- 1School of Medicine, Universidad César Vallejo, Piura, Peru
- 2Center for Transdisciplinary Research in Psychology, Universidad Autónoma del Estado de Morelos, Cuernavaca, Mexico
- 3Faculty of Administrative Sciences, Universidad Nacional Mayor de San Marcos, Lima, Peru
Opportunities to learn (OTL) are considered important variables to enable equitable educational standards in teaching. The main objective of this work was to test a five-level hierarchical model to explain the general achievement and science competencies of Peruvian students in the Program for International Student Assessment (PISA) 2015, controlling for the student’s age and gender, and the economic, social, and cultural status, both for the student (ESCS) and the school (MESCS). The Peru database was taken from PISA 2015 and was based on the results of 6,971 Peruvian students from 281 schools. The 10 plausible values of performance in science reported by PISA 2015 were used, and each of the four sub-competencies was evaluated. The values weighted likelihood estimate (WLE) of the following composite variables were standardized: (1) In teaching-learning activities, the disciplinary environment in science classes, teaching support in science classes at the students’ choice, and inquiry-based science teaching-learning practices; teacher-directed science instruction were considered. (2) For the formative evaluation factor, feedback, student perception about the evaluation, and adaptation of the instruction to the formative evaluation were considered. (3) The control variables were as follows: the age and gender of the students, the index of social, economic, and cultural status (ESCS) at the student level, and the average of the index of the social, economic, and cultural status of each school (MESCS), as well as the interaction of OTL variables with ESCS. The results of the linear hierarchical analysis showed that the achievement of Peruvian students in science in PISA 2015 (general competence) is mainly due to feedback perceived, adaptive instruction, teacher-directed science instruction, and inquiry-based science teaching-learning practices. The feedback perceived and the inquiry-based science teaching predicted negatively and significantly to science performances. The effect of inquiry-based science teaching-learning on science achievement decreased as hierarchical analysis models became more complex and decreased substantially when simultaneously interacting with perceived feedback, the student gender, and ESCS. The results also showed that the gender, the ESCS of the students, and the MESCS of the school were significant and positive predictors of academic performance. When considering each of the scientific competencies evaluated, the models are like the general score. Mainly, the MESCS of the school controls or diminishes the effect that the instructional variables have on science performance, except for the negative effect of feedback on academic performance, even when the ESCS is controlled by the school level.
Introduction
Opportunities to learn (OTL) is a multidimensional construct widely used since the 1960s to explain what happens in learning situations in the classroom, laboratory, or practice settings, and its effect on indicators of academic achievement. From the initial approaches of a psychological and pedagogical instructional model (Carroll, 1963; Haertel et al., 1983; Bokhove et al., 2019), the OTL was consolidated as a useful construct to explain the effects of teaching practices (Stevens, 1993), and it is used as a factor to explain differences in learning outcomes on large-scale assessments and educational inequity (Husén, 1974; Schmidt and Burroughs, 2013). OTL also became a research construct and a reference for promoting public policies (McDonell, 1995).
There is a consensus that OTL constitutes variables of both inputs and preconditions (input), necessary and convenient instructional processes of teaching and learning situations, and curricular coverage and time, which influence academic achievement or outcome (Schmidt, 1992; Cervini, 2011; Elliott, 2015; Elliott and Bartlett, 2016; Hwang and Ham, 2021). Likewise, factors such as quality of instruction and assessment experiences include learning opportunity variables associated with academic achievement. The OTL indicators and categories used, however, may vary depending on the various theoretical approaches and types of learning assessments being carried out, as well as the type of secondary analysis concerning the various large-scale assessments.
Thus, for example, for some, OTL could mean a set of teacher practice activities, such as, linking theories to practical situations, lesson planning and teaching, and reflection on practice (König et al., 2017), or they could be considered as time spent by the student studying or doing homework at home or instructional time covered by the teacher (Bokhove et al., 2019). Also, OTL could involve instructional activities, such as fostering an appropriate learning environment, classroom management, clear instruction, activating instruction, differentiated instruction, and learning strategies (André et al., 2020).
The PISA has included the association between the assessment of competencies and cognitive domains and context indicators to classroom (school) level, which inquiries about cognitively challenging activities in the classroom, which can be identified in the context questionnaires applied to students in the PISA 2006, 2009, 2012, and 2015 tests (Organization for Economic Co-operation and Development [OECD], 2017a). In the 2012 application, the OTL variables have been presented in three groups (Organization for Economic Co-operation and Development [OECD], 2013): (a) as part of the teacher’s behavior in the teaching situation (Variables: teacher-directed teaching, formative assessment, and student-oriented instruction), (b) as part of instruction, referred to as quality of instruction (Variables: Support in teaching mathematics, cognitive activation, disciplinary climate, teacher support, and classroom management), and (c) as part of teaching and assessment experiences (familiarity with learning content and its application to real-life problems).
On the other hand, in the PISA 2015 test with emphasis on science, the focus was oriented on identifying teaching and learning activities in science learning in the classroom, and the general category that OTL was not explicitly used to characterize such activities. However, the PISA 2015 assessment framework did consider those categories as part of assessing teaching and learning processes (see Table 1), including the categories of inquiry-based teaching and learning (cognitive activation) and teacher-directed instruction. Likewise, in this category of teaching and learning processes, the dimensions of teaching quality were included, such as the disciplinary climate in the classroom and support for teachers (Organization for Economic Co-operation and Development [OECD], 2017a). On the other hand, under formative evaluation, feedback and adaptability of instruction were included, dimensions that have to do with the formative evaluation in teaching-learning processes.
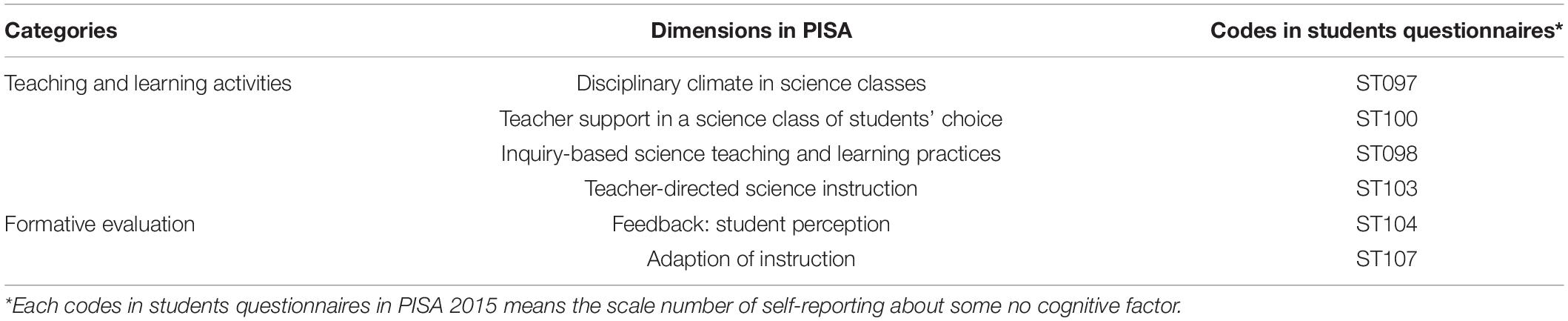
Table 1. Opportunities to learn (OTL) variables for assessing teaching and learning processes [Program for International Student Assessment (PISA) 2015].
In the broadest sense of the word, these dimensions are indicators of learning opportunities that are structured in science teaching and learning situations, which occur especially at the school level, and specifically in the classroom or laboratories. They are part of the instructional processes that go into facilitating learning and are expected to favorably impact the students’ achievement, as well as their academic achievement (Elliott, 2015; Elliott and Bartlett, 2016; Hwang and Ham, 2021). On the other hand, the index of economic, social, and cultural status (ESCS) has been considered a variable that affects the relationship between opportunity variables for the learning and academic achievement (Quiroz et al., 2020; Murillo and Carrillo, 2021; Rolfe et al., 2021; Bazán-Ramírez et al., 2022b).
Background of Studies on Opportunities to Learn and Science Competencies in Program for International Student Assessment 2015
In the PISA 2015 test, scientific literacy was assessed as an overall average performance based on three scientific competencies: explaining phenomena scientifically, evaluating and designing scientific investigations, and interpreting data and evidence scientifically. In PISA 2015, in the area of science, Peru obtained an overall average of 397 points, well below the OECD average of 493 points, and in Latin America, below Chile, Uruguay, Costa Rica, Colombia, Mexico, and Brazil (Organization for Economic Co-operation and Development [OECD], 2016; Ministerio de Educación, 2017). As for the performance level that PISA manages in a range of less than 1b (level 1) and 6 (level 8), Peru’s average was equivalent to level 1a, which is the third-lowest level in PISA’s range of eight levels. Likewise, the performance of Peruvian students in the three science competencies in PISA was also low.
To explain students’ performance in science in PISA 2015, different secondary analysis studies have been reported, taking as predictor variables the scores in non-cognitive assessments based on contextual questionnaires answered by students worldwide, including OTL variables of instructional practices and formative evaluation and associated student and contextual variables, such as Index of economic, social and cultural status, gender, and student motivational variables (Kang et al., 2019; Teig et al., 2020; Campbell, 2021; Liou, 2021; Kang, 2022).
Although it is true that in the reference framework of the PISA 2015 test, only the variables inquiry-based science teaching and learning practices and teacher-directed science instruction can be distinguished as instructional practices. Authors, like Mostafa et al. (2018), included as part of practical instructional practice the variables adaptive teaching and teacher feedback, which according to Table 1 of this study, are part of a category called formative evaluation. Regardless of this first categorical confusion, the analysis by Mostafa et al. (2018), with data from the countries participating in PISA 2015, showed very important results. For example, negative and significant associations were reported between science inquiry-based instruction and science performance and a positive relationship between classroom discipline climate and science performance. Likewise, the authors reported that this negative relationship between science inquiry-based instruction and science performance is increased in science classes that lack an adequate disciplinary climate. Another important aspect shown by this secondary analysis by Mostafa et al. (2018) was that in 27 countries, out of the 70 countries participating in PISA 2015, more instruction based on scientific inquiry was reported in schools with a disadvantaged socio-economic profile. In addition, these countries present indicators of higher educational inequality, among which was Peru.
Taking the database of 10 countries of different socioeconomic levels and performance in science PISA 2015, including Peru, Hwang et al. (2018) conducted another secondary analysis study to assess the differential effect between two instructional strategies (the traditional one called teacher-centered instruction, and the other called student-oriented instruction, based on scientific inquiry) and considering the socio-economic level of the students. The results showed, in those ten countries, a significant association between a higher presence of science inquiry-based instruction and low scores on students’ overall science proficiency (She et al., 2018), but it was also found that a higher frequency of student-centered and science inquiry-based instruction causes the gap in science proficiency between students of low and high socioeconomic status to generally narrow or remain the same (Hwang et al., 2018).
Taking the PISA 2015 test databases from six English-speaking countries (Canada, Australia, Ireland, New Zealand, United Kingdom, and United States), Oliver et al. (2021) investigated the effect of types of instructional practice on overall science scores (scientific literacy). They included inquiry-based instruction in science, teacher-directed instruction, and adaptive teaching as types of instructional practice. It is worth emphasizing that the last variable was included in the reference framework of the PISA 2015 test, as a category of formative evaluation. As in the two studies referred to above, students in the six countries that reported experiencing high frequencies of scientific inquiry-based teaching strategies had lower levels of scientific literacy. Likewise, a strongly positive association was found between the frequency of adapted teaching strategies and teacher-directed instruction with science literacy scores.
Several secondary analysis studies with PISA 2015 databases from various countries have consistently shown the negative relationship between science inquiry-based instruction and science performance and the positive relationship between teacher-directed instruction and science scores. Likewise, classroom climate variables, teacher support during instruction, formative evaluation variables, and other variables related to the students and the context of origin have been included as predictors of achievement in science.
The Peruvian Ministerio de Educación (2020) published results of secondary analyses with data from Peruvian students in PISA 2015, and among the most striking was the negative relationship between the availability of educational materials and overall science scores and the significant but negative association of both inquiry-based instruction and feedback received, with overall science scores. A significant and positive association was also reported between exposure to teacher-directed instructional practices and science achievement, but no significant relationship was found between instructional adaptation (formative evaluation) and overall science achievement.
Using Taiwan’s PISA 2015 database, Liou (2021) found that after controlling for students’ demographic and socio-economic characteristics, teacher-directed instructional practices had a positive and significant effect on students’ achievement in science proficiency, whereas inquiry-based instructional practices had a significant negative effect on students’ scientific performance. On the other hand, the inquiry-based instructional practice had greater predictive power for attitudes of students toward science compared to the effect of teacher-directed instructional practices.
Secondary analyses with data from Argentina in the PISA 2015 test (Quiroz et al., 2020) showed the effect of three dimensions of OTL (Ability to understand the task, Student willingness to actively participate in learning, and quality of instruction), on achievement levels in science, having controlled for student and school socio-economic levels, and available school resources. Instructional quality included three composite variables of student perceptions of teaching practice: teacher-directed science teaching, teacher feedback, and inquiry-based teaching-learning. An important aspect is that inquiry-based instruction had a positive and significant effect, as did teacher-directed instruction, upon achievement levels in science. At the school level, significant institutional segmentation in OTL was reported, while at the student level (intra-school), OTL indicators had a significant effect on science achievement.
Yetişir and Batı (2021), using Turkey’s PISA 2015 database, analyzed the effect of school and student-related factors on science performances, including disciplinary climate and teacher support in instruction. The results showed that the teacher’s management of the disciplinary climate in the classroom positively influences student performance and that ESCS, whether at the student or school level, is one of the most important variables in predicting student performance. The authors conclude that students’ science performance can be improved by eliminating the lack of educational materials or personnel because Turkish schools differ greatly in terms of sociocultural structure, unlike other OECD countries. Likewise, Grabau et al. (2021), with the Finnish PISA 2015 databases, modeled through multilevel analysis the relationship between science dispositions (epistemology, enjoyment, interest, and self-efficacy) and school science climate (disciplinary climate and teacher support) with science performance (scientific literacy). The results showed that science score was significantly and positively associated with the four scientific dispositions and with the disciplinary climate in science classes.
Beyond showing the effect of composite variables of science inquiry-based instruction and direct teacher instruction on science performance in PISA 2015, other secondary analyses have conducted disaggregation of the individual items of these composite variables (constructs) to assess their differential effect on science achievement (Cairns, 2019; Cairns and Areepattamannil, 2021; Oliver et al., 2021; Kang, 2022). These studies help to demystify the linear assumption that inquiry-based instructional practice has a negative effect on global science achievement, since some items of the construct inquiry-based instruction have been reported to have positive effects on science performance, and others have an indirect effect on achievement, mediated by direct instructional practice items from the teacher.
Present Study
As can be observed from the first part of this article, variables related to opportunities for learning, essentially those of instructional practices, classroom management, and formative evaluation, have proven to have differential effects on academic achievement results in science in PISA 2015 in different countries and their different contexts of origin of their students and schools. A common denominator has been, except for the data from Argentina, the negative relationship between the composite variables’ inquiry-based science teaching and learning practices and feedback received, with overall scores in science (scientific literacy). Likewise, the antecedents reflected the significant and positive relationship between teacher-directed science instruction and overall science achievement, and to a lesser extent, also the positive relationship between adaption of instruction (a component of a Formative evaluation construct) and science achievement. Another aspect that has been reiterative in the secondary analysis of PISA 2015 science data is the role of ESCS as a variable regulating the effect of OTL and overall achievement in science competencies.
In the Peruvian case, the most direct antecedent is reported by the Ministerio de Educación (2020), regarding the negative but significant effect of both inquiry-based science teaching and learning practices and feedback: student perception on overall achievement in science, as well as of the significant and positive association between teacher-directed science instruction and overall achievement in science. However, little is known about the differential effect of all OTL variables for assessing teaching and learning processes in PISA 2015 (Organization for Economic Co-operation and Development [OECD], 2017a) on both overall scores and on each of the competencies of Peruvian students who participated in PISA 2015, controlling for gender and student and school ESCS levels. For this reason, the present study posed the following research question: How do the variables of teaching and learning activities and formative evaluation activities influence the performance of Peruvian students in the overall average and science competencies in PISA 2015, when controlling for the Index of social, economic, and cultural status at the individual and school level, and the gender of the students?
In accordance with the research question, two main objectives were proposed: (1) To test a five-level hierarchical model explaining the overall science achievement of Peruvian students in PISA 2015, and (2) To determine the differences in science achievement of Peruvian students in PISA 2015 between high ESCS level students and low ESCS level students.
Materials and Methods
Sample
Peru’s PISA 2015 database was taken with results from 6,971 Peruvian students aged 15 years or older. The final sample was composed of N = 6,971 students (49.6% female and 50.4% male) from 281 schools, in 4th year or less (74.62%) and 5th year of high school (25.38%). The average age was 15.7 years with a DE = 0.47.
Measurements
Competencies in Science
The PISA 2015 database provides the overall (global) score that each student obtains in the area of science (scientific literacy) and the scores in each of the three science competencies that make up scientific literacy: “(1) Explain phenomena scientifically. Recognize, offer, and evaluate explanations for a range of natural and technological phenomena. (2) Evaluate and design scientific enquiry. Describe and appraise scientific investigations and propose ways of addressing questions scientifically. (3) Interpret data and evidence scientifically” (Organization for Economic Co-operation and Development [OECD], 2017a,b; Bazán-Ramírez et al., 2022b). In both the overall science score and the scores in each of the three competencies, the PISA 2015 database provides ten plausible values. The use of plausible values (PV’s) in large-scale standardized assessments allows estimating the value within a reference framework that is very large and each student does not respond to the entire test in question. To carry out these evaluations, a matrix design of test sections is used; respondents are exposed to different sections and their ability is estimated by the imputation of the PVs. In this method, a posteriori distribution of the latent trait that is associated with the ability of the individual is found (Córdoba Perozo, 2016), and such distribution obtained by the PVs estimates the true distribution of the ability of the population (Marsman et al., 2016). Each plausible value was standardized by an overall performance mean of 500 points with a standard deviation of 100 points.
Teaching and Learning Activities (Opportunity to Learn)
The standardized values weighted likelihood estimate (WLE) of the variables listed below were taken. The standardization of the variables establishes a mean of zero and an SD for the values; therefore, values above zero represent positive attitudes or traits, and those below zero, negative attitudes or traits.
The Disciplinary Climate in Science Classes (WLE)
This index details whether noise and clutter are controlled in the classroom, and that the teacher can ensure that students have an environment where their students can concentrate on academic tasks.
Teacher Support in Science Classes of Students’ Choice (WLE)
This index represents the interest that teachers show in each student’s learning; provide additional help when needed; assist students with their learning; continue teaching until there is a full student understanding of the material; and allow students to express their opinions.
Inquiry-Based Science Teaching and Learning Practices (WLE)
Inquiry-based teaching is based on involving students in experimentation and hands-on activities and challenging and encouraging them to develop a conceptual understanding of scientific ideas.
Teacher-Directed Science Instruction (WLE)
It refers to teaching strategies that, although they make students assume a passive role, it is essential that the teacher communicate for students to learn. Such practices are teacher explanations, class discussions, and students’ questions.
Formative Evaluation
This latent variable in turn included two composite variables collected with the student questionnaire (StQ), one referring to feedback: student perception and the other referring to adaptation of instruction.
Perceived Feedback (WLE)
This index of perceived feedback is composed of five questions that measure the degree to which students perceive that teachers regularly provide them with feedback.
Adaption of Instruction (WLE)
Adaptive instruction refers to the flexibility of teachers in their lessons, in which they adapt lessons to students, including students who have difficulty with a topic or task.
Control Variables
Gender
It is set as a discrete variable, where the reference value (0) corresponds to female students, while male students (1) were considered to interpret the coefficients.
Age
Age of the students.
Index of Economic, Social, and Cultural Status
The ESCS is a composite index that was constructed by parental educational level (PARED), highest parental occupation (HISEI), and household possessions (HOMEPOS), as well as total books in the household, using principal components analysis (PCA).
The Average Index of Social, Economic, and Cultural Status by the School (MESCS)
It is the average of the different ESCS values of each student by the school, it establishes the ESCS context to which students are exposed.
Data Analysis
Data Cleaning and Preparation
For science achievement, 10 plausible values of the overall achievement or total score were taken as the dependent variable in the multilevel hierarchical model. To analyze the differences in science achievement for each of the three competencies assessed in PISA 2015, the 10 plausible values for each of them were also used.
Multilevel Hierarchical Models
To explain the general achievement in science, the 10 plausible values of the overall achievement or total score were taken as the dependent variable, and five hierarchical models of multilevel analysis were tested. Model 1 only tested the effect on science achievement of the four personal and contextual control variables: gender, age, index of ESCS, and average index of MESCS. Model 2 tested the effect on the overall achievement of the four variables of teaching and learning activities: disciplinary climate in science classes, teacher support in science classes, inquiry-based science teaching and learning, and teacher-directed science teaching. Model 3 tested the effect on the overall achievement of the two formative assessment variables: perceived feedback and instructional adaptation. Model 4 tested the effect on overall achievement, simultaneously including the four variables of teaching and learning activities and the two variables of formative evaluation. Finally, model 5 simultaneously evaluated the effect on science achievement of all the variables included in models 1, 2, and 3. Likewise, in the multilevel models, the program produces a prior model called “the null model,” which does not include any parameter or variable associated with the achievement; in fact, this model allows to know the variance explained by the schools and the general mean of performance when controlling for all the variables included in the model, which will be incorporated in other models.
These five hierarchical models were replicated for each of the three science competencies that PISA 2015 evaluated, taking as dependent variables the 10 plausible values in each competency. For a more streamlined presentation, only model 5 will be shown comparatively in the results.
Differences in Science Achievement by the Level of Program for International Student Assessment Scores According to Two Extreme Levels of Economic, Social, and Cultural Status
In addition to the multilevel hierarchical analysis to explain achievement in science, differences in low and medium-high PISA achievement were analyzed according to two extreme ESCS levels, for which two extreme ESCS level categories (high and low) and two PISA achievement level categories were formed: low level (1b) and medium-high level (4, 5, and 6).
Results
Science Achievement (Global Score)
Table 2 presents the results of the null model and the five hierarchical models tested to explain the overall science achievement of Peruvian students in the 2015 PISA test. The upper part of Table 2 shows the null model for general competence in science of the population in general, in which the different coefficients of the student variables were calculated, as well as those of learning opportunities and formative evaluation. The null model shows that the intercept obtained (398) is one SD below the OECD mean; in addition, in this model, the percentage of variance found among schools is 33%, while the remaining 64% is found in the students. In model 1, when the student’s characteristics are considered, it can be seen that there is a marginal difference in the intercept (396.79) with respect to the previous model, which means that when the personal characteristics of the students are incorporated, the intercept undergoes modifications due to these sources of explanation.
In model 1, the gender of the students, who have as a referent the female gender, obtained a significant value (coefficient = 11.86 points for male respondents), and the socio-economic and cultural level of the student has a similar value (10.69 points), while the value of the school context (MESCS) is almost a third of an SD (30.89). In this first hierarchical model, the variance between schools decreases to 10%. In model 2, which analyzed the influence of the four variables of teaching and learning activities (OTL) on the result of global competence in science, only inquiry-based science teaching and learning and teacher-directed science teaching are significant, although the former has a highly negative impact (53 points, approximately), the latter is positive (30 points). In this second model, the percentage of the variance between schools is approximately 34%. The third model estimated the influence of formative assessment on overall science achievement; both variables analyzed were found to be significantly influential, although with different effects. On the one hand, perceived feedback has negative effects and almost one-half of the SD (52 points); on the other hand, instruction adaptation has a positive effect (approximately 31 points). The percentage of variance explained among schools by this model is slightly higher than that obtained in model 2 (35%).
Model 4 incorporates the opportunity for learning. The intercept is slightly higher by 8 points (398.33 points) compared to the previous model; while the variables on learning opportunities only two of them are significantly associated with achievement: science teaching and learning are negatively related to achievement, while science teaching exhibits a positive significant impact of about half a deviation (52.79 points). The formative assessment variables continue to have a significant effect on achievement, although with some differences in scores with respect to Model 3: perceived feedback (−62.27 points) and adaptation of instruction (41.92 points). This model reduces the percentage of the variance attributable to the school (25%). Finally, Model 5 incorporated all variables, both characteristics of the students and the learning opportunities and formative evaluation variables. In the case of the personal characteristics of the students, the variables of the gender of the student, the ESCS, and the school average of the student are maintained, although they have marginal differences from the values obtained in previous models: the influence of gender increases by two points, while the ESCS average decreases by four points. Finally, ESCS shows no change. In the same way, it can be seen in the model that the significant effects of the variables in the previous models persist in this last one; however, the size of the effects changes. The variables that exhibit notable differences in their magnitude are those of learning opportunities such as teaching and learning science which decreased by approximately 33 points (−12.83); for its part, teacher-directed science teaching also decreased, in this case, 31 points (21.22). Regarding the formative evaluation variables, these also decreased perceived feedback by 28 points (−34.79), and adaptation of instruction by 18 points (23.20). One result to emphasize is the reduction of the variance among schools, which in this model is only 8%.
Achievement in the Three Program for International Student Assessment 2015 Science Competencies
Table 3 shows the results of the multilevel 5 model for each of the three science competencies of Peruvian students in the PISA 2015 test. Model 5 simultaneously includes, as predictors of each scientific competency, the variables of model 1 (personal and school variables), model 2 (teaching and learning activities), and model 3 (formative evaluation: feedback on student perception and adaptation of instruction). This table shows that the personal and school variables (control variables) have differentiated effects depending on the scientific competency. For example, gender and age are variables have greater predictive weight on Explain phenomena scientifically competency, gender in a positive way, and age in a negative way. Thus, being a male has a greater impact on this competency compared to the other two, but it is also observed that the higher the age, the lower the score in the Explain phenomena scientifically competency. Coincidentally, age has a significant effect only on this competency and not on the other two, or the overall science score. Likewise, as other elements highlight, a greater predictive effect of the socio-economic and cultural level of the school was observed compared to the effect of the ESCS, with a greater effect in the third competency evaluated (Interpret data and evidence scientifically).
Regarding the effect of the four variables of OTL Teaching and learning activities, the predictive trend in the overall science score shown in Table 2 is generally confirmed for only two variables: teacher-directed science teaching in a positive way, followed by inquiry-based science teaching and learning in a negative way. However, the negative effect of the last variable is not significant in the first competency (Explain phenomena scientifically). As for the effect on each of the scientific competencies of the variables contained in evaluative training, the same as in the global competency, it is perceived feedback that has the greatest predictive weight, and in a negative way, while the variable adaptation of instruction has a positive and significant effect on only two of the three competencies (Explain phenomena scientifically and Interpret data and evidence scientifically).
The variance of the school explained in these models is slightly higher (between 2 and 5% values) than in Model 5 of the general science competency. This means that the variables considered in these models, both individual characteristics and learning opportunities and formative evaluation, explain a smaller percentage of the differences between schools than is the case in Model 5 of general competency. They contribute less to reducing the variance explained by schools. On the other hand, the percentage of variance explained by schools is higher than in general science competency, and this difference ranges between 3 and 4% points. On the other hand, the percentage of variance explained by the students is lower (between 87.24 and 87.84), compared to the percentage of variance explained by the students in the overall science competency (91.39%).
Differences in Science Achievement According to Program for International Student Assessment Score Level, Based on Two Extreme Economic, Social, and Cultural Status Levels
In addition to the multilevel hierarchical analysis to explain achievement in science, differences in low and medium-high PISA achievement were analyzed according to two extreme ESCS levels, for which two extreme ESCS level categories (high and low) and two PISA achievement level categories were formed: low level (1b) and medium-high level (4, 5, and 6). Table 4 shows the averages obtained from crossing the levels of the aforementioned variables. It is possible to observe that there is a very small difference between the low and high ESCS levels when the achievement is Low, while when comparing the Medium High achievement, no student with Low ESCS level, while the students with High ESCS have on average a performance that significantly exceeds their counterparts of the Low ESCS level.
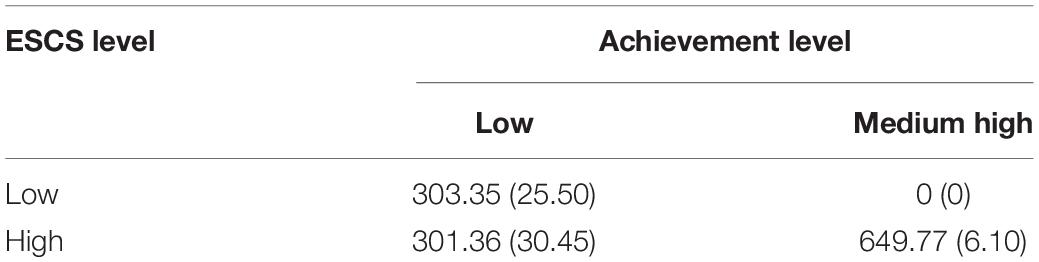
Table 4. Averages (and SDs) of science proficiency in PISA 2015 by achievement level and economic, social, and cultural status (ESCS) level.
Discussion
This research sought to answer the question, how do the variables of teaching and learning activities and formative evaluation activities influence the performance of Peruvian students in the overall science average and science competencies in PISA 2015? For this purpose, control variables were also included, such as the index of social, economic, and cultural status at the individual and school levels, and the gender of the students. When evaluating the overall science performance of Peruvian students in PISA 2015, as a predictor variable, only two of the four variables of teaching and learning activities were found to have significant effects: inquiry-based science teaching and learning, in a negative way, and teacher-directed science teaching in a positive way.
The first indication of these findings is the trend of the negative effect of inquiry-based science teaching and learning practices, and the positive effect of teacher-directed science instruction on overall science scores, which have already been reported in comparative studies of countries that participated in PISA 2015 (Hwang et al., 2018; Mostafa et al., 2018; Oliver et al., 2021) and in secondary analyses with specific data by country (Ministerio de Educación, 2020; Liou, 2021). However, the data from our study show important findings that should be considered when analyzing the effect of instructional practices as opportunities for learning, including simultaneously, formative assessment variables, which are also learning opportunity variables.
Our data show that the effect of inquiry-based science teaching and learning practices on science achievement compared to the effect of teacher-directed science instruction decreases substantially when the linear hierarchical models are complexified and the two formative evaluation variables are added. That is to say, the effect of science inquiry-based science instruction is sensitive to both the variables of ESCS, gender, formative evaluation, and teacher-directed science instruction. This association of formative evaluation variables (OTL manifestations of instructional quality) was also demonstrated by the study of Kang (2022) with Finnish students in PISA 2055, who also showed that the negative association between inquiry-based science instruction and scientific literacy (science achievement), an error has been made in considering the variable inquiry-based science instruction as a unidimensional variable, and to demonstrate this, he incorporated two types of scientific inquiry, open and closed, finding differential effects on science achievement.
A second clue derived from the findings of this study concerns the important role of teacher-directed science teaching as an instructional practice in science. In this study, the effect of this variable on science achievement increased and remained superior to the effect of inquiry-based instruction, when formative assessment variables and ESCS were added. The importance of teacher-centered or teacher-oriented instruction in explaining overall science performance in PISA 2015 was evidenced in Mostafa’s et al.(2018) report and has been strongly highlighted by both Hwang et al. (2018) and Liou (2021), as well as Oliver et al. (2021). Likewise, in the study of secondary analyses with data from Argentine students, Quiroz et al. (2020) found that the variable of teacher-oriented instruction had a positive and significant effect, as did instruction based on scientific inquiry.
Regarding the effect of formative evaluation variables, the findings of this study coincide with results from other secondary analysis studies, in which adaptation of instruction has a positive effect on science achievement (Ministerio de Educación, 2020; Oliver et al., 2021). This aspect is very important because it places the practices of teachers that favor student-centered instruction as a central element (Hwang et al., 2018, Hwang et al., 2021; Liou, 2021). In fact, in our study, this variable had greater predictive weight in model 5 on the overall science score of Peruvian students in PISA 2015, compared to the effect of the four variables of teaching and learning activities. The adaptation of instruction to the needs of the student body consists of teachers orienting their instructional practice to the needs of their students and based on the formative assessment and curricular objectives. Mostafa et al. (2018) emphasizes that adaptive teaching is positively correlated with science performance in the countries that participated in PISA 2015, mainly in countries that have incorporated personalized instructional practices, that is, student-oriented.
Regarding the negative effect of feedback on academic achievement, the data coincided with the findings reported by the Peruvian Ministerio de Educación (2020). But in the report of the Peruvian Ministry of Education’s linear hierarchical analysis, feedback is the second variable of the four instructional practice variables with the greatest predictive weight on the overall science logo, after the negative effect of science inquiry-based instruction. But in the present study, feedback is the variable with the highest predictive weight considering the four instructional variables and the two formative competency variables.
Although it is true that feedback has been proposed as one of the fundamental variables in the instructional process at various educational levels and that it can positively impact learning and academic achievement (Forsythe and Jellicoe, 2018; Jellicoe and Forsythe, 2019; Winstone et al., 2019; Krijgsman et al., 2021; Bazán-Ramírez et al., 2022a), in this study it has a negative effect on the overall science score. One possible explanation is that this relationship may be because students who perceive greater feedback on their learning from their teachers are those who have poor learning in science. In the same way, and according to the students’ assessment, it is possible that the teachers could have shown the students more frequent feedback behaviors when they were already showing learning difficulties. In this sense, it is suggested that research be conducted to cross-check information from both teachers and students regarding the feedback provided to students and its subsequent association with academic achievement in science.
Another aspect to consider is the multi-trait nature of the feedback construct (Jellicoe and Forsythe, 2019; Krijgsman et al., 2019), which when investigated with self-reports may be collecting various feedback features at a time distant from the time of their assessment. In this regard and based on a meta-analysis, Wisniewski et al. (2020) have pointed out that the impact of feedback can be significantly influenced by the content of the information transmitted in the feedback process so that feedback may include different forms and may have different effects on student learning.
The second point of discussion is the differential effect of the instructional practices and formative competency variables, especially in the full model of the multilevel hierarchical analyses tested (model 5), on achievement in each of the three science competencies in PISA 2015. Although the general trend of the negative effect of science inquiry-based instruction and the positive effect of teacher-oriented instruction on the science performances of Peruvian students that supported PISA 2015 is maintained, these vary according to scientific competency. For example, the negative effect of instruction based on scientific inquiry does not reach significance in the competency “Explain phenomena scientifically” and is higher and more significant (negative) in the competency “Evaluate and design scientific inquiry.” Likewise, teacher-directed science education had different significant (positive) effects on the three competencies, having greater weight on the competence “Evaluate and design scientific inquiry,” the other two variables of teaching and learning activities (Disciplinary climate in science classes; Teacher support in science classes) had no significant effects on any of the scientific competencies.
About the formative assessment variables, perceived feedback maintained significant but negatively predictive weights in all three competencies, having a greater impact on the first scientific competency (Explain phenomena scientifically). In contrast, the variable adaptation of instruction showed significant predictive weights only in two of the three scientific competencies (Explain phenomena scientifically and Interpret data and evidence scientifically). In most of the resulting analyses, when including, on the one hand, the four instructional practice variables and the two formative competency variables, the regression coefficients in model five for the specific competencies were higher than the predictive coefficients in model five for global science competency.
According to our results, instructional practice and formative competency variables, as part of learning opportunities, may influence academic achievement competencies in different ways, depending on whether a general index is taken, such as science literacy, or whether indexes of specific science competencies are taken. These differential data on the effect of instructional variables on the three scientific competencies assessed in PISA 2015 can provide important guidelines for decision-makers at the national, regional, and municipal levels to direct efforts to improve instructional opportunities according to each scientific competency.
It is worth reiterating that these results are substantially important and should be considered by educational planners and decision makers in educational policies in Peru, and by basic education teachers, to direct efforts to address in a differential manner the possible effect of the instructional and formative evaluation variables, depending on the type of scientific competencies that one wishes to work on with students, not only in secondary education but also in elementary school. Likewise, these data can guide the self-reflection of teachers and mid-level managers in the improvement of their teaching practices and in the importance of comprehensively influencing the various scientific competencies of their students.
A third relevant aspect to consider from the data derived from the present study is the effect of the control variables on the science performance indicators of Peruvian students in PISA 2015. First, the gender variable had a greater significant effect on overall achievement when it was included along with all the variables (socio-economic, educational, cultural indexes, teaching strategies, and formative evaluation). That is, the effect of gender was larger than ESCS on the overall science score. On the other hand, when the scores in each of the three science competencies were taken, the effect of the gender variable was also superior in all cases on the effect of ESCS, with greater emphasis on the competency “Explain phenomena scientifically.” This fact confirms the findings of other studies with PISA 2015 results in sciences, which have shown that the gender of the students explains, to a greater extent, the differences in achievement (Kang et al., 2019; Teig et al., 2020; Campbell, 2021; Liou, 2021).
The previous results seem to reflect that performance in science is associated with different traits between women and men, which makes it a primary objective of science literacy education for students, not only the teaching strategies of teachers and formative assessment but also the need to include these differences by gender in decision making as part of a public policy of education and culture. Science teaching in secondary (and elementary) schools will have to take into consideration the differences students have shown regarding their preferences in different science subjects, even before high school, when they start learning science as a distinct academic subject (Kang et al., 2019). Considering what happens in other countries in which gender equity is a distinctive feature of the educational systems, the development of scientific interests of students depends on the role of teachers in basic education, who should motivate students to be oriented toward some area of scientific interest regardless of their gender. In addition to school factors, there are social and economic factors, women do not show interest in their future science-related career paths being linked to occupying a leadership position, social recognition, and economic well-being (Kang et al., 2019).
Regarding the indexes of socio-economic, educational, and cultural levels, the results of our study have shown that it is the MESCS (at the school level) that has the greatest predictive weight on achievement in all multilevel hierarchical models, both in the overall science score and in each of the science competencies, compared with the effect of student gender and ESCS (at the individual level), and with the effect of all four pedagogical strategy variables and the two formative evaluation variables, except the effect of the perceived feedback variable.
Both ESCS and MESCS play a very important role in educational inequalities within a nation’s school system; namely, to the extent that the concentration of both types of factors occurs in only one type of school (such as privately maintained schools), the quality of education and the corresponding inequality is accentuated. Latin America is characterized as the region whose school systems have the greatest educational inequality, where the socio-economic and cultural level of students (which they brought to the institution, i.e., MESCS), is one of the main causes of such inequity (Murillo, 2016). In these contexts, students who, relatively independent of their own ESCS, attend schools that have on average a better school context for this factor, benefit more than those who attend an institution with a low ESCS average. In addition to this contextual benefit of peers, schools that concentrate students with high ESCS levels in their enrollment usually have greater educational resources, teachers with better initial and ongoing training, principals with desirable characteristics for good school management, better school infrastructure, etc. These school features are associated with better educational quality, which in turn, increases the gaps in education among the population with different socio-economic and cultural levels.
As an additional issue, it is pertinent to point out that in this study the 10 plausible values of the general competency and the different sub-competencies that make it up were used to estimate student performance in science. This type of analysis allows having a better estimation of the latent trait of ability or competency of individuals (Córdoba Perozo, 2016), where the distribution of ability scores, because of employing the different plausible values, reflects the true population distribution (Marsman et al., 2016).
Conclusion
According to the first objective set out in this study, it is concluded, first, that both the overall achievement score in science and achievement in the three science competencies of Peruvian students assessed with PISA 2015, it is the perceived feedback variable that has the greatest predictive impact on such achievement, although in a negative way. The high valuation of receiving feedback from their teachers is associated with low scores in science. Likewise, the instructional practice referred to inquiry-based science teaching negatively but significantly affects the achievement of Peruvian students in science; however, this effect is significantly reduced when controlling for gender and socio-economic and cultural levels at the school level.
The second conclusion of this study is that academic achievement in science is positively determined by the variable adaptation of instruction based on the formative evaluation. After perceived feedback (in a negative way) and the average of the index of MESCS, adaptation of the instruction is the third-best predictor of science achievement indicators in the most complete hierarchical models, both in the overall score and in the three science competencies. Likewise, teacher-centered instruction (teacher-directed science instruction) is also a positively determinant variable for academic achievement in science, although to a lesser extent than the instructional adaptation variable.
As third conclusion, the average of the index of MESCS is a determinant for the academic achievement of Peruvian students in science (globally and in each science competency), after perceived feedback. The MESCS index (at the school level) is the variable that had the greatest predictive weight on achievement in all multilevel hierarchical models, both in the overall science score and in each of the science competencies, compared to the effect of student gender and ESCS (at the individual level) and to the effect of all four pedagogical strategy variables and the two formative evaluation variables, except the effect of the perceived feedback variable.
As a fourth conclusion of the present study, it can be stated that student gender is also a determinant of academic achievement in science, but with more emphasis on overall achievement in science as compared to achievement in each of the three science competencies. The sex of the student body has a greater explanatory weight for science achievement indicators than the socio-economic and cultural level of the students. In other words, under conditions of equality in educational, economic, and cultural terms, being a woman implies lower achievements in science than being a man.
In accordance with the second objective of this work and as conclusion five, it can be pointed out that the index of ESCS level makes a substantial difference in the academic achievement of Peruvian students in science in the 2015 PISA evaluation, for example, in the medium-high academic achievement, the difference by ESCS level is abysmal in favor of students with a high ESCS level. Although this study shows the worrying panorama in terms not only of the low performance of Peruvian students in science in PISA 2015, these data also provide space and opportunity to reflect on the relationship between these variables of learning opportunities (instructional and formative assessment), and the importance of considering gender and the student body, such as the index of social, economic, and cultural status at the school level, to take better measures to generate changes in the instructional processes and terms of public education policies in Peru.
Data Availability Statement
The raw data supporting the conclusions of this article will be made available by the authors, without undue reservation.
Author Contributions
AB-R led the secondary data analysis study, managed the database, prepared the version for this study, conducted the literature search, wrote the initial version and Frontiers format, and contributed to the data analysis and preparation of tables. EH-P led the statistical analyses and preparation of tables of results, contributed to the conception and development of the study, and drafting of the manuscript. WB-R cooperated in the preparation of the manuscript in the Frontiers format and interpretation of results, supported the search for additional bibliographic information, and reviewed the style of the article. MT-A was in charge of the English version and writing in Frontiers format, contributed to the revision and corrections of the draft of the Spanish version, and supported the search for additional bibliographic information. All authors contributed to the article and approved the submitted version.
Funding
The publication expenses and the edition of the English version of this manuscript are covered by the Universidad César Vallejo of Peru.
Conflict of Interest
The authors declare that the research was conducted in the absence of any commercial or financial relationships that could be construed as a potential conflict of interest.
Publisher’s Note
All claims expressed in this article are solely those of the authors and do not necessarily represent those of their affiliated organizations, or those of the publisher, the editors and the reviewers. Any product that may be evaluated in this article, or claim that may be made by its manufacturer, is not guaranteed or endorsed by the publisher.
References
André, S., Maulana, R., Helms-Lorenz, M., Telli, S., Chun, S., Fernández-García, C.-M., et al. (2020). Student perceptions in measuring teaching behavior across six countries: a multi-group confirmatory factor analysis approach to measurement invariance. Front. Psychol. 11:273. doi: 10.3389/fpsyg.2020.00273
Bazán-Ramírez, A., Capa-Luque, W., Bello-Vidal, C., and Quispe-Morales, R. (2022a). Influence of Teaching and the teacher’s feedback perceived on the didactic performance of peruvian postgraduate students attending virtual classes during the COVID19 Pandemic. Front. Educ. 7:818209. doi: 10.3389/feduc.2022.818209
Bazán-Ramírez, A., Montes-Iturrizaga, I., and Castro-Paniagua, W. (2022b). Household possessions and parental support in Mexican students with high scientific competencies in PISA 2015. Eur. J. Educ. Res. 11, 259–366. doi: 10.12973/eu-jer.11.1.259
Bokhove, C., Miyazaki, M., Komatsu, K., Chino, K., Leung, A., and Mok, I. A. C. (2019). The role of “opportunity to learn” in the geometry curriculum: a multilevel comparison of six countries. Front. Educ. 4:63. doi: 10.3389/feduc.2019.00063
Cairns, D. (2019). Investigating the relationship between instructional practices and science achievement in an inquiry-based learning environment. Int. J. Sci. Educ. 41, 2113–2135. doi: 10.1080/09500693.2019.1660927
Cairns, D., and Areepattamannil, S. (2021). Teacher-directed learning approaches and science achievement: investigating the importance of instructional explanations in australian schools. Res. Sci. Educ. 1–15. doi: 10.1007/s11165-021-10002-0
Campbell, J. A. (2021). The moderating effect of gender equality and other factors on pisa and education policy. Educ. Sci. 11:10. doi: 10.3390/educsci11010010
Cervini, R. (2011). Equidad y oportunidad de aprender en la educación básica [Equity and opportunity to learn in basic education]. Profesorado 15, 67–86.
Córdoba Perozo, M. F. (2016). An application of plausible values to the standardized test scoring through simulation. Común. Estad. 9, 51–72. doi: 10.15332/s2027-3355.2016.0001.03
Elliott, S., and Bartlett, B. (2016). “Opportunity to Learn,” in Oxford Handbook of Education Online, ed. P. Nathan (New York, NY: Oxford Press), doi: 10.1093/oxfordhb/9780199935291.013.70
Elliott, S. N. (2015). Measuring opportunity to learn and achievement growth: key research issues with implications for the effective education of all students. Rem. Spec. Educ. 36, 58–64. doi: 10.1177/0741932514551282
Forsythe, A., and Jellicoe, M. (2018). Predicting gainful learning in higher education; a goal-orientation approach. High. Educ. Ped. 3, 103–117. doi: 10.1080/23752696.2018.1435298
Grabau, L. J., Lavonen, J., and Juuti, K. (2021). Finland, a package deal: disciplinary climate in science classes, science dispositions and science literacy. Sustainability 13:13857. doi: 10.3390/su132413857
Haertel, G. D., Walberg, H. J., and Weinstein, T. (1983). Psychological models of educational performance: a theoretical synthesis of constructs. Rev. Educ. Res. 53, 75–91. doi: 10.3102/00346543053001075
Husén, T. (1974). Multi-National evaluation of school systems: purposes, methodology, and some preliminary findings. Scand. J. Educ. Res. 18, 13–39.
Hwang, J., and Ham, Y. (2021). Relationship between mathematical literacy and opportunity to learn with different types of mathematical tasks. J. Math. Educ. 12, 199–222. doi: 10.22342/jme.12.2.13625.199-222
Hwang, J., Choi, K. M., Bae, Y., and Shin, D. H. (2018). Do teachers’ instructional practices moderate equity in mathematical and scientific literacy?: An investigation of the PISA 2012 and 2015. Int. J. Sci. Math. Educ. 16, 25–45. doi: 10.1007/s10763-018-9909-8
Hwang, G. J., Wang, S. Y., and Lai, C. L. (2021). Effects of a social regulation-based online learning framework on students’ learning achievements and behaviors in mathematics. Comput. Educ. 160:104031.
Jellicoe, M., and Forsythe, A. (2019). The development and validation of the Feedback in Learning Scale (FLS). Front. Educ. 4:84. doi: 10.1016/j.jbi.2021.103952
Kang, J. (2022). Interrelationship between inquiry-based learning and instructional quality in predicting science literacy. Res. Sci. Educ. 52, 339–355. doi: 10.1007/s11165-020-09946-6
Kang, J., Hense, J., Scheersoi, A., and Keinonen, T. (2019). Gender study on the relationships between science interest and future career perspectives. Int. J. Sci. Educ. 41, 80–101. doi: 10.1080/09500693.2018.1534021
König, J., Ligtvoet, R., Klemenz, S., and Rothland, M. (2017). Effects of opportunities to learn in teacher preparation on future teachers’ general pedagogical knowledge: analyzing program characteristics and outcomes. Stud. Educ. Eval. 53, 122–133. doi: 10.1016/j.stueduc.2017.03.001
Krijgsman, C., Mainhard, T., Borghouts, L., van Tartwijk, J., and Haerens, L. (2021). Do goal clarification and process feedback positively affect students’ need-based experiences? A quasi-experimental study grounded in self determination theory. Phys. Educ. Sport Ped. 26, 483–503. doi: 10.1080/17408989.2020.1823956
Krijgsman, C., Mainhard, T., van Tartwijk, J., Borghouts, L., Vansteenkiste, M., Aelterman, N., et al. (2019). Where to go and how to get there: goal clarification, process feedback and students’ need satisfaction and frustration from lesson to lesson. Learn Instr. 61, 1–11. doi: 10.1016/j.learninstruc.2018.12.005
Liou, P.-Y. (2021). Students’ attitudes toward science and science achievement: an analysis of the differential effects of science instructional practices. J. Res. Sci. Teach. 58, 310–334. doi: 10.1002/tea.21643
Marsman, M., Maris, G., Bechger, T., and Glas, C. (2016). What can we learn from Plausible Values? Psychometrika 81, 274–289. doi: 10.1007/s11336-016-9497-x
McDonell, L. (1995). Opportunity to learn as a research concept and a policy instrument. Educ. Eval. Pol. Anal. 17, 305–322. doi: 10.3102/01623737017003305
Ministerio de Educación (2017). El Perú en PISA 2015 Informe Nacional de Resultados. Lima: Ministerio de Educación.
Ministerio de Educación (2020). Factores Asociados al Desarrollo de la Competencia Científica en Estudiantes Peruanos Según PISA 2015. Madrid: Ministerio de Educación.
Mostafa, T., Echazarra, A., and Guillou, H. (2018). The Science of Teaching Science: An Exploration of Science Teaching Practices in PISA 2015 Issue 188 of OECD Education Working Papers. Paris: OECD Education, doi: 10.1787/f5bd9e57-en
Murillo, F. J. (2016). Midiendo la segregación escolar en américa latina. un análisis metodológico utilizando el TERCE [Education in Latin America and the Caribbean. Contributions of TERCE and its Reanalysis]. REICE. Ibero-Amer. J. Qual. Effect. Chang. Educ. 14, 33–60. doi: 10.15366/reice2016.14.4.002
Murillo, F. J., and Carrillo, S. (2021). Incidencia de la segregación escolar por nivel socioeconómico en el rendimiento académico. un estudio desde Perú [Incidence of socio-economic school segregation on academic performance A study from Peru]. Educ. Pol. Anal. Arch. 29:49. doi: 10.14507/epaa.29.5129
Oliver, M., McConney, A., and Woods-McConney, A. (2021). The efficacy of inquiry-based instruction in science: a comparative analysis of six countries using PISA 2015. Res. Sci. Educ. 51, 595–616. doi: 10.1007/s11165-019-09901-0
Organization for Economic Co-operation and Development [OECD] (2013). PISA 2012 Results: Ready to Learn: Students’ Engagement, Drive and Self-Beliefs (Volume III): Preliminary Version. Paris: OECD.
Organization for Economic Co-operation and Development [OECD] (2016). PISA 2015 Results. Excellence and Equity in Education (Vol. I). Paris: OECD.
Organization for Economic Co-operation and Development [OECD] (2017a). PISA 2015 Assessment and Analytical Framework: Science, Reading, Mathematic, Financial Literacy and Collaborative Problem Solving, revised edition, PISA. Paris: OECD Publishing, doi: 10.1787/9789264281820-en
Organization for Economic Co-operation and Development [OECD] (2017b). PISA 2015 Technical Report. Paris: OECD, https://bit.ly/3HgVKG9
Quiroz, S. S., Dari, N. L., and Cervini, R. A. C. (2020). Oportunidad de aprender y segmentación socioeconómica en Argentina-PISA 2015 [Opportunity to Learn and Socio-economic Segmentation in Argentina - PISA 2015]. REICE. Ibero-Amer. J. Qual. Effect. Chang. Educ. 18, 93–112. doi: 10.15366/reice2020.18.3.005
Rolfe, V., Strietholt, R., and Hansen, K. Y. (2021). Does inequality in opportunity perpetuate inequality in outcomes? International evidence from four TIMSS cycles. Stud. Educ. Eval. 71:101086. doi: 10.1016/j.stueduc.2021.101086
Schmidt, W. H. (1992). “The distribution of instructional time to mathematical content: one aspect of opportunity to learn,” in The IEA Study of Mathematics III: Student Growth and Classroom Processes, ed. L. Burstein (New York, NY: Pergamon Press), 129–145. doi: 10.1016/B978-0-08-041371-6.50014-6
Schmidt, W. H., and Burroughs, N. A. (2013). Opening the black box: prospects for using international large-scale assessments to explore classroom effects. Res. Comp. Int. Educ. 8, 236–247. doi: 10.2304/rcie.2013.8.3.236
She, H. C., Stacey, K., and Schmidt, W. H. (2018). Science and mathematics literacy: PISA for better school education. Int. J. Sci. Math. Educ. 16, 1–5. doi: 10.1007/s10763-018-9911-1
Stevens, F. I. (1993). Applying an opportunity-to-learn conceptual framework to the investigation of the effects of teaching practices via secondary analyses of multiple-case-study summary data. J. Negro Educ. 62, 232–248. doi: 10.2307/2295463
Teig, N., Scherer, R., and Kjaernsli, M. (2020). Identifying patterns of students’ performance on simulated inquiry tasks using PISA 2015 log-file data. J. Res. Sci. Teach. 57, 1400–1429. doi: 10.1002/tea.21657
Winstone, N. E., Mathlin, G., and Nash, R. A. (2019). Building feedback literacy: students’ perceptions of the developing engagement with feedback toolkit. Front. Educ. 4:39. doi: 10.3389/feduc.2019.00039
Wisniewski, B., Zierer, K., and Hattie, J. (2020). The power of feedback revisited: a meta-analysis of educational feedback research. Front. Psychol. 10:3087. doi: 10.3389/fpsyg.2019.03010.3389/fpsyg.2019.03087
Keywords: opportunities, teaching, learning, PISA 2015, Peruvians
Citation: Bazán-Ramírez A, Hernández-Padilla E, Bazán-Ramírez W and Tresierra-Ayala M (2022) Effects of Opportunities to Learn on Peruvian Students’ Science Achievement in Program for International Student Assessment 2015. Front. Educ. 7:897473. doi: 10.3389/feduc.2022.897473
Received: 16 March 2022; Accepted: 20 April 2022;
Published: 02 June 2022.
Edited by:
Nicola Pitchford, University of Nottingham, United KingdomReviewed by:
Josiane Campos Cruz, Federal University of Paraíba, BrazilÁfrica Borges, University of La Laguna, Spain
Copyright © 2022 Bazán-Ramírez, Hernández-Padilla, Bazán-Ramírez and Tresierra-Ayala. This is an open-access article distributed under the terms of the Creative Commons Attribution License (CC BY). The use, distribution or reproduction in other forums is permitted, provided the original author(s) and the copyright owner(s) are credited and that the original publication in this journal is cited, in accordance with accepted academic practice. No use, distribution or reproduction is permitted which does not comply with these terms.
*Correspondence: Aldo Bazán-Ramírez, YWJhemFucmFtaXJlekBnbWFpbC5jb20=