- Department of Vocational Education, University of Education Schwäbisch Gmünd, Schwäbisch Gmünd, Germany
While facilitating factors to learning at the workplace have been well investigated, throughout the past decade less has emerged about the barriers that occur when approaching a learning activity at the workplace. Barriers to learning at the workplace are factors that hinder the initiation of successful learning, interrupt learning possibilities, delay proceedings or end learning activities much earlier than intended. The aim of this study is to develop and validate an instrument that measures barriers to informal and formal learning at the workplace. An interview pre-study asked 26 consultants about their learning barriers based on existing instruments. Using this data as groundwork, a novel measuring instrument of barriers to informal and formal learning was developed. The instrument is comprised of five factors with items on individual barriers, organizational/structural barriers, technical barriers, change and uncertainty. To validate the scales, a cross-sectional questionnaire with 112 consultancy employees and freelancers was conducted. The validation included exploratory factor analysis, internal consistency assessment, confirmatory factor analysis (CFA) and convergent validity assessment. The results generated a three-factor scale barrier measurement for formal learning and a two-factor scale barrier measurement for informal learning. All scales featured Cronbach’s alpha values ranging between 0.80 and 0.86. With this developed and validated scales it is intended to help offer insights into factors that hinder individuals from learning at the workplace, and show organizations their potential for change.
Introduction
The factors facilitating learning at the workplace have been well investigated (Kyndt et al., 2018), less is known about barriers that occur when approaching a learning activity at the workplace (Boeren, 2016). Based on Tynjälä’s (2008) conceptualization of workplace learning, learning can be formal, non-formal or informal; emerge in a deliberate or unplanned setting; and occur on the individual, team or organizational level (Segers et al., 2021). Informal learning at the workplace can be defined as a process that is not organized, controlled or structured by individuals or an institution, thereby rendering it unintended learning from experience that occurs in a situation that is meaningful to the learner (Neaman and Marsick, 2018; Hager, 2019). Informal learning usually does not lead to credentials either, in contrast with formal learning, which focuses on achieving certifications or degrees and is well-structured, embedded in institutions and taught according to a curriculum (Marsick and Watkins, 2001; Lecat et al., 2020; Rodriguez-Gomez et al., 2020).
Barriers to learning at the workplace form a holistic set of missing individual, team and organizational aspects. They are factors that hinder the initiation of successful learning, interrupt learning possibilities, delay proceedings or end learning activities much earlier than intended (Crouse et al., 2011). Most of the existing instruments that measure learning barriers or inhibitors have a primary focus on the organizational level (Eken et al., 2020; Kezar and Holcombe, 2020; Allen and Heredia, 2021), specific individual concepts such as anxiety (Piscitelli, 2021) or preferences for new technologies (He and Yang, 2021). Upon identifying barriers, then, it soon becomes clear that well-reviewed facilitating factors and learning conditions are what raise the question of what to add or foster to bolster learning outcomes and thus overcome the expected learning barriers (e.g., Cerasoli et al., 2018; Jeong et al., 2018; Kyndt et al., 2018). Such solutions could be more equipment, a better team climate or more possibilities to learn. Decius et al. (2021) highlighted that employee characteristics and the learning culture in the organization are important in investigating learning.
Learning facilitators and barriers can be opponents (Cerasoli et al., 2018), such as when more equipment is regarded as a facilitator and current equipment at the workplace as a barrier to learning activities (Louws et al., 2017; Keck Frei et al., 2021). This indicates that facilitators and barriers to learning are not of universal application. It is rather based on individual or team related concepts and assumption. What hinders one group of learners could be a facilitator for another, such as time pressure or the provision of certain resources in an office learning environment. Learning barriers lead to the question of what is not working well and should be changed, like miscommunication, poor work environment or lack of autonomy (Brion, 2021; Decius et al., 2021; Kim et al., 2021). This can only be spotlighted when considering the complexity of barriers at the workplace. To best investigate barriers at the workplace, the investigated domains at the workplace should include different aspects of knowledge-intensive service work, should deal with multiple demands and offer the possibility to solve problems in various ways. The consultancy, is suitable in this regard due to its special focus on multiple, complex and challenging work environments; the complexity of tasks; the high variety of solution strategies; and its diverse career development paths (Korster, 2022). These characteristics fall in line with a constant need for professional development and lifelong learning to keep up with the magnificent and ill-structured problems occurring at the workplace (O’Leary, 2020; Dolata et al., 2021). The required competences of consultants are diverse and dynamic as well (Wißhak and Hochholdinger, 2020; Helens-Hart and Engstrom, 2021; van der Baan et al., 2022). Thus, learning is essential to managing the multiple demands and challenges at the workplace in this domain (Dymock and Tyler, 2018), to which interruptions, limitations or barriers can be a serious threat.
In reflection of the assorted barriers that can arise in these varied scenarios, research on barriers to learning form a continuum based on individual learning experiences, situations and roles. Moreover, to fully understand learning activities, it is necessary to reflect on facilitators and barriers equally. Accordingly, based on the explorative interview study (Anselmann, in press) and results and existing concepts from Belling et al. (2004) and Crouse et al. (2011), the aim of this present study is to develop and validate a measurement instrument of barriers to informal and formal learning at the workplace.
Theoretical background
Barriers to learning
Barriers to learning at the workplace are factors that hinder the initiation of successful learning, interrupt learning possibilities, delay learning proceedings or end learning activities much earlier than intended (Crouse et al., 2011). Regarding hierarchy, barriers can occur on the individual, team or organizational level. Research indicates that motivational factors (Nouwen et al., 2022), social interactions (e.g., Mishra, 2020), the general structure or equipment of the workplace (Billett, 2022; Goller and Paloniemi, 2022) and further career development (Matusik et al., 2022) can all be learning barriers. In turn, these barriers can be external, internal or refer to problems with organizational fit. Barriers to learning at the workplace can be external, internal or refer to problems with organizational fit (Johnson et al., 2018; Nel and Linde, 2019).
External barriers are restrictions to learning through authoritative knowledge (Jordan, 2014) or bounded agency (Goller and Paloniemi, 2022). Internal learning barriers can be found, for instance, in personal ideas and concepts which determine how, where, in which frequency and for what reason learning activities are engaged or dismissed (Hager, 2019). Fitting problems, meanwhile, refer mainly to external barriers that create physical, cognitive or emotional separations from work tasks (Alikaj et al., 2021; Papacharalampous and Papadimitriou, 2021). They are regarded as the alienation between learners and their daily work tasks, indicating that fitting problems can be a negative consequence of barriers occurring in an organizational structure. One example of a fitting problem is when employees do not apply organizational rules in a standardized process because they believe they are non-functional or do not fit their own work preferences. The results of non-engagement in learning activities can lead to limited daily work tasks or long-term unachievable targets (Alikaj et al., 2021; Papacharalampous and Papadimitriou, 2021).
While a constant need for life-long learning is addressed multiple times, an engagement in learning activities, does not emerge from itself. Participation and engagement in learning depend on the results of cognitive, emotional and behavioral engagement and evaluations in learning activities themselves (Shuck and Herd, 2012), which are determined by external and internal barriers, as well as fitting problems. The results of non-engagement in learning activities can lead to limited daily work tasks or long-term unachievable targets (Shuck, 2019).
Informal and formal learning
Daily situations at the workplace offer opportunities for informal learning. Informal learning is understood as a process that can be deliberate or reactive and leads to acquiring of competence (Simons and Ruijters, 2004). Furthermore, Marsick and Watkins (2001) outline that informal learning is “usually intentional but not highly structured” and can include “self-directed learning, networking, coaching, mentoring” (Marsick and Watkins, 2001, p. 25–26). It occurs individually or socially outside organized learning settings. Informal learning is also influenced by the characteristics of individuals, workplaces and organizations (e.g., self-efficacy, leadership, feedback; Jeong et al., 2018). In contrast, Marsick and Watkins (2001) define formal learning as “typically institutionally sponsored, classroom-based, and highly structured (Marsick and Watkins, 2001, p. 25).
Workplace learning
Emphasis on the workplace as a place of learning already occurs in Argyris and Schön (1996), where the researchers subdivide the actions occurring in the workplace into knowing in action, knowing what to do in a situation and reflecting in action, or thinking about steps to take. Throughout the last few decades, the workplace has emerged from “an environment not only where learning new knowledge and skills can happen but where learning should be happening. However, despite its ever-increasing importance, there is no broad consensus on what workplace learning is, with many definitions simply stating that it involves all learning that happens at the workplace” (Kankaraš, 2021, p. 9). Based on these considerations, a number of other concepts were emerged and divided into workplace learning (Billett, 1995) and work-related learning (Streumer and Kho, 2006). Billett (2022) assumes that workplace learning and actions follow a structure based on work experience. In this context, learning activities are neither formal, nor incidental, nor unstructured, nor spontaneously directed. On the contrary, they are determined by the requirements of the workplace. The individual and contextual factors that condition the workplace learning thereby function as determinants of workplace learning. Doornbos et al. (2008) similarly break down work-related learning into three components: process, learning environment and outcome of the process. Work-related learning is considered to be a predominantly explicit process that is guided by predefined learning objectives. In turn, learning itself is understood as a cognitive and rational process that takes place in an environment characterized by a certain structure (Billett, 2022). Guidance and control of knowledge access are passed on and reviewed by authorities, and the result of this process is a competent individual increase in knowledge and skills.
Jacobs and Park (2009) summarize the divergently occurring processes of workplace learning through the metaphor of learning cells. Learning events may occur singly or in majority in the workplace or in situations associated with it, all referred to as cells. Each of these cells reflects experiences and learning actions that may emerge in the work context under certain conditions. Learning at the workplace can thus be interpreted as learning for the workplace: the actions initiated serve and improve the skills that may be necessary to perform the job (Segers et al., 2021). In the context of informal learning, the workplace is particularly relevant with its requirement of continuous employee development. Marsick and Watkins (2015) even list informal learning as one of two learning forms that predominantly occur in the workplace, with the other being incidental learning.
In summary, learning at the workplace can be characterized as taking place both on the job and off the job (Hager, 2019), thereby indicating that learning content is linked to the requirements of the job. Informal learning on the job, according to Hager (2019), is mainly contextual and work- and experience-based, and furthermore stems from situations in which learning is not the main goal. The situation is usually initiated by the learning environment itself or the individuals who are learning, rather than by teachers or trainers, and is often socially shared. These learning actions and concepts of workplace learning outline the practical framework in which learning at the workplace can take place.
Scale construction
Scale development
Pre-study
In a pre-study, interviews with 26 consultants (N = 26) were conducted to find out what are the learning barriers to informal and formal learning (Anselmann, in press). The theoretical deduced semi-structured interviews were conducted via phone, Zoom, or Skype and lasted between 17 and 45 min (M = 29:17 min; SD = 6:23 min), they were recorded and transcribed. The interview guidelines were developed based on the definition of learning conditions in the workplace (Skule, 2004; Jeong et al., 2018), formal and informal learning activities (Simons and Ruijters, 2004), and barriers to learning (Crouse et al., 2011). Throughout the whole process of data collection, transcription, interpretation, and storing, EU General Data Protection Regulation (GDPR) rules were followed. In addition, a procedure index was established and approved by the researchers’ universities’ data protection officers.
The results revealed both external barriers, such as vague supervisor requirements, and internal barriers, such as disruptions from clients or supervisors, with additional subcategories for internal barriers like organizational fit (e.g., ineffective IT) and individual barriers (e.g., uncertainty). Table 1 shows all identified internal and external barriers on the individual, team and organizational levels, as well as denotes the most common of these barriers.
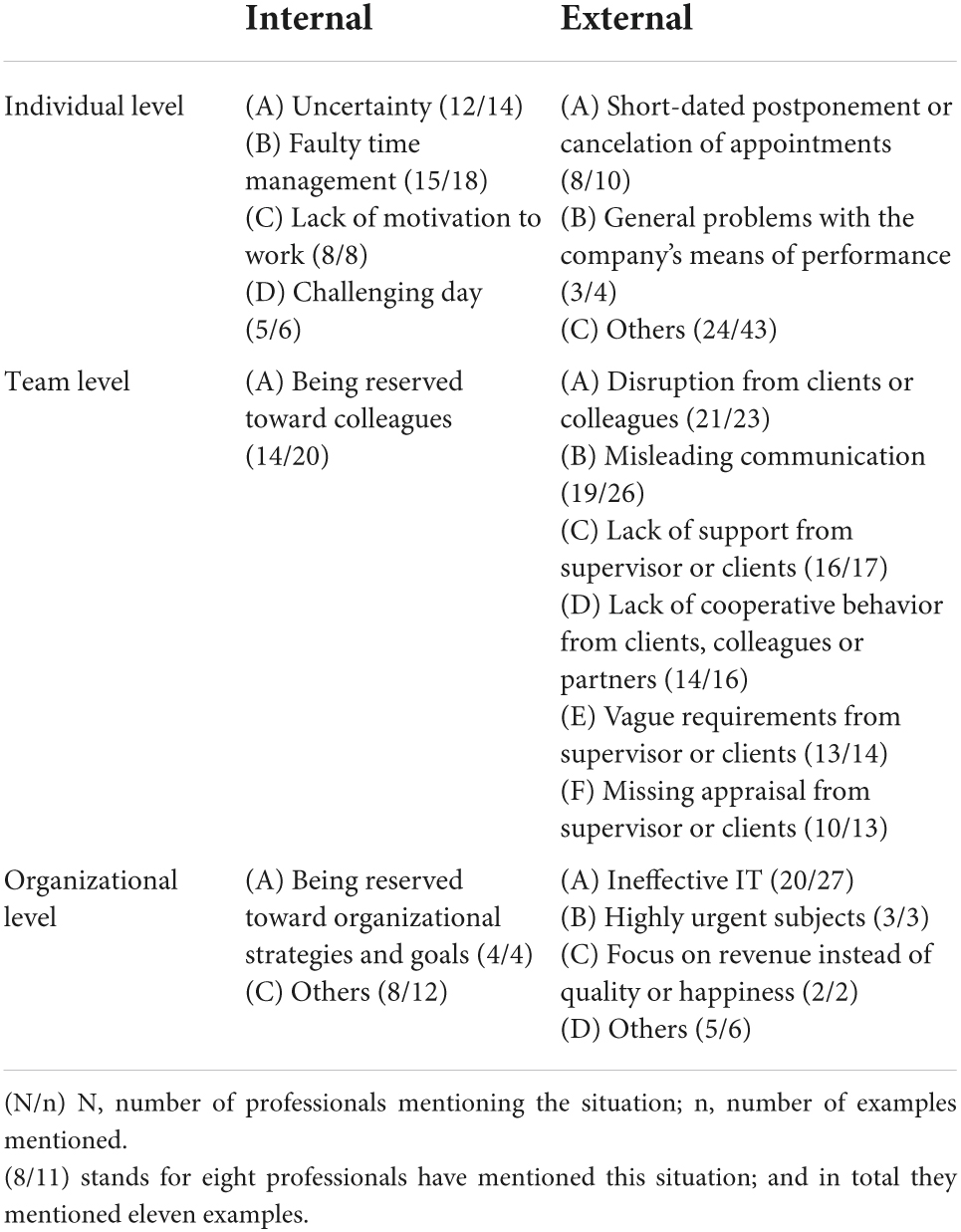
Table 1. Internal and external barriers to learning at the individual, team and organizational levels (Anselmann, in press).
Based on these results, 27 statements for the questionnaire used in the current study were identified. The developed items refer to topics like lack of cooperative behavior from clients, colleagues or partners, disruptions from clients or colleagues and ineffective IT. Table 2 shows all items and related examples per category.
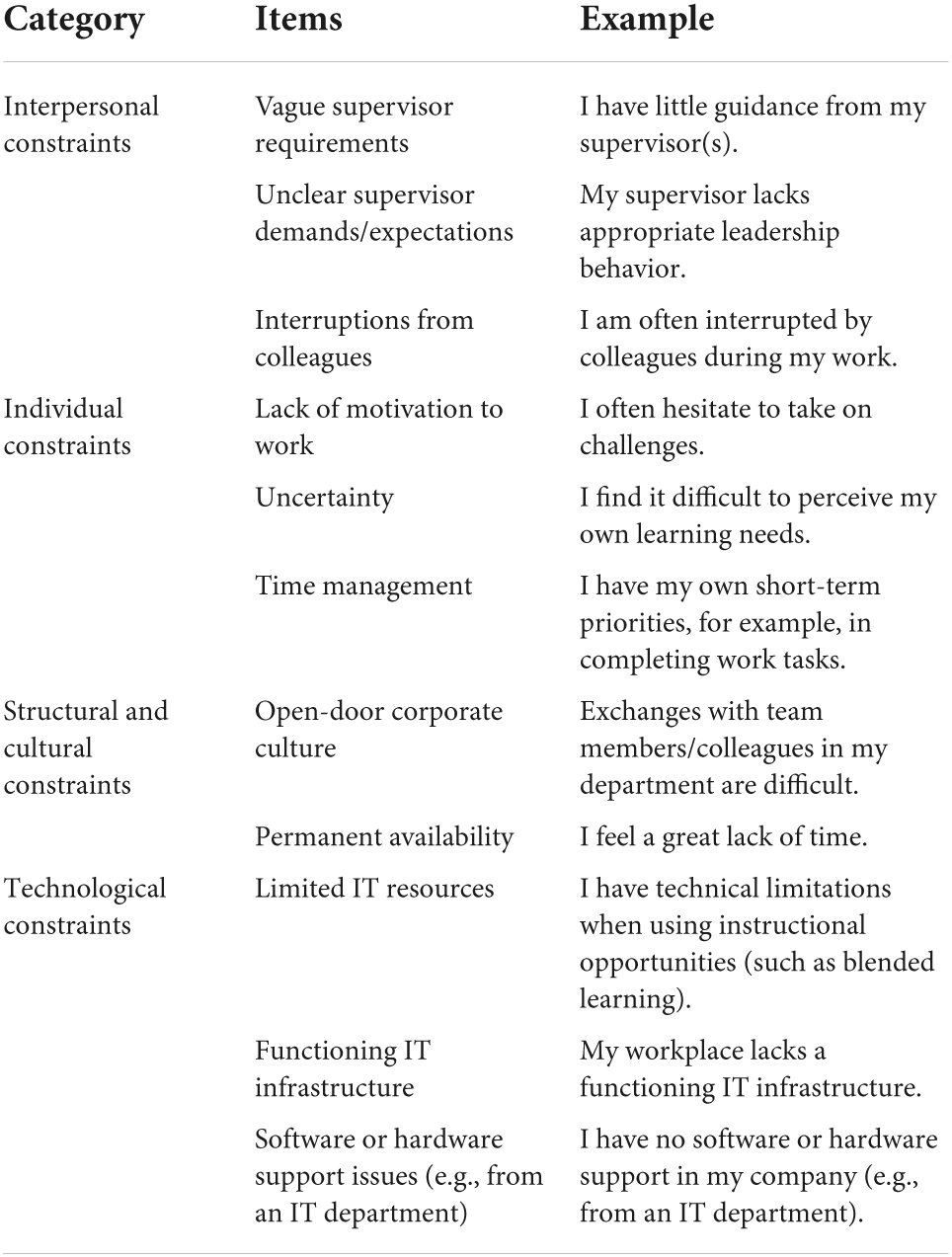
Table 2. Categories items and related examples (Anselmann, in press).
Existing instruments
As a starting point an intensive literature review to find relevant measurement instruments was conducted. From the review, a study on learning and transfer to learning within organizations by Belling et al. (2004) was identified as fitting. In this study, the researchers review quantitative research settings with a focus on managers, thereby taking the individual characteristics of the learners and the workplace’s conditions and facilities into account in their measurement instrument.
Accordingly, 26 items on perceived barriers and 17 items measuring facilitators linked to the transfer of learning from Belling et al.’s (2004) instrument were used to construct the newly questionnaire. The new developed questionnaire referred to a number of common barriers like lack of support (especially lack of managerial support), missing criteria for a clear organizational structure and hierarchy, mechanism of workplace curricular and hidden agendas, and pressure to work with limited resources and knowledge. The items used for the new measurement instrument also feature topics such as pressure to give priority to “bottom line,” short-term, financial targets; lack of resources to implement new ideas/plans from the program; and too many changes in the workforce. The items were rephrased and embedded to fit the overall concept of the questionnaire.
Another source that has been taken into account has been Crouse et al. (2011). Their research focused on factors that hindering learning at the workplace in a qualitative study. The study highlights learning strategies, barriers, facilitators and potential outcomes of learning activities. From this study, 46 potential barriers that can be divided into nine categories of hindering factors (source constraints, lack of access, technological constraints, personal constraints, interpersonal constraints, structural and cultural constraints, course/learning content and delivery, power relationships and change) were used. Examples of these barriers are no managerial commitment to learning (structural and cultural constraints), cynicism/reluctance toward learning (personal constraints) and limited decision-making power in organizational affairs (power relations). The identified areas were reformulated into items and optimized for the new questionnaire context.
Preliminary questionnaire
Based on the described framework (internal barriers, external barriers and fitting problems), the results of the interview study (Anselmann, in press) and existing research (Belling et al., 2004; Crouse et al., 2011), an instrument to measure learning barriers was developed. The instrument includes scales regarding informal learning, formal learning and both. The questionnaire consists of 89 items measured on a 5-point Likert scale (1 = totally disagree, 5 = totally agree). Following the arguments of Babakus and Mangold (1992); Dawes (2008) and Saleh and Bista (2017), a 5-point Likert scale was preferred for three reasons: (1) to increase the response rate, (2) to increase the quality within the responses, especially for longer sections, and (3) to reduce participant frustration. The questionnaire features structural and organizational relations, as well as individual components. The nine categories cover resource constraints (10 items, item example: “In my organization, there are financial constraints such as a continuing education budget to attend continuing education.”), insufficient access (6 items, item example: “Because of my educational qualifications, I do not have access to continuing education opportunities.”), individual components (14 items, item example: “I find it difficult to perceive my own learning needs.”), team or interpersonal constraints (10 items, item example: “I am often interrupted by colleagues during my work.”), structural, cultural or organizational constraints (20 items, item example: “In my organization, management does not promote learning opportunities.”), course or learning content and delivery (6 items, item example: “I think in further education or training, I can only gain inappropriate knowledge.”), hierarchical relationships (4 items, item example: “Resistance from others prevents me from participating in continuing education or training.”), change (3 items, item example: “Business goals in my organization change quickly.”), technical limitations (6 items, item example: “I have limited IT resources at my workplace, e.g., lack of software, limited platform access, inadequate hardware.”) and unclear career perspective (10 items, item example: “I can’t assess the career opportunities available to me.”). Furthermore the categories refer to barriers to formal learning (47 items) and barriers to informal learning (42 items).
Validation of the measurement instrument
Method
The validation of the before described measurement instrument follows the concept of Hinkin (1998); Moosbrugger and Schermelleh-Engel (2012) and Petri et al. (2015). Their recommendations refer to a set of fundamental requirements for ensuring the quality and validation of the analytical procedure. Aiming to prove that the instrument meet the required standards for newly designed measurements (Cohen et al., 2018). This will cover Kaiser-Meyer-Olkin (KMO) and the Bartlett’s Sphericity Test (BTS) determine the samples ability to run a factor analysis, explorative factor analysis (EFA), taking care of the reliability by using Cronbach’s alpha, using convergent validity to detect correlations within different scales, and finally a confirmatory factor analysis (CFA).
To validate the measurement instrument, an online questionnaire was developed. The questionnaire combined four scales (researchers’ own development; Spector, 1985; Peng, 2013; Decius et al., 2019) and several personal background variables, such as education level, expertise, years in the current position, gender and participation in formal training. The first scale refers to barriers to learning (researchers’ own development) with 89 items. This set of items is the preliminary version of the barriers to learning scale and covers the fields described in 3.1.3. The second scale relates to the informal workplace component scale (IWC) by Decius et al. (2019). Here 24 items cover the wide range of informal workplace learning. The third scale deals with the concept of hiding knowledge within a company (Peng, 2013). 14 items measure why and when employees hide knowledge within daily work situation. The forth scale relies on Spectors’ (1985) job satisfaction survey. Within this scale 38 items are used to identify how satisfied employees are with their working conditions, their career options as well as their supervisors.
The process time of the online questionnaire took the participants around 20 min. The participants were acquired by using social media channels like Linkedin, Xing, or Facebook, as well as relevant groups and blogs. To identify possible participants from consultancy keywords were used, such as “HR consultancy,” “outplacement consultancy,” “recruitment,” “human resource development,” “professional training” and “professional coaching.”
In a next step the consultants were contacted by mail, sending an invitation to the questionnaire, followed by a short primer and a summary of facts. A kind reminder has been send 2 weeks after the first e-mail contact.
Sample
The participants came from the consultancy domain. They are varied in position and jobs, and worked in different fields throughout Germany, such as human resource development (HRD) or HR, recruiting, automotive and strategic management consulting. The sample consisted of 112 participants, of which 58.7% were female and 40.3% male. In sum, 91 permanent employees took part in the survey; the remaining 21 were freelancing professionals. Their workplaces were settled in small and medium sized companies, as well as in nationwide and internal operating enterprises. Their average work experience was 12.29 years with a standard deviation of 9.18 years. The consultants worked in different domains with different backgrounds and showed great variation in educational level, tasks and decision-making authority. Throughout the whole process of data collection, calculation, interpretation and storing, the EU GDPR rules were followed. In addition, a procedure index was established and approved by the researchers’ universities’ data protection officers. Furthermore, the questionnaire, as well as the whole research process was approved by the universities’ ethic committee with a positive vote without constraints.
Data analysis
Based on Hinkin’s (1998) framework for scale validation and in close liaison with Moosbrugger and Schermelleh-Engel (2012), four major methods to determine the scale’s validity were used: exploratory factor analysis (EFA; oblique rotation), an internal consistency assessment (Cronbach’s alpha and McDonald’s omega), CFA and a convergent validity assessment. To analyze the data SPSS (IBM SPSS Statistics 27) and Mplus (Mplus Version 8.7) were used.
Regarding the EFA, it has been conducted with oblique rotation (Osborne, 2015), based on the assumption of having correlations between the factors. As proposed by Costello and Osborne (2005) the cut off point for items has been a factor loading below 0.40.
Referring to Mondiana et al. (2018) KMO and the BTS determine the samples ability to run a factor analysis. In this sample the values of KMO and BTS both highly indicate this ability. According to Mondiana et al. (2018) the sample is suitable when reaching a KMO over 0.60 and the BTS should be at α < 0.05. Here the KMO is at 0.80 and the BTS is 0.000. This indicated the high quality of the sample.
The measurement has been conducted with Mplus (Mplus Version 8.7). For the CFA SPSS (IBM SPSS Statistics 27) has been used. CFI measurements compare the extent to which the model is better than the assumption that all variables are uncorrelated. Hu and Bentler (1999) recommend values above 0.90. The fit indices were measured by referring to RMSEA. This describes how closely the correlation matrix implied by the model, matches the correlation matrix found in the data. The smaller the RMSEA values, the better the calculated values match those actually found. Hu and Bentler (1999) recommend that the value for RMSEA should be less than 0.05. The correlation analysis relies on Crombach’s alpha and McDonald’s omega, using SPSS (IBM SPSS Statistics 27). The internal consistency of a scale as a measure of reliability is usually indicated by Cronbach’s alpha. Increasingly, however, the coefficient McDonald’s Omega is appearing for this purpose.
Within the measurement, data cleaning has been applied, using EDC-technique for data cleaning (Krishnamoorthy et al., 2014). The results show that there are less than 1% of missing data. In these cases, single imputation via regression provided by SPSS (Weaver and Maxwell, 2014) has been used.
Results
Exploratory factor analysis
The EFA was using the preliminary questionnaire, which contained 89 items within 10 factors divided into formal and informal barriers. This step aims to reduce the number of items and factors, and thus conducted several more EFA rounds. The final EFA resulted in a suitable and practical questionnaire for barriers at the workplace. The results are presented in Table 3, yielded a three-factor scale for the barriers to formal learning (individual, organizational/structural/hierarchical and technical barriers) and a two-factor scale for the barriers to informal learning (individual and structural barriers).
In addition to the factor loadings, the average variance extracted (AVE) has been calculated using SPSS 27 and Excel. The AVE is a measure of the quality of how a single latent variable explains its indicators. Here, the variance of each indicator is decomposed into the variance, the squared regression coefficient, explained by the latent variable, and an error not explained by the construct. For this factor the AVE is 0.61. Scores above 0.50 are regarded suitable (dos Santos and Cirillo, 2021). Furthermore the calculated composite reliability is at 0.93. Composite reliability can be used as an additional measurement to assess the internal consistency of items within one factor. Values above 0.70 are regarded as necessary for a suitable construct (Kalkbrenner, 2021).
According to Ahire and Devaraj (2001) different statistical methods can be used to estimate different types of validity. As suggested by many authors (Brown, 2015; Muthén and Muthén, 2017; Kyriazos, 2018a) it is an adequate way for confirm the EFA-structure with the CFA-method. CFA could also be used to assess discriminant validity. For factors measuring barriers to formal learning (individual components AVE 0.61, CR 0.93/technical limitations AVE 0.72, CR 0.88/organizational limitations and power relations AVE 0.60, CR 0.82) and for factors measuring barriers to informal learning (individual components AVE 0.61; CR 0.70; structural components AVE 0.68, CR 0.88) average variance reliability and composite reliability has been estimated and checked for the intercorrelation of factor loadings. For all factors estimates are acceptable and there are no greater correlations as estimates of AVE. Tables 4, 5 give an overview about the measuring and the values.
Based on the results, the measurement instrument is formed of the five factors: individual barriers (15 items on motivation or fears), organizational/structural barriers (21 items on hierarchy, team climate and leadership), technical barriers (5 items on technical conditions), change (4 items on turnover intention) and uncertainty (5 items on career options).
Internal consistency assessment
The internal consistency assessment revealed satisfying Cronbach’s alpha and McDonald’s omega results for all scales (α = 0.80–0.86; ω = 0.77–0.93). Cronbach’s alpha results up from 0.70 are regarded acceptable, while results up from 0.80 are interpreted as satisfying or good (Warrens, 2016). McDonald’s omega results are regarded as comparable to the scale used for Cronbach’s alpha (Şimşek and Noyan, 2013). Therefore, values above 0.70 are regarded acceptable, while results up from 0.80 are interpreted as satisfying or good and values above 0.90 refer to a highly reliable. Table 6 lists the scales, item examples and their Cronbach’s alpha values, mean and standard deviation.
Confirmatory factor analysis
The CFA was conducted, and the result is presented in Table 7. The measurement model for the barrier scale for formal learning show the following fit indices: χ2 = 324.243; df = 234; χ2/df = 1.06; CFI = 0.90; RMSEA = 0.06; and TLI = 0.88. While, the fit indices of the measurement model for the barrier scale for informal learning are χ2 = 210.027; df = 144; χ2/df = 1.02; CFI = 0.92; RMSEA = 0.065; and TLI = 0.89.
As stated, the CFI measurements compare the extent to which the model is better than the assumption that all variables are uncorrelated. Hu and Bentler (1999) recommend values above 0.90. The fit indices were measured by referring to RMSEA. This describes how closely the correlation matrix implied by the model matches the correlation matrix found in the data. The smaller the RMSEA values, the better the calculated values match those actually found. Hu and Bentler (1999) recommend that the value for RMSEA should be less than 0.05. Referring to Kyriazos (2018b) the models goodness of fit is more than acceptable. This is underpinned by RMSEA = 0.06 for formal barriers to learning and a RMSEA = 0.065 for barriers to informal learning.
Figures 1, 2 give an overview about the measurement model of barriers to formal learning and to informal learning.
The measurement model of barriers to learning consists of formal barriers to learning with its factors individual components formal setting (IC_F), organizational limitations and power relations (OL) and technical limitations (TL), as well as of informal barriers to learning with its factors individual components informal setting (IC_IF) and structural components (SC). The measurement model for the barrier scale for formal learning have the following fit indices: χ2 = 324.243; df = 234; χ2/df = 1.06; CFI = 0.90; RMSEA = 0.06; and TLI = 0.88. The fit indices of the measurement model for the barrier scale for informal learning are χ2 = 210.027; df = 144; χ2/df = 1.02; CFI = 0.92; RMSEA = 0.065; and TLI = 0.89.
Convergent validity
The assessment of the convergent validity was quite difficult, because there are no instruments comparable to the newly designed measurement of barriers to learning. Therefore, it was compared to the IWC (Decius et al., 2019), hiding knowledge (Peng, 2013) and job satisfaction survey (Spector, 1985). There were negative correlations (e.g., individual barriers and intrinsic intent to learn, β = −0.19), and positive ones with hiding knowledge; for example, the individual components of both formal (0.282) and informal barriers to learning (0.279). Referring to Amora (2021) the cut-off point is at 0.50. This is not an uncommon situation when testing a new instrument with no comparable measurements on hands. To face this situation an AVE has been calculated using SPSS 27 and Excel. The scores showed acceptable values as previously indicated (Kalkbrenner, 2021).
Discussion
The purpose of this study was to develop and validate a measurement instrument of barriers to informal and formal learning at the workplace. Based on the results of the pre-study (Author, under review) and existing instruments (Belling et al., 2004; Crouse et al., 2011), an instrument that measures barriers to both informal and formal learning was developed.
The scale was validated through a cross-sectional questionnaire with 112 consultancy employees and freelancers. The results lead to the development of instrument formed of five factors; three-factor scale measuring barriers to formal learning and a two-factor scale measuring barriers to informal learning. The five factors are: individual barriers (15 items on motivation or fears), organizational/structural barriers (21 items on hierarchy, team climate, and leadership), technical barriers (5 items on technical conditions), change (4 items on turnover intention) and uncertainty (5 items on career options). The Cronbach’s alpha values for all factors ranged between 0.80 and 0.86.
General reflection on the study
As stated, the steps undertaken rely on Hinkin’s (1998) framework for scale validation and are in line with Moosbrugger and Schermelleh-Engel (2012). Firstly, the results of the exploratory factor analysis (EFA; oblique rotation), were used to reduce the number of items significantly. By using EFA the multiple manifest variables, here items of the questionnaire on barriers to learning can be used to draw conclusions about underlying latent variables and factors. As concluded by Nguyen and Waller (2022) an EFA leads to a reduction of variables to a few underlying factors of the manifest variables. This meant reducing the number of items from 89 items and 10 factors to 44 items and five factors.
Secondly, an internal consistency assessment (Cronbach’s alpha and McDonald’s omega), has been carried out. This assessment refers to the precision or accuracy of a measurement (Marcial and Launer, 2021). This indirectly addresses two points. The reliability as the measurement accuracy of a scale without regard to the content, and a reliability as the determination of the measurement error with which the individual values are afflicted, without regard to whether the scale also measures what it purports to measure (validity). With Cronbach’s alpha results for all scales from 0.80 to 0.86 the overall accuracy of the measurement can be regarded as satisfying (Warrens, 2016).
Thirdly a CFA was conducted. Keeping in mind that here the CFA was used to test the structure and functioning of the barriers to learning measurement instrument. The fit indices of the measurement model show a more than acceptable fit, for barriers to formal learning as well as to informal learning (Brown and Moore, 2012).
Fourthly convergent validity assessment was carried out. This testing reveals weather a scale shows correlations with comparable scales or instruments (Cheah et al., 2018). For this newly designed instrument the IWC (Decius et al., 2019), hiding knowledge (Peng, 2013) were used successfully. Going in line with Messick (1995), the validation process is not a static procedure. After successfully developing and testing a new instrument, the next consequent step is the actual implementation of the instrument in research. Followed by adequate interpretation of the results from it.
Limitations of the study
According to Lambriex-Schmitz et al. (2020) every validation study “has several limitations that should be addressed in future research and questionnaire validity testing” (Lambriex-Schmitz et al., 2020, p. 334). Since the instrument is based on an explorative interview study (Anselmann, in press) and can be seen as an innovative measurement instrument, completely comparable instruments are not available. This could be seen as a limitation to the instrument’s convergent validity, so the instrument will be used in a follow-up study in which its validity is analyzed. Furthermore, the instrument was tested in the consultancy domain. However, it is not limited to this field, it should therefore be applied in other fields with knowledge-intensive service works (Korster, 2022) or even domains in the blue-collar sector (Decius et al., 2021).
Another limitation of this work can be seen by the small sample size of 112 participants from the domain of consultancy. The problem with a sample size that is regarded as small is a lack of statistical power. Although Muthén and Muthén (2017) would recommend a sample size from at least 150, for confirmatory factor analyses or EFA, this threshold is much bound on the quality of the data. With small samples, estimation problems are more likely to occur in CFA or EFA and estimation problems occur more frequently. But this likeness is strongly bound to missing values, a lack of power, as well as on scales with low numbers regarding the consistency assessment, e.g., values of Cronbach’s alpha below 0.70 (Kyriazos, 2018b). Within this instrument for measuring barriers to learning none of these weaknesses apply. In the used sample for the validation there are no missing values. Only complete samples have been used for the development. There are no estimated values within the whole dataset. The Cronbach’s alpha results are consistent at a level at 0.80 or higher. These values are interpreted as good to satisfying, according to Warrens (2016). In addition Marsh et al. (1998) related factor sizes and sample sizes. Showing that more items per factor more often lead to reliable results. Predicting more accurate and more stable parameter estimation, and fewer less non-convergent solutions and more reliable factors. Other they propose a sample size larger than 100 to run CFA or EFA correctly. Although, 112 participants in a validation study seems not high (Cohen et al., 2018) the conducted measurements all indicated more than satisfying values. The statistical power and the significance could be proven.
The nature of an explorative study itself can also be a limitation, but given the fact that there are always upcoming fields of research, an explorative study like this can provide the very first insight into unexplored concepts and highlight paths for new research with the goal of obtaining insights into barriers to learning in the workplace. Further studies can thus determine any patterns regarding characteristics and barriers in informal workplaces specifically.
Implication of the study
As claimed by Billett (2022) and Harteis (2022) research on workplace learning needs to be more innovative and therefore has to explore more, so far unconventional aspects of learning at the workplace. This research-based measurement combines barriers to learning on various levels and hierarchies. This includes barriers related to resource constraints, structural, cultural or organizational constraints, insufficient access, individual components, as well as team or interpersonal constraints, furthermore hierarchical relationships, barriers to learning rooting in unclear career perspectives, organizational changes, and finally technical limitations. This broad range of detecting barriers to leaning are summarized in five factors: individual barriers (15 items on motivation or fears), organizational/structural barriers (21 items on hierarchy, team climate and leadership), technical barriers (5 items on technical conditions), change (4 items on turnover intention) and uncertainty (5 items on career options). Referring on individual, team and organizational level.
This is a unique approach to tackle the highly complex system of barriers to learning within the workplace. However, further research has yet to shed more light on the complex relationship between learning barriers and learning activities. It can be assumed that learning barriers at different levels have different influences on various learning activities, rendering it very important to get a closer look at these relations, especially because individuals prefer different learning scenarios at the workplace. This emphasis shows that barriers to learning form a continuum based on individual learning experiences, situations and roles. So, to better understand learning activities, it is necessary to reflect on facilitators and barriers equally. Therefore, this validated instrument will be used for further studies on barriers to learning at the workplace. It will be deployed to identify the holistic concept of barriers to learning at the workplace, within individual, team and organizational level. In addition, this measurement is used to identify the role multiple additional factors like formal and informal learning activities (Brion, 2021), toxic leadership (Schmidt, 2008), competitive work environment (Fletcher and Nusbaum, 2010), and the degree of digitalization of the workplace (Görs et al., 2019).
Practical value of the study
This measurement instrument is appropriate to determine what hinders individuals from learning in their organizations. In line with Dolata et al. (2021) The field of consultancy is a challenging work environment with highly complex tasks (O’Leary, 2020) and with a constant need for professional development. While studies often focus on the diverse facilitating factors for learning (Jeong et al., 2018), less is known about how to determine what hinders individuals from learning at the workplace. Therefore, this instrument can be used in research as well as organizations to find out what hinders employees’ learning. It leads to the question of what is not working well and should be changed, like miscommunication, poor work environment and lack of autonomy (Brion, 2021; Decius et al., 2021; Kim et al., 2021). This instrument also features the most relevant factors that can be barriers to learning on all levels (e.g., leadership on the organizational level, team climate on the team level and fear on the individual level). It can therefore serve as even the starting point for justified and established steps in organizational development.
This validation study, as well as the following main study (Anselmann, in prep.) highlights the very importance of identifying, reflecting and tackling barriers to learning at the workplace. Apart from the academic discourse in journals and conference presentations, this theme is distributed in more practical terms to the professionals in the field. Therefore, three major steps have been initiated. Firstly, HR professionals and companies that took part in the interview study (Anselmann, in press), have been updated and informed throughout the process about barriers to learning and first implications. Small workshops and feedback loops have been carried out. Secondly, all the participants of the online questionnaires, the validation of the measurement (N = 112) and the main study on barriers to learning (N = 230) could sign up for a newsletter, providing them with regular information on barriers to learning at the workplace and practical implication. Thirdly, an online workshop is scheduled to bridge academic and research driven approaches with daily work situation in companies. This workshop will contain short but significant inputs on barriers to learning, cases and best practices from companies, presented by HR managers and other researchers, and finally working groups for raising attention to identifying, reflecting and tackling barriers to learning at the workplace.
Data availability statement
The raw data supporting the conclusions of this article will be made available by the author, without undue reservation.
Ethics statement
The studies involving human participants were reviewed and approved by Ethikkommission der PH Schwäbisch Gmünd, Aktenzeichen: EK-2021-14-Anselmann_Sebastian-VK. The patients/participants provided their written informed consent to participate in this study.
Author contributions
The author confirms being the sole contributor of this work and has approved it for publication.
Funding
The article processing charge was funded by the Baden-Württemberg Ministry of Science, Research and Culture and the University of Education Schwäbisch Gmünd in the funding program Open Access Publishing.
Conflict of interest
The author declares that the research was conducted in the absence of any commercial or financial relationships that could be construed as a potential conflict of interest.
Publisher’s note
All claims expressed in this article are solely those of the authors and do not necessarily represent those of their affiliated organizations, or those of the publisher, the editors and the reviewers. Any product that may be evaluated in this article, or claim that may be made by its manufacturer, is not guaranteed or endorsed by the publisher.
References
Ahire, S. L., and Devaraj, S. (2001). An empirical comparison of statistical construct validation approaches. IEEE Trans. Eng. Manag. 48, 319–329. doi: 10.1109/17.946530
Alikaj, A., Ning, W., and Wu, B. (2021). Proactive personality and creative behavior: examining the role of thriving at work and high-involvement HR practices. J. Bus. Psychol. 36, 857–869. doi: 10.1007/s10869-020-09704-5
Allen, C. A., and Heredia, S. C. (2021). Reframing organizational contexts from barriers to levers for teacher learning in science education reform. J. Sci. Teach. Educ. 32, 148–166. doi: 10.1080/1046560X.2020.1794292
Amora, J. T. (2021). Convergent validity assessment in PLS-SEM: a loadings-driven approach. Data Anal. Perspect. J. 2, 1-6.
Anselmann, S. (in press). Trainers’ Learning Conditions, Informal and Formal Learning, and Barriers to Learning.
Anselmann, S. (in prep.). Individual, Structural and Team-Related Barriers to Informal Learning at the Workplace.
Argyris, C., and Schön, D. A. (1996). Organizational Learning II. Theory, Method, and Practice. Wokingham: FT Press.
Babakus, E., and Mangold, W. G. (1992). Adapting the SERVQUAL scale to hospital services: an empirical investigation. Health Serv. Res. 26, 767–786.
Belling, R., James, K., and Ladkin, D. (2004). Back to the workplace: how organisations can improve their support for management learning and development. J. Manag. Dev. 23, 234–255. doi: 10.1108/02621710410524104
Billett, S. (1995). Workplace learning: its potential and limitations. Educ. Train. 37, 20–27. doi: 10.1108/00400919510089103
Billett, S. (2022). “Learning in and through work: positioning the individual,” in Past, the Present, and the Future of Workplace Learning. Research Approaches on Workplace Learning, eds C. Harteis, D. Gjbels, and E Kyndt (Dordrecht: Springer), 157–175. doi: 10.1007/978-3-030-89582-2_7
Boeren, E. (2016). Lifelong Learning Participation in A Changing Policy Context. New York, NY: Palgrave Macmillan. doi: 10.1057/9781137441836
Brion, C. (2021). Culture: the link to learning transfer. Adult Learn. doi: 10.1177/10451595211007926
Brown, T. A. (2015). Confirmatory Factor Analysis for Applied Research, 2nd Edn. New York, NY: The Guilford Press.
Brown, T. A., and Moore, M. T. (2012). “Confirmatory factor analysis,” in Handbook of structural equation modeling, ed. R. H. Hoyle, (361–379). New York, NY: The Guilford Press.
Cerasoli, C. P., Alliger, G. M., Donsbach, J. S., Mathieu, J. E., Tannenbaum, S. I., and Orvis, K. A. (2018). Antecedents and outcomes of informal learning behaviors: a meta-analysis. J. Bus. Psychol. 33, 203–230. doi: 10.1007/s10869-017-9492-y
Cheah, J.-H., Sarstedt, M., Ringle, C. M., Ramayah, T., and Ting, H. (2018). Convergent validity assessment of formatively measured constructs in PLS-SEM: on using single-item versus multi-item measures in redundancy analyses. Int. J. Contemp. Hosp. Manag. 30, 3192–3210. doi: 10.1108/IJCHM-10-2017-0649
Cohen, L., Manion, L., and Morrison, K. (2018). Research Methods in Education, 8th Edn. London: Routledge. doi: 10.4324/9781315456539
Costello, A. B., and Osborne, J. (2005). Best practices in exploratory factor analysis: four recommendations for getting the most from your analysis. Pract. Assess. Res. Eval. 10, 1–9. doi: 10.7275/jyj1-4868
Crouse, P., Doyle, W., and Young, J. D. (2011). Workplace learning strategies, barriers, facilitators and outcomes: a qualitative study among human resource management practitioners. Hum. Resour. Dev. Int. 14, 39–55. doi: 10.1080/13678868.2011.542897
Dawes, J. (2008). Do data characteristics change according to the number of scale points used? An experiment using 5-point, 7-point and 10-point scales. Int. J. Mark. Res. 50, 61–104. doi: 10.1177/147078530805000106
Decius, J., Schaper, N., and Seifert, A. (2019). Informal workplace learning: development and validation of a measure. Hum. Resour. Dev. Q. 30, 495–535.
Decius, J., Schaper, N., and Seifert, A. (2021). Work characteristics or workers’ characteristics? An input-process-output perspective on informal workplace learning of blue-collar workers. Vocat. Learn. 14, 285–326. doi: 10.1007/s12186-021-09265-5
Dolata, M., Schenk, B., Fuhrer, J., Martin, A., and Schwabe, G. (2021). When the system does not fit: coping strategies of employment consultants. Comput. Support. Coop. Work 29, 657–696. doi: 10.1007/s10606-020-09377-x
Doornbos, A., Simons, R.-J., and Denesse, E. (2008). Relations between characteristics of workplace practices and types of informal work-related learning: a survey study among Dutch police. Hum. Resour. Dev. Q. 19, 129–151. doi: 10.1002/hrdq.1231
dos Santos, P. M., and Cirillo, M. Â (2021). Construction of the average variance extracted index for construct validation in structural equation models with adaptive regressions. Commun. Stat. Simul. Comput. doi: 10.1080/03610918.2021.1888122
Dymock, D., and Tyler, M. (2018). Towards a more systematic approach to continuing professional development in vocational education and training. Stud. Contin. Educ. 40, 198–211. doi: 10.1080/0158037X.2018.1449102
Eken, G., Bilgin, G., Dikmen, I., and Birgonul, M. T. (2020). A lessons-learned tool for organizational learning in construction. Autom. Constr. 110:102977. doi: 10.1016/j.autcon.2019.102977
Fletcher, T. D., and Nusbaum, D. N. (2010). Development of the competitive work environment scale: a multidimensional climate construct. Educ. Psychol. Measur. 70, 105–124. doi: 10.1177/0013164409344492
Goller, M., and Paloniemi, S. (2022). “Agency: taking stock of workplace learning research,” in Research Approaches on Workplace Learning. Insights from a Growing Field, eds C. Harteis, D. Gijbels, and E. Kyndt (Berlin: Springer), 3–28. doi: 10.1007/978-3-030-89582-2_1
Görs, P. K., Hummert, H., and Traum, A. and Nerdinger, F. W. (2019). Studien zur Validierung der Skala zur Erfassung des organisationalen Digitalisierungsgrades (ODG). Rostocker Beiträge zur Wirtschafts- und Organisationspsychologie, Nr. 21. Rostock: Universität Rostock.
Hager, P. (2019). “VET, HRD, and workplace learning: Where to from here?,” in The Wiley handbook of vocational education and training, eds D. Guile and L. Unwin (Hoboken, NJ: Wiley). doi: 10.1002/9781119098713.ch4
Harteis, C. (2022). “Research on Workplace Learning in Times of Digitalisation,” in Research Approaches on Workplace Learning. Insights from a Growing Field, eds C. Harteis, D. Gijbels, and E. Kyndt (Berlin: Springer), 415–428. doi: 10.1007/978-3-030-89582-2_19
He, X., and Yang, H. H. (2021). “Technological barriers and learning outcomes in online courses during the Covid-19 pandemic,” in ICBL - Blended Learning: Re-Thinking and Re-Defining the Learning Process, eds R. Li, S. K. S. Cheung, C. Iwasaki, L. F. Kwok, and M. Kageto (Cham: Springer Nature). doi: 10.1007/978-3-030-80504-3_8
Helens-Hart, R., and Engstrom, C. (2021). Empathy as an essential skill for talent development consultants. J. Workplace Learn. 33, 245–258. doi: 10.1108/JWL-06-2020-0098
Hinkin, T. R. (1998). A brief tutorial on the development of measures for use in survey questionnaires. Organ. Res. Methods 1, 104–121. doi: 10.1177/109442819800100106
Hu, L.-T., and Bentler, P. M. (1999). Cutoff criteria for fit indexes in covariance structure analysis: conventional criteria versus new alternatives. Struct. Equ. Model. 6, 1–55. doi: 10.1080/10705519909540118
Jacobs, R. L., and Park, Y. (2009). A proposed conceptional framework of workplace learning: implications for theory development and research in human resource development. Hum. Resour. Dev. Rev. 8, 133–150. doi: 10.1177/1534484309334269
Jeong, S., Han, S. J., Lee, J., Sunalai, S., and Yoon, S. W. (2018). Integrative literature review on informal learning: antecedents, conceptualizations, and future directions. Hum. Resour. Dev. Rev. 17, 128–152. doi: 10.1177/1534484318772242
Johnson, S., Robertson, I., and Cooper, C. L. (2018). “Well-being and employee engagement,” in Well-Being, eds S. Johnson, I. Robertson, and C. L. Cooper (New Jersey: Palgrave Macmillan).
Jordan, B. (2014). Technology and social interaction: notes on the achievement of authoritative knowledge in complex settings. Talent Dev. Excell. 6, 95–132.
Kalkbrenner, M. T. (2021). Alpha, omega, and H internal consistency reliability estimates: reviewing these options and when to use them. Couns. Outcome Res. Eval. doi: 10.1080/21501378.2021.1940118
Kankaraš, M. (2021). Workplace Learning: Determinants and consequences: Insights from the 2019 European Company Survey. Brussels: Publications Office of the European Union. doi: 10.2801/111971
Keck Frei, A., Kocher, M., and Bieri Buschor, C. (2021). Second-career teachers’ workplace learning and learning at university. J. Workplace Learn. 33, 348–360. doi: 10.1108/JWL-07-2020-0121
Kezar, A. J., and Holcombe, E. M. (2020). Barriers to organizational learning in a multi-institutional initiative. High. Educ. 79, 1119–1138. doi: 10.1007/s10734-019-00459-4
Kim, A., Shin, J., Kim, Y., and Moon, J. (2021). The impact of group diversity and structure on individual negative workplace gossip. Hum. Perform. 34, 67–83. doi: 10.1080/08959285.2020.1867144
Korster, F. (2022). Organizations in the knowledge economy. An investigation of knowledge-intensive work practices across 28 European countries. J. Adv. Manage. Res. [Epub ahead of print]. doi: 10.1108/JAMR-05-2021-0176
Krishnamoorthy, R., Kumar, S. S., and Neelagund, B. (2014). “A new approach for data cleaning process,” in International Conference on Recent Advances and Innovations in Engineering (ICRAIE-2014), (Jaipur: IEEE), 1–5. doi: 10.1109/ICRAIE.2014.6909249
Kyndt, E., Govaerts, N., Smet, K., and Dochy, F. (2018). “Antecedents of informal workplace learning: A theoretical study,” in Informal Learning At Work, eds G. Messmann, M. Segers, and F. Dochy (London: Routledge). doi: 10.4324/9781315441962-2
Kyriazos, T. A. (2018a). Applied psychometrics: the 3-faced construct validation method, a routine for evaluating a factor structure. Psychology 9, 2044–2072. doi: 10.4236/psych.2018.98117
Kyriazos, T. A. (2018b). Applied psychometrics: sample size and sample power considerations in factor analysis (EFA, CFA) and SEM in general. Psychology 9, 2207–2230. doi: 10.4236/psych.2018.98126
Lambriex-Schmitz, P., Van der Klink, M. R., Beausaert, S., Bijker, M., and Segers, M. (2020). Towards successful innovations in education: development and validation of a multi-dimensional Innovative Work Behaviour Instrument. Vocat. Learn. 13, 313–340. doi: 10.1007/s12186-020-09242-4
Lecat, A., Spaltman, Y., Beausaert, S., Raemdonck, I., and Kyndt, E. (2020). Two decennia of research on teachers’ informal learning: a literature review on definitions and measures. Educ. Res. Rev. 30:100324. doi: 10.1016/j.edurev.2020.100324
Louws, M. L., Meirink, J. A., van Veen, K., and van Driel, J. H. (2017). Exploring the relation between teachers’ perceptions of workplace conditions and their professional learning goals. Prof. Dev. Educ. 43, 770–788. doi: 10.1080/19415257.2016.1251486
Marcial, D. E., and Launer, M. A. (2021). Test-retest Reliability and Internal Consistency of the Survey Questionnaire on Digital Trust in the Workplace. Solid State Technol. 64, 4369–4381.
Marsh, H. W., Hau, K.-T., Balla, J. R., and Grayson, D. (1998). Is more ever too much? The num ber of indicators per factor in confirmatory factor analysis.Multivariate Behav. Res. 33, 181–220. doi: 10.1207/s15327906mbr3302_1
Marsick, V. J., and Watkins, K. (2015). Informal and Incidental Learning in the Workplace. London: Routledge. doi: 10.4324/9781315715926
Marsick, V. J., and Watkins, K. E. (2001). Informal and incidental learning. New Dir. Adult Contin. Educ. 89, 25–34. doi: 10.1002/ace.5
Matusik, J. G., Ferris, D. L., and Johnson, R. E. (2022). The PCMT model of organizational support: an integrative review and reconciliation of the organizational support literature. J. Appl. Psychol. 107, 329–345. doi: 10.1037/apl0000922
Messick, S. (1995). Validity of psychological assessment: validation of inferences from persons’ responses and performances as scientific inquiry into score meaning. Am. Psychol. 50, 741–749. doi: 10.1037/0003-066X.50.9.741
Mishra, S. (2020). Social networks, social capital, social support and academic success in higher education: a systematic review with a special focus on “underrepresented” students. Educ. Res. Rev. 29:100307. doi: 10.1016/j.edurev.2019.100307
Mondiana, Y. Q., Pramoedyo, H., and Sumarminingsih, E. (2018). Structural equation modeling on Likert scale data with transformation by successive interval method and with no transformation. Int. J. Sci. Res. Publ. 8, 398–405. doi: 10.29322/IJSRP.8.5.2018.p7751
Moosbrugger, H., and Schermelleh-Engel, K. (2012). “Exploratorische (EFA) und konfirmatorische Faktorenanalyse (CFA),” in Testtheorie und Fragebogenkonstruktion, eds H. Moosbrugger and A. Kelava (Cham: Springer Nature). doi: 10.1007/978-3-642-20072-4
Muthén, L. K., and Muthén, B. O. (2017). Mplus User’s Guide, 8th Edn. Los Angeles, CA: Muthén & Muthén.
Neaman, A., and Marsick, V. J. (2018). “Integrating learning into work: Design the context, not just the technology,” in Computer-Mediated Learning for Workforce Development, ed. D. Mentor (Hershey: IGI Global). doi: 10.4018/978-1-5225-4111-0.ch001
Nel, J. H., and Linde, B. (2019). The Art of Engaging Unionised Employees. Singapore: Palgrave Pivot. doi: 10.1007/978-981-13-2197-9
Nguyen, H. V., and Waller, N. G. (2022). Local minima and factor rotations in exploratory factor analysis. Psychol. Methods. [Epub ahead of print]. doi: 10.1037/met0000467
Nouwen, W., Clycq, N., Struyf, A., and Donche, V. (2022). The role of work-based learning for student engagement in vocational education and training: an application of the self-system model of motivational development. Eur. J. Psychol. Educ. doi: 10.1007/s10212-021-00561-1
O’Leary, D. (2020). “Changing nature of public affairs agencies: The role of thought leadership,” in Lobbying the European Union: Changing Minds, Changing Times, eds P. A. Shotton and P. G. Nixon (London: Routledge). doi: 10.4324/9781003075097
Osborne, J. W. (2015). What is Rotating in Exploratory Factor Analysis? Pract. Assess. Res. Eval. 20, 1–7. doi: 10.7275/hb2g-m060
Papacharalampous, N., and Papadimitriou, D. (2021). Perceived corporate social responsibility and affective commitment: the mediating role of psychological capital and the impact of employee participation. Hum. Resour. Dev. Q. 32, 251–272. doi: 10.1002/hrdq.21426
Peng, H. (2013). Why and when do people hide knowledge? J. Knowl. Manag. 17, 398–415. doi: 10.1108/JKM-12-2012-0380
Petri, D., Mari, L., and Carbone, P. (2015). A structured methodology for measurement development. IEEE Trans. Instr. Meas. 64, 2367–2379. doi: 10.1109/TIM.2015.2399023
Piscitelli, A. M. (2021). Overcoming Learning Anxiety in Workplace Learning: A Study of Best Practices and Training Accommodations That Improve Workplace Learning (Publication No. 4169). Ph.D. thesis. Fayetteville, AR: University of Arkansas.
Rodriguez-Gomez, D., Ion, G., Mercader, C., and López-Crespo, S. (2020). Factors promoting informal and formal learning strategies among school leaders. Stud. Contin. Educ. 42, 240–255. doi: 10.1080/0158037X.2019.1600492
Saleh, A., and Bista, K. (2017). Examining factors impacting online survey response rates in educational research: perceptions of graduate students. J. Multidiscip. Eval. 13, 63–74.
Schmidt, A. A. (2008). Development and Validation of the Toxic Leadership Scale. Ph.D. thesis. College Park, MD: University of Maryland.
Segers, M., Endedijk, M., and David, G. (2021). “From classic perspectives on learning to current views on learning,” in Theories of Workplace Learning in Changing Times, eds F. Dochy, D. Gijbels, M. Segers, and P. van den Bossche (London: Routledge). doi: 10.4324/9781003187790-2
Shuck, B. (2019). “Does my engagement matter?,” in The Oxford Handbook of Meaningful Work, eds R. Yeoman, C. Bailey, A. Madden, and M. Thompson (Oxford: Oxford University Press). doi: 10.1093/oxfordhb/9780198788232.013.20
Shuck, B., and Herd, A. M. (2012). Employee engagement and leadership: exploring the convergence of two frameworks and implications for leadership development in HRD. Hum. Resour. Dev. Rev. 11, 156–181. doi: 10.1177/1534484312438211
Simons, P. R., and Ruijters, M. C. (2004). “Learning professionals: Towards an integrated model,” in Professional Learning: Gaps and Transitions On the Way From Novice to Expert, eds H. P. Boshuizen, R. Bromme, and H. Gruber (Dordrecht: Springer).
Şimşek, G. G. and Noyan, F. (2013). McDonald’s ωt, Cronbach’s α, and Generalized θ for Composite Reliability of Common Factors Structures. Commun. Stat. Simul. Comput. 42, 2008–2025. doi: 10.1080/03610918.2012.689062
Skule, S. (2004). Learning conditions at work: a framework to understand and assess informal learning in the workplace. Int. J. Train. Dev. 8, 8–20. doi: 10.1111/j.1360-3736.2004.00192.x
Spector, P. E. (1985). Measurement of human service staff satisfaction: development of the job satisfaction survey. Am. J. Community Psychol. 13, 693–713. doi: 10.1007/BF00929796
Streumer, J. N., and Kho, M. (2006). “The world of work-related learning,” in Work-Related Learning, ed. J. N. Streumer (Dordrecht: Springer). doi: 10.1007/1-4020-3939-5
Tynjälä, P. (2008). Perspectives into learning at the workplace. Educ. Res. Rev. 3, 130–154. doi: 10.1016/j.edurev.2007.12.001
van der Baan, N., Gast, I., Gijselaers, W., and Beausaert, S. (2022). Coaching to prepare students for their school-to-work transition: conceptualizing core coaching competences. Educ. Train. 64, 398–415. doi: 10.1108/ET-11-2020-0341
Warrens, M. J. (2016). A comparison of reliability coefficients for psychometric tests that consist of two parts. Adv. Data Anal. Classif. 10, 71–84. doi: 10.1007/s11634-015-0198-6
Weaver, B., and Maxwell, H. (2014). Exploratory factor analysis and reliability analysis with missing data: a simple method for SPSS users. Quant. Methods Psychol. 10, 143–152. doi: 10.20982/tqmp.10.2.p143
Keywords: barriers to learning, validation of a measurement, learning at work, informal learning, formal learning
Citation: Anselmann S (2022) Learning barriers at the workplace: Development and validation of a measurement instrument. Front. Educ. 7:880778. doi: 10.3389/feduc.2022.880778
Received: 21 February 2022; Accepted: 27 June 2022;
Published: 15 July 2022.
Edited by:
Jesús-Nicasio García-Sánchez, Universidad de León, SpainReviewed by:
Assad Ali Rezigalla, University of Bisha, Saudi ArabiaRandy Tudy, Commission on Higher Education, Philippines
Elina Paliichuk, Borys Grinchenko Kyiv University, Ukraine
Copyright © 2022 Anselmann. This is an open-access article distributed under the terms of the Creative Commons Attribution License (CC BY). The use, distribution or reproduction in other forums is permitted, provided the original author(s) and the copyright owner(s) are credited and that the original publication in this journal is cited, in accordance with accepted academic practice. No use, distribution or reproduction is permitted which does not comply with these terms.
*Correspondence: Sebastian Anselmann, c2ViYXN0aWFuLmFuc2VsbWFubkBwaC1nbXVlbmQuZGU=