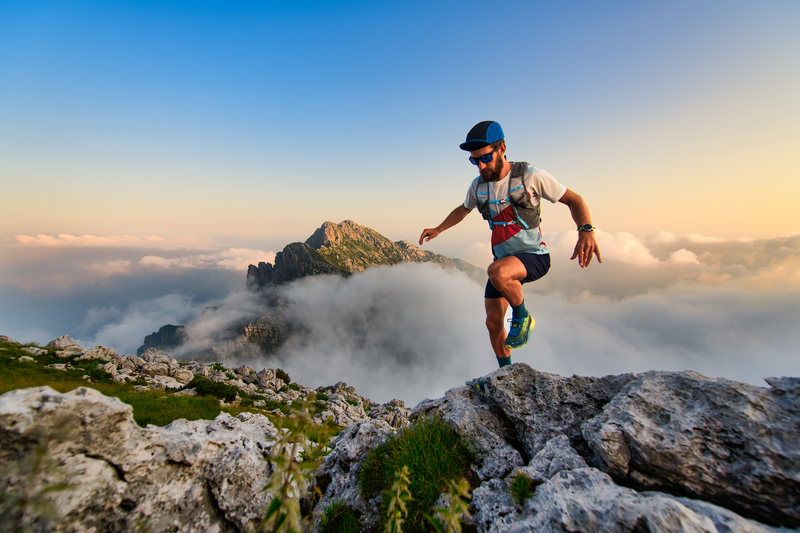
94% of researchers rate our articles as excellent or good
Learn more about the work of our research integrity team to safeguard the quality of each article we publish.
Find out more
ORIGINAL RESEARCH article
Front. Educ. , 27 April 2022
Sec. Higher Education
Volume 7 - 2022 | https://doi.org/10.3389/feduc.2022.868607
This article is part of the Research Topic Pedagogic Innovation and Student Learning in Higher Education: Perceptions, Practices and Challenges View all 23 articles
Objective: The aim of the present work was to investigate the use of verbal language applied to the solution of inverse function problems in comparison to the algebraic method in students of humanities.
Methods: We consider only algebraic functions in our study. Three precalculus problems were chosen and two solution methods used. A total of 120 students participated in this study, half of them used the verbal method and the other half the algebraic method.
Results: To determine if there was a relationship between the utilized method and the effectiveness of the solution, that is the number of exercises solved correctly related to the type of method used, we used Pearson’s chi-square test. We found a significant association between the type of method and the success of the solution χ2 (1) = 10.84, p < 0.001. Regarding the effect size, we found that the probability to get a correct solution was 3.75 times higher when solved with the verbal method in comparison to the algebraic method.
Discussion: We suggest that such efficacy in solving inverse function problems was due to the self-regulation of participant’s language. In this sense, it is necessary to investigate the effects of such self-regulation by means of our experimental paradigm in a future study.
Conclusion: The results of the present study showed that verbal language applied to the solution of inverse function problems is highly effective when compared to the algebraic method.
The fusion between general intellectual and verbal mathematical skills has been on the agenda of mathematics teaching. In particular, several works have described the relationship between the acquisition of mathematical skills and language in various disciplines related to mathematics such as teaching, special education, and the psychology of learning and development (Freudenthal, 1985; Krummheuer, 1994; Maier and Schweiger, 1999; Steinbring, 2000; Bills, 2002; Moreno and Durán, 2004; Krauthausen, 2007; Schütte, 2009; Heinze et al., 2011; Krummheuer, 2012; Pyburn et al., 2013; Vukovic and Lesaux, 2013; Daroczy et al., 2015; Nolte, 2016; Fuchs et al., 2019, 2020; Qolbi et al., 2019). Moreover, recent meta-analyses studies have emphasized the importance of such a relationship (Chow and Jacobs, 2016; Koponen et al., 2017; de Araujo et al., 2018; Peng et al., 2020). In this respect, Peng et al. (2020), found that more complicated language and math skills were associated with stronger relationships between language and math after biasing working memory and intelligence. de Araujo et al. (2018), focused on how English language learners learn mathematics in a multilingual educational setting. These authors suggest that language is a primary means by which English language learners acquire mathematical knowledge. Koponen et al. (2017), showed that rapid automatized naming (RAN; a component of phonological processing that primarily harnesses verbal retrieval skills) is a significant predictor of math success. Chow and Jacobs (2016) targeted the relationship between oral language and fraction scores among school-age students. Based on three studies conducted in the United States, the authors suggested that oral language plays a significant role in fraction performance, and that cognition may partially explain the relationship between oral language and fraction performance.
Austin and Howson (1979) compiled research on language and mathematics dating back to the 1940s. This research began to take hold in the early 1970s. Nearly 40 years later, research within the European Research in Mathematics Education (ERME) still addresses the general themes identified by these authors: the language of the learner, the language of the teacher and the language of mathematics (Planas et al., 2018).
Aiken (1972), considered the effects of verbalization on the learning process of mathematics and analyzed mathematics as a unique language in its own right. Although there is no one-to-one correspondence between the concepts and rules of mathematics and those of native languages, there are many similarities between verbal and mathematical languages (Hickerson, 1959; Capps, 1970). Other researchers consider that, mathematics itself is a special formalized language and therefore should be taught as such (Madden, 1966; Ausubel and Robinson, 1969; Cooper, 1971). However, it is also true that there has been a growth in the diversity and complexity of the domain of this topic as researchers draw on a broader progression of theoretical resources combined in new and multiple ways. One source of diversity is the fact that mathematics and language research considered three main possible approaches. The first adopts language as the object of study, the second uses language as a vehicle to study other phenomena, and the third conceives participation in mathematical communication as learning mathematics itself. All three approaches formulate descriptions of the language in use within a mathematical context but analyze the descriptions in different ways (Planas et al., 2018). A very important contribution to a more adequate description of these topics was the publication of Pimm’s (1987) book, but there is still a need to develop greater rigor in the ways in which we define and distinguish between mathematical language and “everyday” language (Planas et al., 2018). Moreover, the language function hypothesis suggests that language fulfills many functions in our lives, such as exchanging/delivering messages/information, expressing our feelings and attitudes, and informing our thoughts (Bruner, 1966; Vygotsky, 1986; Fetzer and Tiedemann, 2018). In this way, language can lead to the development of other skills such as mathematics. Among all the functions of language, two that are fundamental for the development of mathematics are language as a means of exchange and thought (Bruner, 1966; Fetzer and Tiedemann, 2018). In contrast, the main function of language according to a mathematics hypothesis is that we use language not only as a tool to communicate mathematical knowledge with others, but also to construct and retrieve representations of mathematical knowledge from long-term memory, thus implying a causal relationship between language and later mathematical development (Bruner, 1966; Dehaene, 1992; Dehaene and Cohen, 1995; Gersten et al., 2009; LeFevre et al., 2010; Fetzer and Tiedemann, 2018). Likewise, the language thinking function hypothesis for mathematics recognizes language not only as a means of doing/learning mathematics but also emphasizes its role in cognition (Vygotsky, 1986; Daneman and Merikle, 1996; Rieber, 1997; Lombrozo, 2006; Peng et al., 2018). That is, we use language to think about more abstract mathematical concepts and the relationships between them, such as when we build a diagram and an equation when solving word problems.
Many psychologists have considered the importance of language development for mathematical ability (Piaget, 1954; Bruner, 1966). Piaget argued that the growth of language ability follows the development of concrete operational thinking rather than preceding it. Although it recognized that language is important in the realization of such cognitive structures. In contrast, Bruner et al. (1966) argued that the development of appropriate terminology is essential for cognitive growth. Whether language acquisition is a cause or effect of cognitive development (or maybe, as seems more likely, a little of both), needs further investigation (Aiken, 1972). Ausubel and Robinson (1969), argued that there is an interesting analogy in relation to the learning of algebraic symbols and syntax, since the same problems that exist in the learning of a second language are involved.
Studies of the symbolic processes involved in human thought have frequently used mathematical problems. A common procedure is to analyze the thought process by asking the subject to think aloud and registering the tactic or sequence of questions that they use to arrive to the solution. This type of research has revealed that the translation or encoding procedure varies according to the individual (Rimoldi, 1967; Rimoldi et al., 1968).
In any case, understanding the importance of what is specific in mathematical language acquires high relevance by considering the development of theoretical understanding of mathematics itself as a discursive activity. Recognizing the distinctive features of mathematical communication is a key point to any study of mathematical activity, whether one adopts the “strong” discursive position that mathematical objects do not exist independently of the discursive means of communicating them, or a position less absolutist that there is no direct material access to mathematical objects but the experience of them through some form of “representation” or “realization”. These two points of view reflect different ontological positions: speaking of the representation of a mathematical object suggests that an independent object exists, while speaking of realization proposes that communication about an object is what gives the object existence. In either of these positions, mathematical activity involves participation in a form of discourse about real or discursive objects. Understanding such activity involves studying that discourse and its characteristics (Planas et al., 2018).
Currently, the debate continues about the relationship between mathematical ability and language. Some research suggests that common processes underlie arithmetic and grammar, while other research suggests that these are distinct processes (Baldo and Dronkers, 2007). In the last two decades, studies in both psychology and cognitive neuroscience have converged on the view that mathematical and linguistic skills are largely separate (Prado, 2018). However, Baldo and Dronkers (2007), suggest that arithmetic and language comprehension are mediated by partially overlapping brain networks.
Basic numerical skills, rather than language skills, are increasingly believed to be the basis for the emergence of abstract mathematical concepts (Dehaene and Cohen, 2007). However, there seems to be one exception, when it comes to the most fundamental math and language skills: learning arithmetic and learning to read. Studies show that there is a correlation between children’s arithmetic and reading skills (Hecht et al., 2001; Durand et al., 2005; Hart et al., 2009). Importantly, studies have also found that math skills in children can predict later reading outcomes, sometimes even better than early reading skills (Lerkkanen et al., 2005; Duncan et al., 2007).
In a study of verbalization made by students establishing equations for algebra problems, Paige and Simon (1966) were able to classify subjects as “physical” and “verbal” thinkers. The “physical” thinkers constructed internal representation of the situation described by the equation, while “verbal” thinkers constructed literal translations of the words associated to the equation.
In a previous research, Atkinson et al. (2003) combined successive elimination of increasingly elaborate solution steps (which they called fading) with the introduction of prompts designed to encourage students to identify the underlying principle illustrated in each elaborated work. Their results produced medium to large effects on near and far transfer without requiring additional time on the task. They conclude that the instructional procedure is highly recommendable because (a) it is relatively simple to implement, (b) it does not prolong the learning time, and (c) it encourages near and far transfer performance.
Walkington et al. (2019), manipulated six different linguistic characteristics of algebra problems (number of sentences, pronouns, word specificity, word hyperonymy, sentence consistency, and problem topic) and measured accuracy and response time. They found little evidence that individual language characteristics had a significant effect on verbal math problem-solving performance for a general population of students. However, the consistency of the sentences reduced the response time.
Werner et al. (2019), evaluated mathematical skills, linguistic development, and the ability to verbalize mathematical content, in three experimental groups (students with language problems, with learning problems and without special educational needs). The results of the three subgroups showed specific differences in both mathematical and linguistic-communicative abilities. However, the findings suggested that students with better math skills tend to have better language skills as well. Regardless of which of these skills is the cause and which the effect, it has recently been reported that socioemotional skills influence learning mathematics. Slot et al. (2020), investigated children’s development by assessing their linguistic, social-emotional, mathematical, and arithmetic language skills. It was found that language skills are strong predictors of socio-emotional development, mathematical language, and numeracy skills. This points to the need of considering these foundational skills in curricula and young children’s daily activities such as playing.
On the other hand, Ellerton and Clarkson (1996), suggested that much of the interaction and communication in mathematics classrooms remains predominantly symbolic and artificial. They concluded that the teaching of mathematics is dictated by adults simplifying what they perceive as appropriate “mathematics” for the students.
Experimental paradigms have been developed in which performance in learning and problem solving in mathematics have been attributed to strategies of self-explanation and self-regulation used in training (Bielaczyc et al., 1995). For example, Dresher (1934) and Johnson (1944) found gains in problem-solving ability when students received specific training in mathematical vocabulary, and Sax and Ottina (1958) found that specific training in syntax can also improve language skills performance. In addition, Linville (1970), confirmed the hypothesis that difficult vocabulary and syntax interfere with effective problem solving.
One question that is still open is whether detailed instruction in reading, and especially reading in math, can improve math performance. Experiments on reading in mathematics have focused on the effect of reading instruction on mathematics achievement (Call and Wiggin, 1966; Gilmary, 1967; Henney, 1969; Earp, 1970). Previous research has found positive correlations between study strategies (when students explain difficult concepts to themselves), and test scores associated with those concepts (Chi et al., 1989; Pirolli and Recker, 1994).
Bielaczyc et al. (1995), identified a set of self-explanation and self-regulation strategies used by high-achieving students. These researchers used strategy training to manipulate students’ use of these strategies and examined the impact on explanations and student performance. The results indicated that self-explanation and self-regulation strategies used in training contributed to learning and problem-solving performance.
On the other hand, Hodds et al. (2014) reported about three experiments showing that a simple booklet containing self-explanation training, designed to focus students’ attention on logical relationships within a proof of mathematical theorem, can significantly improve the understanding of the proof. It has been proposed that the educational system must consider those teaching-learning methods and strategies that efficiently lead to these transformative processes, from the external plane to the plane of cognitive activity (Aiken, 1972). That is why we explored the use of verbal language to solve a mathematical problem.
As we have seen, several studies have been addressed in the teaching of mathematics such as language factors, self-explanation, and self-regulation. However, in this work our objective was to propose a simple paradigm in which verbal language, applied to the solution of inverse functions, can successfully replace the use of algebraic language.
120 undergraduate students from the humanities area (20.5 ± 1.66 years) participated in this study. Only first-semester students who had recently entered the university were included. Students who were studying the first semester with more than 6 months of having passed the College Board university admission exam were not included. This guaranteed a homogeneity in the cognitive level of the students. The local ethics committee approved the experimental protocol.
We a priori calculated the sample size of this study by using the free-use software G*Power 3.1.9.7 (Faul et al., 2009). We used values of alpha = 0.05 (Fisher, 1922; Field, 2013) and beta = 0.2 (Cohen, 1992; Field, 2013) for type I and II errors, respectively. Thus, the estimated statistical power was 0.8. A median effect size of 0.3 (Cohen, 1988, 1992) was assumed, which coincided a posteriori with Cramer’s V which was 0.301. With these values, G*Power calculated a total sample size of 88 subjects. Note, that our final sample was 120 participants.
To solve inverse function problems, we only focused on algebraic functions. These types of functions use operations such as addition, subtraction, multiplication, division, powers of integers, and rational powers (roots). Three types of functions were considered to solve their inverse function (Table 1). Each participant solved only one problem, consequently a total of 120 exercises were solved. Thus, each of the three exercises was applied 40 times (20 were solved with the algebraic method and 20 with the verbal method). The experimental session was individual and lasted approximately 30 min, during which participants sat comfortably in a ventilated and illuminated room.
The method for teaching the verbal and algebraic solution was based on the neuropsychological theory of Galperin (1969), called Activity Theory. This theory proposes the orientation for the planning of the field activity in the teaching-learning process, called the Action Guiding Base (AGB). Orientation conditions the actions necessary to plan, develop and move towards learning. In this way, the AGB is a guidance system that directs the learning process, through a series of actions that are put into practice through an activity. The organization of this set of operations prioritizes the realization of a previously established objective. This theory is based on Assimilation by Mental Stages and Concept Formation by Galperin and is framed within the cultural-historical theory of (Vygotsky, 1980, 1986, 1997) and (Leontiev, 1978, 1999, 2005; Leontiev and Luria, 1999).
Using the AGB, the facilitator elaborates the orientation points, presents them in an unfolded way and executes them in a shared way. The Theory of Mental Assimilation by Stages and Formation of Concepts proposed by Galperin, organizes the conversion of concepts into mental actions by three indispensable subsystems (Mendonça et al., 2020): (1) The orientation, (2) The steps of assimilation and (3) The Qualitative learning indicators. We applied AGB in the teaching of both methods as follows. (1) The facilitator gave the orientation. (2) We measured the steps of assimilation by the qualitative errors. Finally, (3) we measured qualitative learning indicators with the dichotomous variable (“could solve it” or “could not solve it”). The teaching methods based on the AGB, seek that the executor of the action (in this case the student), generalizes the idea, synthesizes it and performs the action independently. According to this theory, the AGB allows the student to create the bases of the mental formation of the actions for learning, providing the corrects answers in futures tasks (Engeness and Lund, 2020; Mendonça et al., 2020).
Therefore, in both verbal and algebraic method, the facilitator explained the participants on a blackboard an example very similar to the exercise that she/he had to solve. In this stage he provided instructions on how to proceed to find the correct solution, focusing on the critical steps using tables, diagrams, schemes, or drawings in the blackboard. Once the facilitator developed the solution of the task, he asked the students to explain to themselves the solution and resolved doubts and questions, to reinforce what she/he has been learned. After this, a sheet of paper with the inverse function problem printed on it, was given to the participant. Then the student was left alone to solve the task.
In the verbal method, the facilitator showed the verbal solution to find the inverse function according to the requirements of the AGB, described previously. In this method, the facilitator used only verbal language reasoning to focus on these critical steps: (1) Finding the hierarchy of operations, (2) Inverting the hierarchy of operations, and (3) Finding the opposites of operations. In step 3, the facilitator emphasized that (a) addition and subtraction, (b) multiplication and division, and (c) nth power and nth root are inverse operations, respectively.
As an example, we will use the function f(x) = 8x2−2 (see Table 1) to describe in a very simplified way, the verbal method to find the inverse function.
Step 1: The facilitator explained to the participant the hierarchy of operations. He began saying, given an arbitrary number x, the first thing that does the function is raise to the power of 2 the number x. The second thing to do is multiply the last result by 8. And finally, the last instruction was to subtract 2. The facilitator summarized the hierarchy of operations in a scheme on the blackboard:
(1) raise to the power of 2
(2) multiply by 8
(3) subtract 2
Step 2: The facilitator explained to the subject that the next step in the verbal method is to reverse the list of the hierarchy of operations, writing them on the blackboard.
(3) subtract 2
(2) multiply by 8
(1) raise to the power of 2
Step 3: The facilitator explained how to find the inverse operations, doing examples in the blackboard with the help of diagrams, and drawings.
At the end he summarized all three steps in the blackboard through the next table.
Finally, the facilitator showed how to write in symbolic form the inverse function, following the consecutive order of the information in the column of step 3:
In the algebraic method, the facilitator showed the algebraic solution to find the inverse function according to Stewart (2019). The process of teaching met the AGB requirements as it was explained previously. In this method, the facilitator used mathematical concepts and algebraic notations to explain the critical steps:
Step 1: Write y = f(x).
Step 2: Solve the equation for x in terms of y: x = f(y).
Step 3: Express in terms of x, and swap x and y. The resulting equation is y = f−1(x).
Finally, the facilitator summarized all three steps in the blackboard through the next table.
Since subtraction is a special case of addition; division is a special case of multiplication; and roots are a special case of powers, we proposed the following categories to measure the qualitative errors for the verbal method solution: (1) Read the hierarchy of operations, (2) Invert the hierarchy of operations, and (3) Find the opposite operations.
For the algebraic method, we proposed the following categories to measure the qualitative errors: (1) Addition-subtraction, (2) Multiplication-division and (3) Root-power. In both solution methods, we consider only the first error that led to the wrong solution. These data are available in a Supplementary File.
For each participant we use a categorical value of 1, if she/he solved the problem correctly and 0 if she/he did not. As the main objective of this research was to know if the number of exercises solved correctly is related to the type of method used to solve it (that is, we wanted to know the relationship that exists between the two categorical variables 1 and 0), we used the Test Pearson’s chi-square (Pearson, 1900; Fisher, 1922).
We found a significant association between the type of method to solve inverse functions and the success of the solution χ2 (1) = 10.84, p < 0.001. We calculate the probability of success with the verbal and algebraic method according to Field (2013) as follows,
Although Cramer’s V is adequate to measure the effect size, another more common and useful way to measure the effect size for categorical data is the odds ratio. For this reason, we calculate the odds ratio, dividing the probability of success with the verbal method (2.33) by the probability of success with the algebraic method (0.62) (Field, 2013). We found that the probability of success of the solution was 2.33/0.62 = 3.75 times greater if it is solved with the verbal method than if it is solved with the algebraic method.
For the exercises solved incorrectly in the verbal method, we found the following errors: (a) 38.9% related to reading the hierarchy of operations, (b) 5.55% related to inversing the hierarchy of operations and (c) 55.55% related to finding the opposite operations. On the other hand, for the algebraic method, we found (a) 10.8% errors related to addition-subtraction, (b) 35.2% errors related to multiplication-division and (c) 54% errors related to root-power.
The present study investigated the usefulness of verbal language applied to the solution of inverse functions in comparison with the algebraic method. We found a statistically significant increase in the correct scores by using the verbal method compared to the algebraic one.
Because participants, who correctly solved the inverse function problem, were from the field of humanities, they likely have higher verbal skills compared to math skills. Another explanation is that humanities students, who correctly solved the inverse function problem, could be more familiar with verbal learning than with abstract learning. If this is the case, this result could be in line with previous studies done by Dark and Benbow (1991). These authors compared the working memory performance of highly gifted 13- and 14-year-olds who showed: (1) mathematical and verbal earliness, (2) primarily mathematical earliness, or (3) primarily verbal earliness. In experiment 1 they examined (1) the repeatability of working memory for digit, letter, word, and location stimuli and (2) the manipulation in working memory of digit, letter, and location stimuli. The precocious youth verbally showed a greater capacity for words, and the mathematically precocious youth showed a greater capacity for stimuli of digits and location. In working memory manipulation, the mathematically highly gifted outperformed the verbally highly gifted with digits and letters. Experiment 2 examined the encoding speed in working memory. Verbally precocious participants showed higher coding speed.
Although, in our study, young people with learning disabilities were not explicitly assessed, our results could provide additional support for the hypothesis that insufficient language development, particularly in self-regulation, could be a cognitive mechanism underlying dyslexia as well as dyscalculia. Epidemiological studies describe high rates of comorbidity between reading and mathematical difficulties: approximately 40% of dyslexics also have arithmetic difficulties (Lewis et al., 1994; Robinson et al., 2002; Vilenius-Tuohimaa et al., 2008; Cirino et al., 2015), and the prevalence of dyslexia and dyscalculia is similar, approximately 4–7% (Dirks et al., 2008; Landerl and Moll, 2010; Haase et al., 2014).
It has been suggested that prior to verbalization the most basic numerical intuitions are supported by an evolutionary ancient approximate number system that is shared by adults (Pica et al., 2004), infants (Xu and Spelke, 2000) and non-human animals (Dehaene, 1997; Nieder and Miller, 2004). Despite the pervasive nature of the approximate number system both across species and in human development, it is not known whether some individuals have more accurate non-verbal “number sense” than others. Furthermore, the extent to which this system interacts with formal and symbolic mathematical skills that humans acquire through explicit instruction is unknown (Halberda et al., 2008).
While different types of intellectual talent seem to be associated with different characteristics of working memory, and with differences in how the stimuli of digits and words are represented in memory, it is highlighted that the development of verbal skills directly and positively influences the speed of coding the information (guaranteeing its evocation). Some authors have pointed out (Nelson, 1998; Gruber and Goschke, 2004; Shabani et al., 2010), that language mediates all psychological processes and participates in the regulation and control of all activity. Knowledge of the path of language development and its various functions in cognitive activity, will allow understanding of individual differences in student learning, by favoring the appropriate strategies for students’ success.
It is known that language participates in an essential way, both in the development and in the consolidation of other cognitive processes. In the case of attention, it allows conscious and voluntary development to fulfill its function of controlling cognitive activity directed to a conscious goal by establishing the relationship between attention and other complex cognitive processes, such as memory (Shabani et al., 2010). Some studies showed how visuospatial and verbal memory skills predicted the conditions of mathematical and arithmetic skills (Alloway and Passolunghi, 2011). In this sense, it has been suggested that mental arithmetic is based on specific resources of working memory (Clearman et al., 2017). On the other hand, some authors have stated that inclusion of language enables the successful achievement of the objective of a task (Shabani et al., 2010; Vallotton and Ayoub, 2011; Lonigan et al., 2017).
Studies have showed the relationship between successful readings (as another peculiar form of language that mediates learning) and successful performance in mathematics. Poorly developed reading skills make it difficult to learn math. (Bohlmann and Pretorius, 2002; Hakkarainen et al., 2013). Other studies report that difficulties in both mathematics and reading are related to deficits in the storage and processing of visuospatial information, revealing a general deficit of visuospatial working memory (Kyttälä, 2008). A relatively recent study has determined predictors of student reading performance in math and science (Macdonald et al., 2018).
In contrast, Schöber et al. (2018), tested reciprocal effects between self-efficacy and achievement in the domains of mathematics and reading. They found evidence for reciprocal effects induced in the domain of reading. They concluded that, academic self-efficacy is widely accepted as being both the cause and effect of academic achievement and that their findings highlight the necessity of early interventions and a domain-specific approach.
Instructions to solve a mathematical problem frequently interact with individual learning styles and aptitudes, and both sources of influence need to be considered in mathematics learning (Aiken, 1972). In addition, individual differences in problem solving style continue to be of interest. Gagné (1966) proposed that problem-solving ability and technique vary across individuals and these variables affect the ease with which relevant rules and concepts are recalled.
Taschow (1969) and Dahmus (1970), proposed specialized methods of teaching verbal problem solving. Aiken (1972), suggested that controlled experiments related to the effect of vocabulary and reading instruction on mathematics learning, could be helpful in developing procedures to identify and categorize the lexical and grammatical units that are unique to mathematics, and consequently, could serve as a basis for classifying and comparing mathematical materials. He concluded that these are just some of the challenges for research on language factors in mathematics learning.
There is still the controversy between those who consider mathematics as a language per se and those who do not. Reasoning a little more, we could conclude that mathematics is not but rather a kind of “dialect” because it is a “regional” variant derived from a parent language. A dialect that was created with the need to communicate complex ideas, and over time was formalized and unified into one. In this case, the regional variant would be the community of mathematicians and the parent language would be the fusion of the mother tongues of the civilizations that created it.
The verbal and algebraic teaching methods used in our study were based the theory of the formation of mental actions proposed by Galperin (1969) and framed in the activity theory of Leontiev (1978). This theory consists of growing complexity stages in which language plays a key role.
The first stage is known as the guiding base of the action and is provided by the facilitator in a social context (instructions, references, information about the action to be carried out, the order of the actions, the operations that compose it, or others). The second stage is the formation of the action through a material medium, where the student performs the action, but with the object as a facilitator (he can use diagrams, schemes, drawings, etc.). The third stage is the formation of the action through an external verbal process, where the elements of the action are presented to the student in an external verbal (oral or even written) form. The fourth stage is the externally formation of the action by the student’s own language (that is, the role of orientation, direction of execution and control of the activity is played by the student’s own language, who talks to himself often aloud or in written form). At this stage no external (social) facilitation through language is needed.
In the last stage of mental representation’s formation of the action, neither writing nor speaking one’s own language is required (the processes of the activity are automated). Therefore, it is crucial that the student (who is in the process of acquiring mathematical skills), is provided with adequate guiding base for action through verbal instructions. This will facilitate the acquisition and formation of the following stages, thus allowing the correct performance of the task. This idea applies to both methods, but because formation of mental actions is based on natural language, which has a social component, we expected that the verbal method would have a greater effect than the algebraic method on task scores.
In the verbal method we quantified the categorical errors related to (1) reading the hierarchy of operations, (2) inverting the hierarchy of operations and (3) finding the opposites of the operations. In fact, the last one was the most prevalent. Nevertheless, the verbal method obtained better scores compared to the algebraic method. Since in both methods we apply the AGB of activity theory (Galperin, 1969), the success of the verbal method against the algebraic one can only be explained by the instruction algorithm: one used only verbal language and the other verbal language mixed with algebraic concepts. The instruction given in natural language for the verbal method increased the probability of participants success. This is because the verbal algorithm is easier to understand than the algebraic one. In this way, the verbal method cannot be understood independently without the participation of the facilitator. The facilitator is a crucial part of the verbal method. The facilitator instructed the participants in a way that asked them to follow a simple logical sequence of steps, previously explained by him. In an analogy with computer programming, the facilitator somehow tried to program (or condition) the participants to follow an algorithm simpler than algebraic. Following simple rules is easier than following general rules that apply to both algebraic and transcendental functions. This is when AGB reinforces learning. The next step in the learning process is when the student is left alone to explain the problem to himself and that depends largely on the reading ability to understand and find the correct solution. This is in line with Vilenius-Tuohimaa et al. (2008), who suggested that optimal performance in solving mathematical problems is strongly related to performance in reading comprehension. The fact that there is a method that avoids using symbols and formal conceptualizations of mathematics stresses the fact that there are problems in mathematics where elementary language is still able to operate and be useful at that level.
In the algebraic method we quantified the categorical errors related to addition-subtraction, multiplication-division, and root-power. In this case, the last one was the most prevalent. Although inverse functions operationally use algebra instead of arithmetic, it is important to mention that in the field of arithmetic, Spencer and Russell (1960) identified several reasons why students experience difficulty in reading arithmetic problems: (1) the names of certain numerals are confusing; (2) number languages which are patterned differently from the decimal system are used; (3) the language of expressing fractions and ratios is complicated: (4) charts and other diagrams are frequently confusing; (5) the reading of computational procedures requires specialized skills. The fact that the AGB applied to the algebraic method was less effective than in the verbal method, stresses that a mathematical problem should be moved to a scenario where the solution algorithm is much simpler. In this case, such scenario was provided by the verbal method.
This same situation happens on a greater scale of complexity in the dual theories of physics. Physicists use the term duality to describe theoretical models that appear to be different yet can be shown to give the same physical solutions. That is, two apparently different theories are identical because they lead to the same results. A trivial example is the theory of relativity written in two languages: English and Chinese. A non-trivial example is found in string theory, which identically describes a universe that has a circular dimension of radius R and another that has radius 1/R. These are different geometric situations, but nevertheless, due to the properties of string theory, they are physically identical (Greene, 1999). In that sense it is sometimes easier to find a solution in one theory than in its dual theory. Since we measure the reality of the world in a relative and not absolute way, consistent with the model-dependent reality (Hawking and Mlodinow, 2010), this reflection teaches us that the success of solving a problem will depend on the scenario where the problem is transferred. Most of the time this is not possible to do in a trivial way, so a challenge for the teaching of mathematics is the search for representation spaces that are less abstract and easier to relate to situations in which students are more familiar. This would make the path towards learning mathematics more motivating. And motivation is always the key to success.
The successful of the solution of inverse functions using the verbal method is limited to algebraic functions, where concepts such as addition-subtraction, multiplication-division, power-root, have a much simpler algorithmic representation than those of the transcendental functions. Being simple operations, in any case, they can be exemplified by objects.
On the other hand, the verbal method may not be applicable to transcendental functions such as logarithmic, exponential, and trigonometric functions, in a simple way, since they are operations with abstract concepts. Due to this, an abstract mathematical domain is far from verbal language, in such a way that maybe it is no longer possible to use it, requiring the specialized rules of algebra per se. However, it would be interesting to develop a verbal experimental paradigm that seeks this goal.
Algebra is an extension of arithmetic that studies numbers and their elementary operations such as addition, subtraction, multiplication, and division, which in turn have a geometric (spatial) substrate. However, transcendental functions are so named because they transcend algebraic functions. We have mentioned that the verbal resolution method cannot be applied to transcendental functions, because their inverse operator is abstract and there is no direct and simple correspondence between the mathematical operator and the verbal language. In past centuries, the values of some transcendental functions such as trigonometric and logarithmic functions were published in tables, even before the modern slide rule, electronic calculators, or smartphones. This implies that the calculation of the values corresponding to their inverse functions also required a very elaborate calculation work.
On the other hand, physicists use mathematics to represent the reality of the world. But according to 20th century physics, reality is theoretical information (Wheeler, 1986, 1999) and measurable at the level of Planck scale space-time (Snyder, 1947). It has been proposed that information cannot exist without consciousness (Irwin, 2014). As conscious and thinking beings, we can recognize the meaning of relationships between objects within consciousness itself. Irwin (2014) proposes that defining consciousness as language requires: (1) a set of objects and (2) an ordering scheme with (3) degrees of freedom used to (4) express meaning. Irwin also argues that information on the Planck scale cannot exist without consciousness, so he proposes a language entity called a “primitive unit of consciousness,” which acts as a mathematical operator in a quantized space-time language (a kind of fundamental unity of consciousness.) According to Irwin, the mathematics of quasicrystals based on E8 geometry (Sadoc and Mosseri, 1993) seems to be the candidates for the language of reality.
Taking these ideas, from the Plank scale to the human scale (a scenario more common to everyday life), it is tempting to think that there could be conscious and thinking beings with a higher capacity for abstraction in which complicated mathematical calculations can be solved more easily. Perhaps in this situation, the division between verbal and mathematical language could be shortened. The fact is that there are children who are highly gifted in mathematics, but they represent a very small percentage of the child population (right tale of mathematical skills distribution). One question that arises is what is needed for these outstanding mathematical abilities to lie at the center of the normal distribution. This could perhaps occur in the next evolutionary step of humanity, which Bresch (1977) defines as Monon, which is an evolutionary state above language.
In a recent study, de la Riva and Ryan (2015) studied the effect of self-regulation in young children which did affect academic outcomes for those who transitioned to formal schooling from a preschool environment. They argued that children who are good self-regulators will realize greater academic success than those who cannot self-regulate in the later elementary grades. Is tempting to suggest that the variability that we observed in the scores of verbal method solution, could be attributed to variations in self-regulation of participants. In this regard, it is necessary to investigate the effects of self-regulation by using this paradigm in a future study. In addition, it is necessary to carry out this experimental paradigm in populations with different academic backgrounds to find factors that explain more precisely the good performance of the verbal method in humanities students. Another aspect that would be interesting to consider for future studies is to have a more precise indicator of the academic performance of the participants to make a comparative study of subgroups, for example between high and low performance students.
Finally, an interesting question for future research is to determine the scope of verbal language in penetrating the domain of higher mathematics and particularly understand concepts and solve problems such as: the epsilon definition of convergence, the process of limit to calculate the area under the curve of a function, the graph theory and connectivity, the hand-shaking lemma, the set theory and equinumerous sets, and the groups as actions on an object, among other interesting abstract mathematical concepts. There may be promising hopes for achieving these goals. For example, verbal language applications are highly successful to solve many problems in fuzzy logic control. Fuzzy sets allow the introduction of verbal judgments, instead of numerical ones, with the advantage that they can be interpreted by humans, which implies a better understanding in the evaluation of many dangerous activities. Fuzzy logic has been used to handle inexact and vague information because of its ability to use natural language in terms of linguistic variables (Singh and Mishra, 2015).
The results of the present study showed that the language method applied to the solution of inverse functions, is highly effective, in comparison with the algebraic method. Adapting the verbal method as a possible intervention for schoolchildren with mathematical difficulties should be the focus of future research in the field of pedagogic innovation and student learning.
The original contributions presented in the study are included in the article/Supplementary Material, further inquiries can be directed to the corresponding author.
The studies involving human participants were reviewed and approved by Local Ethics Committee of the Faculty of Psychology, BUAP. The patients/participants provided their written informed consent to participate in this study.
IM-B: conception or design of the work, data analysis and interpretation, drafting the manuscript, critical revision of the manuscript, and final approval of the version to be published. AH-A, DR, and CT: drafting the manuscript, critical revision of the manuscript, and final approval of the version to be published. RL-L, VL-C, MB-S, HP-G, and MG-F: data collection, analysis, and interpretation. All authors contributed to the article and approved the submitted version.
This work was supported by Vicerrectoría de Investigación y Estudios de Postgrado de la Benemérita Universidad Autónoma de Puebla: VIEP-BUAP MEBI-EDH-18 (IM-B), Mexico.
The authors declare that the research was conducted in the absence of any commercial or financial relationships that could be construed as a potential conflict of interest.
All claims expressed in this article are solely those of the authors and do not necessarily represent those of their affiliated organizations, or those of the publisher, the editors and the reviewers. Any product that may be evaluated in this article, or claim that may be made by its manufacturer, is not guaranteed or endorsed by the publisher.
The Supplementary Material for this article can be found online at: https://www.frontiersin.org/articles/10.3389/feduc.2022.868607/full#supplementary-material
Aiken, L. R. Jr. (1972). Language factors in learning mathematics. Rev. Educ. Res. 42, 359–385. doi: 10.3102/00346543042003359
Alloway, T. P., and Passolunghi, M. C. (2011). The relationship between working memory, IQ, and mathematical skills in children. Learn. Individ. Dif. 21, 133–137. doi: 10.1016/j.lindif.2010.09.013
Atkinson, R. K., Renkl, A., and Merrill, M. M. (2003). Transitioning from studying examples to solving problems: effects of self-explanation prompts and fading worked-out steps. J. Educ. Psychol. 95:774. doi: 10.1037/0022-0663.95.4.774
Austin, J. L., and Howson, A. G. (1979). Language and mathematical education. Educ. Stud. Math. 10, 161–197. doi: 10.1007/bf00230986
Ausubel, D. P., and Robinson, F. G. (1969). School Learning: An Introduction to Educational Psychology. New York, NY: Holt, Rinehart Winston. doi: 10.1177/002248717002100125
Baldo, J. V., and Dronkers, N. F. (2007). Neural correlates of arithmetic and language comprehension: a common substrate? Neuropsychologia 45, 229–235. doi: 10.1016/j.neuropsychologia.2006.07.014
Bielaczyc, K., Pirolli, P. L., and Brown, A. L. (1995). Training in self-explanation and self-regulation strategies: investigating the effects of knowledge acquisition activities on problem solving. Cogn. Instr. 13, 221–252. doi: 10.1207/s1532690xci1302_3
Bills, C. (2002). “Linguistic points in young children’s description of mental calculation,” in Proceedings of the 26th Annual Conference of the International Group for the Psychology of Mathematics, Vol. 2, eds D. A. Cockburn and E. Nardi (Norwich: University of East Anglia), 97–104. doi: 10.1111/1467-8659.00187
Bohlmann, C. A., and Pretorius, E. J. (2002). Reading skills and mathematics: the practice of higher education. S. Afr. J. High. Educ. 16, 196–206. doi: 10.4314/sajhe.v16i3.25232
Bresch, C. (1977). Zwischenstufe Leben: Evolution ohne Ziel?. München: R. Piper & Co. Verlag. doi: 10.1002/biuz.19780080509
Bruner, J. S. (1966). Toward a Theory of Instruction, Vol. 59. Cambridge, MA: Harvard University Press. doi: 10.1126/science.152.3719.193
Bruner, J. S., Olver, R. R., and Greenfield, P. M. (1966). Studies in Cognitive Growth. New York, NY: Wiley.
Call, R. J., and Wiggin, N. A. (1966). Experimental programs: reading and mathematics. Math. Teach. 59, 149–157. doi: 10.5951/mt.59.2.0149
Capps, L. R. (1970). Teaching mathematical concepts using language arts analogies. Arithmetic Teach. 17, 329–331. doi: 10.5951/at.17.4.0329
Chi, M. T., Bassok, M., Lewis, M. W., Reimann, P., and Glaser, R. (1989). Self-explanations: how students study and use examples in learning to solve problems. Cogn. Sci. 13, 145–182. doi: 10.1207/s15516709cog1302_1
Chow, J. C., and Jacobs, M. (2016). The role of language in fraction performance: a synthesis of literature. Learn. Individ. Dif. 47, 252–257. doi: 10.1016/j.lindif.2015.12.017
Cirino, P. T., Fuchs, L. S., Elias, J. T., Powell, S. R., and Schumacher, R. F. (2015). Cognitive and mathematical profiles for different forms of learning difficulties. J. Learn. Disabil. 48, 156–175. doi: 10.1177/0022219413494239
Clearman, J., Klinger, V., and Szücs, D. (2017). Visuospatial and verbal memory in mental arithmetic. Q. J. Exp. Psychol. 70, 1837–1855. doi: 10.1080/17470218.2016.1209534
Cohen, J. (1988). Set correlation and contingency tables. Appl. Psychol. Meas. 12, 425–434. doi: 10.1177/014662168801200410
Cohen, J. (1992). Statistical power analysis. Curr. Dir. Psychol. Sci. 1, 98–101. doi: 10.1111/1467-8721.ep10768783
Cooper, F. F. (1971). Math as a second language. Instructor 81, 76–77. doi: 10.1016/j.ridd.2020.103661
Dahmus, M. E. (1970). How to teach verbal problems. Sch. Sci. Math. 70, 121–138. doi: 10.1111/j.1949-8594.1970.tb08580.x
Daneman, M., and Merikle, P. M. (1996). Working memory and language comprehension: a meta-analysis. Psychon. Bull. Rev. 3, 422–433. doi: 10.3758/BF03214546
Dark, V. J., and Benbow, C. P. (1991). Differential enhancement of working memory with mathematical versus verbal precocity. J. Educ. Psychol. 83, 48–60. doi: 10.1037/0022-0663.83.1.48
Daroczy, G., Wolska, M., Meurers, W. D., and Nuerk, H.-C. (2015). Word problems: a review of linguistic and numerical factors contribution to their difficulty. Front. Psychol. 6:348. doi: 10.3389/fpsyg.2015.00348
de Araujo, Z., Roberts, S. A., Willey, C., and Zahner, W. (2018). English learners in K–12 mathematics education: a review of the literature. Rev. Educ. Res. 88, 879–919. doi: 10.1187/cbe.19-03-0069
de la Riva, S., and Ryan, T. G. (2015). Effect of self-regulating behaviour on young children’s academic success. Int. J. Early Child. Spec. Educ. 7, 69–96. doi: 10.20489/intjecse.92329
Dehaene, S. (1992). Varieties of numerical abilities. Cognition 44, 1–42. doi: 10.1016/0010-0277(92)90049-N
Dehaene, S. (1997). The Number Sense: How the Mind Creates Mathematics. New York, NY: Oxford University Press.
Dehaene, S., and Cohen, L. (1995). Towards an anatomical and functional model of number processing. Math. Cogn. 1, 83–120. doi: 10.1016/j.neuropsychologia.2006.11.012
Dehaene, S., and Cohen, L. (2007). Cultural recycling of cortical maps. Neuron 56, 384–398. doi: 10.1016/j.neuron.2007.10.004
Dirks, E., Spyer, G., Lieshout, E., and Sonneville, L. (2008). Prevalence of combined reading and arithmetic disabilities. J. Learn. Disabil. 41, 460–473. doi: 10.1177/0022219408321128
Duncan, G. J., Dowsett, C. J., Claessens, A., Magnuson, K., Huston, A. C., Pagani, L. S., et al. (2007). School readiness and later achievement. Dev. Psychol. 43, 1428–1446. doi: 10.1037/0012-1649.43.6.1428
Durand, M., Hulme, C., Larkin, R., and Snowling, M. (2005). The cognitive foundations of reading and arithmetic skills in 7-to 10-year-olds. J. Exp. Child Psychol. 91, 113–136. doi: 10.1016/j.jecp.2005.01.003
Ellerton, N. F., and Clarkson, P. C. (1996). “Language factors in mathematics teaching and learning,” in International Handbook of Mathematics Education, eds A. J. Bishop, K. Clements, C. Keitel, J. Kilpatrick, and C. Laborde (Dordrecht: Springer), 987–1033. doi: 10.1007/978-94-009-1465-0_30
Engeness, I., and Lund, A. (2020). Learning for the future: insights arising from the contributions of Piotr Galperin to the cultural-historical theory. Learn. Cult. Soc. Interact. 25:100257. doi: 10.1016/j.lcsi.2018.11.004
Faul, F., Erdfelder, E., Buchner, A., and Lang, A.-G. (2009). Statistical power analyses using G*Power 3.1: tests for correlation and regression analyses. Behav. Res. Methods 41, 1149–1160. doi: 10.3758/brm.41.4.1149
Fetzer, M., and Tiedemann, K. (2018). “The interplay of language and objects in the process of abstracting,” in Language and Communication in Mathematics Education, International Perspectives, eds J. Moschkovich, D. Wagner, A. Bose, J. Rodrigues, and M. Schütte (Dordrecht: Springer), 91–103. doi: 10.1007/978-3-319-75055-2_8
Field, A. (2013). Discovering Statistics Using IBM SPSS Statistics. London: Sage. doi: 10.1024/1012-5302/a000397
Fisher, R. A. (1922). On the mathematical foundations of theoretical statistics. Philos. Trans. R. Soc. Lond. Ser. A Containing Pap. Math. Phys. Character 222, 309–368. doi: 10.1098/rsta.1922.0009
Freudenthal, H. (1985). Muttersprache und mathematiksprache. [Mother tongue and mathematics language]. Math. Lehren 9, 3–5.
Fuchs, L. S., Fuchs, D., Seethaler, P. M., and Craddock, C. (2020). Improving language comprehension to enhance word-problem solving. Read. Writ. Q. 36, 142–156. doi: 10.1080/10573569.2019.1666760
Fuchs, L. S., Fuchs, D., Seethaler, P. M., Cutting, L. E., and Mancilla-Martinez, J. (2019). Connections between reading comprehension and Word-Problem solving via oral language comprehension: implications for comorbid learning disabilities. New Dir. Child Adolesc. Dev. 2019, 73–90. doi: 10.1002/cad.20288
Gagné, R. M. (1966). “The learning of principles,” in Analyses of Concept Learning, eds H. G. Klausmeier and C. W. Harris (New York, NY: Academic Press), 81–95. doi: 10.1016/b978-1-4832-3127-3.50011-0
Galperin, P. (1969). “Stages in the development of mental acts,” in A Handbook of Contemporary Soviet Psychology, eds M. Cole and I. Maltzman (New York, NY: Basic Books), 249–273.
Gersten, R., Chard, D. J., Jayanthi, M., Baker, S. K., Morphy, P., and Flojo, J. (2009). Mathematics instruction for students with learning disabilities: a meta-analysis of instructional components. Rev. Educ. Res. 79, 1202–1242. doi: 10.3102/0034654309334431
Gilmary, S. (1967). Transfer effects of reading remediation to arithmetic computation when intelligence is controlled and all other school factors are eliminated. Arithmetic Teach. 14, 17–20. doi: 10.5951/at.14.1.0017
Greene, B. (1999). The Elegant Universe. Superstrings. Hidden Dimensions, and the Quest for the Ultimate Theory. New York, NY: W.E. Norton. doi: 10.1086/426259
Gruber, O., and Goschke, T. (2004). Executive control emerging from dynamic interactions between brain systems mediating language, working memory and attentional processes. Acta Psychol. 115, 105–121. doi: 10.1016/j.actpsy.2003.12.003
Haase, V. G., Júlio-Costa, A., Lopes-Silva, J. B., Starling-Alves, I., Antunes, A., Pinheiro-Chagas, P., et al. (2014). Contributions from specific and general factors to unique deficits: two cases of mathematics learning difficulties. Front. Psychol. 5:102. doi: 10.3389/fpsyg.2014.00102
Hakkarainen, A., Holopainen, L., and Savolainen, H. (2013). Mathematical and Reading difficulties as predictors of school achievement and transition to secondary education. Scand. J. Educ. Res. 57, 488–506. doi: 10.1080/00313831.2012.696207
Halberda, J., Mazzocco, M. M., and Feigenson, L. (2008). Individual differences in non-verbal number acuity correlate with maths achievement. Natureresearch J. 455, 665–669. doi: 10.1038/nature07246
Hart, S. A., Petrill, S. A., Thompson, L. A., and Plomin, R. (2009). The ABCs of math: a genetic analysis of mathematics and its links with reading ability and general cognitive ability. J. Educ. Psychol. 101, 388–402. doi: 10.1037/a0015115
Hawking, S., and Mlodinow, L. (2010). The Grand Design. New York, NY: Bantam Books, doi: 10.1007/s10806-010-9298-7
Hecht, S. A., Torgesen, J. K., Wagner, R. K., and Rashotte, C. A. (2001). The relations between phonological processing abilities and emerging individual differences in mathematical computation skills: a longitudinal study from second to fifth grades. J. Exp. Child Psychol. 79, 192–227. doi: 10.1006/jecp.2000.2586
Heinze, A., Herwartz-Emden, L., Braun, C., and Reiss, K. (2011). “Die Rolle von Kenntnissen der unterrichtssprache beim mathematiklernen. Ergebnisse einer quantitativen Längsschnittstudie in der grundschule [The role of knowledge of the language of instruction in learning mathematics. Results of a quantitative longitudinal study in primary school],” in Mathematiklernen unter Bedingungen der Mehrsprachigkeit. Stand und Perspektiven der Forschung und Entwicklung in Deutschland, eds S. Prediger and E. Özdil (Münster: Waxmann), 11–34.
Henney, M. A. (1969). The Relative Impact of Mathematics Reading Skills Instruction and Supervised Study Upon Fourth Graders’ Ability to Solve Verbal Problems in Mathematics. Doctoral dissertation. Kent, OH: Kent State University.
Hickerson, J. A. (1959). Similarities between teaching language and arithmetic. Arithmetic Teach. 6, 241–244. doi: 10.5951/at.6.5.0241
Hodds, M., Alcock, L., and Inglis, M. (2014). Self-explanation training improves proof comprehension. J. Res. Math. Educ. 45, 62–101. doi: 10.5951/jresematheduc.45.1.0062
Irwin, K. (2014). A new approach to the hard problem of consciousness: a quasicrystalline language of “primitive units of consciousness” in quantized spacetime. Act. Nerv. Super. 62, 48–68. doi: 10.1007/s41470-020-00071-3
Johnson, H. C. (1944). The effect of instruction in mathematical vocabulary upon problem solving in arithmetic. J. Educ. Res. 38, 97–110. doi: 10.1080/00220671.1944.10881316
Koponen, T., Georgiou, G., Salmi, P., Leskinen, M., and Aro, M. (2017). A meta-analysis of the relation between RAN and mathematics. J. Educ. Psychol. 109, 977–992. doi: 10.1037/edu0000182
Krauthausen, G. (2007). “Sprache und sprachliche Anforderungen im Mathematikunterricht der Grundschule [Language and language requirements in primary school mathematics lessons],” in Sonderpädagogik der Sprache, eds H. Schöler and A. Welling (Göttingen: Hogrefe), 1022–1034.
Krummheuer, G. (1994). Der Mathematische Anfangsunterricht: Anregungen für ein Neues Verstehen früher Mathematischer Lehr-Lern-Prozesse [The Initial Mathematical Lessons: Suggestions for a New Understanding of Early Mathematical Teaching-Learning Processes]. Weinheim: Dt. Studien-Verlag.
Krummheuer, G. (2012). The “non-canonical” solution and the “improvisation” as conditions for early years mathematics learning processes: the concept of the “Interactional Niche in the Development of Mathematical Thinking “(NMT). J. Math. Didaktik 33, 317–338. doi: 10.1007/s13138-012-0040-z
Kyttälä, M. (2008). Visuospatial working memory in adolescents with poor performance in mathematics: variation depending on reading skills. Educ. Psychol. 28, 273–289. doi: 10.1080/01443410701532305
Landerl, K., and Moll, K. (2010). Comorbidity of learning disorders: prevalence and familial transmission. J. Child Psuchol. Psychiatry 51, 287–294. doi: 10.1111/j.1469-7610.2009.02164.x
LeFevre, J.-A., Fast, L., Skwarchuk, S.-L., Smith-Chant, B. L., Bisanz, J., Kamawar, D., et al. (2010). Pathways to mathematics: longitudinal predictors of performance. Child Dev. 81, 1753–1767. doi: 10.1111/j.1467-8624.2010.01508.x
Leontiev, A. (1999). “The development of voluntary attention in the child,” in Lev Vygotsky: Critical Assessments, eds P. Lloyd and C. Fernyhough (London: Routledge), 89–113.
Leontiev, A. (2005). Study of the environment in the pedological works of L. S. Vygotsky: a critical study. J. Russ. East Eur. Psychol. 43, 8–28. doi: 10.1080/10610405.2005.11059254
Leontiev, A., and Luria, A. (1999). “The psychological ideas of L.S. Vygotskij,” in Lev Vygotsky: Critical assessments, eds P. Lloyd and C. Fernyhough (London: Routledge), 56–89.
Lerkkanen, M.-K., Rasku-Puttonen, H., Aunola, K., and Nurmi, J.-E. (2005). Mathematical performance predicts progress in reading comprehension among 7-year-olds. Eur. J. Psychol. Educ. 20, 121–137. doi: 10.1007/bf03173503
Lewis, C., Hitch, G., and Walker, P. (1994). The prevalence of specific arithmetic difficulties and specific Reading difficulties in 9 and 10 year old boys and girls. J. Child Psychol. Psychiatry 35, 283–292. doi: 10.1111/j.1469-7610.1994.tb01162.x
Linville, W. J. (1970). The Effects of Syntax and Vocabulary Upon the Difficulty of Verbal Arithmetic Problems with Fourth Grade Students. Doctoral dissertation. Bloomington: Indiana University.
Lombrozo, T. (2006). The structure and function of explanations. Trends Cogn. Sci. 10, 464–470. doi: 10.1016/j.tics.2006.08.004
Lonigan, C. J., Spiegel, J. A., Goodrich, J. M., Morris, B. M., Osborne, C. M., Lerner, M. D., et al. (2017). Does preschool self-regulation predict later behavior problems in general or specific problem behaviors? J. Abnorm. Child Psychol. 45, 1491–1502. doi: 10.1007/s10802-016-0260-7
Macdonald, K., Milne, N., Orr, R., and Pope, R. (2018). Relationships between motor proficiency and academic performance in mathematics and reading in school-aged children and adolescents: a systematic review. Int. J. Environ. Res. Public Health 15:1603. doi: 10.3390/ijerph15081603
Madden, R. (1966). New directions in the measurement of mathematical ability. Arithmetic Teach. 13, 375–379.
Maier, H., and Schweiger, F. (1999). Mathematik und Sprache. Zum Verstehen und Verwenden von Fachsprache im Mathematikunterricht [Mathematics and Language: On Understanding and Using Terminology in Mathematics Lessons]. Wien: öbv & hpt.
Mendonça, R. A., Oliveira, C. B. M., José, A., Oliveira, S., and daSilva, J. F. Jr. (2020). Orientation for field activity planning: contributions of P. Ya. Galperin. Int. J. Adv. Eng. Res. Sci. (IJAERS) 7, 128–139. doi: 10.22161/ijaers.75.18
Moreno, R., and Durán, R. (2004). Do multiple representations need explanations? The role of verbal guidance and individual differences in multimedia mathematics learning. J. Educ. Psychol. 96:492. doi: 10.1037/0022-0663.96.3.492
Nelson, K. (1998). Language in Cognitive Development: The Emergence of the Mediated Mind. Cambridge: Cambridge University Press. doi: 10.2307/417856
Nieder, A., and Miller, E. K. (2004). A parieto-frontal network for visual numerical information in the monkey. Proc. Natl. Acad. Sci. U.S.A. 101, 7457–7462. doi: 10.1073/pnas.0402239101
Nolte, M. (2016). “Sprache und Sprachverstehen in mathematischen Lernprozessen aus einer mathematikdidaktischen Perspektive [Language and language understanding in mathematical learning processes from the a mathematics education perspective],” in Sprache und Inklusion als Chance? Expertise und Innovation für Kita, Schule und Praxis, eds U. Stitzinger, S. Sallat, and U. Lüdtke (Idstein: Schulz-Kirchner), 37–44.
Paige, J. M., and Simon, H. A. (1966). “Cognitive processes in solving algebra word problems,” in Problem Solving: Research, Method, and Theory, ed. B. Kleinmuts (New York, NY: John Wiley & Sons), 51–119.
Pearson, K. (1900). X. On the criterion that a given system of deviations from the probable in the case of a correlated system of variables is such that it can be reasonably supposed to have arisen from random sampling. Lond. Edinburgh Dublin Philos. Mag. J. Sci. 50, 157–175. doi: 10.1080/14786440009463897
Peng, P., Lin, X., Ünal, Z. E., Lee, K., Namkung, J., Chow, J., et al. (2020). Examining the mutual relations between language and mathematics: a meta-analysis. Psychol. Bull. 146:595. doi: 10.1037/bul0000231
Peng, P., Wang, C., and Namkung, J. (2018). Understanding the cognition related to mathematics difficulties: a meta-analysis on the cognitive deficit profiles and the bottleneck theory. Rev. Educ. Res. 88, 434–476. doi: 10.3102/0034654317753350
Piaget, J. (1954). The Construction of Reality in the Child. New York, NY: Basic. doi: 10.1037/11168-000
Pica, P., Lemer, C., Izard, V., and Dehaene, S. (2004). Exact and approximate arithmetic in an Amazonian indigene group. Science 306, 499–503. doi: 10.1126/science.1102085
Pimm, D. (1987). Speaking Mathematically: Communication in Mathematics Classrooms. Language, Education, and Society. London: Routledge. doi: 10.4324/9781315278858
Pirolli, P., and Recker, M. (1994). Learning strategies and transfer in the domain of programming. Cogn. Instr. 12, 235–275. doi: 10.1207/s1532690xci1203_2
Planas, N., Morgan, C. R., and Schütte, M. (2018). “Mathematics education and language: lessons and directions from two decades of research,” in Developing Research in Mathematics Education: Twenty Years of Communication, Cooperation and Collaboration in Europe, eds T. Dreyfus, M. Artigue, D. Potari, S. Prediger, and K. Ruthven (Oxford: Routledge), 196–210. doi: 10.4324/9781315113562-15
Prado, J. (2018). “The interplay between learning arithmetic and learning to read: insights from developmental cognitive neuroscience,” in Heterogeneity of Function in Numerical Cognition, eds A. Henik and W. Fias (Cambridge: Academic Press), 27–49. doi: 10.1016/b978-0-12-811529-9.00002-9
Pyburn, D. T., Pazicni, S., Benassi, V. A., and Tappin, E. E. (2013). Assessing the relation between language comprehension and performance in general chemistry. Chem. Educ. Res. Pract. 14, 524–541. doi: 10.1039/c3rp00014a
Qolbi, M. S. U., Thaariq, Z. Z. A., Az-Zahroh, S. F., Anwar, M. M., and Faiza, N. (2019). Design and development of game based learning applications for mathematics learning based on multiple language to develop verbal capabilities. JPP Jurnal Pendidikan Pembelajaran 26, 51–56. doi: 10.17977/um047v26i22019p051
Rieber, R. W. (1997). “The problem of the development of higher mental functions,” in The Collected Works of L. S. Vygotsky. Cognition and Language (A Series in Psycholinguistics), ed. R. W. Rieber (Boston, MA: Springer). doi: 10.1007/978-1-4615-5939-9_1
Rimoldi, H. J. A. (1967). Thinking and language. Arch. Gen. Psychiatry 17, 568–576. doi: 10.1001/archpsyc.1967.01730290056007
Rimoldi, H. J., Aghi, M., and Burger, G. (1968). Some effects of logical structure, language, and age in problem solving in children. J. Genet. Psychol. 112:127.
Robinson, C. S., Menchetti, B. M., and Torgesen, J. K. (2002). Toward a two-factor theory of one type of mathematics disabilities. Learn. Disabil. Res. Pract. 17, 81–89. doi: 10.1111/1540-5826.00035
Sadoc, J. F., and Mosseri, R. (1993). The E8 lattice and quasicrystals: geometry, number theory and quasicrystals. J. Phys. A Math. Gen. 26:1789. doi: 10.1088/0305-4470/26/8/009
Sax, G., and Ottina, J. R. (1958). The arithmetic achievement of pupils differing in school experience. Cal. J. Educ. Res. 9, 15–19.
Schöber, C., Schütte, K., Köller, O., McElvany, N., and Gebauer, M. M. (2018). Reciprocal effects between self-efficacy and achievement in mathematics and reading. Learn. Individ. Dif. 63, 1–11. doi: 10.1016/j.lindif.2018.01.008
Schütte, M. (2009). Sprache und Interaktion im Mathematikunterricht an Grundschulen [Language and Interaction in Mathematics Lessons at Primary Schools]. Münster: Waxmann.
Shabani, K., Khatib, M., and Ebadi, S. (2010). Vygotsky’s zone of proximal development: instructional implications and teachers’ professional development. Engl. Lang. Teach. 3, 237–248. doi: 10.5539/elt.v3n4p237
Singh, N., and Mishra, R. K. (2015). The modulatory role of second language proficiency on performance monitoring: evidence from a saccadic countermanding task in high and low proficient bilinguals. Front. Psychol. 5:1481. doi: 10.3389/fpsyg.2014.01481
Slot, P. L., Bleses, D., and Jensen, P. (2020). Infants’ and toddlers’ language, math and socio-emotional development: evidence for reciprocal relations and differential gender and age effects. Front. Psychol. 11:580297. doi: 10.3389/fpsyg.2020.580297
Spencer, P. L., and Russell, D. (1960). “Reading in arithmetic,” in Instruction in Arithmetic. Twenty-Fifth Yearbook of the National Council of Teachers of Mathematics, ed. F. E. Grossnickle (Washington, DC: NCTM), 202–223.
Steinbring, H. (2000). Mathematische bedeutung als soziale konstruktion im mathematikunterricht [Mathematical meaning as social construction in mathematics education]. J. Math. Didaktik 21, 28–48. doi: 10.1007/bf03338905
Vallotton, C., and Ayoub, C. (2011). Use your words: the role of language in the development of toddlers’ self-regulation. Early Child. Res. Q. 26, 169–181. doi: 10.1016/j.ecresq.2010.09.002
Vilenius-Tuohimaa, P. M., Aunola, K., and Nurmi, J. E. (2008). The association between mathematical word problems and reading comprehension. Educ. Psychol. 28, 409–426. doi: 10.1080/01443410701708228
Vukovic, R. K., and Lesaux, N. K. (2013). The language of mathematics: investigating the ways language counts for children’s mathematical development. J. Exp. Child Psychol. 115, 227–244. doi: 10.1016/j.jecp.2013.02.002
Vygotsky, L. (1980). Mind in Society: The Development of Higher Psychological Processes. Cambridge, MA: Harvard University Press. doi: 10.2307/j.ctvjf9vz4
Vygotsky, L. (1997). The History of the Development of Higher Mental Functions. Teoksessa the Collected Works of LS Vygotsky, Vol. 4, New York, NY: Plenum.
Walkington, C., Clinton, V., and Sparks, A. (2019). The effect of language modification of mathematics story problems on problem-solving in online homework. Instr. Sci. 47, 499–529. doi: 10.1007/s11251-019-09481-6
Werner, B., Berg, M., and Höhr, R. (2019). “Math, i don’t get it”: an exploratory study on verbalizing mathematical content by students with speech and language impairment, students with learning disability, and students without special educational needs,” in Inclusive Mathematics Education, eds D. Kollosche, R. Marcone, M. Knigge, M. Penteado, and O. Skovsmose (Cham: Springer), 377–399. doi: 10.1007/978-3-030-11518-0_23
Wheeler, J. A. (1986). Hermann Weyl and the Unity of Knowledge: in the linkage of four mysteries—the” how come” of existence, time, the mathematical continuum, and the discontinuous yes-or-no of quantum physics—may lie the key to deep new insight. Am. Sci. 74, 366–375.
Wheeler, J. A. (1999). “Information, physics, quantum: the search for links,” in Feynman and Computation, Exploring the Limits of Computers, 1st Edn, ed. A. J. G. Hey (Boca Raton, FL: CRC Press). doi: 10.1201/9780429500459-19
Keywords: inverse algebraic functions, self-explanation, self-regulation, executive functions, verbal learning, verbal ability, action guiding base, theory of mental assimilation by stages and formation of concepts
Citation: Méndez-Balbuena I, Hermosillo-Abundis AC, Ruge D, Trenado C, Lemuz-López R, López-Cortés VA, Bonilla-Sánchez MdR, Pelayo-González HJ and García-Flores MA (2022) The Use of Language in Solving Inverse Algebraic Functions Problems. Front. Educ. 7:868607. doi: 10.3389/feduc.2022.868607
Received: 03 February 2022; Accepted: 14 March 2022;
Published: 27 April 2022.
Edited by:
Ana Teresa Ferreira Oliveira, Instituto Politécnico de Viana do Castelo, PortugalReviewed by:
Angela Silva, Polytechnic Institute of Viana do Castelo, PortugalCopyright © 2022 Méndez-Balbuena, Hermosillo-Abundis, Ruge, Trenado, Lemuz-López, López-Cortés, Bonilla-Sánchez, Pelayo-González and García-Flores. This is an open-access article distributed under the terms of the Creative Commons Attribution License (CC BY). The use, distribution or reproduction in other forums is permitted, provided the original author(s) and the copyright owner(s) are credited and that the original publication in this journal is cited, in accordance with accepted academic practice. No use, distribution or reproduction is permitted which does not comply with these terms.
*Correspondence: Ignacio Méndez-Balbuena, aWdtZW5kZXpiYWxAZ21haWwuY29t, orcid.org/0000-0002-3054-4944
Disclaimer: All claims expressed in this article are solely those of the authors and do not necessarily represent those of their affiliated organizations, or those of the publisher, the editors and the reviewers. Any product that may be evaluated in this article or claim that may be made by its manufacturer is not guaranteed or endorsed by the publisher.
Research integrity at Frontiers
Learn more about the work of our research integrity team to safeguard the quality of each article we publish.