in the learning of complex tasks. Curr. Dir. Psychol. Sci. 29, 394–398. doi: 10.1177/0963721420922183
Pavlov, G., Maydeu-Olivares, A., and Shi, D. (2021). Using the standardized root mean squared residual (SRMR) to assess exact fit in structural equation models. Educ. Psychol. Meas. 81, 110–130. doi: 10.1177/0013164420926231
Phillips, M., and Sinclair, R. (1973). Conceptual systems and educational environment: relationships between teacher conceptual systems, student conceptual systems, and classroom environment as perceived by fifth and sixth grade students. Classroom Environ. 30, 1–23. Available at: https://files.eric.ed.gov/fulltext/ED076539.pdf
Piaget, J. (1953). The child's conception of number. American Sociological Review. 18, 711–712. doi: 10.2307/2088144
Pintrich, P. R. (2000). “The role of goal orientation in self-regulated learning,” in Handbook of Self-regulation. eds. M. Boekaerts, P. R. Pintrich, and M. Zeidner, 451–494. doi: 10.1016/B978-012109890-2/50043-3
Rao, N., and Sachs, J. (1999). Confirmatory factor analysis of the Chinese version of the motivated strategies for learning questionnaire. Educ. Psychol. Meas. 59, 1016–1029. doi: 10.1177/00131649921970206
Shen, X. J. (2014). Research on Shanghai high school Students' optimal learning time based on PISA data. Res. Educ. Dev. 4:6. doi: 10.14121/j.cnki.1008-3855.2014.04.014
Stoppard, J. M., and Miller, A. (1985). Conceptual level matching in therapy: a review. Current Psychol. Res. Rev. 4, 46–68. doi: 10.1007/BF02686567
Sun, C. Y. (2016). Research on adolescent academic burden from the perspective of cognitive load. Educ. Exploration, 4. Available at: http://www.cnki.com.cn/Article/CJFDTotal-SEEK201604010.htm
Sun, C. Y., and Liu, D. Z. (2016). Effects of the background color of learning materials on cognitive load and learning performance. J. Psychol. Sci. 39, 869–874. doi: 10.16719/j.cnki.1671-6981.20160416
Sweller, J. (2010). Element interactivity and intrinsic, extraneous, and germane cognitive load. Educ. Psychol. Rev. 22, 123–138. doi: 10.1007/s10648-010-9128-5
Sweller, J., van Merrienboer, J. J. G., and Paas, F. (2019). Cognitive architecture and instructional design: 20 years later. Educ. Psychol. Rev. 31, 261–292. doi: 10.1007/s10648-019-09465-5
Tomlinson, P. D., and Hunt, D. E. (1971). Differential effects of rule-example order as a function of learner conceptual level. Can. J. Behav. Sci./revue Canadienne Des Sciences Du Comportement 3, 237–245. doi: 10.1037/h0082265
Trochim, W. M., and Donnelly, J. P. (2008). The Research Methods Knowledge Base. Available at: https://www.researchgate.net/publication/281345819_The_Research_Methods_Knowledge_Base (Accessed May 30, 2022).
Tucker, L. R., and Lewis, C. (1973). A reliability coefficient for maximum likelihood factor analysis. Psychometrika 38, 1–10. doi: 10.1007/BF02291170
Tuckman, W. B. (1965). Integrative complexity: its measurement and relation to creativity. Educ. Psychol. Meas. 26, 369–382. doi: 10.1177/001316446602600209
Wang, H. J., and Lu, J. M. (2004). The compilation of the high school students’ self-control ability questionnaire. J. Psychol. Sci. 6, 198–203. doi: 10.16719/j.cnki.1671-6981.2004.06.055
Wirth, J., St Ebner, F., Trypke, M., Schuster, C., and Leutner, D. (2020). An interactive layers model of self-regulated learning and cognitive load. Educ. Psychol. Rev. 32, 1127–1149. doi: 10.1007/s10648-020-09568-4
Wong, J., Baars, M., Davis, D., Tim, V. D. Z., Houben, G. J., and Paas, F. (2018). Supporting self-regulated learning in online learning environments and moocs: a systematic review. Int. J. Hum.-Comput. Interact. 35, 356–373. doi: 10.1080/10447318.2018.1543084
Wu, M. L. (2018). Practice of questionnaire statistical analysis: Operation and application of SPSS. (Chongqing: Chongqing University Press). 244.
Yazici, H. J. (2016). Role of learning style preferences and interactive response systems on student learning outcomes. Int. J. Inf. Oper. Manage. Educ. 6:109. doi: 10.1504/IJIOME.2016.076046
Zhang, Q., Wang, Y., Zhang, L., and Zhang, H. (2010). The development of high school students' informal reasoning and influence factors. Psychol. Explor. 6:6. doi: 10.3969/j.issn.1003-5184.2010.06.013
Zhao, J.-F. (2011). Deciphering academic load: A study of cognitive load in learning. (Beijing: Science Press). 85–86.
Zhou, H., and Long, L. R. (2004). Statistical remedies for common method biases. Adv. Psychol. Sci. 12:9. doi: 10.3969/j.issn.1671-3710.2004.06.018
Keywords: conceptual level, students, validity, reliability, secondary school
Citation: Yang X, Dong J and Tan DL (2022) Student conceptual level scale: Development and initial validation. Front. Educ. 7:965643. doi: 10.3389/feduc.2022.965643
Edited by:
Christelle Declercq, Université de Reims Champagne-Ardenne, FranceReviewed by:
Jolita Dudaitė, Mykolas Romeris University, LithuaniaSalomon Huancahuire-Vega, Peruvian Union University, Peru
Copyright © 2022 Yang, Dong and Tan. This is an open-access article distributed under the terms of the Creative Commons Attribution License (CC BY). The use, distribution or reproduction in other forums is permitted, provided the original author(s) and the copyright owner(s) are credited and that the original publication in this journal is cited, in accordance with accepted academic practice. No use, distribution or reproduction is permitted which does not comply with these terms.
*Correspondence: DingLiang Tan, MDIwODhAbmpudS5lZHUuY24=