- 1TEL Measurement Consulting LLC, Iowa City, IA, United States
- 2Duolingo Inc, Pittsburgh, PA, United States
In the past few years, our lives have changed due to the COVID-19 pandemic; many of these changes resulted in pivoting our activities to a virtual environment, forcing many of us out of traditional face-to-face activities into digital environments. Digital-first learning and assessment systems (LAS) are delivered online, anytime, and anywhere at scale, contributing to greater access and more equitable educational opportunities. These systems focus on the learner or test-taker experience while adhering to the psychometric, pedagogical, and validity standards for high-stakes learning and assessment systems. Digital-first LAS leverage human-in-the-loop artificial intelligence to enable personalized experience, feedback, and adaptation; automated content generation; and automated scoring of text, speech, and video. Digital-first LAS are a product of an ecosystem of integrated theoretical learning and assessment frameworks that align theory and application of design and measurement practices with technology and data management, while being end-to-end digital. To illustrate, we present two examples—a digital-first learning tool with an embedded assessment, the Holistic Educational Resources and Assessment (HERA) Science, and a digital-first assessment, the Duolingo English Test.
Introduction
In the decade prior to the pandemic, learning and assessment providers were striving to update their pedagogical and delivery models to better meet the needs of learners, test-takers, and other stakeholders including counselors and admissions officers (Mislevy and Haertel, 2006; Dadey et al., 2018; Laurillard et al., 2018). New applications of digital technology were being evaluated regarding their potential for increasing educational access and equity (Blayone et al., 2017; Laurillard et al., 2018). The onset of the COVID-19 pandemic accelerated these efforts. While educational institutions and assessment organizations struggled to adapt to the new normal and meet the need for online tools, the digital-first learning and assessment (LAS) model provided a possible solution. LAS that emphasized an enhanced and personalized learner and test-taker experience were made possible due to rapid technological advances (e.g., platform development, cloud computing, database management), and innovation in machine learning (ML) and artificial intelligence (AI).
In this paper, we discuss digital-first LAS (i.e., digital assessments that use human-in-the-loop artificial intelligence across the learning and assessment pipelines). In addition, we describe how LAS emerged from the integration of multiple innovative advances and examine how they can lead to more democratic and equitable educational opportunities. Further, we present the context that led to their successful adoption, explain the theoretical framework ecosystem that supports them, and illustrate them with two examples: the Holistic Educational Resources Assessment (HERA) Science and the Duolingo English Test. We conclude the paper by commenting on trends and the importance of transparency, privacy, and fairness. The main message of the paper is that the paradigm shift around the design and implementation of digital-first LAS is grounded in theoretical and technological integration.
With the emergence of ubiquitous computer infrastructure over the past 30 years, education has been transforming from the standard on-site, lecture-based classroom to include remote, online learning experiences. Innovators have established online K-12 schools where geographically dispersed students interact in digital classrooms (such as K-12 School, which was launched in 1999, as described in K12 School, 2021). Approximately 10 years ago, Stanford University launched the first Massive Open Online Courses (MOOCs), which offered free education anywhere and to anyone. Shah (2021) reported that during the past 10 years MOOCs have transitioned from being primarily university-led to private for-profit business ventures. Simultaneously, their clientele has transitioned to predominantly adult learners taking courses for career advancement (Shah, 2021). Generally, these online schools and online course providers embraced the concept of personalized learning tools, distancing their pedagogical approaches from traditional “one-size-fits-all education.”
Although online LAS were available to schools and workplace training programs prior to COVID-19, the pandemic accelerated their use and adoption. The pandemic has further caused many educators to evaluate their methods and consider how digital technology can be applied to provide a more learner- and test-taker-centric learning experience (Collison, 2021). This increased use has led to the realization that learners and test-takers need to acquire digitally mediated skills (i.e., skills involving interaction in a digital setting) (van Laar et al., 2017; Jackman et al., 2021). This new skill set requires them to actively participate in educational experiences, such as Zoom for remote video meetings, communicate via chatbox and in virtual forums, and use learning management systems for uploading coursework and managing classroom discussions.
From the assessment perspective, for nearly 100 years, standardized testing required that all test-takers complete a test under uniform administrative conditions. Test publishers maintained that standardization was needed to ensure that scores from different administrations were comparable and fair (Educational Testing Service, 2016). Test publishers began to relax these requirements near the end of the last century with the introduction of computer adaptive testing (CAT) (Educational Testing Service, 2016; Way et al., 2016; Jiao and Lissitz, 2017). As CAT was becoming more prevalent, advances were occurring in measurement, such as computational psychometrics (von Davier, 2017; von Davier et al., 2021) along with advances in technology (from AI to data management and cloud computing—see for example, von Davier et al., 2019a). At the same time, psychometricians and policy makers were re-evaluating their understanding of test fairness (American Educational Research Association, 2014; Sireci, 2021).
Digital-first LAS provide for an individualized learning and assessment experience. Despite concerns about the possible negative effects of digital technology on human behavior (Dale et al., 2020; Korte, 2020) and the current presence of a digital divide (Gorski, 2005; Moore et al., 2018), we concur with Laurillard et al.’s (2018) position that “digital technologies have the characteristics of interactivity, adaptivity, communication, and user control that a good educational experience demands” (p. 3). In this regard, digital-first LAS have the potential to enhance learning, leading to a more democratic and equitable experience. Learning becomes more democratic when learners can shape their experience, engage in self-reflection, and participate in decision making within an inclusive environment (Knight and Pearl, 2000; Garrison, 2008; Pohan, 2012). At their core, both learning and democracy are about personal empowerment - individuals having the means to shape and direct their experiences (Garrison, 2008).
In providing a learner-centric environment, digital-first LAS are focused on the needs of individual learners and provide the means to assist them in growing in both domain knowledge and socio-cognitive development. Combining learning activities with formative assessments tends to result in better learning outcomes and the development of life-long skills (Tomlinson, 2004; Linn and Chiu, 2011; Richman and Ariovich, 2013; Amasha et al., 2018). Along with being learner-centric, digital-first LAS potentially can increase equity by providing access to high-quality learning and assessment experiences. Emerging digital technologies that leverage advances in AI, ML, and psychometrics have made possible the development of high-quality personalized learning and assessment platforms that can be scaled to meet learners’ and test-takers’ needs regardless of geographic location. No other technology has a comparable potential for reaching learners and test-takers globally (Laurillard et al., 2018).
As digital-first LAS are designed and operationalized, developers need to also implement fairness agendas to monitor and evaluate system use. Fairness includes the evaluation of algorithmic fairness, reducing gender, ethnicity, and age bias, and maximizing accessibility through the accommodation of individual needs. LAS further should seek solutions to minimize the negative effects of digital technology related to human behavior and the digital divide.
Digital-First Learning and Assessment Systems
Digital-first LAS require theoretical integration and technological interoperability. Theoretical integration refers to these systems being composed of multiple integrated frameworks that serve as a blueprint for processes and decisions used in design and measurement. Burstein et al. (2022) provide an example of an ecosystem where a complex set of assessment frameworks are integrated to support the test validity argument for a language assessment. Technological interoperability refers to how, within these systems, technology interfaces with the processes and decisions applied to design and measurement. The core is the integration and interoperability of many complex parts across digital platforms with the goal of providing greater access to individualized learning and adaptive testing. Digital-first LAS achieve both greater access and more individualized adaptation, contributing to more equitable educational opportunities. This is achieved through the application of automated tools combined with advanced measurement methodologies within a seamless technology infrastructure. Theoretical integration and technological interoperability are integral parts of the design and not an after-thought. We apply the ecosystem from Burstein et al. (2022) with the data paradigm from von Davier et al. (2019b,2021) to the digital-first LAS and discuss how different frameworks and features support the use of digital-first LAS used for illustration in this paper—the HERA Science and the Duolingo English Test. Next, we describe theoretical integration and technological interoperability in greater detail.
Theoretical Integration
Burstein et al. (2022) describe a theoretical assessment ecosystem composed of an integrated set of complex frameworks guiding the design, measurement, and security of a language assessment. Their ecosystem supports the development of a test validity argument. The digital-first assessment ecosystem includes (a) the domain design framework, (b) the expanded Evidence-Centered Design (e-ECD) framework, (c) the computational psychometrics (CP) framework, and (d) test security framework. In addition, the ecosystem emphasizes the test-taker experience (TTX), which is impacted by factors including the test’s low cost, anytime/anywhere testing, shorter testing time, delightful user interface design, free test-readiness resources and automatically scored practice tests, and rapid score turn-around. Attention to the TTX increases fairness by increasing broader access to the assessment. A parallel concept to TTX for a learning system is the learner experience (LX). For a LAS, we need to expand the concept to include both the learner and test-taker experience (LTTX). Figure 1 expands Burstein et al.’s (2022) ecosystem model to capture digital-first learning and assessment systems, including the LTTX. As illustrated in Figure 1, the ecosystem framework processes contribute to the digital chain of inferences (DCI) to build a validity argument for an LAS. Kane (1992) and Chapelle et al. (2008) define the chain of inferences as the logical link between the claims about test score validity (i.e., scores used for their intended purpose) and the set of inferences that support these claims. For instance, when reviewing Duolingo English Test scores, score users infer that the test scores represent test-takers’ English language proficiency and were derived based on their responses to items that are relevant to the English language constructs, so that the scores reflect speaking, writing, reading, and listening skills. The ecosystem developed for the Duolingo English Test is the first model to consider a comprehensive digitally-informed chain of inferences (DCI; Burstein et al., 2022). Moreover, the DCI addresses digital affordances that contribute to the test validity argument (Burstein et al., 2022. Digital affordances refer to advanced computational methods, such as AI and NLP methods, and more generally, the ability to administer tests on a digital device.
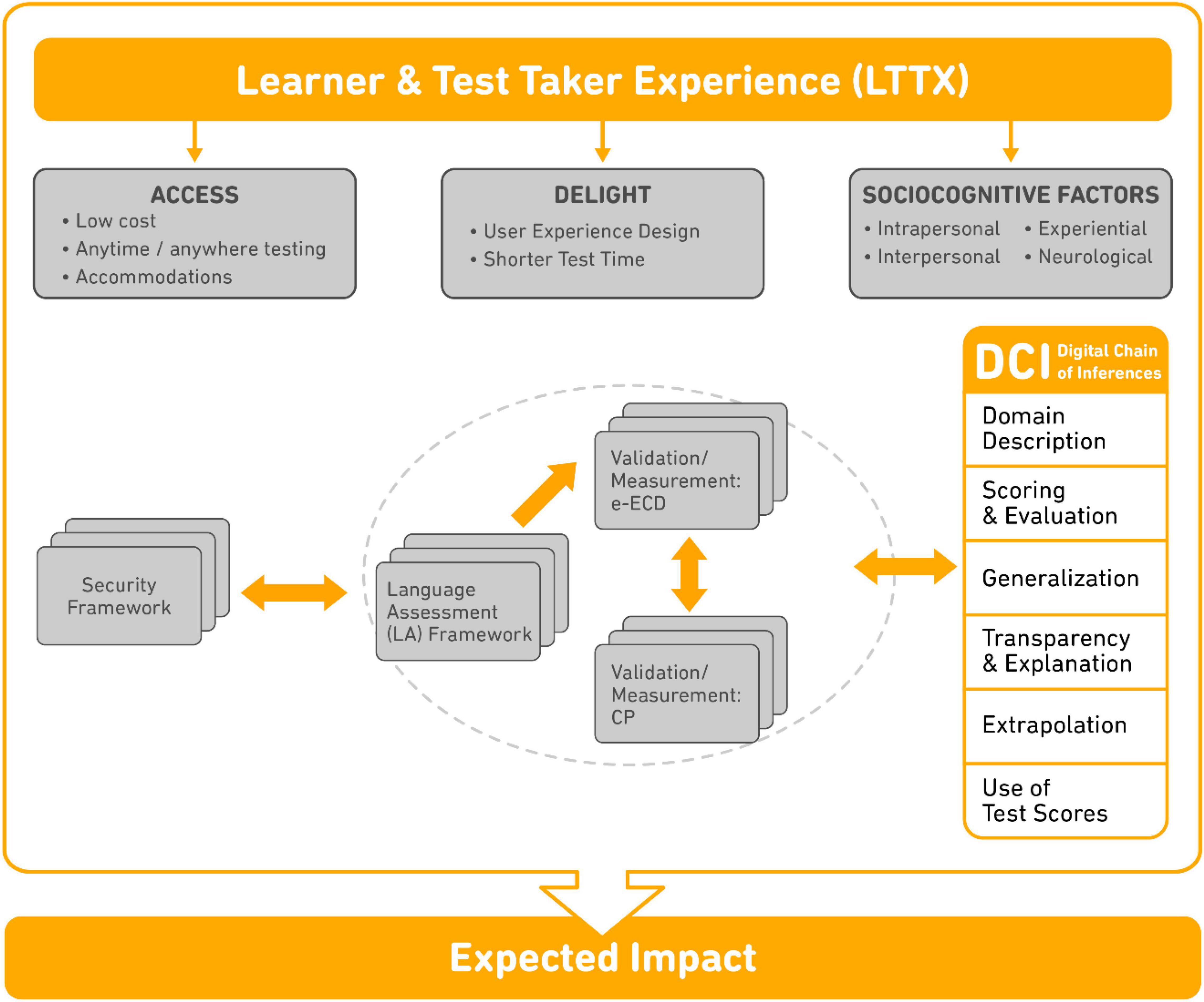
Figure 1. Illustration of the digital-first ecosystem components and their interactions for an LAS. This figure is an expansion of Figure 1 from Burstein et al. (2022). The security framework and the LTTX influence all ecosystem components and together they provide support for achieving the Expected Impact, that is the democratization and equitability of education as described.
Technological Interoperability
In a digital-first LAS, each part of the system is integrated with all other parts so that the learner and test-taker seamlessly transition from one experience to the next. Facilitating a seamless transition between different parts of the LAS is relevant to both learners and test-takers: in the learner case, it is reflected through navigational tools through lessons and levels, data integration from multiple sessions, and mastery-tracking visualization and feedback; in the test-taker case, it is reflected through the test registration process, preparation materials and feedback, the test-taking experience, and score reporting. To describe this system with its seamless transitions, we borrow from computer science and refer to its integration as an interoperable system (Rayon et al., 2014). Interoperability refers to the ability of computerized systems to connect and communicate with one another easily. It refers to a system that can exchange information across devices, applications, and databases without interruption or extensive programming (Sondheim et al., 1999; U.S. Department of Education, 2012; Cooper, 2014).
An interoperable digital-first system, informed by integrated theoretical frameworks, offers students a learner-centric experience that provides accessible learning opportunities aligned with learners’ ability; validates that learning has occurred through embedded personalized assessment and feedback; and maintains secure learner or test-taker personal data, while permitting users to share score and learning outcomes with relevant third-party stakeholders (U.S. Department of Education, 2012; von Davier et al., 2019a). For an assessment, an interoperable system allows test-takers to register, prepare, and take an assessment in an easy-to-navigate platform. Within a learning platform, it allows students to participate in learning activities, evaluate whether they have achieved the objectives and obtained additional assistance if needed. The digital-first system stores and retrieves multiple data points for each participant, data regarding learning activities and assessments, and learning taxonomies and standards. (see Rayon et al., 2014; von Davier et al., 2019a; U.S. Department of Education, 2012 for additional information on interoperable educational systems and data).
To build digital-first systems, designers must resolve the tension between the interrelated requirements of quality measurement, maximum accessibility, and security. Quality measurement requires that content is relevant and representative. It further requires that accurate feedback or score information is provided. In turn, these requirements necessitate the gathering of sufficient evidence to support score interpretations and feedback within an acceptable number of user–system interactions. If a digital-first system requires too many interactions, the evidence for measurement may be strong and the test results may be accurate; however, learners or test-takers may experience fatigue, reducing the level of accessibility as they begin to disengage. By incorporating adaptive learning with testing algorithms and adaptive testing, designers can shorten the LAS experience while keeping it as reliable as traditional learning tools and tests. Maximum accessibility requires that the digital system be accessible anytime and anywhere. Learners and test-takers should not be constrained with respect to when or where they can access the system. Furthermore, the experience of learning and testing needs to support user engagement throughout the interaction with the LAS. The requirement of maximum accessibility and adaptive quality measurement raises security concerns regarding content, scores, and data. Effective programs must include deterrent security tools so that they provide valid, actionable, and usable information. For example, to maintain the security of the adaptive assessment, item development must be designed so that the item pool is large and replenished often. Because quality measurement, maximum accessibility, and system security are critical for developing effective digital-first assessment systems, they are features that infuse all design decisions, which is the reason Burstein et al. (2022) assert that the security framework for digital-first assessments interacts with all frameworks in the theoretical assessment ecosystem.
Digital-first LAS can be evaluated in the context of the socio-cognitive framework (Weir, 2005; Mislevy, 2018; Mislevy and Elliot, 2020). The framework assumes that in addition to domain knowledge (e.g., English language proficiency), achievement in a domain may be affected by general skill proficiency (e.g., critical thinking) as well as intrapersonal (e.g., confidence), interpersonal (e.g., collaboration), experiential (e.g., test item familiarity), and neurological factors (e.g., neurodiversity). For instance, in assessment, digital affordances in LAS support automatically scored practice with test item types. The digital affordances are intended to support test access and opportunity and socio-cognitive factors (such as test-taker confidence and test familiarity) that may play a role in test-taker performance (Weir, 2005; Kunnan, 2018; Mislevy, 2018). Intrapersonal and interpersonal factors can be developed in learning environments (von Davier et al., 2021) and evaluated with interactive tasks, including games, simulations, interactions with chatbots, and virtual reality environments. For example, the learning system described in this paper, HERA Science, includes a measure of confidence.
Next, we focus our discussion on ecosystem frameworks that contribute to the design and measurement of digital-first LAS.
The Domain Design Framework
The discussion of the theoretical learning and assessment ecosystem leverages Burstein et al.’s (2022) language assessment ecosystem, which includes a design framework specific to the language proficiency domain. In this section, we discuss a broader concept of the Domain Design framework. The purpose of the Domain Design framework is to clearly state the purpose and intended uses of the LAS, define the constructs underlying the system, and provide theoretical support for the use of the constructs for the intended purpose (Burstein et al., 2022).
The theoretical rationale of the learning and assessment constructs underlying an LAS must be well articulated in terms of relevance and representativeness (i.e., skills being measured) within the targeted domain (e.g., language proficiency, science, mathematics). In terms of the learning component, relevant constructs are defined to form the basis for the learning activities. To build out the activities, the knowledge, skills, abilities, and other attributes (KSAOs) are explicitly defined. A learning map may be developed with learning progressions, and prerequisites may be identified. Various models of learning maps and taxonomies have been developed with different emphases (Koehler and Mishra, 2009; Koedinger et al., 2012; Kingston et al., 2017; Pelánek, 2017).
Learning maps and taxonomies provide a formal structure for mapping learners’ progression from a novice state to an expert state. The KSAOs may contain multiple nodes of prerequisite knowledge. They may focus primarily on the attainment of domain-specific knowledge and skills, and/or they may take a socio-cognitive approach where additional factors beyond target domain knowledge are included (e.g., general skills—reading and critical thinking, intrapersonal skills—motivation and self-efficacy, interpersonal skills—collaboration, experiential factors—learning activity or test item familiarity, and neurological factors—neurodiversity).
Learning and assessment content is developed to align with the defined constructs that are derived from the purpose of the LAS and, for assessments specifically, the use of the scores. Learning and assessment experiences and items or tasks are mapped to the constructs and designed to elicit behaviors representative of the KSAOs. Designers provide theoretical and empirical evidence substantiating that the tasks assist learning, and in the case of an assessment, that the tasks elicit evidence to assess the latent constructs. Theoretical guidelines for task design are produced by evaluating learning and assessment frameworks and taxonomies and aligning the design features with these taxonomies. Empirical evidence for task design should be collected through cognitive labs and pilots to be considered for the LAS. These types of evidence constitute part of the validity argument supporting the efficacy of the learning system and the intended test score interpretation/use, respectively.
Artificial Intelligence and Natural Language Processing Applied to Domain Design
Advances in the use of AI and natural language processing (NLP) over the past two decades have facilitated the development of digital-first LAS. Advances in AI and NLP have enabled the following processes:
• the development of content at scale through automated item generation (Settles et al., 2020);
• automated item difficulty prediction (McCarthy et al., 2021);
• the collection and analysis of process and product data (Liao et al., 2021);
• automated scoring of constructed-response items (Yan et al., 2020).
Human-in-the-loop AI occurs when the AI algorithm is augmented at critical stages by either having a human worker intervene to improve the performance of the algorithm or having a human worker audit the algorithmic decisions at critical points (Gronsund and Aanestad, 2020). Human-in-the-loop AI has been leveraged to develop assessment tasks aligned to well-defined latent constructs (including estimating the difficulty level) at scale. For example, the Duolingo English Test uses human-in-the-loop AI and NLP to develop items at scale and at target difficulty levels (McCarthy et al., 2021). AI has further enabled the collection and analysis of process and response data to identify misunderstandings and knowledge gaps. For example, in HERA Science, when a learner is unable to respond correctly, the tool either rephrases the task to help the learner better understand the problem, breaks the task down into component parts to assist the learner, or provides additional instruction around the concept (Rosen et al., 2020; Arieli-Attali et al., 2022). Using human-in-the-loop AI, both the Duolingo English Test and the HERA Science systems score complex responses automatically to provide scores or feedback.
The Expanded Evidence-Centered Design Framework
Evidence-Centered Design (ECD) (Mislevy et al., 2002, 2003) is the most widely applied evidentiary assessment framework (Ferrara et al., 2017). ECD requires that assessment specialists clearly explain and analyze all design decisions, building a chain of inferential claims and evidence that support score use (Kane, 2013). ECD consists of three interrelated models: (a) the Proficiency model, (b) the Task model, and (c) the Evidence model. The Proficiency model formulates the claims regarding interpretations of the test-takers’ KSAOs. This model articulates what is to be measured and what relationships exist among the relevant variables. The Task model specifies the tasks or items on the test that are designed to elicit observable behaviors representative of the KSAOs. The Evidence model forms the link that provides evidentiary support for score interpretations and use (Mislevy et al., 2003). The Evidence model generally consists of scoring rules and statistical models that are used to derive scores or other information (e.g., subscores, pass/fail status, diagnostic information). All three interrelated models provide data that instantiate the claims enumerated through the chain of inferences.
Expanded Evidence-Centered Design
The expanded Evidence-Centered Design (e-ECD) builds on Mislevy et al.’s (2003) ECD framework by adding an explicit learning layer (Arieli-Attali et al., 2019). The learning layer expands the ECD framework within all three models—Proficiency, Task, and Evidence. Each model has a parallel learning component; thus, the expanded framework contains six models (Arieli-Attali et al., 2019). Figure 2 provides a visual representation of the e-ECD framework.
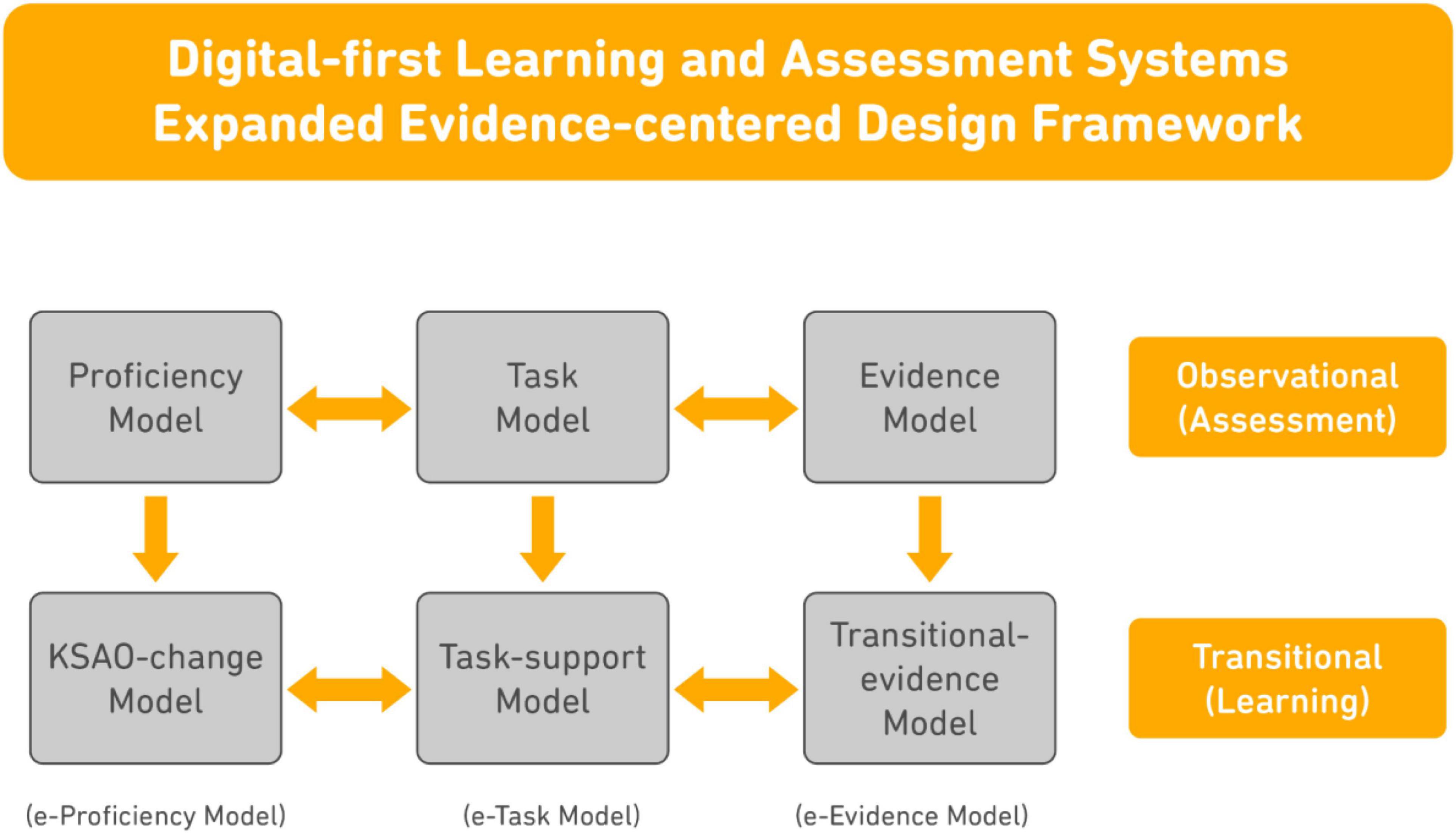
Figure 2. The Expanded Evidence-centered Design framework emphasizes transitional learning with assessment.
Whereas the purpose of an assessment system is to estimate or diagnose the latent KSAO(s) at a single point in time, the purpose of a learning system is to assist the learner in moving from one level of knowledge or skill to a higher level. The primary purpose of a blended learning and assessment tool is to assist the learner in moving from one level to the next, validate that learning has occurred, and substantiate that the system assisted learning. As such, the e-ECD framework requires the formulation of both the learning and assessment goals, which include the specification of the learning processes, the building of learning supports, and the process for ensuring the validity of both learning and assessment.
In e-ECD, the Task model is expanded to include learning and becomes the Task-support model: as weaknesses or misunderstandings are detected, the Task-support model provides support to assist the learner in achieving competency on the KSAOs. This support is illustrated in HERA Science, below.
The Proficiency model in e-ECD is adapted and renamed as the KSAO-change model. In e-ECD, in contrast to the Proficiency model specifying test-taker proficiency at a point in time, the KSAO-change model specifies test-taker proficiency over time, based on the learning theory, principles, and/or goals that form the basis of the learning system. Given a specific learning node, the KSAO-change model defines the prerequisites and/or background knowledge required to learn the target. In addition to identifying the prerequisite knowledge, the change model defines the learning processes that provide support to the learner. The support may include scaffolds, videos, explanations, hints, practice exercises, re-teaching, and other activities.
The Task-support model combined with the KSAO-change model exponentially expands the analyses that can be conducted on the available data. The data include both process and product data, and collectively may provide evidence around the learner’s knowledge, misunderstandings, fluency, reasoning, forgetting, and speed. Because digital learning and assessment tools are highly individualized, the data are complex and multidimensional. Data complexity and dependencies (such as dependencies over the parts within a task, or data dependencies over time within the LAS, or data dependencies across people within collaborative projects) are inherent to game-simulation-based tasks, multimodal learning and assessment tasks, and collaborative tasks that are common in digital-first LAS.
As the Evidence model connects the Task model to the Proficiency model, within the e-ECD framework, the Transitional-evidence model connects the Task-support model to the KSAO-change model. The Transitional-evidence model includes two components: the scoring rules and the statistical models. Designers must develop a coding and scoring system that represents the type, quality, and quantity of the support. As in any scoring system, statistical models must be selected that allow the designers to infer learners’ cognitive changes based on the observables.
Because of the complexity and data dependencies found in an LAS, traditional psychometric approaches (i.e., classical test theory and item response models) are inadequate for analyzing and interpreting the data. Psychometric models traditionally assume that students’ latent skills are fixed over the course of the assessment. In a LAS, however, the goal is to change (increase) skills during the use of the system. For this reason, new ways of modeling and reasoning with data are needed. Computational psychometrics advances psychometric theory and addresses the shortcomings of traditional psychometric modeling theory, which is not sufficiently robust for modern LAS.
The Computational Psychometrics Framework
Psychometrics is the design and analysis of models that describe, infer, or predict test-takers’ KSAOs from their responses. DiCerbo and Behrens (2012) argued that, in digital assessments, limiting analyses to test-taker responses restrict the analysis to a “digital desert” when an “ocean” of information is available. To maximize the potential of LAS, psychometricians have partnered with learning and computer scientists to develop new methods for analyzing learning and assessment data (Mislevy et al., 2016).
Where traditional psychometrics transforms response data (products) into evidence about latent constructs, computational psychometrics (CP) is an integrative measurement framework: it blends theory-based psychometrics and data-driven approaches from machine learning, AI, and data science. This interdisciplinary approach provides a theory-based methodology for analyzing complex product and process data from digital-first learning and assessment tools (von Davier, 2017; Cipresso et al., 2019). In essence, it strives to utilize the expansive data ocean available, through which inferences are derived regarding student learning and knowledge. CP models are developed to analyze various data types, including multimodal data, to establish how information and evidence can be connected to the learning and assessment domain.
Traditional psychometric methods can be viewed as a top-down approach where experts develop and apply measurement theory, analyzing data to derive score interpretations. CP supplements the top-down confirmatory approach with a bottom-up exploratory approach wherein data mining and AI algorithms identify patterns and trends. The information provided through the bottom-up analysis may include online actions, time stamps, interactions with the virtual environment, and clicks on specific features of the digital system. With AI analyzing massive quantities of data with various dependencies, patterns begin to emerge that may provide evidence regarding the efficacy of different learner actions and supports. These emergent patterns then lead to modifications in the hypothesized relationships and a second set of confirmatory top-down analyses. The findings from additional sets of analyses lead to additional exploratory bottom-up analyses leading to further insights and patterns around learning and assessment (Mislevy et al., 2016; Polyak et al., 2017; von Davier et al., 2019a,2021). Content information, such as learning taxonomies, curricular standards, knowledge maps, and behavioral classifications, can be integrated with instructional content to further empower the system (von Davier, 2021).
Computational psychometrics reconceptualizes traditional psychometrics. In addition to the traditional modeling approaches, CP includes the application of machine learning algorithms that are made possible by modern big data management and computational power to gain insights into knowledge and learning gains (von Davier, 2017; von Davier et al., 2021). In the spirit of ECD (Mislevy et al., 2003), intentional data collection is a key feature of CP (von Davier, 2017; Cipresso et al., 2019; von Davier et al., 2019a). In this context, as part of the domain design, evidence specifications are determined to ensure that relevant data are available for modeling learning and assessment. Process and product data can be identified, gathered, and analyzed using CP principles (i.e., leveraging traditional psychometric modeling approaches and advanced machine learning algorithms).
The Security Framework
Digital-first LAS require an effective security framework to achieve two vital objectives: (a) the minimization of fraudulent learner or test-taker behaviors and (b) the protection of learners’ and test-takers’ personal data. The two most common fraudulent behaviors are cheating and the theft of content (Foster, 2015). Minimizing cheating and content theft is required to maintain program integrity and the validity of score-based interpretations (Langenfeld, 2020). Digital-first LAS must not only be cognizant of fraudulent behaviors, they must also ensure that learners’ and test-takers’ personally identifiable information (PII) is secured and protected. The Standards for Educational and Psychological Testing (American Educational Research Association, 2014) state that programs are responsible for the security of all PII (not just score results). Programs must protect PII during all stages including the collection of information, transfer of data, storage of PII, and the reporting of results to authorized third parties.
The security framework informs design decisions in digital-first LAS from end-to-end. To minimize cheating behaviors and to protect content, security protocols are incorporated into the design of the registration and authentication system, the learner or test-taker onboarding process, the administration of content, and in the development of content (LaFlair et al., 2022). Regarding the security of learners’ or test-takers’ PII, the European Union (2016) formulated the General Data Protection Regulation (GDPR) to ensure that users’ data is secured and protected from unauthorized use. The regulations define the critical components that programs must ensure in the protection of learners’ and test-takers’ personal data.
Digital-First Learning and Assessments: Examples
In the remainder of the paper, we provide two illustrations of digital-first systems. Both examples are situated in the ecosystem of integrated theoretical frameworks and technological interoperability allowing for synergies and coherence from design to delivery.
The first example is HERA Science (henceforth, HERA), a holistic and personalized LAS (Ozersky, 2021; Arieli-Attali et al., 2022). The second example is the Duolingo English Test, a high-stakes English language assessment that is the pioneer in digital-first assessments (Settles et al., 2020; Cardwell et al., 2022).
Holistic Educational Resources and Assessment
Holistic Educational Resources Assessment is an adaptive learning system that blends learning with formative assessment using simulations. It is designed for middle and high school students with the objective of building students’ understanding of scientific principles and reasoning. Its design provides a personalized learning experience, and it engages students through self-reflection and gamified simulations (Ozersky, 2021). By blending learning and assessment, the system (a) enables learning to continue within the assessment, (b) enhances students’ confidence and self-reflection, and (c) supports the delivery of meaningful feedback.
Scientific Principles Design Framework
Aligned to the Next-Generation Science Standards (Lead State Partners, 2013) and the science domain of ACT’s holistic framework of cognitive skills (Camara et al., 2015), HERA combines computer-based simulations with adaptive learning supports (Ozersky, 2021; Arieli-Attali et al., 2022). HERA currently includes lessons covering eight scientific concepts. In addition to providing learning activities in the science domain, in the spirit of the socio-cognitive framework, the system includes features that support the development of intrapersonal skills (such as engagement, motivation, confidence, and self-regulation).
All HERA lessons have gamified elements to increase engagement (Ryan and Rigby, 2019); students earn medals based on the accumulation of points. To avoid misuse of the feedback, students are provided coins, which they may spend on learning scaffolds. Lessons are further designed to promote growth in self-reflection. After students respond to an item, before they can move forward to the next activity, they must rate their confidence in the correctness of their response. The confidence index has been designed to help students develop a healthy level of confidence, as research indicates that girls and minority students tend to underestimate their skills, especially in STEM (Kloper and Thompson, 2019). At the conclusion of each lesson, students reflect on the learning scaffolds, their responses, and learning growth. As such, lessons are designed to assist students in learning scientific principles while increasing their scientific self-efficacy and metacognitive skills.
A HERA lesson begins by introducing a scientific phenomenon. The student then explores a simulation designed to enrich their understanding. All simulations are constructivist-based where students learn by doing. Students explore the simulation autonomously, leading to deeper understanding and greater motivation. As students engage with the simulations and learn principles around the concept, they respond to different assessment items. When students respond incorrectly, they are given the option of using a learning scaffold before responding again. They are given a choice of three different adaptive metacognitive learning scaffolds: (a) rephrase – the student receives a rephrasing of the item in simplified form, (b) break-it-down—the student is provided the first step as a hint to solve the item, and (c) teach-me—the student is provided information regarding the scientific concept and works through a parallel task. The first support targets the skill itself by assisting the student in decoding the item stem, thereby reducing construct irrelevant variance. The second support addresses the proximal precursor in that the student may have partial knowledge but is unable to respond correctly. The third support addresses the initial, proximal, and distal precursors by providing full instruction around the scientific concept (Rosen et al., 2020).
The three adaptive scaffolds require students to assess their current level of understanding and then select appropriately from the supports to assist them in correctly responding. Figure 3 presents a sample HERA item and the three adaptive scaffolds.
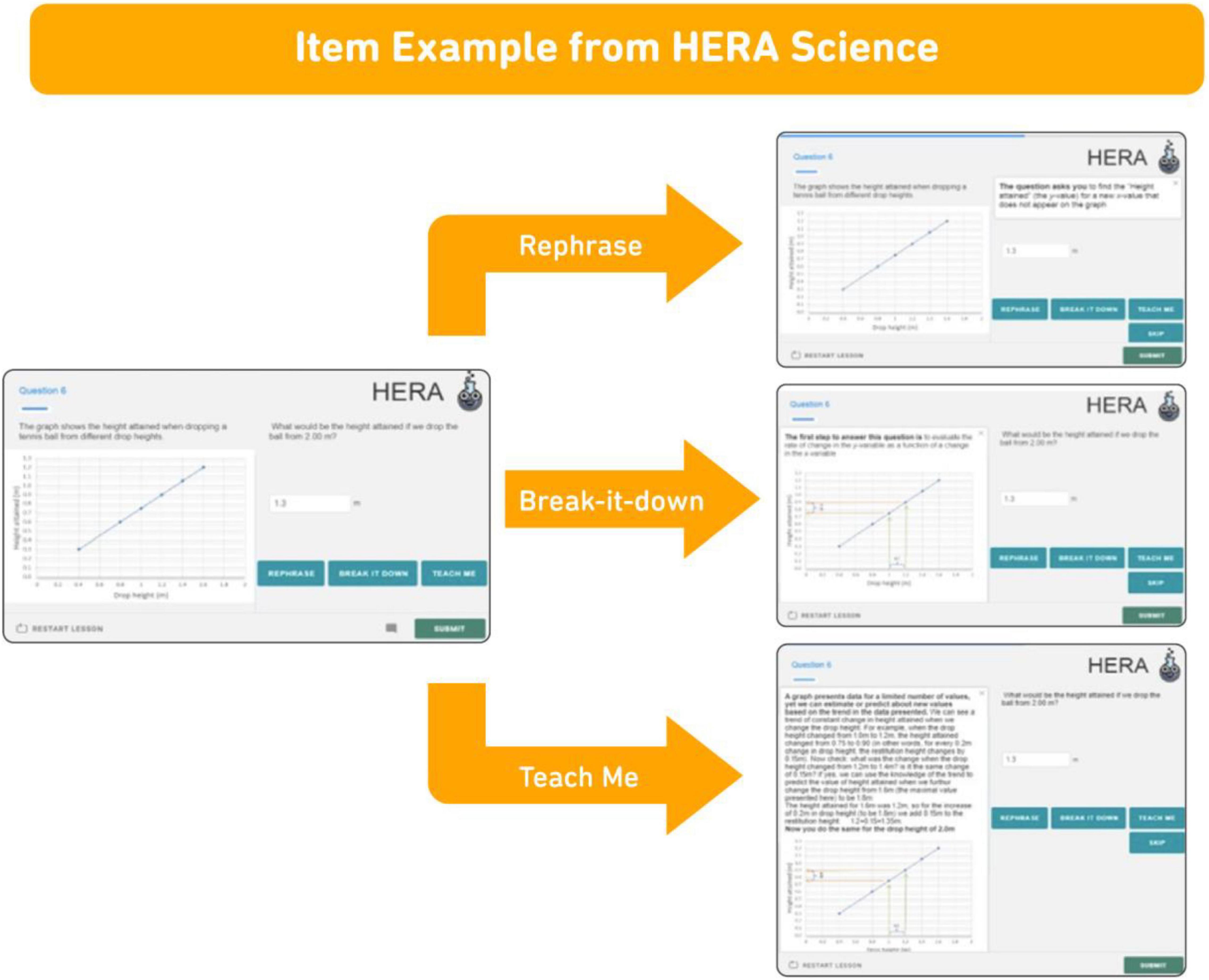
Figure 3. Sample item from HERA Science with the three adaptive learning scaffolds to assist students. Reprinted with permission from ACT, Inc.
Expanded Evidence-Centered Design Framework
Holistic Educational Resources Assessment was designed using an e-ECD approach (Arieli-Attali et al., 2019) to elicit changes in the KSAOs through the completion of each interactive lesson. The system was designed so that task models collect evidence of learning and growth. Data are not only collected on how students respond to items, but data are collected regarding student growth and use of the supports. This dual concept of items partnered with learning supports is termed an Assessment and Learning Personalized Interactive item (AL-PI). Because HERA lessons are adaptable to a student’s level, it can be used in the classroom, and educators can implement the lessons to align with the students’ learning needs (Rosen et al., 2020).
Computational Psychometrics Framework
As HERA is a learning and formative assessment prototype, it does not yet provide a traditional score. Nevertheless, it is important to understand how students respond to the simulations and items, and whether they respond consistently. To investigate student responses, Rosen et al. (2020) conducted a pilot study with 2,775 adult participants. Participants were assigned to one of five conditions and completed three HERA lessons. Across all lessons and conditions, reliability was acceptable and comparable to other cognitive assessments (Cronbach’s Alpha = 0.76).
The proposed HERA measurement model was designed to assess knowledge when feedback and hints were provided. Examples of previous work in this area are assessing partial knowledge (Ben-Simon et al., 1997), assessing knowledge when feedback and multiple attempts are provided (Attali and Powers, 2010; Attali, 2011), and assessing knowledge/ability when a hint is used (Bolsinova and Tijmstra, 2019). In addition, ACT is considering the application of the learning models; these include the Bayesian Knowledge Tracing applied to a subset of (correct/incorrect) responses and the Elo algorithm. The Elo algorithm was developed to track and calibrate rankings of chess players (Elo, 1978). An example of the Elo algorithm applied in an educational context can be found in Pelánek (2016) and in von Davier et al. (2019a). For the HERA data, an Elo-like algorithm was considered to estimate the values in a model that is inspired by the linear logistic test model (LLTM) (Pelánek, 2016, 2017). Exploratory data mining was also planned to investigate whether different learners use different strategies and to evaluate which of these strategies lead to better learning outcomes. Work is still in progress on the prototype, and it is too early to say which approach will be chosen.
Security Framework
The HERA security framework is designed to protect the learner registration system, HERA content and learning scaffolds, and PII. As HERA was designed to be a learning system, the need to minimize cheating behaviors and content theft is not as great as for an assessment program. At the same time, it is imperative that system security protects content and learner data so as to provide valid evidence of learning and to ensure that learner data is not compromised. To ensure that all PII is protected, the system is designed to conform to the requirements of the GDPR.
The Duolingo English Test
The Duolingo English Test is a digital-first, computer adaptive, high-stakes language assessment measuring English language proficiency (Settles et al., 2020). The primary test use is to provide information to determine whether an L2 English learner (i.e., a person learning English as a second language) has sufficient English proficiency to be admitted to an English-medium post-secondary institution. The test is aligned with Duolingo’s broader social mission to lower barriers to assessment and create educational opportunities for English language learners everywhere, while providing a positive test experience (Burstein et al., 2022; Cardwell et al., 2022). To that end, the test is offered at a low cost ($49 USD); test-takers can access the test online 24/7 at home or in another location of their choosing. Because the test is computer adaptive, testing time is only one hour, which is shorter than comparable tests. The shorter time supports test-takers who may have physical or cognitive constraints and are therefore unable to sit for longer time periods. The test also offers free, online test readiness resources and an automatically scored practice test, which assists test-taker familiarity with item types. These features promote accessibility and improve educational opportunities.
Language Assessment Design Framework
The language assessment design framework includes the following processes: (a) domain definition, (b) item design, (c) development of item content, (d) evidence specification, and (e) development of user experience and accessibility.
Domain Definition
The Duolingo English Test’s constructs are informed by, and grounded in, English language learning and assessment theory (Chalhoub-Deville, 2009; Bachman and Palmer, 2010; Chalhoub-Deville and O’Sullivan, 2020). Consequently, subconstructs identified for design include speaking, writing, reading, and listening, as well as relevant skill interactions (i.e., a task might involve both reading and writing). The test is aligned with the Common European Framework of Reference (CEFR; Council of Europe, 2020), which identifies six levels of language proficiency from Basic to Proficient.
Item Design
Items are designed to measure English language proficiency subconstructs (i.e., independent and integrated speaking, writing, reading, and listening skills). Items are aligned to the CEFR statements for an academic setting where digital interactions (e.g., reading a digital publication, writing an email to a university administrator) are used frequently.
Item design operationalizes constructs and consists of identifying the critical attributes of real-world language usage that can be replicated across numerous language tasks. Currently, the Duolingo English Test assesses test-takers skills in speaking, listening, reading, and writing. The assessment designers are striving to develop items that also assess test-takers skill in using language that includes the relationships of interlocutors (e.g., the power relationship between teacher and student). Additionally, as part of the item design process, when new items are developed but prior to their inclusion in the item pool, a formal fairness review process occurs. The formal fairness review process is adapted from Zieky (2013, 2015).
Development of Item Content
The system leverages emerging technologies for automated item generation, scoring, and difficulty prediction (Settles et al., 2020). Human-in-the-loop AI incorporating NLP with ML is applied to generate items at scale. The automated item generation processes further provide an estimate of item difficulty based on CEFR alignment.
Evidence Specification
The Duolingo English Test designers identify specific evidence that needs to be collected for each item type. The test designers consider evidence regarding test-takers’ response processes (e.g., writing keystroke logs, time stamps) and products (e.g., written text and speaking output). Test-taker product data provide the critical evidence required to evaluate score reliability and the validity of score interpretations.
User Experience and Accessibility
Within the design framework, designers evaluate issues and make decisions regarding the test interface and administrative process. The goal is to achieve a seamless user experience that minimizes the extent to which construct-irrelevant variance affects scores. To illustrate, if the user interface potentially confuses test-takers and hinders their ability to focus, their scores may be more representative of the problems with the user interface than of their English language proficiency and lead to inaccurate score interpretations and decisions.
To assist test-takers in navigating the user interface and in understanding the item types, the Duolingo English Test provides test-takers with a no-cost practice assessment. The practice assessment provides test-takers with the opportunity to practice responding to items using an identical interface for all item types. Following a practice test, the test-taker receives an estimated range of their possible score. The program further provides written information and videos to assist test-takers in understanding the different item types and the skills that they are designed to measure.
Test accessibility has two components. The first component is making test administration as convenient as possible for test-takers. This component is accomplished by the Duolingo English Test being available to test-takers anytime and anywhere at a relatively low cost. A second component of accessibility is the availability of sufficient accommodations to meet test-takers’ learning and testing needs. The Standards (American Educational Research Association, 2014) emphasize that testing programs should strive to provide all test-takers with appropriate accommodations to ensure that their test scores represent their performance on the constructs and that scores are not conflated with construct irrelevant variance due to lack of or ineffective accommodations.
Expanded Evidence-Centered Design Framework
The e-ECD framework includes the Proficiency model, the Task model, and the Evidence model. While the e-ECD framework provides both a learning and assessment path, the Duolingo English Test currently leverages only the assessment branch.1 The learning branch currently serves as a placeholder as the test continues to develop (Burstein et al., 2022). In e-ECD, item configurations are defined, and response data are collected and leveraged to create construct-relevant features that are used to model test-taker performance. NLP is leveraged to develop feature measures. For example, NLP can identify the writing features that may represent test-taker writing quality. Examples include grammatical errors in writing and mispronunciations in speech, and the extraction of positive characteristics of quality writing and speaking (such as lexical sophistication).
Computational Psychometrics Framework
In the CP framework, feature measures are developed from the raw test-taker response data and used to model test-taker performance with respect to a proficiency model. For the Duolingo English Test, statistical and AI modeling approaches have been used to develop features of the complex multimodal responses (writing, speech) and to fit appropriate models for these discrete or continuous data (Settles et al., 2020). Traditional psychometric studies evaluating scores from the Duolingo English Test indicate that scores are reliable (indices range from 0.80 to 0.96), and they support score-based interpretations (Langenfeld and Oliveri, 2021; Langenfeld et al., 2022).
In addition, to maintain the integrity of scores and ensure that the system is functioning as intended, the Duolingo English Test developed a bespoke quality control system—the Analytics for Quality Assurance in Assessment (AQuAA) system (Liao et al., 2021). The AQuAA system continuously monitors test metrics and trends. It has an interactive dashboard that integrates data mining techniques with psychometric methods, and it supports human expert monitoring to evaluate the interaction between tasks, test sessions, scoring algorithms, and test-taker responses and actions. The monitoring system allows for the analysis of data in real time, ensuring that problems are addressed immediately and score integrity is ensured (Liao et al., 2021).
Security Framework
As Duolingo English Test scores are used for high-stakes decisions, the security framework holds a critical role. Security issues begin when a test-taker first enters the system to register and continues through score reporting and data storage (LaFlair et al., 2022). The Duolingo registration system requires that perspective test-takers present a government-issued photo identification, and then they must provide specific demographic information including gender, date of birth, country of origin, and first language. For online tests, personal authentication is critical. Personal authentication is the process that ensures that the person who begins the test and is at the workstation throughout testing is the same person whose name is on the registration and identification documents (Foster, 2015). For online testing, the most common form of cheating is to have someone other than the registered test-taker sit for the assessment (Langenfeld, 2020). During Duolingo English Test administration, test-takers’ actions are monitored through human-in-the-loop AI proctoring. Problematic test-taker behaviors are flagged and reviewed by human proctors before a score is issued.
The Duolingo English Test designers formulated design decisions based on security requirements that would assist in ensuring the validity of score-based interpretations. Designers built the test development system to minimize the potential of cheating and theft. The application of automated item generation, which enabled the development of a large item pool, along with the application of CAT has resulted in some of the lowest item exposure rates and lowest item overlap rates in the testing industry (LaFlair et al., 2022). The low exposure rates and the low item overlap rates discourages the stealing of content as it is unlikely that stolen content would assist test-takers. Collectively, the design of the Duolingo English Test coupled with human-in-the-loop AI minimizes cheating and deters the stealing of content.
The Duolingo English Test is fully compliant with the GDPR. In addition, test-takers may request a copy of their test data, or they may request that Duolingo delete their data from the system. All test-related data, including photos and videos, are encrypted and stored in a secure location that only a limited number of employees can access (LaFlair et al., 2022).
Table 1 provides an overview of HERA and the Duolingo English Test, including their respective purposes and the contributions of the design and measurement frameworks to support the validity arguments.
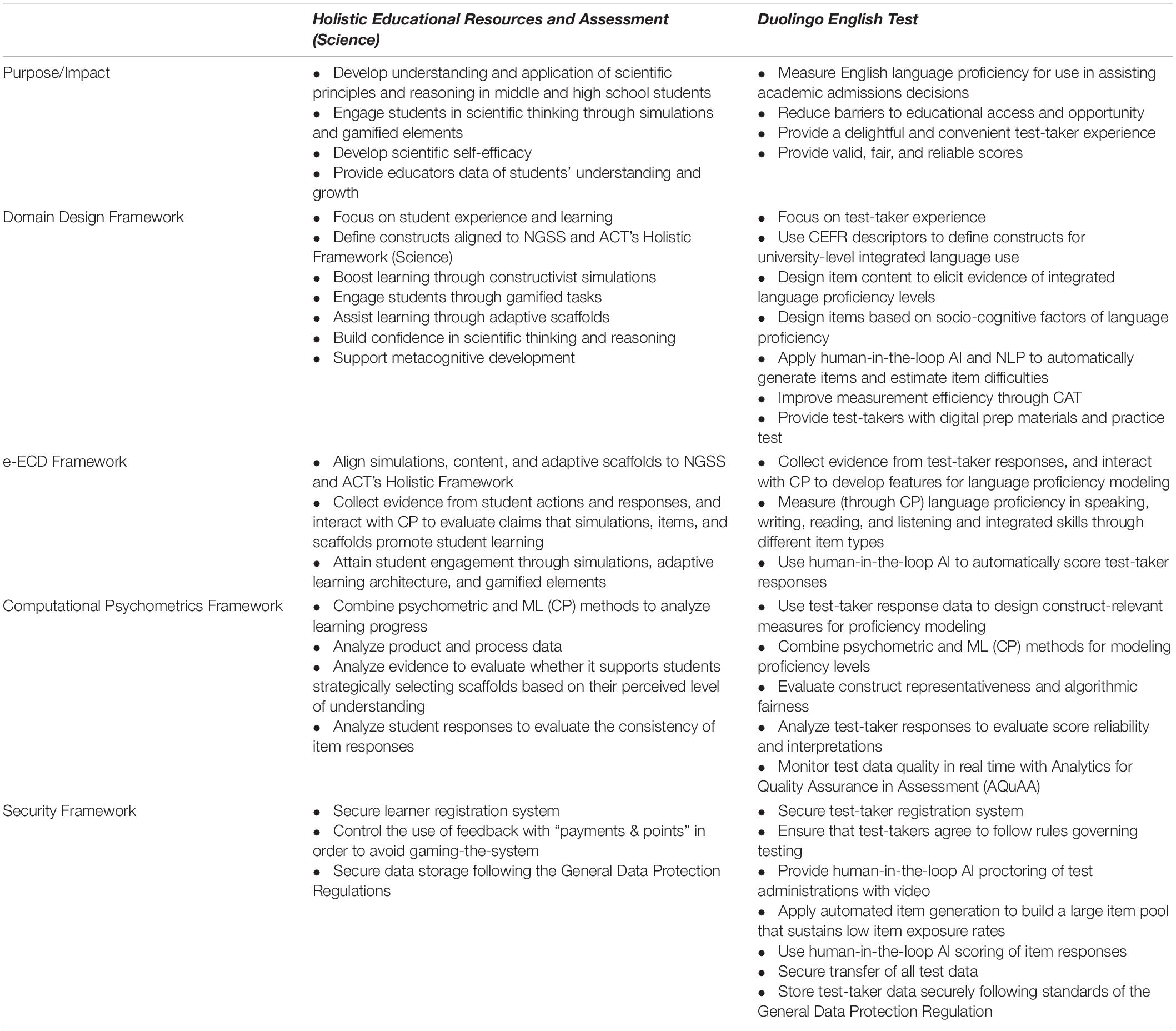
Table 1. Features of the digital-first design and measurement frameworks applied to the Holistic Educational Resources and Assessment (Science) and the Duolingo English Test.
Digital-First Systems and Looking to the Future
Combining learning activities with short formative assessments generally leads to improved learning outcomes, better student engagement, and the development of life-long learning skills (Tomlinson, 2004; Linn and Chiu, 2011; Richman and Ariovich, 2013; Amasha et al., 2018). Although computer technology has been widely embedded in education for more than 30 years, only recently has digital technology been applied to develop blended leaning and assessment activities. Emerging digital technologies that leverage advances in AI and measurement (such as computational psychometrics) have made possible the development of high-quality personalized learning and assessment platforms at scale (Laurillard et al., 2018). Collison (2021) argues that education is at an inflexion point. He maintains that technology has enabled educators to provide learners and test-takers with digitally based authentic learning and assessment activities, adapted to the individual’s needs, with near immediate feedback. This digitally based learning model has tremendous appeal to both educators and students. Digital learning and/or assessment systems are being designed by large organizations such as the National Assessment of Educational Progress (2022) in the United States, RM Education (2022) in the United Kingdom, and the Program for International Student Assessment (PISA) (OECD, 2022). In addition, local school districts and universities are studying how they might effectively implement digitally based learning and assessment systems (Gunder et al., 2021).
As digitally based learning and assessment systems are designed for various educational and training programs, designers should be mindful of several concerns. To achieve the full potential of digital-first LASs and gain widespread acceptance, they must provide transparent measurement, protect privacy, and safeguard fairness (von Davier et al., 2019b). Educators and other stakeholders who make decisions based on outcomes or scores derived from digital-first LAS require measurement transparency. Measurement transparency includes access to information concerning the system interfaces, learning and assessment objectives, validation evidence, and appropriate uses and interpretations of information. In addition to measurement transparency, learners and test-takers require privacy protections. Learners and test-takers need assurances that their personally identifiable information is protected, and that data transfers and storage systems have sufficient safeguards (Langenfeld, 2020). Additionally, learners and test-takers must be informed if the LAS uses video or data forensics to identify unauthorized behaviors. Lastly, digital-first LAS must be designed to continuously monitor and evaluate fairness across the whole ecosystem. Fairness issues include multiple conditions. Digital-first LAS should develop fairness agendas that continuously monitor conditions that include evaluating algorithmic fairness; bias concerning gender, ethnic, or racial groups; and accessibility and accommodations.
To achieve the potential of digital-first LAS, when designers make system decisions, they must prudently evaluate the effect of that decision on measurement quality, accessibility, and score and content security. A natural tension exists between maximizing accessibility and maintaining security, but both are essential to the learning and assessment system. Notwithstanding these competing requirements, the framework we have articulated provides a model that promotes accessibility, maintains security, and attains the potential of digital-first LAS.
Conclusion
In this paper, we have proposed an ecosystem for the design of digital-first LAS and emphasized that both theoretical and technological integration are vital to digital-first LAS. Using the ecosystem to build these systems can address challenges to achieving more democratic and equitable learning opportunities. The full ecosystem adapted here for learning and assessment from Burstein et al. (2022) consists of (a) the domain design framework, (b) the expanded Evidence-Centered Design framework, (c) the computational psychometric framework, and (d) the test security framework.
We presented the digital-first LAS ecosystem and the two exemplars to provide designers with guidance for developing future learning and assessment systems. In doing so, we presented limited information regarding the efficacy and validity of either HERA Science or the Duolingo English Test. Whereas current studies evaluating the validity of the use of HERA Science are limited (Rosen et al., 2020; Arieli-Attali et al., 2022), we cited numerous studies evaluating the validity of test score interpretations for the Duolingo English Test (Settles et al., 2020; von Davier and Settles, 2020; Cardwell et al., 2022; LaFlair et al., 2022; Langenfeld et al., 2022). We encourage researchers and designers to review these studies to better understand its score efficacy and validity. In this paper, we supplied limited information on the development of accommodations needed to assist test-takers with special needs. Developing appropriate accommodations for digital-first assessments is not a trivial matter, and while discussion exists that describes successful adoption to the Burstein et al. (2022) ecosystem (Care and Maddox, 2021), future research detailing their successful adoption should be conducted. Lastly, we provided limited information about the psychometric models that have been applied to derive digital-first LAS score information. The evaluation of psychometric models for use in digital-first LAS constitutes a broad area of study. [See the recent edited volume on computational psychometrics (von Davier et al., 2021) for examples of models applied to digital-first LAS.].
Our goal for this paper was to articulate an ecosystem that others can apply, as well as adapt to future digital-first LAS. With additional research and dissemination, digital-first LAS potentially can transform educational and learning systems to be more effective, accessible, equitable, and democratic.
Data Availability Statement
The original contributions presented in the study are included in the article/supplementary material, further inquiries can be directed to the corresponding author.
Author Contributions
TL and AvD made a substantial contribution to the conceptualization and writing of the manuscript, worked on the draft and revision, approved submitted version, and accountable for its accuracy and integrity. JB made a substantial contribution to the conceptualization and writing of the manuscript, wrote portions of the draft and revision, approved submitted version, and accountable for its accuracy. All authors contributed to the article and approved the submitted version.
Conflict of Interest
TL was employed by TEL Measurement Consulting LLC. JB and AvD were employed by Duolingo Inc.
Publisher’s Note
All claims expressed in this article are solely those of the authors and do not necessarily represent those of their affiliated organizations, or those of the publisher, the editors and the reviewers. Any product that may be evaluated in this article, or claim that may be made by its manufacturer, is not guaranteed or endorsed by the publisher.
Acknowledgments
We want to thank Ramsey Cardwell and Maria Elena Oliveri for reviewing an earlier version of this manuscript. We also thank Sophie Wodzak for designing the three figures that appear in this manuscript. We lastly want to thank the editor and the three reviewers whose comments assisted us in clarifying several concepts and in developing a more complete presentation.
Footnotes
- ^ The Duolingo English Test does not currently include a learning branch. Despite this, Duolingo has developed the Duolingo Learning App, which is designed to assist learners in building their English language skills. The future goal is to merge learning activities with assessment preparation aligned to test content and thereby close the loop to be fully representative of the e-ECD framework.
References
Amasha, M. A., Abougalala, R. A., Reeves, A. J., and Alkhalaf, S. (2018). Combining online learning and assessment in synchronization form. Educ. Inf. Technol. 23, 2517–2529. doi: 10.1007/s10639-018-9728-0
American Educational Research Association, American Psychological Association, and National Council on Measurement in Education (2014). Standards for Educational and Psychological Testing. Washington, DC: American Educational Research Association.
Arieli-Attali, M., Ward, S. J., Simmering, V., Rosen, Y., and von Davier, A. A. (2022). “Leveraging ideas from adaptive testing to adaptive learning: the HERA showcase,” in Enhancing Effective Instruction and Learning Using Assessment Data. The MARCES Book Series, eds H. Jiao and R. W. Lissitz (Charlotte, NC), 215–235. Available online at: https://www.infoagepub.com/products/Enhancing-Effective-Instruction-and-Learning-Using-Assessment-Data (accessed January 15, 2022).
Arieli-Attali, M., Ward, S., Thomas, J., Deonovic, B., and von Davier, A. A. (2019). The expanded evidence-centered design (e-ECD) for learning and assessment systems: a framework for incorporating learning goals and processes with assessment design. Front. Psychol. 10:853. doi: 10.3389/fpsyg.2019.00853
Attali, Y. (2011). Immediate feedback and opportunity to revise answers: application of a graded response IRT model. Appl. Psychol. Meas. 35, 472–479. doi: 10.1177/0146621610381755
Attali, Y., and Powers, D. (2010). Immediate feedback and opportunity to revise answers to open-ended questions. Educ. Psychol. Meas. 70, 22–35. doi: 10.1177/0013164409332231
Bachman, L. F., and Palmer, A. S. (2010). Language Assessment in Practice. Oxford: Oxford University Press.
Ben-Simon, A., Budescu, D. V., and Nevo, B. (1997). A comparative study of measures of partial knowledge in multiple-choice tests. Appl. Psychol. Meas. 21, 65–88. doi: 10.1177/0146621697211006
Blayone, T. J. B., vanOostveen, R., Barber, W., DiGiuseppe, M., and Childs, E. (2017). Democratizing digital learning: theorizing the fully online learning community model. Int. J. Educ. Technol. High. Educ. 14:13. doi: 10.1186/s41239-017-0051-4
Bolsinova, M., and Tijmstra, J. (2019). Modeling differences between response times of correct and incorrect responses. Psychometrika 84, 1018–1046. doi: 10.1007/s11336-019-09682-5
Burstein, J., LaFlair, G. T., Kunnan, A. J., and von Davier, A. A. (2022). A Theoretical Assessment Ecosystem for a Digital-First Assessment – The Duolingo English Test. Available online at: https://duolingo-papers.s3.amazonaws.com/other/det-assessment-ecosystem.pdf (accessed February 3, 2022).
Camara, W., O’Connor, R., Mattern, K., and Hanson, M. A. (2015). Beyond Academics: A Holistic Framework for Enhancing Education and Workplace Success. ACT Research Report Series 2015. Available online at: https://www.act.org/content/dam/act/unsecured/documents/ACT_RR2015-4.pdf (accessed September 15, 2016).
Cardwell, R., LaFlair, G. T., and Settles, B. (2022). Duolingo English Test: Technical Manual. Available online at: https://duolingo-papers.s3.amazonaws.com/other/det-technical-manual-current.pdf (accessed February 3, 2022).
Care, N., and Maddox, B. (2021). Improving Test Validity and Accessibility With Digital-First Assessments. Available online at: https://duolingo-papers.s3.amazonaws.com/other/det-improving-test-validity.pdf (accessed January 10, 2022).
Chalhoub-Deville, M. (2009). The intersection of test impact, validation, and educational reform policy. Annu. Rev. Appl. Linguist. 29, 118–131. doi: 10.1017/s0267190509090102
Chalhoub-Deville, M., and O’Sullivan, B. (2020). Validity: Theoretical Development and Integrated Arguments. Sheffield: Equinox Publishing Limited.
Chapelle, C., Enright, M., and Jamieson, J. (Eds.) (2008). Building a Validity Argument for the Test of English as a Foreign Language. New York, NY: Routledge.
Cipresso, P., Colombo, D., and Riva, G. (2019). Computational psychometrics using psychophysiological measures for the measurement of acute mental stress. Sensors 19:781. doi: 10.3390/s19040781
Collison, P. (2021). The Most Authentic Assessment is Digital. The Digital Assessment News. RM Results. Available online at: https://blog.rmresults.com/the-most-authentic-assessment-is-digital (accessed October 10, 2021).
Cooper, A. (2014). Learning Analytics Interoperability – The Big Picture in Brief. Learning Analytics Community Exchange. Available online at: https://web.archive.org/web/20180415023305id_/http://laceproject.eu/publications/briefing-01.pdf (accessed November 15, 2021).
Council of Europe (2020). Common European Framework of Reference for Languages: Learning, teaching, assessment – Companion volume. Strasbourg: Council of Europe Publishing.
Dadey, N., Lyons, S., and DePascale, C. (2018). The comparability of scores from different digital devices: a literature review and synthesis with recommendations for practice. Appl. Meas. Educ. 31, 30–50. doi: 10.1080/08957347.2017.1391262
Dale, G., Joessel, A., Bavelier, D., and Green, C. S. (2020). A new look at the cognitive neuroscience of video game play. Ann. N. Y. Acad. Sci. 1464, 192–203. doi: 10.1111/nyas.14295
DiCerbo, K. E., and Behrens, J. T. (2012). “Implications of the digital ocean on current and future assessments,” in Computers and Their Impact on State Assessment: Recent History and Predictions for the Future, eds R. Lissitz and H. Jiao (Charlotte, NC: Information Age Publishing), 273–306. doi: 10.1007/s10661-015-4690-4
Educational Testing Service (2016). ETS International Principles for the Fairness of Assessments: A Manual for Developing Locally Appropriate Fairness Guidelines for Various Countries. Princeton, NJ: ETS.
European Union (2016). General Data Protection Regulation (GDPR). Available online at: https://gdpr-info.eu/ (accessed April 7, 2020).
Ferrara, S., Lai, E., Reilly, A., and Nichols, P. D. (2017). “Principled approaches to assessment design, development, and implementation,” in The Handbook of Cognition and Assessment: Frameworks, Methodologies, and Applications, eds A. A. Rupp and J. P. Leighton (Chichester: Willey), 41–72. doi: 10.1002/9781118956588.ch3
Foster, D. (2015). The Language of Security and Test Security: Caveon White Paper. Available online at: https://www.caveon.com/wp-content/uploads/2014/03/The-Language-of-Security-and-Test-Security-White-Paper-Foster.pdf (accessed April 12, 2020).
Garrison, W. H. (2008). Democracy and education: empowering students to make sense of their world. Phi Delta Kappan 89, 347–348. doi: 10.1177/003172170808900507
Gronsund, T., and Aanestad, M. (2020). Augmenting the algorithm: emerging human-in-the-loop work configurations. J. Strateg. Inf. Syst. 29:101614. doi: 10.1016/j.jsis.2020.101614
Gunder, A., Vignare, K., Adams, S., McGuire, A., and Rafferty, J. (2021). Optimizing High-Quality Digital Learning Experiences: A Playbook for Faculty. Every Learner Everywhere. Available online at: https://www.everylearnereverywhere.org/wp-content/uploads/ele_facultyplaybook_2021_v3a_gc.pdf (accessed November 15, 2021).
Jackman, J. A., Gentile, D. A., Cho, N.-J., and Park, Y. (2021). Addressing the digital skills gap for future education. Nat. Hum. Behav. 5, 542–545. doi: 10.1038/s41562-021-01074-z
Jiao, H., and Lissitz, R. W. (Eds.) (2017). Test Fairness in the New Generation of Large-Scale Assessment. Charlotte, NC: International Age Publishing.
K12 School (2021). How Our Curriculum is Developed. Available online at: https://www.k12.com/about-k12/how-our-curriculum-is-developed.html (accessed November 15, 2021).
Kane, M. T. (1992). An argument-based approach to validity. Psychol. Bull. 112, 527–535. doi: 10.1037/0033-2909.112.3.527
Kane, M. T. (2013). Validating the interpretations and uses of test scores. J. Educ. Meas. 50, 1–73. doi: 10.1111/jedm.12000
Kingston, N. M., Karvonen, M., Thompson, J. R., Wehmeyer, M. L., and Shogren, K. A. (2017). Fostering inclusion of students with significant cognitive disabilities by using learning map models and map-based assessments. Inclusion 5, 110–120. doi: 10.1352/2326-6988-5.2.110
Kloper, E., and Thompson, M. (2019). “Game-based learning in science, technology, engineering, and mathematics,” in Handbook of Game-Based Learning, eds J. L. Plas, R. E. Mayer, and B. D. Homer (Cambridge, MA: The MIT Press), 387–409. doi: 10.2196/20537
Knight, T., and Pearl, A. (2000). Democratic education and critical pedagogy. Urban Rev. 32, 197–226.
Koedinger, K. R., Corbett, A. T., and Perfetti, C. (2012). The knowledge-learning-instruction framework: bridging the science-practice chasm to enhance robust student learning. Cogn. Sci. 36, 757–798. doi: 10.1111/j.1551-6709.2012.01245.x
Koehler, M., and Mishra, P. (2009). What is technological pedagogical content knowledge (TPACK)? Contemp. Issues Technol. Teach. Educ. 9, 60–90.
Korte, M. (2020). The impact of the digital revolution on human brain and behavior: where do we stand? Dialogues Clin. Neurosci. 22, 101–111. doi: 10.31887/DCNS.2020.22.2/mkorte
Kunnan, A. J. (2018). “Assessing languages for specific purposes,” in Presentation at the ALTE Cluj Meeting. Available online at: https://www.alte.org/resources/Documents/ALTE%20talk%20on%20LSP,%20April%202018.pptx%20v4.pdf (accessed September 20, 2020).
LaFlair, G. T., Langenfeld, T., Baig, B., Horie, A. K., Attali, Y., and von Davier, A. A. (2022). Digital-first assessments: a security framework. J. Comput. Assist. Learn. Available online at: https://onlinelibrary.wiley.com/doi/10.1111/jcal.12665 (accessed January 15, 2022).
Langenfeld, T. (2020). Internet-based proctored assessments: security and fairness issues. Educ. Meas. Issues Pract. 39, 24–27. doi: 10.1111/emip.12359
Langenfeld, T., and Oliveri, M. E. (2021). The Duolingo English Test: Validity Evidence and the Requirements of the Standards. Pittsburgh, PA: Duolingo, Inc. Unpublished report.
Langenfeld, T., Gao, X., and Oliveri, M. E. (2022). Analyzing Sources of Variance to Evaluate the Validity and Fairness of Duolingo English Test Scores. Unpublished report. Pittsburgh, PA: Duolingo, Inc.
Laurillard, D., Kennedy, E., and Wang, T. (2018). “How could digital learning at scale address the issue of equity in education?,” in Learning at Scale for the Global South. Digital Learning for Development, Eds C. P. Lim and V. L. Tinio, (Quezon City, PH: Foundation for Information Technology Education and Development)
Lead State Partners (2013). Next Generation Science Standards. Washington, DC: NextGen Science Publications
Liao, M., Patton, J., Yan, R., and Jiao, H. (2021). Mining process data to detect aberrant test takers measurement. Interdiscip. Res. Perspect. 19, 93–105. doi: 10.1080/15366367.2020.1827203
Linn, M. C., and Chiu, J. (2011). Combining learning and assessment to improve science education. Res. Pract. Assess. 6, 5–14.
McCarthy, A. D., Yancy, K. P., LaFlair, G. T., Egbert, J., Liao, M., and Settles, B. (2021). “Jump-starting item parameters for adaptive language tests,” in Proceedings of the 2021 Conference on Empirical Methods in Natural Language Processing, (Stroudsburg, PA: Association for Computational Linguistics).
Mislevy, R. J. (2018). Sociocognitive Foundations of Educational Measurement. New York, NY: Routledge.
Mislevy, R. J., Almond, R. G., and Lukas, L. A. (2003). A Brief Introduction to Evidence-Centered Design. ETS Research Report, RR 03-16. Princeton, NJ: ETS.
Mislevy, R. J., and Elliot, N. (2020). “Ethics, psychometrics, and writing assessment: a conceptual model,” in After Plato: Rhetoric, Ethics, and the Teaching of Writing, eds J. Duffy and L. Agnew (Logan, UT: Utah State University Press), 143–162. doi: 10.7330/9781607329978.c008
Mislevy, R. J., and Haertel, G. D. (2006). Implications of Evidence-Centered Design for educational testing. Educ. Meas. Issues Pract. 25, 6–20. doi: 10.1111/j.1745-3992.2006.00075.x
Mislevy, R. J., Oranje, A., Bauer, M. I., von Davier, A. A., Hao, J., Corrigan, S., et al. (2016). Psychometric Considerations in Game-Based Assessments. Educational Testing Service Research. New York, NY: Institute of Play.
Mislevy, R. J., Steinberg, L. S., and Almond, R. G. (2002). “On the roles of task model variables in assessment design,” in Generating Items for Cognitive Tests: Theory and Practice, eds S. Irvine and P. Kyllonen (Hillsdale, NJ: Erlbaum), 97–128.
Moore, R., Vitale, D., and Stawinoga, N. (2018). The Digital Divide and Educational Equity: A Look at Students With Very Limited Access to Electronic Devices at Home. ACT Technical Report. Iowa City, IA: ACT.
National Assessment of Educational Progress (2022). Digitally Based Assessments: What’s Happening Now?. Washington, DC: National Center for Education Statistics.
OECD (2022). PISA 2025 Learning in the Digital World. Programme for International Student Assessment. Paris: OECD.
Ozersky, L. (2021). HERA Science – An Adaptive Learning System. Available online at: https://www.laurelozersky.com/hera.html (accessed December 1, 2021).
Pelánek, R. (2016). Applications of the Elo rating system in adaptive educational systems. Comput. Educ. 98, 169–179. doi: 10.1016/j.compedu.2016.03.017
Pelánek, R. (2017). Bayesian knowledge tracing, logistic models, and beyond: an overview of learner modeling techniques. User Model. User-Adapt. Interact. 27, 313–350. doi: 10.1007/s11257-017-9193-2
Pohan, C. A. (2012). Creating caring and democratic communities in our classrooms and schools. Child. Educ. 79, 369–373. doi: 10.1080/00094056.2003.10521237
Polyak, S. T., von Davier, A. A., and Peterschmidt, K. (2017). Computational psychometrics for the measurement of collaborative problem solving skills. Front. Psychol. 8:2029. doi: 10.3389/fpsyg.2017.02029
Rayon, A., Guenaga, M., and Nunez, A. (2014). “Ensuring the integrity and interoperability of educational usage and social data through Caliper framework to support competency-assessment,” in Proceedings of the 2014 IEEE Frontiers in Educational Conference (FIE) Proceedings, (Piscataway, NJ: IEEE).
Richman, W. A., and Ariovich, L. (2013). All-in-One: Combining Grading, Course, Program, and General Education Outcome Assessment. National Institute for Learning Outcomes Assessment. Champaign, IL: University of Illinois.
RM Education (2022). Modernizing Digital Assessment: Assessment from RM. Available online at: https://rmresults.com/ (accessed March 10, 2022).
Rosen, Y., Arieli-Attali, M., Ward, S., Seery, J., Simmering, V., Ozersky, L., et al. (2020). “HERA: exploring the power of adaptive scaffolding on scientific argumentation and modelling competencies in online learning systems,” in The Interdisciplinarity of the Learning Sciences, 14th International Conference of the Learning Sciences (ICLS) 2020, Vol. 3, eds M. Gresalfi and I. S. Horn (Nashville, TN: International Society of the Learning Sciences), 1665–1668.
Ryan, R. M., and Rigby, C. S. (2019). “Motivational foundations of game-based learning,” in Handbook of Game-Based Learning, eds J. L. Plass, R. E. Mayer, and B. D. Homer (Cambridge, MA: MIT Press).
Settles, B., LaFlair, G. T., and Hagiwara, M. (2020). Machine learning–driven language assessment. Trans. Assoc. Comput. Linguist. 8, 247–263. doi: 10.1162/tacl_a_00310
Shah, D. (2021). A Decade of MOOCs: A Review of Stats and Trends for Large-Scale Online Courses in 2021. EdSurge. Available online at: https://www.edsurge.com/news/2021-12-28-a-decade-of-moocs-a-review-of-stats-and-trends-for-large-scale-online-courses-in-2021?utm_campaign=site&utm_content=share-1229 (accessed October 10, 2021).
Sireci, S. G. (2021). NCME presidential address 2020: valuing educational measurement. Educ. Meas. Issues Pract. 40, 7–16. doi: 10.1111/emip.12415
Sondheim, M., Gardels, K., and Buehler, K. (1999). “GIS Interoperability,” in Geographic Information Systems 1: Principles and Technical Issues, eds P. Longley, M. Goodchild, D. Maguire, and D. Rhind (New York, NY: Wiley), 347–358.
Tomlinson, M. (2004). 14-19 Curriculum and Qualifications Reform: Final Report of the Working Group on 14-19 Reform. Technical Report. Department for Education and Skills (UK). Available online at: http://www.educationengland.org.uk/documents/pdfs/2004-tomlinson-report.pdf (accessed November 15, 2021).
U.S. Department of Education (2012). Assessment Interoperability Framework: Definitions and Requirements Document. Washington, DC: Department of Education.
van Laar, E., van Deursen, A. J. A. M., van Dijk, J. A. G. M., and de Haan, J. (2017). The relation between 21st-century skills and digital skills: a systematic literature review. Comput. Hum. Behav. 72, 577–588. doi: 10.1016/j.chb.2017.03.010
von Davier, A. A. (2017). Computational psychometrics in support of collaborative educational assessment. J. Educ. Meas. 54, 3–11. doi: 10.1111/jedm.12129
von Davier, A. A. (2021). What can Artificial Intelligence Teach Us? Invited Address to Brigham Education Institute – AI applications for assessment. Available online at: https://bei.brighamandwomens.org/event/ai-workshop-applications-assessment (accessed October 30, 2021).
von Davier, A. A., and Settles, B. (2020). “Dynamic testing and computational psychometrics,” in Paper presented at the Annual conference of the National Council for Measurement in Education. Virtual. Available online at: https://www.youtube.com/watch?v=dfN26b65adw (accessed September 15, 2020).
von Davier, A. A., Deonovic, B., Yudelson, M., Polyak, S. T., and Woo, A. (2019a). Computational psychometrics approach to holistic learning and assessment systems. Front. Educ. 4:69. doi: 10.3389/feduc.2019.00069
von Davier, A. A., Mislevy, R. J., and Hao, J. (Eds.) (2021). Computational Psychometrics: New Methodologies for a New Generation of Digital Learning and Assessment. New York, NY: Springer.
von Davier, A. A., Wong, P. C., Polyak, S., and Yudelson, M. (2019b). The argument for a “data cube” for large-scale psychometric data. Front. Educ. 4:71. doi: 10.3389/feduc.2019.00071
Way, W. D., Davis, L. L., Keng, L., and Strain-Seymour, E. (2016). “From standardization to personalization: the comparability of scores based on different testing conditions, modes, and devices,” in Technology in Testing: Improving Educational and Psychological Measurement, ed. F. Drasgow (New York, NY: Routledge), 260–284. doi: 10.1007/s12265-016-9720-2
Yan, D., Rupp, A. A., and Foltz, P. W. (Eds.) (2020). Handbook of Automated Scoring: Theory Into Practice. New York, NY: CRC Press Taylor and Francis Group.
Zieky, M. J. (2013). “Fairness review in assessment,” in APA Handbook of Testing and Assessment in Psychology, Test Theory and Testing and Assessment in Industrial and Organizational Psychology, Vol. 1, eds K. F. Geisinger, B. A. Bracken, J. F. Carlson, J.-I. C. Hansen, N. R. Kuncel, S. P. Reise, et al. (Washington, DC: American Psychological Association), 293–302. doi: 10.1037/14047-017
Keywords: digital-first learning and assessment systems, ecosystem, frameworks, design, equitable opportunities
Citation: Langenfeld T, Burstein J and von Davier AA (2022) Digital-First Learning and Assessment Systems for the 21st Century. Front. Educ. 7:857604. doi: 10.3389/feduc.2022.857604
Received: 19 January 2022; Accepted: 20 April 2022;
Published: 09 May 2022.
Edited by:
Mohammed Saqr, University of Eastern Finland, FinlandReviewed by:
Mariel Fernanda Musso, Centro Interdisciplinario de Investigaciones en Psicología Matemática y Experimental (CONICET), ArgentinaElizabeth Archer, University of the Western Cape, South Africa
Okan Bulut, University of Alberta, Canada
Copyright © 2022 Langenfeld, Burstein and von Davier. This is an open-access article distributed under the terms of the Creative Commons Attribution License (CC BY). The use, distribution or reproduction in other forums is permitted, provided the original author(s) and the copyright owner(s) are credited and that the original publication in this journal is cited, in accordance with accepted academic practice. No use, distribution or reproduction is permitted which does not comply with these terms.
*Correspondence: Thomas Langenfeld, telangenfeld@gmail.com