- Department of Educational Psychology, University of Wisconsin – Madison, Madison, WI, United States
Educational video games can engage students in authentic STEM practices, which often involve visual representations. In particular, because most interactions within video games are mediated through visual representations, video games provide opportunities for students to experience disciplinary practices with visual representations. Prior research on learning with visual representations in non-game contexts suggests that visual representations may confuse students if they lack prerequisite representational-competencies. However, it is unclear how this research applies to game environments. To address this gap, we investigated the role of representational-competencies for students’ learning from video games. We first conducted a single-case study of a high-performing undergraduate student playing an astronomy game as an assignment in an astronomy course. We found that this student had difficulties making sense of the visual representations in the game. We interpret these difficulties as indicating a lack of representational-competencies. Further, these difficulties seemed to lead to the student’s inability to relate the game experiences to the content covered in his astronomy course. A second study investigated whether interventions that have proven successful in structured learning environments to support representational-competencies would enhance students’ learning from visual representations in the video game. We randomly assigned 45 students enrolled in an undergraduate course to two conditions. Students either received representational-competency support while playing the astronomy game or they did not receive this support. Results showed no effects of representational-competency supports. This suggests that instructional designs that are effective for representational-competency supports in structured learning environments may not be effective for educational video games. We discuss implications for future research, for designers of educational games, and for educators.
Introduction
Educational video games are powerful tools that can engage students in authentic practices of STEM disciplines (Clark et al., 2009). Disciplinary practices often include the use of visual representations (visuals for short) to solve problems and communicate with others (Airey and Linder, 2009). Prior research shows that visual representations can confuse students unless they have prerequisite representational-competencies (Kozma and Russell, 2005; Ainsworth, 2006; Rau, 2017a). These competencies include the ability to make sense of how visual representations show relevant information and to fluently perceive disciplinary information in them (Rau, 2017a). Further, research on representational competencies shows that students’ learning outcomes can be enhanced if they receive instructional support for these representational competencies while interacting with visuals (Bodemer et al., 2005; Rau and Wu, 2018).
However, prior research on representational competencies focused on structured learning environments. We consider structured learning environments as those where instruction follows a specific plan, usually directed by a teacher or instructional designer (Stanescu et al., 2016). By contrast, in unstructured learning environments, learning activities are constructed by students, within the constraints of the given context (Stanescu et al., 2016). We consider video games as unstructured learning environments because students can decide on the path they take through the game (Honey and Hilton, 2011).
Structured environments are designed to purposefully engage students with visual representations in ways that encourage reflection, whereas video games aim to intuitively engage students with visual representations without requiring reflection (Virk et al., 2015). Further, in structured environments, the dominant medium for information delivery is usually text or speech, and visual representations are used to augment this information. By contrast, video games are highly visual at their core (Virk et al., 2015). Therefore, it is unclear whether prior research on structured learning environments generalizes to educational video games. Indeed, as detailed below, we found few studies that investigated the role of representational competencies for students’ learning with visual representations in video games.
To address this gap, we conducted two studies. First, we conducted a single-case study that served to ascertain whether representational competencies are an issue in learning within educational video games in the first place. To this end, we selected a high-performing student playing an astronomy video game for an undergraduate astronomy course. We expected that this student would successfully learn from visual representations within the game. We found that the student had difficulties with the visual representations that seemed to impede his learning from the game. This motivated a second study, which experimentally that tested whether representational-competency supports designed based on prior research on structured learning environments would enhance learning from the game. We found that the representational-competency supports were ineffective. Our findings yield novel insights into how representational competencies affect learning in unstructured educational contexts and have implications for the design of educational video games.
Theoretical background
In the following, we briefly review prior research on visual representations in educational video games and in structured learning environments to highlight the gap we seek to address.
Visual representations in educational video games
Defining educational video games is not straightforward, in part, because platforms differ in terms of their game-like features (de Freitas and Oliver, 2006). For the purpose of our studies, we define games as interactive experiences that model disciplinary phenomena of interest, allow students to take actions that impact aspects of the phenomena, and incorporate goals and ongoing feedback for measuring progress (Clark et al., 2009). Further, we focus on video games that are played via computers, gaming platforms (e.g., Xbox), or other digital devices (e.g., smart phones) (Gee, 2003). We also focus on educational video games that are specifically designed to achieve learning goals, as opposed to recreational games designed purely for entertainment (Belanich et al., 2009). Finally, we focus on educational video games that are systematically designed based on educational research. We discuss the specific game we chose for our studies in section 3.1.1.
By definition, video games are highly visual, and one of their strengths is that they can engage students in disciplinary practices that involve interactions with visual representations (Clark et al., 2009; Holbert and Wilensky, 2019). Many STEM disciplinary practices centrally involve the ability to use visual representations to solve problems and communicate with others both verbally and non-verbally (Airey and Linder, 2009; Rau, 2017a). Indeed, scientists in multiple disciplines use visual representations to make abstract concepts visible, predict and explain scientific phenomena, and communicate ideas within their community of practice (Kozma and Russell, 2005; Gilbert, 2008). For example, line emission spectra (see Figure 1) are used to determine the presence of compounds in celestial bodies in astronomy or to determine the identity of gases in chemistry. Hence, an important goal of educational video games is to immerse students in disciplinary practices with visual representations (Habgood and Ainsworth, 2011; Virk et al., 2015; Virk and Clark, 2019). The present studies focus on those visual representations within games that serve as disciplinary tools (e.g., line emission spectra), as opposed to visual representations that purely serve to engage students with the game environment (e.g., a spaceship) or to navigate the game (e.g., a map of the environment).

Figure 1. Example line emission spectrum of a gas. Each element emits a unique line emission spectrum, which allows astronomers and chemists to use line emission spectra to determine the presence of compounds.
While little prior research specifically focused on the role of disciplinary visual representations in educational video games, our review of the literature identified several ways in which visual representations may enhance students’ learning within games. First, we found that educational video games include visual representations that allow students to see and interact with abstract concepts within the discipline [e.g., speed of light; Kortemeyer et al., 2013]. Second, visual representations can support students’ conceptual understanding of disciplinary phenomena (e.g., DNA encoding; Corredor et al., 2014). Third, because the visual representations mimic tools used within the discipline, they serve to enculturate students into disciplinary practices (e.g., modeling physical phenomena based on data; Sengupta et al., 2015).
However, our review also identified several obstacles that may reduce the effectiveness of visual representations within educational video games. First, some games (e.g., Annetta et al., 2013) place high demands on students to learn gameplay conventions and navigation, which may impede their ability to invest cognitive effort into making sense of disciplinary visual representations. Second, encountering a multitude of visual representations within games can make it difficult for students to focus on those visual representations that depict relevant disciplinary content, which can reduce their ability to make sense of the visual representations (Lim et al., 2006). Finally, in some games, visual representations are incorporated into passive components of the game (e.g., students may view but not interact with an animation), which may reduce students’ focus on these visual representations, thereby interfering with their ability to make sense of the visual representations (Anderson and Barnett, 2013).
In sum, visual representations within games offer opportunities for students to engage with disciplinary content. However, students may have difficulties in making sense of these visual representations.
Visual representations in structured learning environments
Our review of research on visual representations in educational video games parallels findings from prior research on visual representations in structured learning environments. That is, this research shows that visual representations can enhance students’ learning because they visualize abstract concepts, allow for modeling of domain-relevant processes, and enable students to participate in disciplinary practices (Gilbert, 2008; Rau, 2017a).
This line of prior research also identified several obstacles that could impede students’ learning with visual representations, which parallel difficulties observed in the context of video games. Specifically, making sense of visual representations is cognitively demanding, especially when students are asked to make connections among multiple visual representations (Ainsworth et al., 2002; Rau, 2017a). Further, students tend to have difficulties distinguishing relevant visual features from irrelevant ones (Goldstone and Son, 2005). Finally, students tend not to make sense of visual representations unless they have to actively manipulate them (Bodemer et al., 2004).
In contrast to research on educational video games, research on learning with visual representations has investigated how to help students overcome these difficulties by supporting their representational competencies: knowledge about how visual representations show disciplinary information (Rau, 2017a). In particular, this research suggests they need support for two broad types of representational competencies—sense-making competencies and perceptual fluency (Ainsworth, 2006; Kellman and Massey, 2013; Rau, 2017a).
Sense-making competencies describe the ability to explain how visual features of a representation depict discipline-specific concepts and to explain connections between multiple visual representations based on conceptual mappings (Seufert, 2003; Ainsworth, 2006). For example, when learning about the Doppler shift, students must make sense of the visual representations in Figure 2. Specifically, this involves understanding that when a planet orbits a star (shown by the animated celestial object), the gravity of the planet causes the star’s light emissions to shift red or blue (shown by the electromagnetic spectrum) based on the movement toward or away from a point in space at a specific speed (shown by the graph) (Franknoi et al., 2017). Students acquire these sense-making competencies through sense-making processes (Ainsworth, 2006; Rau, 2017a), which involve effortful, verbal explaining of conceptual information (Chi et al., 1989; Koedinger et al., 2012).
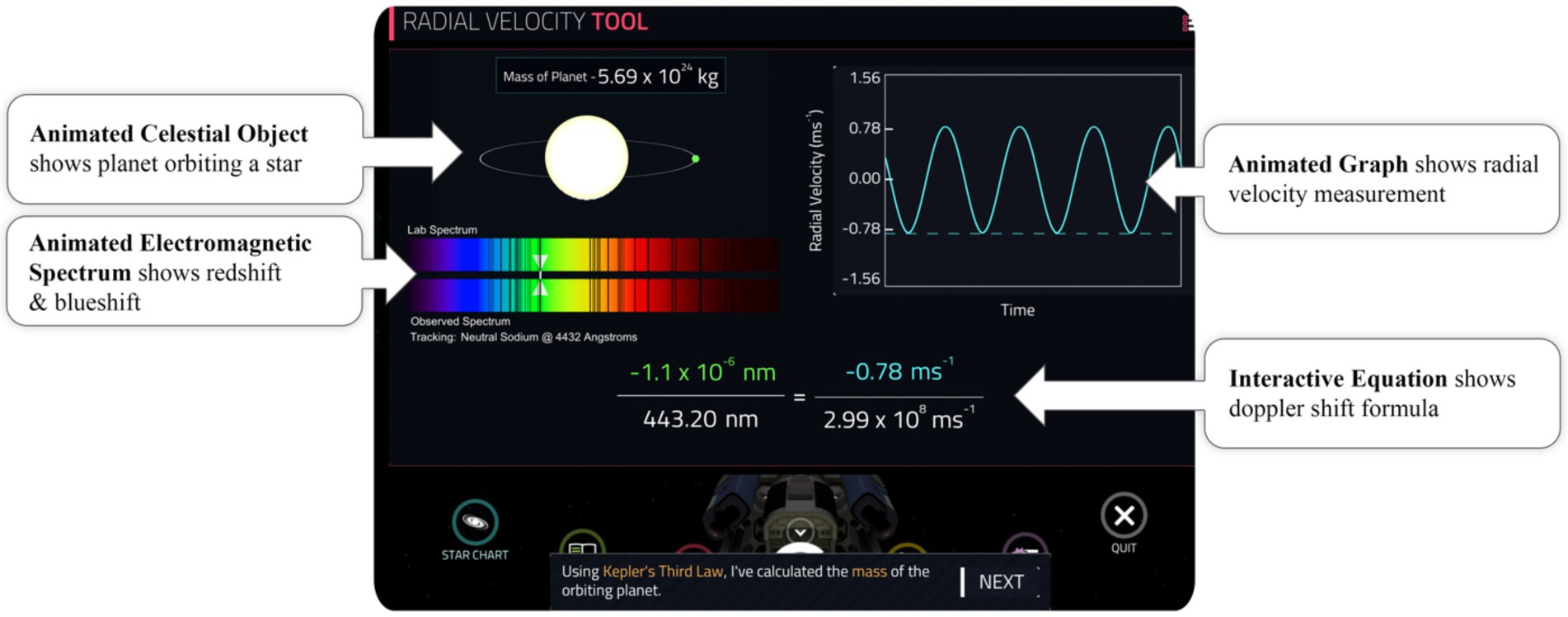
Figure 2. Example sense-making task: Students must connect the visual representations related to the Doppler shift: planet orbiting a star (top left), electromagnetic spectrum (bottom left), radial velocity graph (top right), and the corresponding equation (bottom center) to understand the concept of using doppler shift to identify planets orbiting a star. This task is presented in the Radial Velocity Tool of the game, discussed below.
This line of research developed design principles for instructional interventions that support sense-making processes (see Seufert, 2003; Bodemer et al., 2004; Koedinger et al., 2012; Rau, 2017a,b). Sense-making interventions should prompt students to explain to themselves or to someone else how visual representations show disciplinary concepts. These prompts should encourage students to actively establish mappings between the visual representations and the concepts they show. Sense-making interventions can deliver prompts in a variety of ways, for examples as self-explanation prompts (Rau, 2017b), prompts to draw (Fiorella and Mayer, 2016), or prompts to explain the visual representations to someone else (Rau et al., 2017).
Perceptual fluency describes the ability to quickly and effortlessly extract meaningful information from visual representations and to fluently translate among different representational systems (Kellman and Massey, 2013; Rau, 2017a). For instance, when working with line emission spectra, the ability to quickly see whether an observed spectrum matches a lab spectrum allows students to determine which elements are present in a given compound, as illustrated in Figure 3. Students acquire perceptual fluency through inductive pattern-recognition processes that are nonverbal (Goldstone, 1997; Gibson, 2000; Fahle and Poggio, 2002). These processes are non-verbal because they do not require verbal explanation (Kellman and Garrigan, 2009; Kellman and Massey, 2013). They are considered to be inductive because they do not require explicit instruction (Koedinger et al., 2012) but rely on recognizing patterns that appear across many examples (Gibson, 1969, 2000).
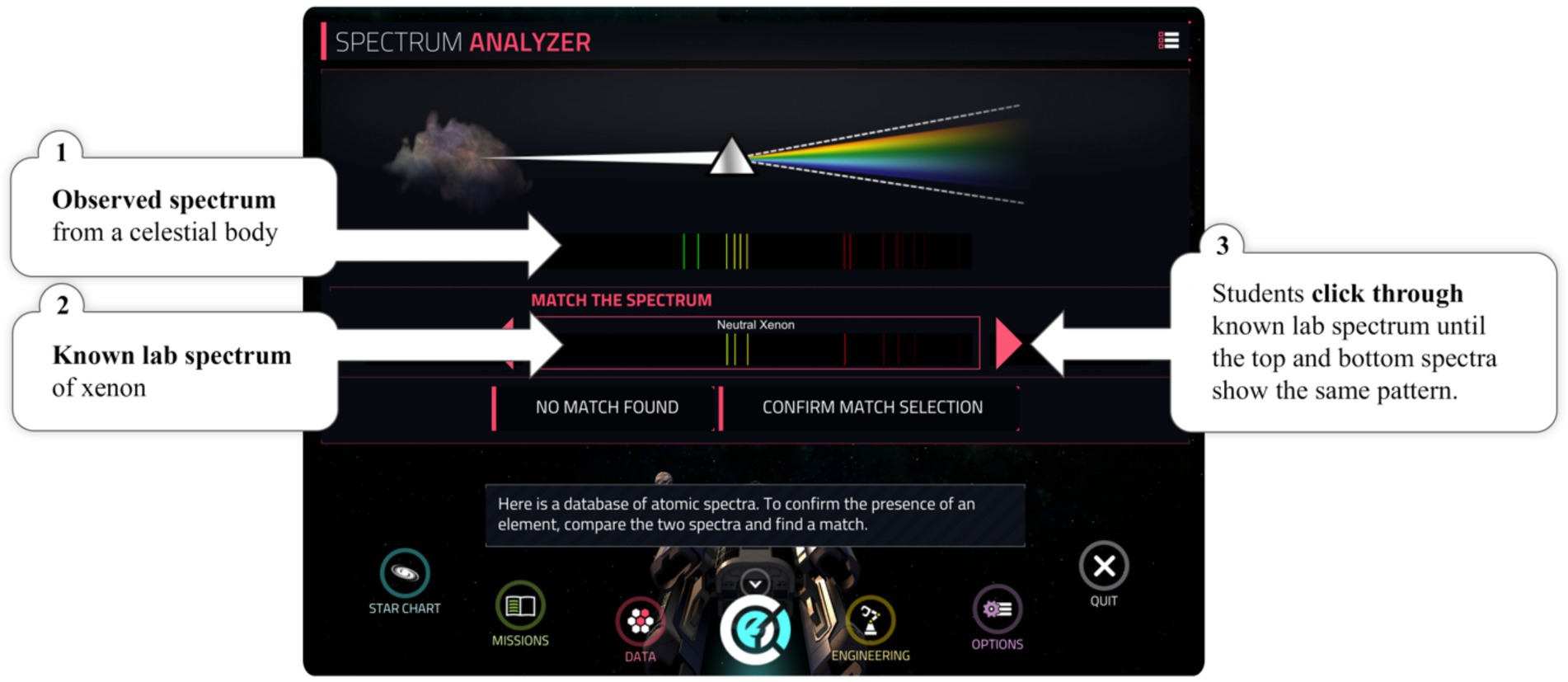
Figure 3. Example line emission spectra task: Students are asked to judge whether the observed spectrum (top) matches a lab spectrum (bottom). In this case, the student should see that the two spectra match. This task was presented in the Spectrum Analyzer Tool of the game, discussed below.
This line of research has developed instructional design principles for interventions that support perceptual-induction processes (see Kellman et al., 2008, 2010; Rau, 2017a). Perceptual-fluency interventions expose students to a large variety of visual representations and ask students to quickly judge or classify the visual representations based on the information they show. The visual representations should be sequenced in ways that contrast relevant visual features while varying irrelevant visual features. Throughout, students should be prompted to solve the tasks quickly without explaining their answers because explanations can interfere with perceptual processing (Schooler et al., 1997). Similarly, feedback should be provided on the accuracy of student answers but without explanations, so as not to disrupt perceptual processing. Perceptual-fluency interventions can prompt perceptual processing in a variety of ways, for example, by asking students to categorize systematically varied examples (Rau, 2017b) or to rapidly construct disciplinary visual representations (Eastwood, 2013).
Prior research shows that combining these two types of instructional support for sense-making and perceptual-fluency competencies enhances students’ learning of disciplinary knowledge from structured learning environments (Rau et al., 2017; Rau and Wu, 2018). Further, perceptual-fluency interventions are usually offered after students have acquired sense-making competencies (Kellman et al., 2008, 2010), and this sequence was found to be more effective in experimental studies (Rau, 2018).
Study 1: Single-case study
Our brief literature review suggests that the difficulties students encounter with visual representations in educational video games are similar to those they encounter in structured learning environments. While this suggests that a lack of representational competencies could be a stumbling block in the context of educational video games, research on video games has not focused on representational competencies specifically. To close this gap, we conducted a single-case study to explore:
Research question 1 (RQ1): How does an undergraduate student use visual representations in an educational video game?
The single-case study methodology allowed us to take a holistic approach to gain deep understanding of one student’s entire learning experience over multiple weeks within an undergraduate course. The goal of the single-case study was to ascertain whether representational competencies might pose an issue in learning within educational video games. We considered this goal as a prerequisite for investigating whether support for representational competencies may enhance students’ learning from an educational video game (see Study 2).
Methods
Video game context: At play in the cosmos
We chose a game for our study that has the explicit goal to familiarize students with multiple visual representations of astronomical phenomena: At Play in the Cosmos (Squire, 2021). The game is a single-player adventure designed to engage students in applying introductory astronomy concepts. Specifically, the game design targeted students with non-STEM majors with no prior astronomy experience to align with those students who typically took introductory astronomy courses at the undergraduate level (Gear Learning, 2017). In the game, students take on the role of an intergalactic explorer and contractor for a corporation that mines resources in space. They captain their own spaceship and are aided by the on-board Cosmic Operations Research Interface who acts as their guide during 22 scripted missions. In each mission, students travel through space using an interactive star chart (see Figure 4) to complete challenges. In doing so, they use real astronomical data to observe and measure real astrophysical objects such as galaxies and nebulas. The first three missions of the game serve as a tutorial which introduces students to basic gameplay mechanics, the user interface including a subset of seven tools available in the game, and introductory physics concepts. After the first three missions, students can play the remaining missions in any order, however, the game presents an unfolding narrative to rescue the galaxy from the Corporation if played in linear order. In addition to the scripted missions, the game includes a sandbox mode where students can freely explore galaxies and instructors can design activities using the objects and tools within the game.
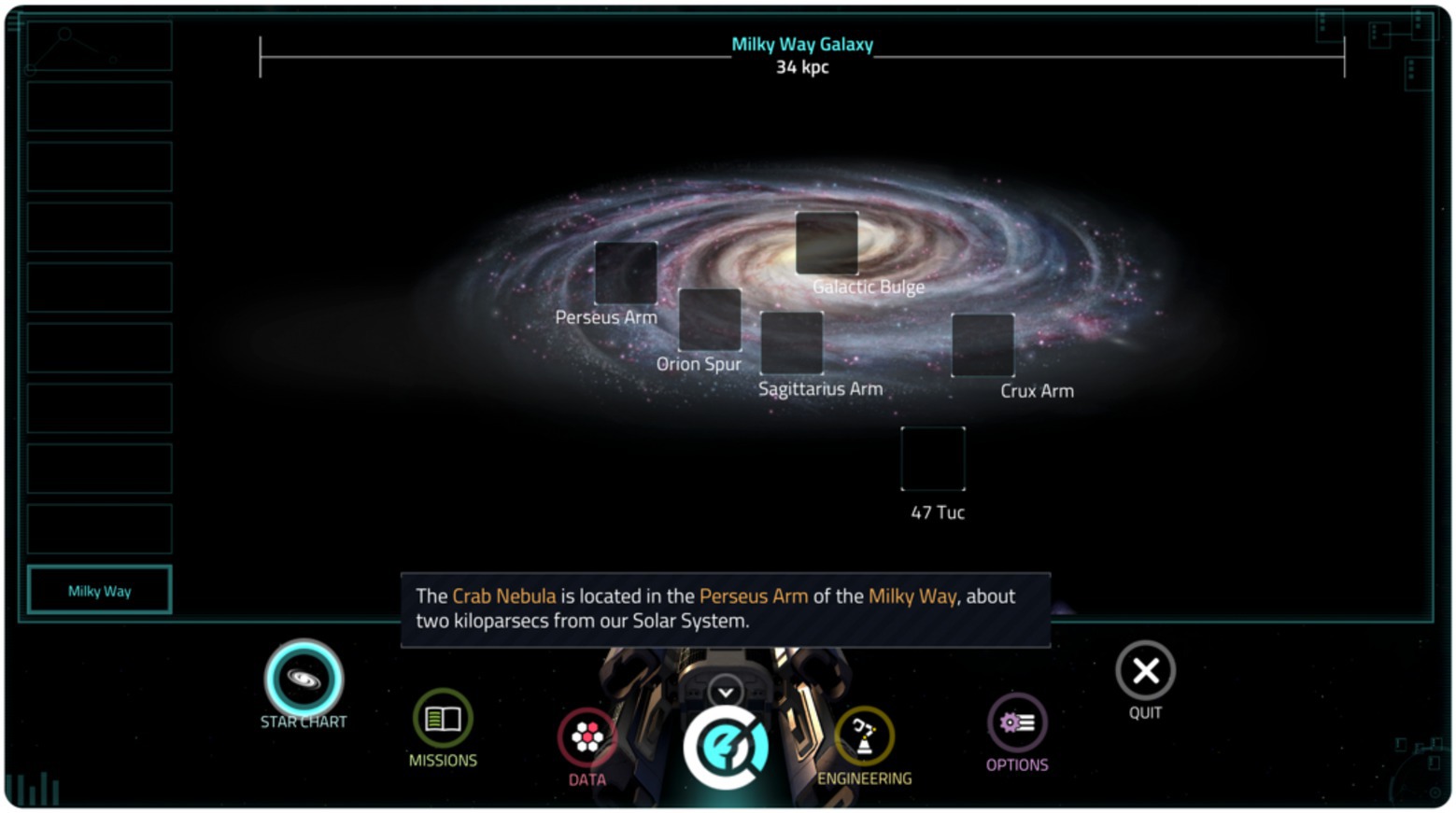
Figure 4. Interactive Star Chart. Students use the in-game Star Chart to navigate to various locations in the galaxy and access tools to observe and measure data from celestial objects. The game designers purposely created the star chart as “slices” of the galaxy to enable students to understand the scale of the universe while designing within the constraints of the gaming engine.
One major goal of the game is for students to learn how and why astronomers use visual representations as tools for understanding the universe and its evolution (e.g., line emission spectra, see Figure 1). Hence, students engage with visual representations as part of the tools they use to gather information and resources (Squire, 2021). In using the visual representations, students learn about concepts related to measuring celestial objects (e.g., Doppler shift, see Figure 2). While most of the interactions within the game focus on the visual representations, it also aims at helping students connect visual representations to equations that describe the same concepts (see Figure 2).
The game offers several visual representations that serve as tools for problem solving within the game because they parallel the scientific process that astronomers use in the field. The electromagnetic spectrum (Figure 1) is an important tool in multiple disciplines, such as astronomy and chemistry. Electromagnetic spectra represent the electromagnetic radiation emitted or absorbed by objects, such as visible light and ultraviolet radiation (Franknoi et al., 2017). Electromagnetic spectra play an important role in many subject areas. For example, astronomy students use electromagnetic spectra when learning to identify critical properties of celestial bodies (Bardar et al., 2005); and chemistry students use electromagnetic spectra when learning about Planck’s quantum theory (Moore and Stanitski, 2015). Within the game, multiple tools expose students to electromagnetic spectra including: the Radial Velocity Tool (Figure 2) and the Spectrum Analyzer Tool (Figure 3). Students use the Radial Velocity Tool to determine the presence of planets orbiting stars. For instance, students use the tool to identify a habitable planet within the Milky Way galaxy. Students use the Spectrum Analyzer to match observed spectra to laboratory spectra to determine the properties of celestial objects. For instance, students travel to Supernova 1987A to determine if sodium gas is present in its ejecta (Bary, 2017).
The game was designed as a collaboration between the University of Wisconsin-Madison and W.W. Norton & Company. The design team included multiple astronomy subject matter experts, educators, artists, and game designers (Squire, 2021). The design team used an iterative development process that included multiple rounds of playtesting with students. They also conducted a formal beta-test pilot study across five universities with 440 students) (Dalsen, 2017) and an alpha-test pilot study with professors at two universities with 184 students (Squire, 2021). Both pilot studies showed positive perceptions of the game and its contribution to learning astronomy concepts.
Study context and participant
We conducted the single-case study as part of an eight-week online introductory astronomy course at our institution. The course was titled “The Evolving Universe: Stars, Galaxies, and Cosmology” and covered the importance of light in astronomy for understanding the past, present, and future of the universe. The instructor chose At Play in the Cosmos to provide students with an immersive experience into the topics covered in the course. In particular, the game was intended to provide experience in how astronomers use tools to work with the light emitted by celestial objects to identify their composition and structure. It was also intended to provide students with a sense of what the various celestial objects look like and how simple mathematical equations serve to calculate critical information about these celestial objects. Students in this course used the game for six homework assignments. Students played through the scripted missions in the game in the first four weeks of class. Then, they used the sandbox mode to complete activities designed by the instructor in the next two weeks of the course. The instructor of the course considered the game an add-on to the course that was relatively independent of other course materials. Specifically, the instructor did not ask students to reflect on their experiences in the game and did not provide information on how the game aligned with astronomy practices.
For our study, we selected one student, Simon (pseudonym). The instructor considered him a high performing student based on his performance on weekly short papers, weekly quizzes, and a long-term project within the class. The instructor noted that Simon’s written assignments were very thorough and demonstrated conceptual understanding of the astronomy content addressed in the class. Further, he had extensive gaming experience. Simon started playing games when he was 3 years old and played on multiple platforms including GameCube, GameBoy, DS, Xbox, and PC. He also had prior experience with educational video games especially in Math where he played Cool Math (2018). Simon was majoring in political science and Chinese. He enrolled in the course to fulfill a physical science prerequisite for his majors. Although he had no previous formal experience with astronomy, he followed NASA and SpaceX on social media and watched space related content on YouTube. Simon was an appropriate selection because he fit the target audience for the game given that he had no previous formal experience with astronomy and given that he was a non-STEM major (Gear Learning, 2017). Further, based on his performance on the assignments within the course, we expected him to be successful in learning with the visual representations in the game. On the flipside, we reasoned that if Simon has difficulties learning with visual representations in the game, we could expect lower-performing students to experience difficulties as well.
Data collection and analysis
We conducted seven semi-structure interviews. The goal of the interviews were to understand Simon’s successes and challenges while playing the game as well as how he used the visual representations related to the electromagnetic spectrum to gain conceptual understanding of the spectra and how they are used within astronomy. The interview questions were designed to encourage Simon to reflect on his experiences in the game and to explain how he interacted with the visual representations within the game to better understand astronomy concepts. Further, the interview questions aimed to elicit explanations of concepts related to the electromagnetic spectrum outside the context of the specific experiences Simon had in the game.
The first interview was conducted one week before he started playing the game to gain some general insights into his prior experiences with astronomy, visual representations, and video games. The remaining six interviews occurred after each game assignment in Weeks 1–4, 6, and 8. Each of the six interviews followed the same semi-structured procedure. Specifically, to start the interview, we asked Simon some general questions about when he completed the game in the preceding week, whether he played it in one or multiple sessions, and about successes and difficulties in playing the game. We then asked about the specific visualization tools he encountered in his game play, what role the visual tool played in learning within the game, how he learned to interact with the visual tool, and in what scenarios an astronomer might use the visual tool. We asked follow-up questions depending on how the student responded to the questions, asking him to say more about the question or to elaborate on specific parts of the answer. Example questions from the interview protocols are included in section data collection and analysis Supplementary Appendix S1.
All interviews were transcribed. We analyzed the data using grounded theory (Glaser and Strauss, 1967). That is, we qualitatively reviewed the interview data to identify key themes that characterized Simon’s experiences with the visual representations in the game tools. To establish inter-subjectivity, we then formalized the recurring themes into a coding scheme. Shaffer (2017) argues that social moderation is the best strategy for very small data sets. Because our transcripts comprised only 541 lines of data, we followed this recommendation. Thus, two independent coders applied the coding scheme to the data and used social moderation until there was agreement on each code (Herrenkohl and Cornelius, 2013).
Results
Our grounded analysis revealed two major themes. First, Simon engaged with the visual representations at a superficial level. For example, when asked about the Spectrum Analyzer Tool (see Figure 3) in Week 2, he focused on the operations used to manipulate the visual representation rather than the conceptual interpretation of the visual representation: “if there was a gas cloud, you could shine the spectrum analyzer and shine beams and see if anything was reflected or see what colors come out,” and when prompted to elaborate: “you can determine the composition, you know, the composition of anything, I guess like a cloud or something like that,” and “I mean, you just look and look what matches.” In addition to Simon’s focus on operations, these excerpts illustrate that he did not actually understand the visual representation’s role as an astronomy tools (because the Spectrum Analyzer Tool captures light; it does not shine light). Similarly, in Week 3, when asked about the Radial Velocity Tool (see Figure 2), he said: “I could figure out […] if there was a planet in front of a star or not by measuring it over time.” When asked to elaborate, he said: “it shows radial velocity going up and down.” When proved further if he was confused about any aspects of the visual representation, he stated: “I mean, yeah, there's a lot of stuff on the screen.” These examples illustrate that Simon described the operations used to manipulate them (e.g., “you could shine the spectrum analyzer”), described superficial visual features (e.g., the graph “going up and down”) and engaged in pattern recognition (e.g., “you just look and look what matches). He did not engage with the visual representations at a conceptual level; that is, he did not reflect on the meaning of these features nor any connections to other visual representations that were present at the same time. Across the interviews, this superficial processing of the visual representations and a resulting lack of sense-making seemed to be a recurring theme.
Second, Simon had difficulties understanding the disciplinary practices with visual representations that were portrayed in the game, which was the learning goal of the game. When asked about how the game portrayed what astronomers do, he said, “I mean, sure, it was more sci-fi type like, obviously you’re not in space doing all that stuff.” He also said: “I don’t really know if it's realistic or not because I don’t know how scientists go about doing all that stuff.” When probed further about specific ideas that may be more realistic, he said: “It all felt the same. I guess where we were just doing calculations of the star during the first part was more realistic than the back half of the game where it just went completely sci-fi.” When probed further to relate what he said to the visual representations in the game, he said, “the [electromagnetic spectra] probably don’t differ, but maybe how they get them probably differs from the way the spaceship uses them.” Simon never went beyond focusing on the spaceship in explaining which aspects of the game reflect realistic astronomy practices from game.
When asked about the concept underlying the in-game tool, however, he stated: “well, that’s more important to me because the electromagnetic spectrum allows [astronomers] to see different things in space like using a higher wavelength of light, they can see deeper into the milky way galaxy, so it allows them to see different things and observe different things that would be of importance to whatever they would want to do for research.” This statement indicated that Simon was able to articulate the importance of the concept underlying the game, that the electromagnetic spectrum can be used to analyze light, but he did not connect this concept to the tools used in the game nor their real-world equivalents. Across the interviews, we observed a recurring emphasis on surface-level differences between the game and real-world astronomy and a lack of a deep understanding of which of the portrayed astronomy practices around visual representations were realistic.
Discussion
Overall, our single-case study revealed that Simon engaged with the visual representations at a relatively superficial level. We did not find evidence that he attempted to make sense of how the visual representations showed astronomy concepts or that he reflected on which aspects of the visual representations reflected realistic disciplinary practices. Simon’s lack of sense-making of the game visual representations aligns with findings from prior research on educational video games and on structured learning environments that students often have difficulties in making sense of visual representations spontaneously (e.g., Ainsworth et al., 2002; Anderson and Barnett, 2013). We also found that Simon had difficulties understanding how the visual representations within the game correspond to disciplinary tools within astronomy. Because this was the learning goal of the game, this finding suggests that Simon did not benefit from the game as intended by the instructor or the game designers (Squire, 2021).
A plausible explanation for these findings is that the design of the game aligns more with the design of perceptual-fluency supports than with the design of sense-making supports. Specifically, recall that prior research suggests that effective sense-making supports prompt students to explain relations between visual representations and the concepts they show (e.g., Seufert, 2003; Bodemer et al., 2004; Koedinger et al., 2012). While students interact with the visual representations in the game, they do not receive prompts to explain how the visual representations show astronomy concepts or how various visual representations relate to one another. Likewise, when students made mistakes, the game did not offer reflective feedback. In contrast, the design of the game seems more aligned with the instructional design principles for perceptual-fluency supports, which target non-verbal, inductive learning processes of pattern recognition (e.g., Kellman et al., 2008, 2010; Rau, 2017a). Specifically, the game asks students to identify visual patterns and provides only correctness feedback. In line with this interpretation, Simon’s interview responses indicate that he engaged in some level of perceptual processing of the visual representations based on pattern recognition.
Further, it seems plausible that Simon’s issues in understanding the role of the game visual representations as disciplinary visual representations results from his lack of sense-making of the visual representations. If Simon did not make sense of how the visual representations in the game depict astronomy concepts, he may not have been able to understand why these visual representations are tools for disciplinary practices in astronomy. In other words, if Simon did not understand the conceptual ideas underlying the visual representations in the game, he may not have been able to connect those ideas to the broader disciplinary context outside of the game. This issue may be particularly relevant in the context of video games that provide a fictional narrative as a context of the game, which could obscure the authenticity of the visual representations in the game. While the game seemed to support some level of perceptual fluency by virtue of engaging Simon in pattern recognition, prior research suggests that this may be suboptimal: perceptual-fluency support has been shown to be ineffective if students did not previously receive sense-making support because it can give students a false sense of familiarity with visual representations they do not conceptually understand (e.g., Rau, 2018).
In sum, the single-case study indicates that a high-performing student had difficulties making sense of visual representations within the game, which seemed to reduce his understanding of the visual representations as disciplinary tools.
Study 2: Class experiment
The single-case study suggests that a lack of sense-making support within the game could pose an issue to students’ benefit from the game. This leads to the question whether providing instructional support for representational competencies (i.e., sense-making competencies and perceptual fluency) would improve students’ benefit from the game. Specifically, we ask:
RQ2: Does the game lead to pretest-to-posttest learning gains of sense-making competencies (RQ2a), of perceptual fluency (RQ2b), and astronomy content knowledge (RQ2c)?
RQ3: Does support for representational competencies enhance undergraduate students’ benefit from the game with respect to their learning of sense-making competencies (RQ3a), of perceptual fluency (RQ3b), and astronomy content knowledge (RQ3c)?
Further, we explored:
RQ4: How do undergraduate students experience the visual representations within the game?
We addressed these questions with an experiment that compared how representational-competency supports affect learning within the game.
Methods
Participants
We recruited 45 undergraduates from an introductory chemistry course at our institution—a large midwestern university. The course had no formal prerequisites but was advertised to students in beginning or intermediate level chemistry courses. Of the 45 students, three students had prior astronomy courses, ten had prior astronomy lessons in another science course, 16 had prior informal experiences with astronomy such as planetarium visits or social media, 15 students had no experience at all, and one student did not complete the survey. 40 of the students had at least one STEM major, one student had a non-STEM major, three students were undecided, and one student did not complete the survey. 29 students rarely or never played video games, twelve students played sometimes, and three students played very often. Thus, although the student majors did not align with the target population of the game (the game was designed primarily for non-STEM majors as explained above), the students’ prior gaming experiences aligned with the target population of game. Further, the instructor was willing to implement multiple conditions within the course, so we could test our research questions.
A portion of the same game from the case study was used for two required homework assignments. Students played ten missions of approximately 70 minutes of gameplay. The instructor used the game as an illustration of how electromagnetic spectra, which students encountered earlier in the semester, are used in related disciplines.
Experimental design
Students were randomly assigned to one of two conditions. The control condition received no support for representational competencies while playing the game. Because prior research on representational competencies shows that the combination of support for sense-making competencies and perceptual fluency improve disciplinary learning outcomes, the support condition received support for sense-making competencies and support for perceptual fluency at separate times while playing the game. Support was designed based on prior research on structured learning environments described above.
Specifically, sense-making support was given in the form of prompts, displayed on the computer screen prior to playing a set of five missions of the game (see Figure 5). Students were able to revisit this screen at any time, but whether and how often they did was not recorded. The prompts outlined how to engage with the visual representations in a three-step process. First, students were asked to interpret each visual representation separately. Second, they were asked to compare pairs of visual representations. Third, they were asked to explain the comparison in reference to astronomy concepts. For example, as shown in Figure 5, when students were about to work with the Spectrum Analyzer Tool, the prompt would first ask students to interpret what each electromagnetic spectrum showed, second to compare similarities and differences of two electromagnetic spectra, and third to explain what the comparison indicates about the presence of a substance in a star.
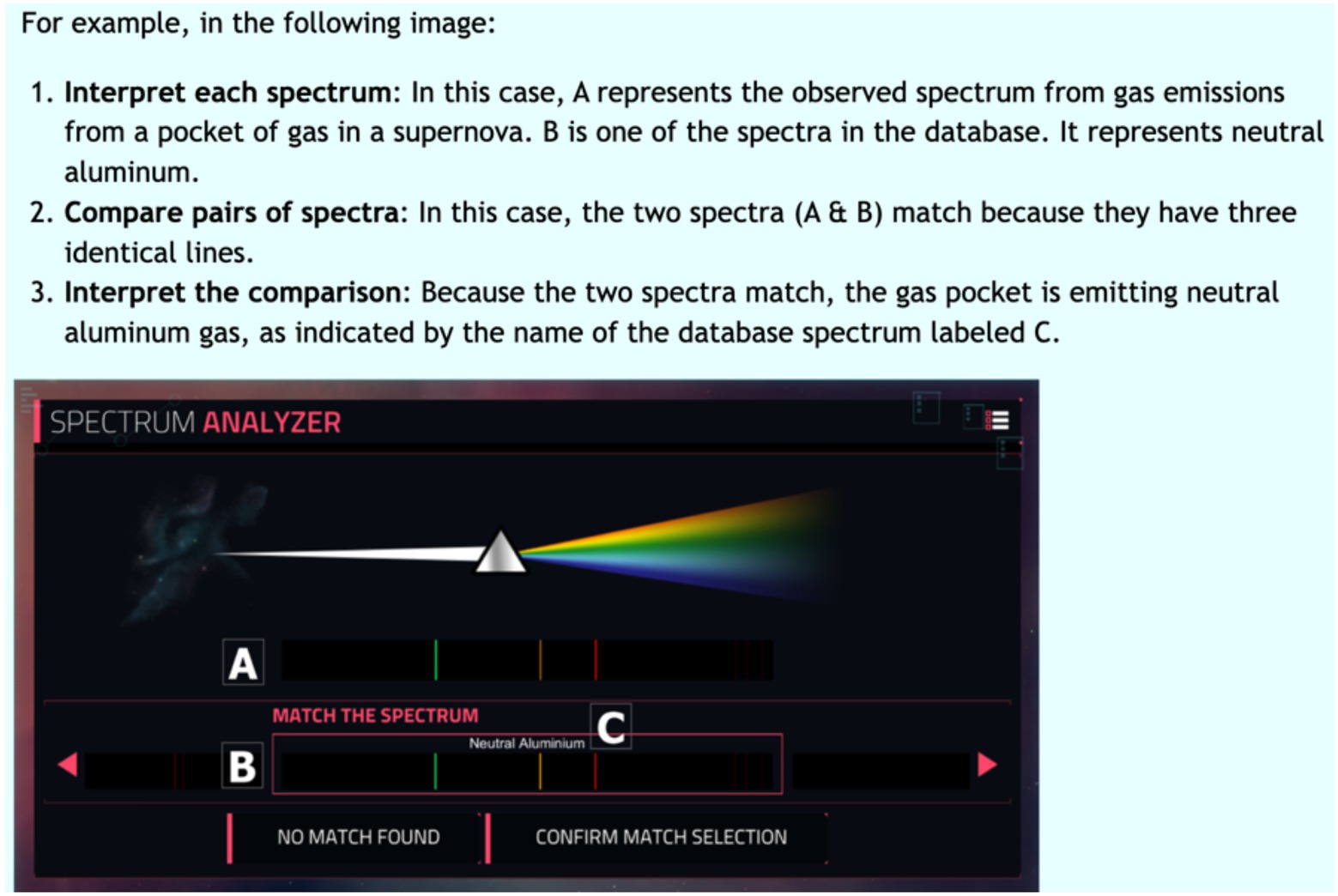
Figure 5. Example sense-making support: Students received a prompt that asked students to interpret each spectrum, compare pairs of spectra, and interpret the comparison, with a concrete example (in this case, with the Spectrum Analyzer within Mission 1).
Perceptual-fluency support was also delivered via prompts, displayed on the screen prior to playing a set of five missions of the game (Figure 6). Students were able to revisit this screen at any time, but whether and how often they did was not recorded. The prompts instructed students to train their ability to use the visual representations quickly to make decisions within the game. To this end, students were asked not to overthink their interactions with the visual representations, to focus on speed, rely on their intuitions about the match, and to accept mistakes.
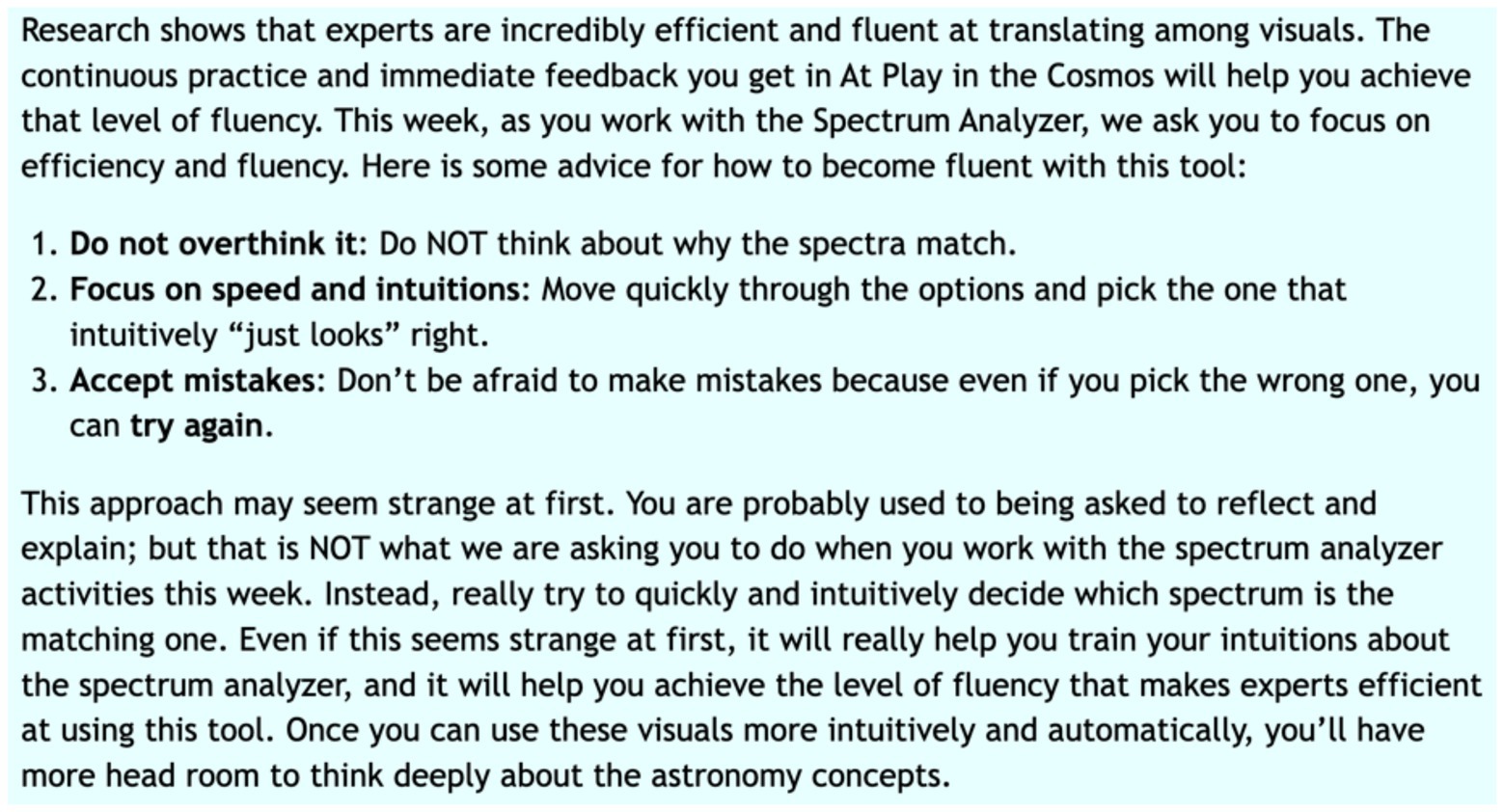
Figure 6. Example perceptual-fluency support: Students received a prompt that asked students to not overthink it, focus on speed and intuitions, and accept mistakes, with a concrete example (in this case, with the Spectrum Analyzer).
In line with prior research (Rau, 2018), sense-making support was given during the first homework assignment with the game, and perceptual-fluency support during the second homework assignment with the game.
Measures
To address RQs 2a and 3a, we assessed students’ sense-making competencies. We created three isomorphic tests (i.e., with questions that were structurally identical but asked about different example celestial objects or compounds). The sense-making test included four questions with three parts each, yielding twelve items each worth one point for a total of twelve possible points. Each question provided two electromagnetic spectra and asked students whether they matched, why or why not they matched, what they could infer from the match, and how they went about determining the match. Open-response items were graded based on a codebook that described the sense-making competencies assessed by each item and captured multiple ways of describing how the given visual representation depicts astronomy concepts. Inter-rater reliability was established by two independent raters on 20% percent of the data and revealed a kappa over.700 on each code. Prior to statistical analysis, we investigated the internal reliability of the scale using Cronbach’s Alpha with results indicating questionable internal reliability of a = 0.671. Step-wise item analysis indicated that removing two questions would increase the internal reliability resulting in a ten-point scale with acceptable internal reliability of a = 0.702. We computed the average of the correct items for each student for each test time. The test was administered as a pretest, an intermediate posttest given after students had finished the first homework assignment with the game, and a posttest given after students had finished the second homework assignment with the game. The order of the test versions was counterbalanced across participants (e.g., some students received test version A as the pretest, B as the intermediate test, and C as the posttest, whereas the sequence for other students was A-C-B, etc.).
To address RQs 2b and 3b, we assessed students’ perceptual fluency with three isomorphic test versions that were counterbalanced across the three test times. The perceptual-fluency test included three items that showed an electromagnetic spectrum and asked students to select the best match out of four other electromagnetic spectra. Each correct question was worth one point for a total of three possible points. Cronbach’s Alpha indicated poor internal reliability of a = 0.270, however, due to the short scale, no items were eliminated. We computed the average of correct items for each student for each test time.
To address RQs 2c and 3c, we assessed students’ content knowledge with three isomorphic test versions that were counterbalanced across the three test times. The content test included two multiple-choice items and eight open-ended items, which asked students to solve problems with the visual representations they encountered in the game. One item was worth two points while the rest of the items were worth one point for a total of nine points. Open-response items were graded based on a codebook that described the conceptual knowledge assessed by each item and captured multiple ways of describing the targeted concept. Inter-rater reliability was established by two independent raters on 20% percent of the data and revealed substantial agreement with kappa over.700 on each code. Cronbach’s Alpha indicated a poor internal reliability of a = 0.295. Step-wise individual item analysis indicated that the internal reliability would increase with the removal of the two multiple-choice items resulting in poor internal reliability of a = 0.384. The final resulting scale was six-items with a possible total of seven points. We computed the average of the correct items for a content outcome score for each student for each test time.
To address RQ4, we made use of weekly reflection papers that students had to write for the course. In the course, students had to submit several reflection papers over the course of the semester, and they were typically allowed to choose which topics to reflect on. However, for the purpose of this study, we asked students specifically to reflect on what they did or did not understand about the visual representations in the game and what role the visual representations played in their learning from the game. Since the study span multiple homework assignments, students had multiple opportunities to write reflection papers, and several students chose to reflect on the video game multiple times. Consequently, the number of reflection papers for each student ranged from one to three papers. We included all reflection papers that regarded the game in our analyses. We started our analysis with the broad idea of confusion and completed an iterative open-coding process until clear themes emerged related to students’ (1) general impressions of the game, (2) indications of difficulties with visual representations, and (3) game critiques. We defined difficulties as expressions of confusion, uncertainty or difficulty understanding, or a lack of learning related to a concept or visual representations within the game. We defined game critiques as explanations about reasons why the game did not support their learning or led to confusion or struggle. Together, our analysis of these aspects was intended to capture students’ internal struggles related to the content and visual representations and their external attribution of those struggles to the game. Once the codebook was finalized, each reflection paper was coded for each instance students expressed each code. Inter-rater reliability was established by two independent raters coding twenty percent of the reflection papers and revealed substantial agreement with kappa over.700 on each code.
Results
In the following analyses, we report d for effect sizes. According to Cohen (1988), an effect size d of 0.20 corresponds to a small effect, 0.50 to a medium effect, and 0.80 to a large effect. We excluded two participants because their scores on the content knowledge test were statistical outliers. This resulted in a final sample of N = 43. Table 1 shows the means and standard deviations by condition and measure.
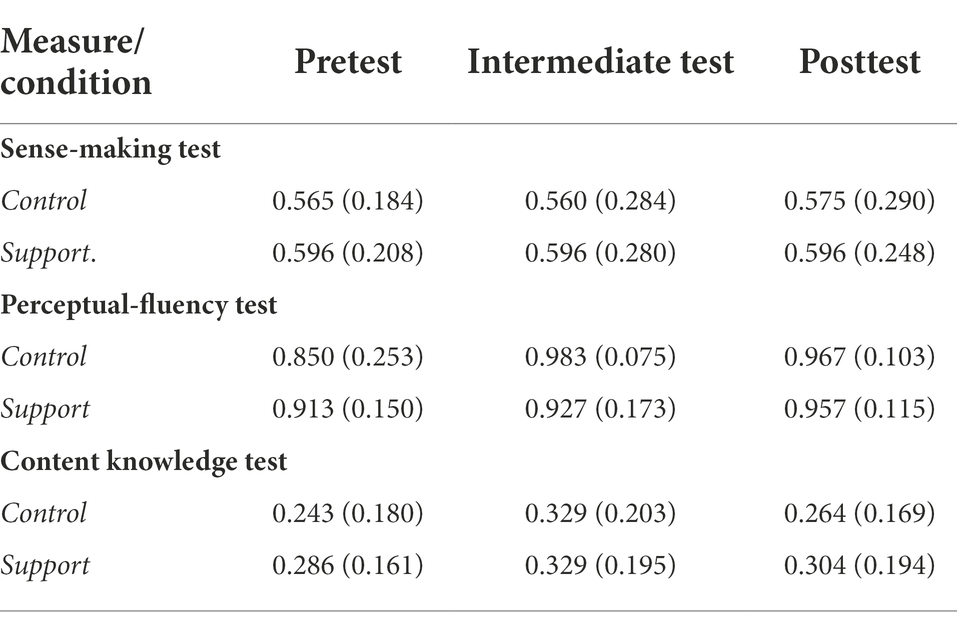
Table 1. Means (standard errors) for each test by measure, condition, and test time. All measures are on a scale from 0 to 1.
Prior checks
We checked for differences between conditions on the pretests. A MANOVA found no significant differences between conditions on the sense-making pretest (F < 1), perceptual-fluency pretest, F(1,42) = 1.020, p = 0.318, or the content knowledge pretest (F < 1).
Pretest-to-posttest learning gains
To address RQ2, we used repeated measures ANOVAs with test-time (pretest, intermediate test, post-test) as the repeated, within-subjects factor and test scores as dependent measures. The ANOVA model for RQ2a found no significant gains of sense-making competencies (F < 1). The ANOVA model for RQ2b found significant learning gains of perceptual fluency, F(2,41) = 3.188, p = 0.046, d = 0.276. The ANOVA model for RQ2c found no significant gains of content knowledge (F < 1).
Effects on representational-competency support on learning outcomes
To address RQ3, we used repeated measures ANCOVAs with condition as the between-subjects factor, test-time (intermediate test, posttest) as the repeated, within-subjects factor, pretest scores as the covariate, and scores on the intermediate test and the posttest as dependent measures. The ANCOVA model for RQ3a that tested the effect of condition on sense-making competencies revealed no significant effect (F < 1). The ANCOVA model for RQ3b that tested the effect of condition on perceptual fluency found no significant effect, F(1,42) = 1.796, p = 0.188. The ANCOVA model for RQ3c that tested the effect of condition for content knowledge found no significant effect (F < 1).
To explore whether the null effects were related to students’ prior content knowledge or prior representational competencies, we added an interaction of pretest with condition to the original ANCOVA models. There were no significant interactions between condition and pretest for sense-making competencies, perceptual fluency, nor content knowledge (F < 1).
Students’ experiences of visual representations within the game
To address RQ4, we conducted a qualitative analysis of students’ reflection papers. We identified several themes related to students’ general impressions, difficulties with visual representations, and game critiques.
With respect to general impressions of the game, we identified two major themes. First, students expressed liking the game because it was enjoyable (e.g., “have fun and learn at the same time” and “more fun to learning about chemistry”). Second, they suggested that the game was a helpful learning experience (e.g., “it [the game] taught me about different types of line spectra and what they mean”) that was relevant to the chemistry course (e.g., “it was interesting to learn how light can be used to analyze chemicals,” and “it made me realize how our base understandings of chemistry can be applied to something so grand and daunting as analyzing absorption spectra in stars”).
With respect to difficulties with visual representations in the game, we identified two major themes. First, students mentioned being distracted by visual components of the game that related to the game narrative as opposed to the learning content (e.g., “there’s just too much […] I’m not sure what’s important” and “flying through planets is […] visually stimulating [… but] completely irrelevant”). Second, students mentioned being confused about specific visual representations, such as the electromagnetic spectrum (e.g., “I’m not sure I could replicate them [the visual representations] on my own” or “I don’t remember the exact procedure or purpose”). This confusion ranged from not understanding why celestial bodies emit spectra (e.g., “I still don’t feel like I understand why a star or planet emits a certain type of spectrum.”) to not gaining a deeper understanding of electromagnetic spectra from the game (e.g., “I don’t feel like I deepened my understanding of electromagnetic spectra or its importance in the study of outer space.”
With respect to critiques of the game, we identified two major themes. First, students mentioned that it was “too easy to click through activities” without “having to learn what we’re doing or why we did it” and without having to think deeply about the visual representations within the game. For instance, students mentioned they would just click through the electromagnetic spectra without being engaged in learning when, why, and how to use them (e.g., “it was a lot of clicking and guessing for me” and “all I’m doing is matching up the colors and locations of [lines]”). Second, students mentioned that there was not enough explanation and feedback in the game in general (e.g., “I really like the illustrations of stars, nebulas, and other cosmic objects, but without a better explanation of them, just a simple one would do, I’m not really getting as much understanding as I could.”) or related to specific visual representations within the game (e.g., the game lacked a definition of “what the energy/light spectr[a] really are” or once a student had found two matching spectra, she was wondering “now what”).
We also noted that none of the students’ reflection papers mentioned the prompts delivered as part of our intervention in the support condition.
Discussion
Building on the results from Study 1, we investigated whether representational-competency supports would enhance students’ benefit from the visual representations in At Play in the Cosmos. To this end, we compared students playing the game without support to students playing the game with sense-making and perceptual-fluency supports, which were delivered in the form of prompts. In response to RQ2, results showed significant pretest-to-posttest learning gains only for perceptual fluency—a type of representational competency that allows students to quickly and effortlessly use information shown by the visual representations. However, we found no evidence of pretest-to-posttest learning gains that students learned content knowledge or sense-making competencies from the game. Further, in response to RQ3, we found no evidence that representational-competency support enhanced students’ learning of sense-making competencies, perceptual fluency, or content knowledge. Finally, in response to RQ4, the results suggest that students had difficulties focusing on the conceptual aspects of the visual representations because they were distracted by other visual elements of the game, and that they struggled to understand the concepts depicted by the visual representations. Students attributed these difficulties to the game encouraging them to click through visual features without providing conceptual explanations or feedback on how the visual representations depict concepts.
The pattern of results regarding learning gains is in line with our interpretation of the single-case study that the game design aligns with design principles of perceptual-fluency supports by engaging students in inductive pattern-matching processes. Indeed, students’ comments in the reflection papers noted that they could easily click through the game without deeply considering the visual representations and concepts they depicted. This might explain the lack of learning of sense-making competencies. The game’s focus on inductive pattern-recognition processes might have interfered with effortful, verbal explaining of conceptual information that characterizes sense-making processes. Students’ comments in the reflection papers speak to this interpretation when they attributed their difficulties in understanding the visual representations to lack of definitions, explanations, and feedback during gameplay – the features that prior research has shown to support sense-making processes (Seufert, 2003; Bodemer et al., 2005).
With respect to RQ3, we see two possible explanations why our representational-competency supports were ineffective at supporting sense-making competencies. First, it is possible that the sense-making support was ineffective because it may have been incompatible with the use of the visual representations in the game. The visual elements of the game engaged students in more intuitive interactions and seemed to have detracted students from thinking deliberately about how they interacted with the visual representations or why. Students’ comments from the reflection papers that the game involved a lot of clicking, guessing, and matching speaks to this interpretation. Hence, when the sense-making support prompted students to deliberately think and reflect on relations between the visual representations and the content, the game’s pull toward intuitive interactions may have detracted from the sense-making processes that the sense-making support intended to engage students in. Second, a related explanation is that students may have intended to make sense of the visual representations but did not receive sufficient information from the game to do so effectively. Students’ comments about the game lacking explanations and feedback is in line with this interpretation. Third, it is possible that delivering the sense-making support via prompts on screens that were displayed in between missions in the game (rather than integrated during game play) rendered them ineffective. Students may have needed additional reminders to engage in sense-making processes during game play.
We see four possible explanations why our representational-competency supports were ineffective at supporting perceptual fluency. First, it is possible that because the game incorporated design principles for perceptual-fluency supports, it already supported perceptual fluency for all students, maximizing the game’s capacity of promoting perceptual fluency. Therefore, the additional perceptual-fluency support that prompted students to engage in inductive pattern-recognition processes may not have had any additional effectiveness. The finding that students in all conditions showed pretest-to-posttest gains in perceptual fluency supports this interpretation. Second, it is possible that the method of delivering the perceptual-fluency support outside the game was not maximally effective, as discussed above. Third, it is possible that without any gains in sense-making competencies, perceptual-fluency support cannot fall on fruitful ground: to benefit from perceptual-fluency support, students need to first understand the meaning of the visual representations (Rau, 2018). Finally, the perceptual-fluency test was comprised of three items and had low reliability given the lack of variance in students’ scores. Although we saw learning gains across the three test times, it is possible that we were unable to find differences across the conditions due to a ceiling effect.
Given that representational-competency support failed to support sense-making competencies and perceptual fluency, it is not surprising that it failed to enhance students’ learning of content knowledge. If, as indicated by prior research both sense-making competencies and perceptual fluency are necessary for students’ learning of content knowledge from visual representations (Rau, 2017a), then the lack of students’ learning of sense-making competencies may explain their lack of learning of content knowledge. It seems that a focus on perceptual fluency with the visual representations by itself is not sufficient to enable students to learn content from the visual representations. Unless students can make sense of the visual representations, they may not understand the underlying concepts. However, our content measure had poor reliability due to the majority of the students’ inability to correctly answer the questions. Thus, it is possible that this lack of learning gains as well as lack of differences across conditions results from measurement error. Indeed, in a follow-up study with revised measures, we saw content learning gains as a result of interacting with the game paired with representational-competency supports (Herder and Rau, 2022).
Taken together, the main finding from our research is that the game did not support students’ learning, even though students found it enjoyable and saw its merit in the context of the course. Our results show that the game supported students’ acquisition of perceptual fluency but fell short of supporting their sense-making competencies. Students’ reflection papers indicate that their difficulties in making sense of the game visual representations impeded their learning of the underlying concepts.
General discussion
We set out to investigate whether a lack of representational competencies might be an issue in students’ learning from educational video games that are highly visual by definition. Our single-case study showed that even a high performing student had difficulties making sense of visual representations in a game that seemed to primarily encourage inductive pattern-recognition processes that lead to perceptual fluency with visual representations. We reasoned that supporting students’ representational competencies via prompts might alleviate these issues. A class experiment revealed similar issues as the single-case study, showing that the game supported perceptual fluency but not sense-making competencies. Further, we found that the representational-competency supports were ineffective. Qualitative analyses lend credibility to our interpretation of both the single-case study and the class experiment that a lack of sense-making competencies accounts for students’ lack of benefits from the game.
Altogether, these findings suggest that sense-making competencies that enable students to map visual features of visual representations to concepts are an important component of learning with visual representations in games. This finding parallels prior research on structured learning environments that has established the importance of sense-making competencies for learning with visual representations (Ainsworth, 2006; Rau, 2017a). Further, our findings suggest that perceptual fluency alone is not sufficient at supporting students’ learning with visual representations within games, again paralleling prior research on structured learning environments (Kellman et al., 2008; Rau, 2017a). Contrasting with prior research on structured learning environments, our findings indicate that delivering representational-competency supports in the form of prompts may not be effective in the context of games. It is possible that due to their more immersive nature, video games require more integrated ways of supporting representational competencies during game play. Thus, future research should examine alternative implementations of representational-competency supports. Further, it is possible that games that are designed in ways that align more with one type of representational competencies (in our case, perceptual fluency) cannot be tweaked to support a different representational competency (in our case, sense-making competencies). In this case, it might be advisable to support a given representational competency through other means (e.g., in our case, supporting sense-making competencies via a reflective problem-solving activity before students play the game).
Limitations
Our findings must be interpreted in light of the following limitations. First, the single-case study took place within the broader context of an astronomy course, so we were unable to distinguish between aspects of the student’s learning that were due to the game and aspects that were due to other experiences within the course. While Simon provided information about what he thought he was learning from the game versus the rest of the course, we did not have any measures that may have disambiguated his actual learning from the game from the rest of the course. Future research could address this limitation by adding tests immediately after game play, as was done in our class experiment.
Second, the single-case study was meant to serve as an initial exploration of the themes related to learning with visual representations in the game. Despite the focus on one participant, Simon was an interesting candidate because he was a high-performing student in the class and had extensive video game experience. We reasoned that if even he had difficulties with the visual representations in the game, then other lower-performing students may exhibit similar if not more difficulties. However, we cannot make claims about unstudied students based on the single-case study. Thus, future research could investigate cases of lower-performing students learning with visual representations in games.
Third, the class experiment had a relatively small sample size. It is possible that the representational-competency supports had effects that were smaller than what was detectible. Thus, future research should replicate our findings with larger sample sizes.
Fourth, the class experiment was our first attempt at creating measures to assess the constructs of perceptual fluency, sense-making competencies, and astronomy content within the context of an educational video game. Our content measure and perceptual-fluency measures, in particular, had poor reliability. Further, our perceptual-fluency measure was comprised of only three items. Despite showing learning gains, we may have encountered a ceiling effect that may have contributed to the lack of differences across conditions. To address this limitation, future research should use improved measures.
Fifth, across our studies, we focused on a particular game, At Play in the Cosmos. As any game, it incorporates specific disciplinary visual representations in specific ways to support students’ game play and learning. In particular, throughout this article, we repeatedly emphasize the game’s alignment with perceptual-fluency supports. While we think this is not unusual because many games aim to engage students intuitively through visual means, we readily acknowledge that other games may incorporate design features that are more likely to engage students in reflective, conceptual thinking that encourages sense-making processes. Therefore, future research should investigate representational competencies in the context of a larger variety of educational games.
Conclusion
Even though educational video games are highly visual, we are not aware of research that has investigated the role of representational competencies for students’ learning with visual representations in games. The present article takes a first step toward addressing this gap. We found that a lack of a specific type of representational competency, namely students’ difficulties in making sense of visual representations within the game, seemed to impede their learning of content knowledge from the game. While we acknowledge that a lack of sense-making competencies may not be the only issue that accounts for the students’ failure to learn content knowledge from the game, we consider sense making of visual representations a prerequisite to their learning from the game because the purpose of the game was to familiarize students with the use of visual representations for disciplinary problem solving. At the very least, our findings suggest that representational competencies may be an important ingredient to students’ learning within educational video games that warrants further research.
Data availability statement
The datasets presented in this article are not readily available because of IRB restrictions. Requests to access the datasets should be directed to corresponding author.
Ethics statement
The studies involving human participants were reviewed and approved by University of Wisconsin—Madison IRB 2018-0662 and 2018-1131. The patients/participants provided their written informed consent to participate in this study.
Author contributions
TH conducted the studies and analyses as well as most of the writing activities. MR served as the academic advisor to TH and oversaw all study activities and writing activities. All authors contributed to the article and approved the submitted version.
Conflict of interest
The authors declare that the research was conducted in the absence of any commercial or financial relationships that could be construed as a potential conflict of interest.
Funding
This work was supported by the Institute of Education Sciences, United States Department of Education, under Grant [#R305B150003] to the University of Wisconsin-Madison. Opinions expressed are those of the authors and do not represent views of the United States Department of Education.
Supplementary material
The Supplementary material for this article can be found online at: https://www.frontiersin.org/articles/10.3389/feduc.2022.919645/full#supplementary-material
References
Ainsworth, S. (2006). DeFT: A conceptual framework for considering learning with multiple representations. Learn. Instruct. 16, 183–198. doi: 10.1016/j.learninstruc.2006.03.001
Ainsworth, S., Bibby, P., and Wood, D. (2002). Examining the effects of different multiple representational systems in learning primary mathematics. J. Learn. Sci. 11, 25–61. doi: 10.1207/S15327809JLS1101_2
Airey, J., and Linder, C. (2009). A disciplinary discourse perspective on university science learning: Achieving fluency in a critical constellation of modes. J. Res. Sci. Teach. 46, 27–49. doi: 10.1002/tea.20265
Anderson, J. L., and Barnett, M. (2013). Learning physics with digital game simulations in middle school science. J. Sci. Educ. Technol. 22, 914–926.
Annetta, L., Frazier, W., Folta, E., Holmes, S., Lamb, R., and Cheng, M. (2013). Science teacher efficacy and extrinsic factors toward professional development using video games in a design-based research mode. J. Sci. Educ. Technol. 22, 47–61.
Bardar, E. M., Prather, E. E., Brecher, K., and Slater, T. F. (2005). The need for a light and spectroscopy concept inventory for assessing innovations in introductory astronomy survey courses. Astron. Educ. Rev. 4. doi: 10.3847/aer2005018
Bary, J. (2017). Game Instructor’s Manual for at Play in the Cosmos: The Videogame. New York, NY: WW Norton & Company.
Belanich, J., Orvis, K. A., Horn, D. B., and Solberg, J. L. (2009). “Bridging game development and instructional design,” in Handbook of Research on Effective Electronic Gaming in Education. ed. R. E. Ferdig (Hershey, PA: IGI Global), 1088–1103.
Bodemer, D., Ploetzner, R., Bruchmüller, K., and Häcker, S. (2005). Supporting learning with interactive multimedia through active integration of representations. Inst. Sci. 33, 73–95. doi: 10.1007/s11251-004-7685-z
Bodemer, D., Ploetzner, R., Feuerlein, I., and Spada, H. (2004). The active integration of information during learning with dynamic and interactive visualizations. Learn. Inst. 14, 325–341. doi: 10.1016/j.learninstruc.2004.06.006
Chi, M. T., Bassok, M., Lewis, M. W., Reimann, P., and Glaser, R. (1989). Self-explanations: How students study and use examples in learning to solve problems. Cogn. Sci. 13, 145–182. doi: 10.1016/0364-0213(89)90002-5
Clark, D. B., Nelson, B., Sengupta, P., and D’Angelo, C. (2009). Rethinking science learning through digital games and simulations: genres, examples, and evidence. Paper commissioned for the National Research Council Workshop on Gaming and Simulations., October 6-7, Washington, DC.
Cohen, J. (1988). Statistical Power Analysis for the Behavioral Sciences. 2nd Edn. New York, NY: Lawrence Erlbaum Associates.
Cool Math (2018). Coolmath games. Available at: https://www.coolmathgames.com/
Corredor, J., Gaydos, M., and Squire, K. (2014). Seeing change in time: Video games to teach about temporal change in scientific phenomena. J. Sci. Educ. Technol. 23, 324–343. doi: 10.1007/s10956-013-9466-4
Dalsen, J. (2017). “Classroom implementation of a games-based activity on astronomy in higher education,” in Proceedings of the ACM Conference on Foundations of Digital Games eds. A. Canossa, C. Harteveld, J. Zhu, M. Sicart, and S. Deterding New York, NY: Association for Computing Machinery.
de Freitas, S., and Oliver, M. (2006). How can exploratory learning with games and simulations within the curriculum be most effectively evaluated? Com. Educ. 46, 249–264.
Eastwood, M. L. (2013). Fastest Fingers: A molecule-building game for teaching organic chemistry. J. Chem. Educ. 90, 1038–1041. doi: 10.1021/ed3004462
Fiorella, L., and Mayer, R. E. (2016). Eight ways to promote generative learning. Educ. Psychol. Rev. 28, 717–741. doi: 10.1007/s10648-015-9348-9
Franknoi, A., Morrison, D., and Wolff, S. C. (2017). Astronomy. OpenStax. https://openstax.org/details/books/astronomy
Gear Learning (2017). At play in the cosmos. Available at: https://gearlearning.org/at-play-in-the-cosmos/
Gee, J. P. (2003). What Video Games Have to Teach Us About Learning and Literacy. New York, NY: Palgrave Macmillan.
Gibson, E. J. (1969). Principles of Perceptual Learning and Development. Englewood Cliffs, New Jersey: Prentice Hall.
Gibson, E. J. (2000). Perceptual learning in development: Some basic concepts. Ecol. Psychol. 12, 295–302. doi: 10.1207/S15326969ECO1204_04
Gilbert, J. K. (2008). “Visualization: An emergent field of practice and inquiry in science education,” in Visualization: Theory and Practice in Science Education. eds. J. K. Gilbert, M. Reiner, and M. B. Nakhleh, vol. 3 (Springer), 3–24.
Glaser, B. G., and Strauss, A. L. (1967). The Discovery of Grounded Theory: Strategies for Qualitative Research. Chicago, Illinois: Aldine.
Goldstone, R. L., and Son, J. (2005). The transfer of scientific principles using concrete and idealized simulations. J. Learn. Sci. 14, 69–110. doi: 10.1207/s15327809jls1401_4
Habgood, M. J., and Ainsworth, S. E. (2011). Motivating children to learn effectively: Exploring the value of intrinsic integration in educational games. J. Learn. Sci. 20, 169–206. doi: 10.1080/10508406.2010.508029
Herder, T., and Rau, M. A. (2022). Representational-competency supports in an educational video game for undergraduate astronomy. Comp. Educ. 190, 1–13. doi: 10.1016/j.compedu.2022.104602
Herrenkohl, L. R., and Cornelius, L. (2013). Investigating elementary students' scientific and historical argumentation. J. Learn. Sci. 22, 413–461. doi: 10.1080/10508406.2013.799475
Holbert, N., and Wilensky, U. (2019). Designing educational video games to be objects-to-think-with. J. Learn. Sci. 28, 32–72. doi: 10.1080/10508406.2018.1487302
Honey, M. A., and Hilton, M. L. (2011). Learning Science Through Computer Games and Simulations Washington, DC: National Academy Press.
Kellman, P. J., and Garrigan, P. B. (2009). Perceptual learning and human expertise. Phys. Life Rev. 6, 53–84. doi: 10.1016/j.plrev.2008.12.001
Kellman, P. J., and Massey, C. M. (2013). “Perceptual learning, cognition, and expertise,” in The Psychology of Learning and Motivation. ed. B. H. Ross, vol. 558 (Cambridge, Massachusetts: Elsevier Academic Press), 117–165.
Kellman, P. J., Massey, C. M., Roth, Z., Burke, T., Zucker, J., Saw, A., et al. (2008). Perceptual learning and the technology of expertise: Studies in fraction learning and algebra. Prag. Cogn. 16, 356–405. doi: 10.1075/pc.16.2.07kel
Kellman, P. J., Massey, C. M., and Son, J. Y. (2010). Perceptual learning modules in mathematics: Enhancing students’ pattern recognition, structure extraction, and fluency. Top. Cogn. Sci. 2, 285–305. doi: 10.1111/j.1756-8765.2009.01053.x
Koedinger, K. R., Corbett, A. T., and Perfetti, C. (2012). The knowledge-learning-instruction Framework: Bridging the science-practice chasm to enhance robust student learning. Cogn. Sci. 36, 757–798. doi: 10.1111/j.1551-6709.2012.01245.x
Kortemeyer, G., Tan, P., and Schirra, S. (2013). “A Slower Speed of Light: Developing intuition about special relativity with games,” in International Conference on the Foundations of Digital Games (Chania, Crete, Greece: Foundation of Digital Games), 400–402.
Kozma, R., and Russell, J. (2005). “Students becoming chemists: Developing representational competence,” in Visualization in Science Education. ed. J. Gilbert (Dordrecht, Netherlands: Springer), 121–145.
Lim, C. P., Nonis, D., and Hedberg, J. (2006). Gaming in a 3D multiuser virtual environment: Engaging students in science lessons. Br. J. Educ. Technol. 37, 211–231. doi: 10.1111/j.1467-8535.2006.00531.x
Moore, J. W., and Stanitski, C. L. (2015). Chemistry: The Molecular Science. 5th Edn. Mason, Ohio: Cengage Learning.
Rau, M. A. (2017a). Conditions for the effectiveness of multiple visual representations in enhancing STEM learning. Educ. Psychol. Rev. 29, 717–761. doi: 10.1007/s10648-016-9365-3
Rau, M. A. (2017b). A framework for discipline-specific grounding of educational technologies with multiple visual representations. IEEE Trans. Learn. Technol. 10, 290–305. doi: 10.1109/TLT.2016.2623303
Rau, M. A. (2018). Sequencing support for sense making and perceptual induction of connections among multiple visual representations. J. Educ. Psychol. 110, 811–833. doi: 10.1037/edu0000229
Rau, M. A., Aleven, V., and Rummel, N. (2017). Supporting students in making sense of connections and in becoming perceptually fluent in making connections among multiple graphical representations. J. Educ. Psychol. 109, 355–373. doi: 10.1037/edu0000145
Rau, M. A., Bowman, H. E., and Moore, J. W. (2017). Intelligent technology-support for collaborative connection-making among multiple visual representations in chemistry. Comp. Educ. 109, 38–55. doi: 10.1016/j.compedu.2017.02.006
Rau, M. A., and Wu, S. P. W. (2018). Support for sense-making processes and inductive processes in connection-making among multiple visual representations. Cogn. Inst. 36, 361–395. doi: 10.1080/07370008.2018.1494179
Schooler, J. W., Fiore, S., and Brandimonte, M. (1997). At a loss from words: Verbal overshadowing of perceptual memories. Psychol. Learn. Motiv. 37, 291–340. doi: 10.1016/S0079-7421(08)60505-8
Sengupta, P., Krinks, K. D., and Clark, D. B. (2015). Learning to deflect: Conceptual change in physics during digital game play. J. Learn. Sci. 24, 638–674.
Seufert, T. (2003). Supporting coherence formation in learning from multiple representations. Learn. Inst. 13, 227–237. doi: 10.1016/S0959-4752(02)00022-1
Squire, K. (2021). At Play in the Cosmos. Int. J. Des. Learn. 12, 1–15. doi: 10.14434/ijdl.v12i1.31262
Stanescu, I. A., Stefan, A., and Baalsrud Hauge, J. M. (2016). “Using gamification mechanisms and digital games in structured and unstructured learning contexts,” in International Conference on Entertainment Computing (Vienna, Austria: Springer), 3–14.
Virk, S. S., and Clark, D. B. (2019). “Signaling in disciplinarily-integrated games: Challenges in integrating proven cognitive scaffolds within game mechanics to promote representational competence,” in Contemporary Technologies in Education. eds. O. Adesope and A. Rub (London, United Kingdom: Palgrave Macmillan), 67–95.
Keywords: representational competencies, sense-making, perceptual fluency, educational video games, unstructured learning environments, astronomy
Citation: Herder T and Rau MA (2022) The role of representational competencies for students’ learning from an educational video game for astronomy. Front. Educ. 7:919645. doi: 10.3389/feduc.2022.919645
Edited by:
Vassilia Hatzinikita, Hellenic Open University, GreeceReviewed by:
Konstantinos Ravanis, University of Patras, GreeceShaaron Ainsworth, University of Nottingham, United Kingdom
Copyright © 2022 Herder and Rau. This is an open-access article distributed under the terms of the Creative Commons Attribution License (CC BY). The use, distribution or reproduction in other forums is permitted, provided the original author(s) and the copyright owner(s) are credited and that the original publication in this journal is cited, in accordance with accepted academic practice. No use, distribution or reproduction is permitted which does not comply with these terms.
*Correspondence: Tiffany Herder, therder@wisc.edu