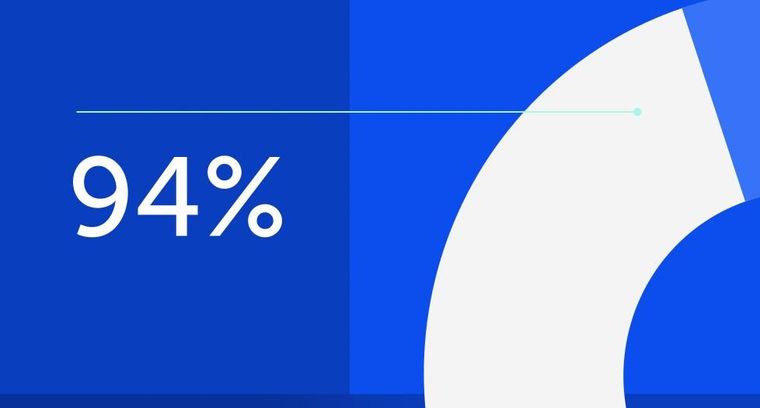
94% of researchers rate our articles as excellent or good
Learn more about the work of our research integrity team to safeguard the quality of each article we publish.
Find out more
ORIGINAL RESEARCH article
Front. Educ., 10 June 2022
Sec. Digital Learning Innovations
Volume 7 - 2022 | https://doi.org/10.3389/feduc.2022.903572
This article is part of the Research TopicDigital Learning Innovations in Education in Response to the COVID-19 PandemicView all 17 articles
The COVID-19 epidemic has become a significant global obstacle as it has impacted people's lives in various sectors, including social, economic, and education. To respond to the shock caused to education systems, massive efforts—such as conducting formal education through online classes—have been made. This study has employed Structural Equation Modeling (SEM) to examine this arena during the COVID-19 pandemic and has elaborated on how effectively the education system responded, especially through online lecturing. The Technology Acceptance Model (TAM) was implemented as this study's theoretical framework. Partial Least Squares Structural Equation Modeling was employed to measure and assess the proposed model. This study was conducted through a survey with 112 student participants in a postgraduate program between January and December 2021. The findings showed that (1) the TAM-based proposed variables have been successfully explained during the pandemic through factors predicting the use by an online class of postgraduate students, (2) significant effects were reported from perceived ease of use and perceived usefulness toward actual system use through behavioral intentions to use, (3) there were no significant results to show an indirect effect from perceived ease of use and perceived usefulness toward actual system use through behavioral intentions to use.
The WHO declared the COVID-19 outbreak to be a pandemic on March 11, 2020. COVID-19 is a monumental problem affecting more than 200 countries, including Indonesia (WHO, 2021), which has been exposed to the COVID-19 virus since it was first detected on March 2, 2020. COVID-19 has also interrupted the education process, affecting approximately 1.6 billion students from more than 190 countries. To date, nearly 178 million cases have been detected (WHO, 2021). Ensuring learning continuity while schools are closed has become a priority for governments worldwide. Many education authorities are adopting Information and Communication Technology (ICT) and asking teachers to switch to online education. However, there is no clear evidence that school closures have been effective in controlling the spread of the virus (Isfeld-Kiely and Moghadas, 2014). While online learning in higher education is usually implemented through recorded lectures and online platforms, some universities have been warned that both students and faculty members lack IT infrastructure. Some programs have been successfully implemented online, while others have not, leaving questions about the harmony between semesters and academic calendars [United Nations (UN), 2020]. Since there is a definite need to use cognitive process technology during the pandemic, several studies have addressed this issue (Almanthari et al., 2020; Kerres, 2020). Specifically, this technology is an online virtual classroom application that leverages the communication process during classroom activities. The use of e-learning in higher education is generally detailed in terms of affordable cost and learning effectiveness (Clark and Mayer, 2016). Under normal circumstances, e-learning can support face-to-face learning as a complementary tool. Several studies on the effectiveness of e-learning have been published (Shi et al., 2020). However, COVID-19 has necessitated the immediate implementation of e-learning, and several studies on e-learning applications in education have been published (Abbasi et al., 2020; Almanthari et al., 2020; Favale et al., 2020; Radha et al., 2020). However, research on the implications of e-learning use is still scarce. Therefore, this study aimed to understand the predictors of e-learning effectiveness, especially the use of online classroom learning, through pathway analysis of doctoral students in Indonesia. It adopted the Technology Acceptance Model (TAM) as an academic model for understanding the relationship between extrinsic and intrinsic components.
E-learning, especially online learning, entails students being physically separated from their teachers and requiring technology as a delivery method (Wilde and Hsu, 2019). Interactions between students and faculty using technology can affect learning outcomes (Bower, 2019; Gonzalez et al., 2020). Online education and effective online education outcomes from well-prepared instructional design and planning have been studied for many years (Hodges et al., 2020). However, the COVID-19 pandemic required students around the world to switch from face-to-face classes to an online learning environment in the middle of the semester, although there is no clear evidence of the effectiveness of school closures in reducing the spread of the virus (Isfeld-Kiely and Moghadas, 2014). Human beings have limited information processing capabilities; hence, learning modality combinations can lead to cognitive overload, and impair the ability to master new information. If students are inexperienced with technology and lack a sense of cognitive involvement and social connectivity, learning outcomes may be adversely affected (Bower, 2019). By contrast, the effective use of technology can allow students and faculty members to engage and collaborate with each other (Gonzalez et al., 2020). Studies on users' intent have examined how students influence the success of online learning and the usefulness of technology (Kemp et al., 2019; Yakubu and Dasuki, 2019). Another study reported that the effectiveness of online learning depends on the level of user acceptance (Tarhini et al., 2016). Therefore, it is crucial to analyze factors related to the use and adoption of technology, which is one of the aims of this study. The TAM was developed by Fred Davis and Richard Bagozzi (Davis, 1989; Bagozzi, 2007) and is one of the most widely used models to examine user acceptance and technology use (Venkatesh, 2000; Kemp et al., 2019). The first TAM was based on a cognitive theory that described the process of adopting behaviors, and this model relates to users' willingness to use, and their continued use of, technology (García Botero et al., 2018; Teran-Guerrero, 2019).
During the COVID-19 pandemic, universities and their faculties were unable to appropriately plan and design their online learning lessons. Further, the social distancing necessitated by COVID-19 created a new social reality, which is the subject of this study.
Numerous science education frameworks have been used to understand the integration of technologies, especially the use of e-learning and online learning. Within this framework, the TAM is the most widely used and reported model in the social sciences context (Teo et al., 2018). The TAM defines this setting and predicts people's positive or negative emotions about behavioral intent performance associated with system adoption by the perceived usefulness and perceived ease of use of the system (Davis, 1989). In the original TAM theory, the model comprises perceived ease of use and perceived usefulness. In addition, it adapts behavioral intent (the extent to which people perform or do not perform certain future actions) to the system, predicting attitudes and perceived utility. Finally, the actual usage, called system usage, is predicted by behavioral intent (Davis, 1989). Studies have reported several external factors associated with the original TAM structure (Venkatesh and Davis, 2000; Venkatesh and Bala, 2008). Specifically, the TAM has been enhanced through a report on the integration of e-learning in education (Cakir and Solak, 2015; Mohammadi, 2015; Ramírez-Correa et al., 2019). For the purposes of this study, based on the original TAM, a hypothetical factor for predicting actual usage systems in terms of perceived ease of use, perceived usefulness, and behavioral intent to use is proposed. Table 1 depicts the TAM's framework.
This study uses quantitative data methods aimed at analyzing the usefulness, ease of use, behavioral uses, and actual use systems of online courses in Indonesian graduate education during the COVID-19 pandemic. The data were collected from January 2021 until December 2021. The sample selection method uses purposive sampling, which is based on the willingness of members to participate and respond to questionnaires shared via Google forms. The target population comprised 112 students in graduate programs. The structural equation model (SEM) was used to analyze multiple regression, as well as path analysis diagrams to visualize what was happening. All the variables integrated into the questionnaire were obtained from a previous literature search. In addition, all the questions were discussed with a panel of scholars and industry experts to assess the questionnaire items' validity. Throughout the questionnaire, a 5-point Likert Attitude Scale (1—totally disagree; 5—completely agree) and a behavioral rating scale (1- never; 5 -always) were used.
The relationship among constructs is depicted in a theoretical framework (Figure 1). The actual use system, as an endogenous construct, is measured with the following indicators: consistent usage (asu1, asu2, asu3, and asu4), transparency usage (asu5, asu6, asu7, and asu8), suitability of procedure (asu9, asu10, asu11, and asu12), and satisfaction usage (asu13, asu14, and asu15) adapted from the work of Davis (1989). The reason for adapting instruments that have been used by Davis (1989) and Venkatesh (2000) is that it links this study to all other research studies that have used the same instrument.
Conversely, the exogenous variable of perceived usefulness is measured through the following: responding to the needs of purposes (pu1, pu2, and pu3), control over the job (pu4, pu5, and pu6), the importance of the job (pu7, pu8, and pu9), job improvisation (pu10, pu11, and pu12), and improved user performance (pu13, pu14, and pu15), which were adapted from the work of Davis (1989) and Venkatesh (2000).
Perceived ease of use is measured through easy to learn (peu1, peu2, peu3, and peu4), easy to remember (peu5, peu6, peu7, and peu8), easy to understand (peu9, peu10, peu11, and peu12), and the availability of usage instructions (peu13, peu14, and peu15), which were adapted from the work of Davis (1989) and Venkatesh (2000).
Behavioral intentions to use were measured with motivation for permanent usage (biu1, biu2, and biu3), willingness to use in the future (biu4, biu5, and biu6), experiences (biu7, biu8, and biu9), motivation for usage (biu10, biu11, and biu12), motivating others (biu13, biu14, and biu15), that were developed from the work of Davis (1989).
Based on the parameters, the following hypotheses will be tested:
1. Perceived usefulness positively affects actual system use.
2. Perceived ease of use positively affects actual system use.
3. Behavioral intention to use positively affects actual system use.
4. Perceived usefulness positively affects behavioral intention to use.
5. Perceived ease of use positively affects behavioral intention to use.
6. Perceived usefulness positively affects actual system use through behavioral intention to use.
7. Perceived ease of use positively affects actual system use through behavioral intentions to use.
The testing phase of the measurement model included testing for convergent and discriminant validity and combined reliability. The results of partial least squares (PLS) analysis can be used to test research hypotheses, if all indicators of the PLS model pass the requirements of convergent and discriminant validities, and reliability checking. Convergence validation was performed by comparing the load factor value of each indicator with its components (Figure 2). Weights of factors greater than or equal to 0.5 are considered well-validated to explain the latent structure (Hair et al., 2020). In this study, if the average variance extracted (AVE) value was >0.5 for each configuration, the minimum allowable load factor limit was 0.5.
Based on the PLS model estimation results in Figure 2, given that the load factor values for all indicators exceeded 0.5, the model met the convergence validity requirement. In addition to checking the load factor values for each indicator, the validity of convergence was also evaluated using the AVE values for each configuration. Since each configuration's AVE was over 0.5, the convergent validity of this study's model met the requirements. The charge value, Cronbach's alpha, composite reliability, and AVE for each complete configuration are shown in Table 2.
The reliability of a construct can be assessed using the Cronbach's alpha score and the combined reliability of each construct. The recommended association reliability and Cronbach's alpha values are over 0.7, as shown in the reliability test results in Table 2, wherein all configurations have compound reliability and Cronbach's alpha values >0.7 (>0.7). In summary, all configurations met the required reliability values.
Respondents' demographic profiles related to gender, age, and academic level are presented in Table 3. Most of the respondents were male (65%), with the majority (44%) of respondents were in between 30 and 39 years old. Further, most of the respondents (58%) are master's degree students compared to (42%) who are doctoral degree students.
The validity and reliability test includes a significance test for direct and indirect effects and a measure of the magnitude of the effect of the exogenous variables on the endogenous variable. Impact testing was performed using statistical testing of a PLS analytical model using SmartPLS 3.3 software. The bootstrapping technique is used to obtain the R Square value and the significance test values, as shown in Table 4.
Table 4 also shows that the actual system usage R2 value is.619. This shows that the organizational variable is 61.9% for actual system usage. This is explained by the perceived usefulness and ease of use, and behavioral intent to use. The remaining 38.1% was explained by other variables not discussed in this study. Similarly, the coefficient of determination for behavioral intent is 0.789, implying that 78.9% of the behavioral intent variable is explained by the perception of usefulness and ease of use, and the remaining 21.1% by other variables that have not been discussed in this study. Table 5 shows the results of hypotheses testing for all the variables having a direct impact.
This study confirms that during COVID-19, students need to immediately switch from a face-to-face to an online learning environment and use virtual classroom applications for the implementation of the educational process, as the results of several studies on the application of e-learning in education have been published (Abbasi et al., 2020; Almanthari et al., 2020; Favale et al., 2020; Radha et al., 2020).
The proposed TAM-based variables are well described as predictors of the evolution of online graduate classes during the pandemic. However, the intended use of behavior does not significantly affect the use of the system (Hypothesis 3, 6, and 7 through Behavioral Intention show that the hypothesis is not proven).
Although the use of e-learning has succeeded in encouraging the implementation of the educational process through virtual classrooms, this study has shown that the use of e-learning alone on an ongoing basis is undesirable. It should be remembered that student intentions will affect learning outcomes (Tarhini et al., 2016; Kemp et al., 2019; Yakubu and Dasuki, 2019).
The public, including students in the education process, is tired of dealing with COVID-19. However, the end of physical restrictions is still not in sight, given that the COVID-19 mutant has brought about the next wave of the pandemic. In the educational process, students must learn physically apart from the teacher and class (Wilde and Hsu, 2019). Due to the unpredictable ending, several countries have decided that COVID-19 should be treated as endemic, where humans must live and survive in the new situation.
This study shows that although the perceived usefulness and ease of use variables directly show a significant effect, the indirect effect through behavioral intentions for the actual use of the system shows insignificant results. This can be interpreted that although students have experienced the convenience and benefits of virtual classroom application technology, they do not consider it desirable or show interest in maintaining this situation in the future. This shows that a long online learning process affects the learning process and outcomes (Bower, 2019; Gonzalez et al., 2020).
Thus, we can conclude that face-to-face learning is an irreplaceable educational process, and technology is a complement rather than a major factor in the educational process.
Based on this context, education is a process of interaction between humans through a series of instruments that facilitate the educational process to run more effectively and efficiently (Megahed and Mohammed, 2020; Shi et al., 2020). Technology is one of the instruments that can improve the educational process. However, technology cannot replace the role of lecturers in the classroom.
Technology Acceptance Model has been used everywhere to analyze the effectiveness of information technology, including in education. Many studies on TAM have demonstrated its success in defining people's attitudes, and positive or negative feelings about behavior toward adopting a system, which is predicted by perceived usefulness and ease of use.
The current results suggest that several variables in TAM, such as perceived usefulness and ease of use, directly influence behavioral intentions to use, including actual system use. However, the indirect effect did not show significant results. This is presumably because information technology is a complementary factor in the educational process and is not the main factor. Furthermore, the role of lecturers in direct interaction with students is suspected to be an irreplaceable factor in the educational process. This assumption requires further research to show that direct interaction between lecturers and students is human-human interaction which is an important part of the educational process.
Since this research was conducted in higher education institutions, especially in postgraduate programs, this article does not aim to generalize but rather to identify and develop preliminary discussions related to theoretical elements. This is undoubtedly the main limitation of this article. Future research on investigating the effectiveness of information technology use in the educational process should capture the importance of this underlying tension.
The raw data supporting the conclusions of this article will be made available by the authors, without undue reservation.
Ethical review and approval was not required for the study on human participants in accordance with the local legislation and institutional requirements. The patients/participants provided their written informed consent to participate in this study.
MT and WS contributed to the conception and design of the study. H performed the data collection and statistical analysis. DW wrote the first draft of the manuscript. MT, WS, DW, and H wrote sections of the manuscript. All authors contributed to manuscript revision, read, and approved the submitted version.
The authors declare that the research was conducted in the absence of any commercial or financial relationships that could be construed as a potential conflict of interest.
All claims expressed in this article are solely those of the authors and do not necessarily represent those of their affiliated organizations, or those of the publisher, the editors and the reviewers. Any product that may be evaluated in this article, or claim that may be made by its manufacturer, is not guaranteed or endorsed by the publisher.
We would like to thank Editage (www.editage.com) for English language editing.
Abbasi, S., Ayoob, T., Malik, A., and Memon, S. I. (2020). Perceptions of students regarding E-learning during Covid-19 at a private medical college. Pak. J. Med. Sci. 36, 5. doi: 10.12669/pjms.36.COVID19-S4.2766
Almanthari, A., Maulina, S., and Bruce, S. (2020). Secondary school mathematics teachers' views on E-learning implementation barriers during the COVID-19 pandemic: the case of Indonesia. Eurasia J. Math. Sci. Technol. Educ. 16, em186. doi: 10.29333/ejmste/8240
Bagozzi, R. P. (2007). The legacy of the technology acceptance model and a proposal for a paradigm shift. J. Assoc. Inf. Sys. 8 244–254. doi: 10.17705/1jais.00122
Bower, M. (2019). Technology-mediated learning theory. Br. J. Educ. Technol. 50, 1035–1048. doi: 10.1111/bjet.12771
Cakir, R., and Solak, E. (2015). Attitude of Turkish EFL learners towards e-learning through tam Model. Proc. Soc. Behav. Sci. 176, 596–601. doi: 10.1016/j.sbspro.2015.01.515
Clark, R. C., and Mayer, R. E. (2016). E-learning and the Science of Instruction: Proven Guidelines for Consumers and Designers of Multimedia Learning. New Jersey: John Wiley and Sons. p. 292–311.
Davis, F. D. (1989). Perceived usefulness, perceived ease of use, and user acceptance of information technology. MIS Q. 13, 319–340. doi: 10.2307/249008
Favale, T., Soro, F., Trevisan, M., Drago, I., and Mellia, M. (2020). Campus traffic and e-learning during COVID-19 Pandemic. Comput. Netw. 176, 107290. doi: 10.1016/j.comnet.2020.107290
García Botero, G., Questier, F., Cincinnato, S., He, T., and Zhu, C. (2018). Acceptance and usage of mobile assisted language learning by higher education students. J. Comput. High. Educ. 30, 426–451. doi: 10.1007/s12528-018-9177-1
Gonzalez, T., de la Rubia, M., Hincz, K., Lopez, M. C., Subirats, L., Fort, S., et al. (2020). Influence of COVID-19 confinement in students' performance in higher education. Jama 20, 90. doi: 10.1371/journal.pone.0239490
Hair, J. F., Howard, M. C., and Nitzl, C. (2020). Assessing measurement model quality in PLS-SEM using confirmatory composite analysis. J. Bus. Res. 109, 101–110. doi: 10.1016/j.jbusres.2019.11.069
Hodges, C., Moore, S., Lockee, B., Trust, T., and Bond, A. (2020). The difference between emergency remote teaching and online learning. EDUCAUSE Review. Available online at: https://er.educause.edu/articles/2020/3/the-difference-between-emergency-remote-teaching and-online-learning
Isfeld-Kiely, H., and Moghadas, S. (2014). Effectiveness of School Closure for the Control of Influenza. National Collaborating Centre for Infectious Diseases. Available online at: https://nccid.ca/publications/effectiveness-of-school-closure-for-the-control-of-influenza/ (accessed August 24, 2021).
Kemp, A., Palmer, E., and Strelan, P. (2019). A taxonomy of factors affecting attitudes towards educational technologies for use with technology acceptance models. Br. J. Educ. Technol. 50, 2394–2413. doi: 10.1111/bjet.12833
Kerres, M. (2020). Against all odds: education in Germany coping with Covid-19. Postdigit. Sci. Edu. 20, 690–694. doi: 10.1007/s42438-020-00130-7
Mohammadi, H. (2015). Investigating users' perspectives on e-learning: an integration of TAM and IS success model. Comput. Hum. Behav. 45, 359–374. doi: 10.1016/j.chb.2014.07.044
Radha, R., Mahalakshmi, K., Kumar, V. S., and Saravanakumar, A. R. (2020). E-Learning during lockdown of covid-19 pandemic: a global perspective. Int. J. Control Autom. 13, 1088–1099. Available online at: http://sersc.org/journals/index.php/IJCA/article/view/26035
Ramírez-Correa, P. E., Arenas-Gaitán, J., and Rondán-Cataluña, F. J. (2019). Gender and acceptance of e-learning: a multi-group analysis based on a structural equation model among college students in Chile and Spain. PloS One 10, e0140460. doi: 10.1371/journal.pone.0140460
Shi, D., Wang, T., Xing, H., and Xu, H. (2020). A learning path recommendation model based on a multidimensional knowledge graph framework for e-learning. Knowl. Base Syst. 195, 105618. doi: 10.1016/j.knosys.2020.105618
Tarhini, A., Hone, K., Liu, X., and Tarhini, T. (2016). Examining the moderating effect of individual-level cultural values on users' acceptance of E-learning in developing countries: a structural equation modeling of an extended technology acceptance model. Interact. Learn. Environ. 3, 306–328, doi: 10.1080/10494820.2015.1122635
Teo, T., Sang, G., Mei, B., and Hoi, C. K. W. (2018). Investigating pre-service teachers' acceptance of Web 2.0 technologies in their future teaching: a Chinese perspective. Interact. Learn. Environ. 27, 1–17. doi: 10.1080/10494820.2018.1489290
Teran-Guerrero, F. N. (2019). Aceptación de los estudiantes universitarios en el uso de los sistemas e-learning Moodle desde la perspectiva del modelo TAM.//Acceptance of university students in the use of Moodle e-learning systems from the perspective of the TAM model. Revista Ciencia UNEMI. 12, 63–76. doi: 10.29076/issn.2528-7737vol12iss29.2019pp63-76p
United Nations (UN) (2020). Policy Brief: Education During Covid-19 and Beyond. New York, NY: UN, Available online at: https://www.un.org/development/desa/dspd/wp-content/uploads/sites/22/2020/08/sg_policy_brief_covid-19_and_education_august_2020.pdf.
Venkatesh, V. (2000). Determinants of perceived ease of use: integrating control, intrinsic motivation, and emotion into the technology acceptance model. Inf. Sys. Res. 11, 342–365. doi: 10.1287/isre.11.4.342.11872
Venkatesh, V., and Bala, H. (2008). Technology acceptance model 3 and a research agenda on interventions. Decis. Sci. 39, 273–315, doi: 10.1111/j.1540-5915.2008.00192.x
Venkatesh, V., and Davis, F. D. (2000). A theoretical extension of the technology acceptance model: four longitudinal field studies. Manage. Sci. 46, 186–204. doi: 10.1287/mnsc.46.2.186.11926
WHO (2021). WHO Coronavirus Disease (COVID-19) Dashboard. Available online at: https://covid19.who.int/ (accessed June 28, 2021).
Wilde, N., and Hsu, A. (2019). The influence of general self-efficacy on the interpretation of vicarious experience information within online learning. Int. J. Educ. Technol. High. Educ. 16, 1–20. doi: 10.1186/s41239-019-0158-x
Keywords: COVID-19 pandemic, e-learning, technology acceptance model, education technology, education process
Citation: Tukiran M, Sunaryo W, Wulandari D and Herfina (2022) Optimizing Education Processes During the COVID-19 Pandemic Using the Technology Acceptance Model. Front. Educ. 7:903572. doi: 10.3389/feduc.2022.903572
Received: 24 March 2022; Accepted: 09 May 2022;
Published: 10 June 2022.
Edited by:
Mark Bedoya Ulla, Walailak University, ThailandReviewed by:
Jayrome Nunez, Visayas State University, PhilippinesCopyright © 2022 Tukiran, Sunaryo, Wulandari and Herfina. This is an open-access article distributed under the terms of the Creative Commons Attribution License (CC BY). The use, distribution or reproduction in other forums is permitted, provided the original author(s) and the copyright owner(s) are credited and that the original publication in this journal is cited, in accordance with accepted academic practice. No use, distribution or reproduction is permitted which does not comply with these terms.
*Correspondence: Martinus Tukiran, bWFydGludXMudHVraXJhbkB1bnBhay5hYy5pZA==
Disclaimer: All claims expressed in this article are solely those of the authors and do not necessarily represent those of their affiliated organizations, or those of the publisher, the editors and the reviewers. Any product that may be evaluated in this article or claim that may be made by its manufacturer is not guaranteed or endorsed by the publisher.
Research integrity at Frontiers
Learn more about the work of our research integrity team to safeguard the quality of each article we publish.