- Methods in Learning Research, University of Augsburg, Augsburg, Germany
This study tested the predictive validity of Ajzen’s theory of planned behavior with a sample of 182 non-traditional students in higher education to develop our understanding of non-traditional students’ intentions to transfer trained knowledge and skills from university courses to the workplace. After completing their courses, a survey measured behavioral, normative, and control beliefs, transfer attitudes, perceived social norms, transfer self-efficacy, and transfer intentions. One year later, a follow-up survey measured transfer of training. Partial least squares-based structural equation modeling (PLS-SEM) revealed that behavioral beliefs were positively associated with transfer attitudes, normative beliefs were positively associated with social norms, and control beliefs were positively associated with self-efficacy. Transfer attitudes and transfer self-efficacy predicted transfer intentions. Social norms, however, were non-significantly associated with intentions. Transfer intentions and transfer self-efficacy predicted transfer 1 year after training. These findings are discussed in terms of their predictive validity of Ajzen’s theory and their educational implications for non-traditional students enrolled in higher education programs.
Introduction
Non-traditional students in higher education
Non-traditional students are people who return to colleges or universities with substantial employment experiences, a completed apprenticeship, a first academic degree, or people who had to leave academia previously because of familial, economic, or cultural reasons (Gegenfurtner et al., 2018; Carreira and Lopes, 2021). Typically, non-traditional students are considered being a heterogeneous and educationally disadvantaged group of learners traditionally underrepresented in or even excluded from higher education institutions. Schuetze and Slowey (2002) argue that this group includes “older or adult students with a vocational training and work experience background, or other students with unconventional educational biographies” (p. 313). Numbers of non-traditional students at colleges and universities grow constantly (Tieben, 2020; Carreira and Lopes, 2021), so it is a timely matter to address the educational experiences of this population and explore the extent to which they use their newly trained knowledge and skills from higher education programs in their jobs. This use of training and learning content in their workplaces outside academia is generally conceptualized as a transfer of training (Gegenfurtner, 2019; Testers et al., 2019).
A number of studies in higher education identified differences between traditional and non-traditional students in terms of their motivational orientation (Francois, 2014) and subjective task value (Gorges, 2016). For example, Johnson et al. (2016) reported that non-traditional students had higher levels of interest and self-efficacy than traditional students in US colleges. Gegenfurtner et al. (2019) showed that non-traditional students had strong epistemic and developmental motives to participate in higher education programs. If it is true that non-traditional students are more interested, more intrinsically motivated, and value education more strongly than traditional students, then we would expect advantages also in terms of their training-related motivation and their intentions to transfer trained knowledge and skills to their work outside of higher education. Still, studies examining non-traditional students’ motivation and transfer are rare; without more evidence, it would be premature to conclude on their levels of transfer intentions.
Theory of planned behavior
A useful theory to examine transfer intentions is Ajzen’s (2020) theory of planned behavior. Based on the theory of reasoned action, Ajzen (1991) developed the theory as “a theory designed to predict and explain human behavior in specific contexts” (p. 181). The underlying assumption is that attitudes, social norms, and perceived behavioral control are associated with an individual’s intentions to engage in a certain behavior. The theory thus seems useful to examine (predictors of) trainees’ intentions to engage in transfer after attending a course or training program. For the purposes of the present study, transfer attitudes are understood as trainees’ positive or negative values associated with transfer of training (Jacot et al., 2018). Social norms are defined as perceived social pressures to engage or not to engage in transfer of training. Perceived behavioral control is conceptually equal to self-efficacy (Ajzen, 2020); transfer self-efficacy reflects the trainees’ perceptions associated with their ability to transfer training and take control of its application. Transfer intentions are defined as indications of trainees’ readiness to transfer training (Quesada-Pallarès, 2012; Testers et al., 2020). According to the theory of planned behavior, attitudes, social norms, and self-efficacy are associated with behavioral, normative, and control beliefs. Behavioral beliefs reflect the subjective likelihood of consequences when transferring training, such as reaching a higher work quality. Normative beliefs reveal the perceived behavioral expectations toward transfer articulated by significant others, including colleagues or friends. Control beliefs reflect a perceived presence of contextual aspects that hamper transfer, including time pressure or high workloads.
Past research has successfully used Ajzen’s theory of planned behavior to predict and explain transfer of training (Casper, 2007; Gegenfurtner et al., 2010; Quesada-Pallarès, 2012; Cheng et al., 2015; Cheng, 2016; Jacot et al., 2018). For example, Jacot et al. (2018) examined the intentions of participants in a Belgian driver rehabilitation program; trainee attitudes, perceived moral norms, and perceived behavioral control predicted their intentions not to drive again under the influence of alcohol. Cheng (2016) examined Chinese teachers who participated in faculty development programs; the findings supported the predictive validity of the theory of planned behavior, with strong positive relations between attitudes and intentions, as well as between intentions and transfer of training. Hodge et al. (2017) identified predictors of the intention to use newly trained knowledge in academic skills training courses. The present study stands on the shoulders of these previous investigations and explores the usefulness of Ajzen’s (1991, 2020) theory of planned behavior to develop our understanding of the underlying mechanisms behind non-traditional students engaging in transfer.
Aims and hypotheses
This study aimed to test the predictive validity of Ajzen’s theory of planned behavior in the context of non-traditional student training transfer in higher education. A set of three hypotheses was examined. First, we assumed that behavioral beliefs would be positively associated with transfer attitudes (Hypothesis 1a), normative beliefs would be positively associated with social norms (Hypothesis 1b), and control beliefs would be positively associated with transfer self-efficacy (Hypothesis 1c). Second, we expected that transfer intentions would be predicted by transfer attitudes (Hypothesis 2a), social norms (Hypothesis 2b), and transfer self-efficacy (Hypothesis 2c). Third, we assumed that transfer intentions (Hypothesis 3a), and transfer self-efficacy (Hypothesis 3b) would predict transfer of training.
Methods
Participants and design
Participants in the study were 182 non-traditional students (72 women, 110 men) with a mean age of 34.60 years (SD = 9.65). The non-traditional students were enrolled in courses at a large university training center that offered an elective course program with diverse topics ranging from early childhood education to supply change management. Course delivery was hybrid, including in-presence meetings, webinars, and asynchronous online learning. Participation in the training courses and in the study was voluntary. Participants completed two online surveys: a first questionnaire at the end of training and a follow-up questionnaire 1 year later.
Measures
Table 1 presents the wording of all measures. Participants responded on a 1 (strongly disagree) to 7 (strongly agree) Likert scale. At the end of training, the survey included items measuring behavioral, normative, and control beliefs, transfer attitudes, perceived social norms, transfer self-efficacy, and transfer intentions. One year later, the follow-up survey included items measuring transfer of training. These items were adapted from Gegenfurtner et al. (2010) and Ajzen (2020).
Behavioral beliefs were measured with three items on the trainees’ beliefs about the likely consequences of transferring training. An example item was: “My work performance improves when I use my knowledge and skills acquired from training.”
Normative beliefs were measured with three items on the trainees’ beliefs about their significant others’ normative expectations regarding transfer of training. An example item was: “My colleagues think I should use my knowledge and skills acquired from training.”
Control beliefs were measured with three items on the trainees’ beliefs about the presence of work environment factors that may impede transfer. An example item was: “Time pressure hampers the use of knowledge and skills acquired from training.”
Transfer attitudes were measured with three items about the extent to which trainees valued transfer of training. An example item was: “I find it positive to use knowledge and skills acquired from training.”
Social norms were measured with three items regarding the extent to which the trainees perceived their important referents’ social pressure to engage in transfer of training. An example item was: “Most people who are important to me think I should use my knowledge and skills acquired from training.”
Transfer self-efficacy was measured with three items about the extent to which the trainees perceived behavior control to transfer their training. An example item was: “I am confident that I can use my knowledge and skills acquired from training.”
Transfer intentions were measured with three items about the trainees’ readiness and willingness to transfer training. An example item was: “I intend to use my knowledge and skills acquired from training.”
Transfer of training was measured with three items about the extent to which trainees applied the trained knowledge and skills to their work tasks. An example item was: “I have used my knowledge and skills acquired from training in my job.”
Analyses
Data analysis included two steps: data screening and path modeling. First, data screening suggested that data were non-normally distributed and missing at random; missing data were thus handled with the expectation maximization imputation implemented in SPSS 28. Second, path modeling was performed using partial least squares-based structural equation modeling (PLS-SEM). PLS-SEM is robust against departures from normality in small samples (Hair et al., 2022) and was used in this study to test the hypothesized relationships among variables based on the path weighting scheme algorithm implemented in SmartPLS 3.3 (Ringle et al., 2015). As recommended in Hair et al. (2022), we estimated the psychometric properties based on three reliability coefficients and used the cut-off criteria of the average variance extracted (AV) > 0.50, composite scale reliability (CS) > 0.60, and Cronbach’s α > 0.70.
Results
Table 2 presents the scales’ psychometric properties and an interfactor correlation matrix. Reliability estimates suggest that the variance-extracted measures were ≥ 0.63, composite scale reliability was ≥ 0.83, and Cronbach’s α was ≥ 0.70; these estimates demonstrate the reliability of the measures used. The correlation matrix shows the interrelations between the variables, which were in the hypothesized direction.
Figure 1 presents the parameter estimates of the PLS path model. The results indicate that behavioral beliefs were positively associated with transfer attitudes, normative beliefs were positively associated with social norms, and control beliefs were positively associated with self-efficacy; these findings support Hypotheses 1a–c. Transfer attitudes and transfer self-efficacy predicted transfer intentions, supporting Hypotheses 2a and 2c. Social norms, however, was non-significantly associated with intentions; thus, Hypothesis 2b was rejected. Transfer intentions and transfer self-efficacy predicted transfer 1 year after training, which supported Hypotheses 3a–b.
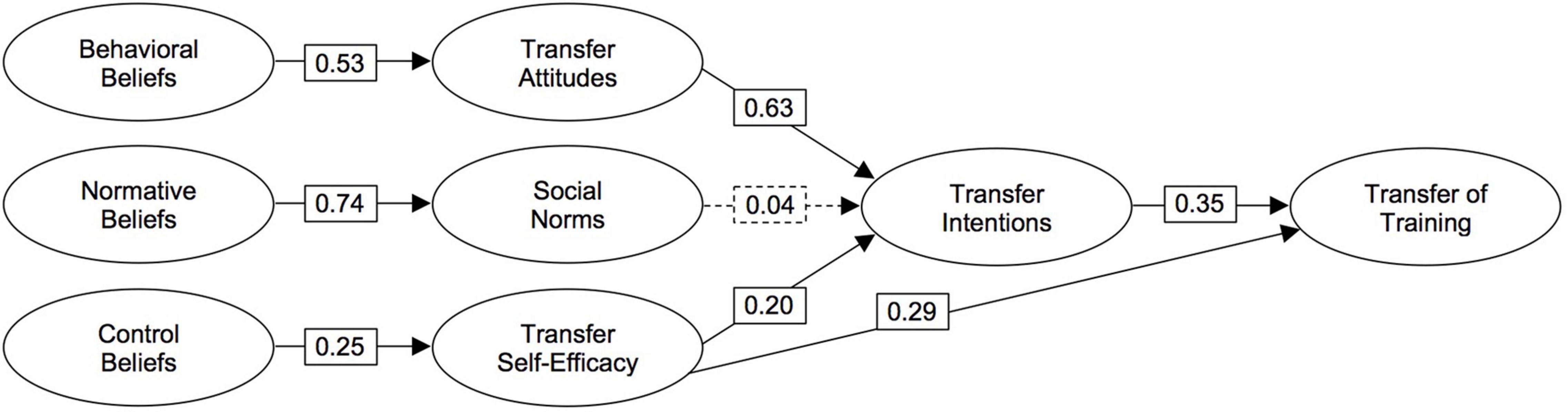
Figure 1. Parameter estimates of the PLS path model. Solid lines indicate significant estimates (p < 0.05) and the dashed line indicates a non-significant estimate (p > 0.05).
Discussion
This study aimed to test the predictive validity of Ajzen’s theory of planned behavior in explaining transfer of training. Based on academic training courses for 182 non-traditional students, the results tended to confirm the hypothesized relationships. Below, we discuss the major findings of the present study in relation to their limitations, directions for future research, and educational implications.
First, in terms of major findings, the theory of planned behavior was successfully applied to academic training courses for non-traditional students (Schuetze and Slowey, 2002; Carreira and Lopes, 2021). Transfer attitudes were most strongly associated with transfer intentions, which, in turn, were most strongly associated with transfer of training. When comparing the findings reported in the present study with previously reported evidence on the theory of planned behavior, we see that the beta coefficients in the path model correspond with results reported in a number of different training contexts and countries, including mental health practitioner training in the United States (Casper, 2007), workplace safety training in Germany (Gegenfurtner et al., 2010), education for public service workers in Spain (Quesada-Pallarès, 2012), construction workers’ and in-service teachers’ training in Hong Kong (Cheng et al., 2015; Cheng, 2016), academic skills training courses in Australia (Hodge et al., 2017), and driver rehabilitation programs in Belgium (Jacot et al., 2018). An exception, however, is the non-significant relationship between social norms and transfer intentions, which deserves more attention in future research to understand how non-traditional students perceive and cope with social pressure when attempting to apply newly trained knowledge and skills to their job tasks. Still, although this hypothesis was rejected, the weak link between social norms and intentions is not uncommon and frequently observed in empirical research (La Barbera and Ajzen, 2020).
A strength of the study was the adopted long-term perspective in measuring transfer. A majority of studies in the training literature tends to assess transfer shortly after training. If we assume that transfer needs time to unfold, with trainees needing to identify opportunities to implement what has been learned in their job routines (Testers et al., 2019), then it is useful to ask trainees not immediately after training, but after longer time intervals if they have used trained knowledge and skills at work. Such a long-term perspective has been adopted in the present study, recognizing the temporal affordances of transfer processes.
Second, in terms of limitations and directions for future research, the study used a convenience sampling approach of learners participating voluntarily in the training courses. It is possible that the influence of normative beliefs and social norms was more strongly accentuated in contexts of mandatory training programs (Jacot et al., 2018). Future research can thus aim to examine the relative influence of voluntary vs. mandatory training participation on theory of planned behavior variables. Another limitation of the present study was the single-scale approach toward measuring transfer motivation. In line with Ajzen’s (2020) model, transfer intentions were measured with a single scale, conceptualizing intention to vary in size, not in quality. As a remedy, further research can adopt a multicriterial and multicontextual approach to assess transfer intentions and transfer of training (Testers et al., 2015; Gegenfurtner and Quesada-Pallarès, 2022). Moreover, the findings reported in this study are limited to the population of non-traditional students and should not be generalized blindly to other trainee populations with different levels of workplace affordances (Brand-Gruwel et al., 2014; Hodge et al., 2017), autonomy (Siewiorek and Gegenfurtner, 2010; Cheng, 2016), or supervisor support (Quesada-Pallarès, 2012; Froehlich and Gegenfurtner, 2019; Testers et al., 2019).
Finally, the study has some educational implications that should be noted. First, trainers and educational professionals can use the scales of the present study to assess behavioral, normative, and control beliefs prior to or during an educational program as a screening instrument to assess the extent to which learners feel in control or under pressure to adopt trained knowledge and skills post training. Particularly, ratings on the control belief items can be used during training in group reflections how to overcome transfer barriers associated with time constraints or task overload. Second, the findings indicated that transfer attitudes had the strongest influence on transfer intentions. Thus, it seems advisable to promote positive attitudes and values related to training content and training application as a means to support the intentions of training participants to make use of what they have learned once the course program ends. We would argue that this should be particularly feasible for the population of non-traditional students in higher education, considering their positive motivation profiles (Francois, 2014; Gorges, 2016; Johnson et al., 2016; Gegenfurtner et al., 2018, 2019).
Data availability statement
The raw data supporting the conclusions of this article will be made available by the authors, without undue reservation.
Ethics statement
Ethical review and approval was not required for the study on human participants in accordance with the local legislation and institutional requirements. The patients/participants provided their written informed consent to participate in this study.
Author contributions
AG and LT contributed to conception, design of the study, manuscript revision, read, and approved the submitted version. AG organized the database, performed the statistical analysis, and wrote the first draft of the manuscript.
Conflict of interest
The authors declare that the research was conducted in the absence of any commercial or financial relationships that could be construed as a potential conflict of interest.
Publisher’s note
All claims expressed in this article are solely those of the authors and do not necessarily represent those of their affiliated organizations, or those of the publisher, the editors and the reviewers. Any product that may be evaluated in this article, or claim that may be made by its manufacturer, is not guaranteed or endorsed by the publisher.
References
Ajzen, I. (1991). The theory of planned behavior. Organ. Behav. Hum. Decis. Process. 50, 179–211. doi: 10.1016/0749-5978(91)90020-T
Ajzen, I. (2020). Perceived behavioral control, self-efficacy, locus of control and the theory of planned behavior. Hum. Behav. Emerg. Technol. 2, 314–324. doi: 10.1002/hbe2.195
Brand-Gruwel, S., Testers, L., and Gegenfurtner, A. (2014). “Motivation to transfer: Factors influencing transfer of learned competences to the job,” in New Technologies and the Future of Teaching and Learning, eds A. Baran et al. (Istanbul: EADTU).
Carreira, P., and Lopes, A. S. (2021). Drivers of academic pathways in higher education: traditional vs. non-traditional students. Stud. High. Educ. 46, 1340–1355. doi: 10.1080/03075079.2019.1675621
Casper, E. S. (2007). The theory of planned behavior applied to continuing education for mental health. Psychiatr. Serv. 58, 1324–1329. doi: 10.1176/appi.ps.58.10.1324
Cheng, E. W. L. (2016). Maintaining the transfer of in-service teachers’ training in the workplace. Educ. Psychol. 36, 444–460. doi: 10.1080/01443410.2015.1011608
Cheng, E. W. L., Sanders, K., and Hampson, I. (2015). An intention-based model of transfer of training. Manage. Res. Rev. 38, 908–928. doi: 10.1108/MRR-05-2014-0107
Francois, E. J. (2014). Motivational orientations of non-traditional adult students to enroll in a degree-seeking program. New Horizons Adult Educ. Hum. Resour. Dev. 26, 19–35. doi: 10.1002/nha3.20060
Froehlich, D. E., and Gegenfurtner, A. (2019). “Social support in transitioning from training to the workplace: A social network perspective,” in Beziehungen in pädagogischen Arbeitsfeldern und ihren Transitionen über die Lebensalter, ed. H. Fasching (Bad Heilbrunn: Klinkhardt), 208–222.
Gegenfurtner, A. (2019). Reconstructing goals for transfer of training in faculty development programs for higher education teachers: A qualitative documentary method approach. Heliyon 5:e02928. doi: 10.1016/j.heliyon.2019.e02928
Gegenfurtner, A., Fisch, K., and Ebner, C. (2019). Teilnahmemotivation nicht-traditionell Studierender an wissenschaftlicher Weiterbildung: Eine qualitative Inhaltsanalyse im Kontext von Blended Learning. Beitr. Hochschulforschung 41, 58–83.
Gegenfurtner, A., and Quesada-Pallarès, C. (2022). Toward a multidimensional conceptualization of motivation to transfer training: Validation of the Transfer Motivation Questionnaire from a self-determination theory perspective using bifactor-ESEM. Stud. Educ. Eval. 73:101116. doi: 10.1016/j.stueduc.2021.101116
Gegenfurtner, A., Schwab, N., and Ebner, C. (2018). “There’s no need to drive from A to B”: Exploring the lived experience of students and lecturers with digital learning in higher education. Bavar. J. Appl. Sci. 4, 310–322. doi: 10.25929/bjas.v4i1.50
Gegenfurtner, A., Vauras, M., Gruber, H., and Festner, D. (2010). “Motivation to transfer revisited,” in Learning in the disciplines, Vol. 1, eds K. Gomez, L. Lyons, and J. Radinsky (Buenos Aires: ISLS), 452–459.
Gorges, J. (2016). Why adults learn: Interpreting adults’ reasons to participate in education in terms of Eccles’ subjective task value. Int. Online J. Educ. Teach. 3, 26–41.
Hair, J. F., Hult, G. T. M., Ringle, C., and Sarstedt, M. (2022). A primer on partial least squares structural equation modeling (PLS-SEM), 3rd Edn. Thousand Oaks, CA: Sage.
Hodge, B., Wright, B., and Bennett, P. (2017). Does academic training change intentions? Drawing upon the theory of planned behaviour to improve academic performance. Int. J. Train. Res. 15, 105–118. doi: 10.1080/14480220.2016.1259004
Jacot, A., Raemdonck, I., and Frenay, M. (2018). Intra-individual differences in offenders’ motivation and behavioral change after a driver rehabilitation program. Trans. Res. Part F Traff. Psychol. Behav. 58, 302–318. doi: 10.1016/j.trf.2018.05.026
Johnson, M. L., Taasoobshirazi, G., Clark, L., Howell, L., and Breen, M. (2016). Motivations of traditional and nontraditional college students: From self-determination and attributions, to expectancy and values. J. Cont. High. Educ. 64, 3–15. doi: 10.1080/07377363.2016.1132880
La Barbera, F., and Ajzen, I. (2020). Control interactions in the theory of planned behavior: Rethinking the role of subjective norm. Eur. J. Psychol. 16, 401–417. doi: 10.5964/ejop.v16i3.2056
Quesada-Pallarès, C. (2012). Training transfer evaluation in the public administration of Catalonia. Procedia Soc. Behav. Sci. 46, 1751–1755. doi: 10.1016/j.sbspro.2012.05.372
Schuetze, H. G., and Slowey, M. (2002). Participation and exclusion: A comparative analysis of non-traditional students and lifelong learners in higher education. High. Educ. 44, 309–327. doi: 10.1023/A:1019898114335
Siewiorek, A., and Gegenfurtner, A. (2010). “Leading to win: The influence of leadership style on team performance during a computer game training,” in Learning in the disciplines, Vol. 1, eds K. Gomez, L. Lyons, and J. Radinsky (Thousand Oaks, CA: ISLS), 524–531.
Testers, L., Gegenfurtner, A., and Brand-Gruwel, S. (2015). “Motivation to transfer learning to multiple contexts,” in The school library rocks: living it, learning it, loving it, eds L. Das, S. Brand-Gruwel, K. Kok, and J. Walhout (Thousand Oaks, CA: IASL), 473–487.
Testers, L., Gegenfurtner, A., and Brand-Gruwel, S. (2020). Taking affective learning in digital education one step further: Trainees’ affective characteristics predicting multicontextual pre-training transfer intention. Front. Psychol. 11:2189. doi: 10.3389/fpsyg.2020.02189
Testers, L., Gegenfurtner, A. A., Van Geel, R., and Brand-Gruwel, S. (2019). From monocontextual to multicontextual transfer: Organizational determinants of the intention to transfer generic information literacy competences to multiple contexts. Front. Learn. Res. 7:23–42. doi: 10.14786/flr.v7i1.359
Keywords: theory of planned behavior, non-traditional students, higher education, transfer of training, PLS-SEM
Citation: Gegenfurtner A and Testers L (2022) Transfer of training among non-traditional students in higher education: Testing the theory of planned behavior. Front. Educ. 7:928996. doi: 10.3389/feduc.2022.928996
Received: 26 April 2022; Accepted: 04 July 2022;
Published: 22 July 2022.
Edited by:
William Nketsia, Western Sydney University, AustraliaReviewed by:
Maxwell Peprah Opoku, United Arab Emirates University, United Arab EmiratesAssunta Di Vaio, University of Naples Parthenope, Italy
Sahil Gupta, Chandigarh University, India
Copyright © 2022 Gegenfurtner and Testers. This is an open-access article distributed under the terms of the Creative Commons Attribution License (CC BY). The use, distribution or reproduction in other forums is permitted, provided the original author(s) and the copyright owner(s) are credited and that the original publication in this journal is cited, in accordance with accepted academic practice. No use, distribution or reproduction is permitted which does not comply with these terms.
*Correspondence: Andreas Gegenfurtner, andreas.gegenfurtner@phil.uni-augsburg.de