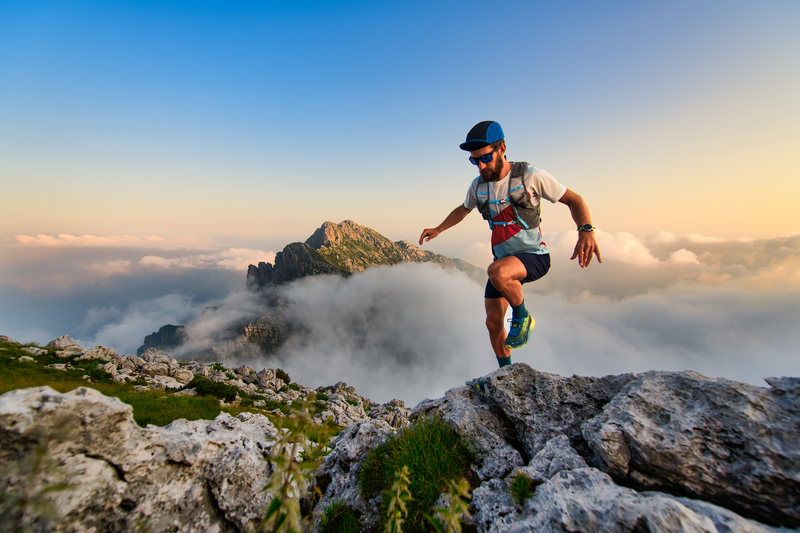
95% of researchers rate our articles as excellent or good
Learn more about the work of our research integrity team to safeguard the quality of each article we publish.
Find out more
ORIGINAL RESEARCH article
Front. Educ. , 28 April 2022
Sec. Digital Education
Volume 7 - 2022 | https://doi.org/10.3389/feduc.2022.847571
This descriptive study was conducted to evaluate the factors affecting the quality of online learning of Pakistani students during the COVID-19 pandemic. Developing economies such as Pakistan present a unique context to online education as there is limited access to technology, ITC services, and the internet for the general public. This paper integrates the factors affecting the online learning process of students while taking the situational factors as a boundary condition (moderating variable). Data were collected through a Appendix Questionnaire administered to students studying in different universities in Pakistan. Stepwise linear regression and PROCESS Macro by Hayes (2013) was used for data analysis. Results revealed that university support, instructors’ support, and motivational factors predicted the quality of online learning. The situational factors negatively influenced the relationship of instructors’ support and motivational factors and the quality of online learning. University support and the quality of online learning relationships were not moderated by situational factors.
The outbreak of COVID-19 was a significant event that pushed educational institutions to transition from the traditional classroom environment to the various online learning options. COVID-19 has affected all spheres of human life (Chang et al., 2020; Mokter, 2020; Wong et al., 2020), including the delivery of education and learning (Panesar et al., 2020; Laksana, 2021). To avoid the spread of COVID-19, the majority of the educational activities like classroom teaching, seminars, conferences, workshops, etc., were either delayed or canceled by the educational institutions (Khachfe et al., 2020; Patricia, 2020) till their online availability.
Online learning is the learning transferred through internet technologies by synchronous and asynchronous means. These modes help students interact with their teachers and colleagues while keeping a social distance (Dong et al., 2020). Online learning provides students with the opportunities to learn, interact, share their views, be independent in learning, and use time on their own (Azzi et al., 2021; Hwang et al., 2021). Using online technology to learn requires the students and teachers to have the ability to use technology to develop and maintain a sound social interaction (Andel et al., 2020). Apart from the effective use of technology, other factors such as the availability of suitable facilities, infrastructure and the financial state of the students also play an important role in online learning (Rusli et al., 2020; Laksana, 2021).
In this era of rapid development of Information and Communication Technologies (ICT) and their various applications, it is crucial to look at the quality of online learning (Zhafira et al., 2020). Information technology systems provide a wide range of facilities that allow for the fast, effective, and efficient delivery of education online. While the prevailing pandemic (COVID-19) has forced students to use Information and Communication Technologies (ICT) (Mustakim, 2020), ICT systems enable students to learn more varied material while enjoying time and space flexibility (Laksana, 2021). Moreover, the online text is generally aided by audio-visual enrichments (Hasibuan, 2016) which further expand the learning experience.
Although online resources offer countless benefits for students, the effectiveness and efficiency of online learning are affected by many variables (Pratiwi, 2020). These variables include the role of faculty, support from the university, learner readiness, and motivation. These variables can be perceived differently by different students. Many students face several challenges that influence the smooth flow of educational services (Laksana, 2021). Students in regions with low internet quality and electricity outages generally experience a lower quality of online learning than others. The role of faculty, support available from the university, study environment at home, and motivational factors are all important factors that can affect the quality of online learning.
Students, as learners, are central to online learning and experience the greatest impact of online learning (Chen et al., 2021; Laksana, 2021). Furthermore, the faculty members also play a pivotal role in online learning. They have to prepare the relevant teaching material and presentations that are based on facts and figures and are compatible with the online environment. Similarly, online educational services cannot be delivered without the cooperation of the university authorities. Several resources are required for the smooth online delivery of learning resources as well as the learning process itself. The application of online teaching and learning in most developing countries has been a consequence of the constraints caused by the COVID-19 pandemic (Suprianto et al., 2020; Suryaman et al., 2020). However, several issues such as limited internet access, discontinuous availability of electricity, and the lack of support from family and university (Djidu et al., 2021) may have hampered the students’ learning journey. It is necessary to investigate the students’ perceptions regarding their online learning experience during the COVID-19 pandemic to assess the quality of online learning in the context of a developing economy.
Earlier qualitative studies (Khalil et al., 2020; Djidu et al., 2021; Laksana, 2021) have empirically examined the set of factors influencing the quality of online learning. The literature suggests that there is much to learn about online learning environments. Previous studies have demonstrated that these environments have both positive and negative impacts on students’ effectiveness and achievements (Franklin et al., 2001). Examining and illuminating the perspective of students directly involved in online courses could offer useful insights by identifying the factors responsible for the quality of online learning. This research focused on the students using asynchronous technology for learning to assess the quality of learning in online learning environments. In previous studies, students preferred web-based learning to face-to-face, as it offered convenience and flexibility. Students have also reported that web-based learning opened up a whole new world of information to them (Young and Norgard, 2006).
This study is different from the other studies in the area in many ways; firstly, this study has been conducted in a country with limited energy resources that restrict the continuous flow of online learning. Secondly, this study was conducted during the COVID-19 pandemic lockdown. The lack of face-to-face interaction with teachers and heavy dependence on technology use demanded heavy investments in technology by both the universities and students’ families. This study highlights the various factors influencing online learning during the COVID-19 pandemic. It also provides insights for educational institutions to help them effectively manage the quality of learning and facilitate the students’ educational journey.
The aim of current investigation is to study the impact of university support, instructor’s support and motivational factors on quality of online learning of students during the COVID-19 pandemic lockdown and whether situational factors play moderating role. To achieve the objectives of proposed study, quantitative research design is adopted. The first section is regarding theoretical framework and literature review, followed by methodology, data analysis and results sections. Next, based on results, discussion, implications, limitations and future directions are provided.
Literature on the topic is full of contradictory accounts of the online learning experience of students, therefore, it is very important to conduct an empirical study of the quality of online learning. Students have reported web-based learning to be more convenient than face-to-face learning as it offered flexibility (Soffer et al., 2019). However, O’Malley and McGraw found that students did not believe that they learned more in online courses as they were more concerned about how to contribute towards class discussions in online courses than in their face-to-face classes. The quality of online education determines students’ satisfaction levels (Tj and Tanuraharjo, 2020) and adds to the brand value of the institutions (Shehzadi et al., 2020). The quality of online education refers to the ease of use and access to just-in-time support by both instructors and institutions that lead to greater student satisfaction. Online education is the learning transferred through internet technologies by synchronous and asynchronous means. The greater satisfaction achieved through such means will be referred to as the quality of online education. Online learning can rightly be called distance learning aided with the use of the internet (Yang and Cornelius, 2004).
Kearsley (2000) viewed a good-quality online course as having ten critical characteristics; “the content, pedagogy, motivation, feedback, coordination/organization, usability, assistance, workload, and flexibility” (p.105). The literature review highlighted a scarcity of research measuring the quality of online education from the point of view of the students. Little is known about the quality of programs offered through the internet. Faculty, administrators, and policymakers need to know how their “customers” view the quality of online education programs based on their personal learning experiences. The following factors affecting the quality of online learning have been considered and discussed in the current study.
Moore and Kearsley (2005) argued that university support means the availability of guidance and counseling services for students in the university. These include orientations to online learning, administrative assistance, and social interaction with participants. The support from the university can be classified into institutional support and course support (Thorpe, 2002; Lee et al., 2011). Institutional support is wherein the students need support regarding admission, registration, scholarship, research, and student life issues. Selim (2007) has added library services, help desk, computer labs, and facilities to the institutional support. On the other hand, in the course support, students look for clarification of course materials, assignments, activities, and assessments for the specific course.
Support provided to the students during the learning process is crucial for enhancing their learning experience (Chang et al., 2008). During the COVID-19 pandemic, most universities started to offer their coursework online. Most chief academic officers of universities (58%) perceived that online learning was critical for continued learning activities and considered it a part of the overall organizational strategy (Ward et al., 2010). Online learning is impacted by instructor behaviors and characteristics as well as the techniques and media used for the delivery of online instruction. Improving the techniques utilized and providing a reliable source of education delivery is the university’s core responsibility. Equipping the faculty members with the required knowledge, skills, and abilities contributed towards the delivery of quality education.
The support provided to the students enables them to remain motivated to learn, especially during difficult times. Provision of student support is essential to help students achieve their learning goals and objectives (Curley and Strage, 1996). Universities must provide students with a supportive learning environment (Moisey and Hughes, 2008). Similarly, the support provided to the students determines their success in an online learning environment (Rovai and Downey, 2010). Appropriate support strategies to meet students’ needs and learning styles are likely to enhance students’ learning experiences.
Woo and Reeves (2007) argued that universities must adopt the criteria necessary for learning that is: “1) engage learners in authentic learning tasks; 2) create opportunities for meaningful collaboration among the instructor, experts, and other students; 3) engage the students themselves in defining, implementing, and negotiating perspectives relative to these tasks; 4) use collaboration, debate, and analysis to refine and complete the learning tasks; and 5) assure that students have access to the instructor, resources, and one another in order to clear points of confusion and expand concepts. Such a meaningful interaction process is required for enhanced learning” (Woo and Reeves, 2007, p. 20). Based on the above discussion, we propose the following hypothesis:
H1: The university support will significantly influence the quality of online learning.
Faculty members play an important role in shaping the quality of e-learning (Abbasi et al., 2020). They are the driving force behind the delivery of quality education. They are the motivators, guides, and mentors (Campbell and Campbell, 1997) who lead the students through all situations and help them resolve their problems and concerns. They act as role models and inspire students to see life from different angles. They are also instrumental in reducing academic dishonesty (Chirikov et al., 2020) that fosters students’ academic performance. The faculty members encourage students to engage in educational activities and strive for their best performance. In the case of online learning, they help resolve communication issues, provide detailed explanations to avoid confusion, record their lectures and provide extra materials to the students to improve their learning (Mishra et al., 2020). During the COVID 19 pandemic, the economic downturn and job security concerns have further pushed the faculty members to strive to put in their best efforts that have further counted towards the overall improved quality of learning (Mishra et al., 2020). However, technical problems have occasionally hampered their efforts (Ward et al., 2010; Mukhtar et al., 2020). It is noted that some faculty members have been reluctant to offer online courses because of significant concerns relative to the impact of such formats on the quality of instruction, learning, and participant interaction (Ward et al., 2010). It has been noted that the faculty play a vital role in the attrition of the online courses (Pittenger and Doering, 2010), especially when the faculty members viewed online learning as a negative thing (Shieh, 2009). In the United States, despite the proliferation of online courses, various faculty members were reluctant to teach courses over the internet. According to Allen and Seaman (2006), the lack of acceptance of online instruction by instructors is a significant barrier to the large-scale implementation of online courses.
Even the instructors who were comfortable using technology in instruction were found to be concerned about online formats. Some concerns highlighted by them were the reduced human interaction, technology malfunctions, variable technology proficiencies of students, and increased faculty workload (Beard and Harper, 2002). The necessary preparations needed for online teaching and the development of clear, specific, observable, and measurable learning objectives to change learner behavior in the online environment (Pratiwi, 2020) required a lot of time and effort from instructors and increased their workload tremendously. Consequently, the instructors had less time for the actual delivery of instruction.
The quality of learning is directly related to the expertise and involvement of the faculty members. Onwuegbuzie et al. (2007) found that college students believed that their teachers were effective when they were responsive, enthusiastic, student-centered, professional, and expert. It is possible that instructors would be unable to maintain all these criteria while using online modes and thus impede the student’s learning. The educational experiences and expectations of students particularly have a foundation on the teacher-student relationship. The stronger the relationship, the better the result. The student’s attitudes and behaviors are critical to learning and are generally shaped by the social exchanges between the students and their teachers. Lao and Gonzales (2005) conducted a study involving graduate students and found that for online learning to be effective, the courses offered needed well-developed online learning communities, professors who were available for the students, and students who had the right equipment and technology. The above mentioned arguments support the hypothesis that the faculty members who provide support to the students and are available to motivate them and guide them through problems are likely to have better online learning outcomes. Hence, we propose:
H2: Instructor’s support will significantly influence the quality of online learning.
Motivational factors in the study refer to the factors that motivate the students for a positive evaluation of an online learning environment. According to Lumsden (1994), they provided the learner with the value, relevance, and benefits of academic tasks. Motivation plays an imperative role in the whole process of learning. Learners are motivated to improve their ability when the learning is linked with incentives, such as grades, etc., (Lim and Kim, 2003). Aviv (2004) suggested that the strongest motivator for pursuing an online education was the learner’s motivation and life situation. Similarly, self-regulation, collaborative learning opportunities, goal achievement (Paechter et al., 2010), self-efficacy, time management, and learner autonomy (Holder, 2007) have been identified as important motivational factors for online learning experiences of individuals.
After studying the online program of a virtual university of Pakistan, Khan (2021) opined that the ease of access and no travel requirements were the biggest motivators for online students. Kikuchi (2006) identified that the e-learning experiences of Japanese MBA students were motivated by interdependence, flexibility, and sharing the rewards with their families. Similarly, Selim (2007) has also suggested that learning motivation was incremental in the positive adoption of e-learning environments among learners. This cause-and-effect relationship between motivation and engagement in online learning environments has been recognized by earlier literature (Cull et al., 2010; Baturay and Yükseltürk, 2015). Based on the above discussion following hypothesis is proposed:
H3: Motivational factors significantly influence the quality of online learning.
Several other factors could influence the relationship between the role of faculty and the quality of online learning, university support and the quality of online learning, and motivational factors and the quality of online learning. These are situational factors and include factors like hands-on/practical training, student-student interaction, interpersonal and communication skills, student-teacher interaction, boredom/difficulty in concentrating, sense of time/irregular routine, feeling overburdened with academic tasks and responsibilities, etc.
If the individuals are not provided hands-on training, they are likely to commit errors, waste time as well as their energies (Eltaybani et al., 2020). The online learning experience is disrupted when there is no or little support available to the students for learning the technical aspects of how to use the available technology. They are likely to have conflicts with the technology that may result in the development of stress and thus diminish the quality of online learning (Jung et al., 2012). Similarly, a lack of hands-on training shatters one’s confidence and restricts one’s forward mobility. In the online scenario, the faculty members deliver the material by either speaking or recording their lectures, thus limiting opportunities for hands-on training for the students. This lack of hands-on training could have negative consequences for the quality of online learning.
The student-to-student interaction occurs in the natural classroom environment when the students listen to each other’s comments, ask each other questions, and build rapport through frequent contact (Johnson, 1981). When there is little or no interaction between students, they are less likely to exchange resources and resolve their mutual problems. Daunted by the faculty member’s presence, the students could become hesitant to ask questions or request resources from the teacher. The student-student interaction fills this gap and allows them to enjoy a better level of learning by better resource sharing (Wanders et al., 2020). However, in the online learning environment, the student- to- student interactions are limited. The LMS discussion boards and breakout room may provide some opportunity for this type of interaction but these facilities are very limited in developing countries like Pakistan, where the majority of universities don’t have their own LMS.
The presence of interpersonal communication skills is another situational factor that is crucial for effective online learning. These skills encompass one’s capability to interact with other students and teachers. The students with poor communication skills are likely to not understand instructions, the correct meanings of the words or even convey their meaning in an effective manner (Lolli, 2013). Based on the above discussion, it is proposed that situational factors negatively influence the positive relationship between university support, instructor support, motivational factors, and the quality of online learning.
H4a: Situational factors will moderate the positive relationship of university support and the quality of online learning.
H4b: Situational factors will moderate the positive relationship of instructor support and the quality of online learning.
H4c: Situational factors will moderate the positive relationship of motivational factors and the quality of online learning.
The proposed research framework is presented in Figure 1.
The quantitative survey methodology was employed for data collection. The intended participants of the study were university students (undergraduate, graduate, and research students) being instructed via online modalities of teaching and learning in Pakistan during the COVID-19 epidemic. The sampling process was carried out in two steps. In the first phase, the researchers identified 157 institutions offering online education in Pakistan using data from the Higher Education Commission (HEC), Pakistan. They then used random sampling to identify 45 universities offering multiple degree programs in technologies, sciences (pure and social), business, and arts and humanities. In the second phase, convenience sampling was utilized, due to the non-availability of the complete students’ lists, and requested the respective departments in the selected 45 universities to collect the data from the students.
Due to the absence of a comprehensive list of the student population, the convenient sampling approach was chosen for sampling. An online survey was created using QuestionPro and shared with students via social media groups including WhatsApp and Facebook groups. In addition, several universities faculties groups and department heads were approached and requested to disseminate the URL of the online Appendix Questionnaire in their student study circles for timely and maximum data collection. These efforts yielded responses from 707 respondents, 441 of whom were male and 266 were female students.
To assess the proposed model, we have developed scales for each construct. Both exploratory and confirmatory factor analysis tests were conducted while they were being developed to check the reliability and validity of these scales. For measuring the university support, five items were developed. The sample items were: “Guidance/tutorials on the access and use of learning management systems or other modes of online education.” and “Availability of learning material and library resources for course completion.” The scale presented an acceptable alpha value of 0.89. For the measurement of the instructor’s support, ten items were developed. The sample items were: “Availability for consultation and guidance after class hours (WhatsApp, Messages, E-mails, etc.)” and “In-time delivery of course content.” The scale presented an acceptable alpha value of 0.91.
Similarly, for measuring the motivational factors of students, ten items were developed. The sample items were: “My ability to practice self-discipline has increased” and “I was able to focus on additional things (part-time job, hobbies, etc.)”. The scale presented an acceptable alpha value of 0.80. For the situational factors, a scale of 10 further items was developed. The sample items were: “Lack of sense of time/irregular routine” and “Disruption in sleeping pattern.” The scale for situational factors also presented an acceptable alpha value of 0.91. Lastly, the quality of online learning was measured with the help of 4 questions, the questions were adapted from Cobb (2009). This scale also presented an acceptable Cronbach alpha value of 0.84.
A two-step approach (Gerbing and Hamilton, 1996) was employed for scale validations while the scale for the current investigation was being developed. First, Exploratory Factor Analysis (EFA) using SPSS was performed. A principal component method with varimax rotation was also used. All items were loaded in a single analysis. The Kaiser-Meyer-Olkin measure of sampling adequacy value was greater than 0.6, and Bartlett’s test of sphericity value was significant, indicating that the factor analysis was appropriate for the data. Results of EFA identified that all items were appropriately loaded in their respective constructs. Results of the exploratory factor analysis are presented in Table 1.
As the second step for scale validation, Confirmatory factor analysis (CFA), also known as the measurement model, was conducted using AMOS 17. Based on the results, there was no issue of cross-loading, and no items were removed. All observed variables were retained at this stage as all were successfully loaded into their respective latent constructs. The results of CFA provided acceptable model fit indices and are presented in Table 2.
The reliability of scales used to measure latent constructs was assessed with the help of Cronbach’s alpha and composite reliability values. The results identified that values of both reliability measures were greater than the recommended cut-off value of 0.60 (Nunnally and Bernstein, 1994). Similarly, validity was assessed with the help of convergent validity and average variance extracted (AVE) values. For convergent validity, all observed variables were successfully loaded (having regression weights greater than 0.60) into their respective latent construct, and the AVE of all variables was greater than the proposed cut-off value of 0.5. Results of the reliability and validity analyses are presented in Table 2.
Finally, the discriminant validity was assessed with the help of the criterion given by Fornell and Larcker (1981). According to this criterion, the square of correlation of each construct present in the model should be less than the AVE of all variables. As the AVE of all variables was greater than the shared variance of each variable present in the model, it was concluded that there was no issue of discriminant validity. Results of correlation and discriminant validity are presented in Table 3.
We have used a quantitative approach for analyzing the proposed model with the help of primary data collected through the Appendix Questionnaires. A final sample of 707 students from different universities of Pakistan was used for data analysis. A stepwise linear regression and PROCESS Macro by Hayes (2013) Model no. 1 was used for data analysis. Out of the 707 respondents, 62% were male, and 83.3 % were from government universities. About 62 % were enrolled in undergraduate programs, 28% in graduate programs, and 10% were research students. Respondents were from a variety of departments, with the highest percentage from the social sciences (24.5%).
We have used a One-way ANOVA test for the identification of any control variables from the demographic variables. The university sector was significant for the US (f-value:12.03; p-value: 0.001), IS (f-value:5.38; p-value: 0.02), QOLE (f-value:4.40; p-value: 0.036). Level of the study was significant for US (f-value:8.38; p-value: 0.00), IS (f-value:11.25; p-value: 0.00), QOL (f-value:9.06; p-value: 0.00), SM (f-value:9.20; p-value: 0.00). Gender was significantly associated with SF (f-value:4.35; p-value: 0.04), and the department was not significant for any variable present in the model. Based on these results, we have controlled the University sector, level of study, and gender variables for further analysis.
To test the univariate normality of data we have used skewness and kurtosis values for each construct. The skewness and kurtosis values of all variables lie between +/− 3. Hence indicating that the collected data is normally distributed. The skewness and kurtosis values are given in Table 4.
To test the linearity of the variables we have used the significance value of linearity and deviation from linearity. The results indicated that the linear relationship between the independent variables and the dependent variable was significant while the deviation from linearity was insignificant. Results of the linearity test are presented in Table 5.
The first set of proposed hypotheses was analyzed using the stepwise linear regression in SPSS. In the first step of the stepwise linear regression, gender, the university sector, and level of study were included as control variables. In the second step of the stepwise linear regression, university support, instructor support, and student motivation were entered. The results identified that university support, instructor support, and student motivation had a significant positive impact on the quality of online education in the presence of control variables. For every one unit increase in the US, IS, and SM the quality of online learning increased by about 10, 12, and 59%, respectively. The results of the stepwise linear regression analysis are presented in Table 6.
We have used PROCESS Macro (extension in SPSS) by Hayes (2013) to test the proposed moderation hypotheses for situational factors. PROCESS Macro by Hayes (2013) was preferred over simple regression analysis using interaction terms and structural equation modeling because of its robustness. PROCESS Macro uses a bootstrapping approach with biased corrected 95% confidence intervals and calculates the Johnson-Neyman outputs for the interaction term. The variables that define product terms were first mean-centered. Conditioning values at mean and +/−1SD and Johnson-Neyman outputs for the interaction graph were also calculated. We have used separate PROCESS Model No1 for university support, instructor support, and students’ motivation while taking situational factors as moderators. We have used Model No 1 with 5,000 bootstraps sampling and 95% biased corrected confidence intervals. The results of PROCESS Model 1 are presented in Table 7.
The results highlighted that the interaction terms for IS and SM were significant, and that there was no zero in the lower and upper bound of 95% confidence interval. However, the interaction term for the US was insignificant. We plotted interaction graphs for low and high (Mean+/− SD) values of SF. The interaction graph of the IS and QOL relationship (Shown in Figure 2) suggests that the interaction was significant for low levels of SF and insignificant for the high levels of SF. The slope test shows that the presence of SF suppressed the positive relationship between IS and QOLL.
Similarly, the interaction graph of the SM and QOLL relationship (Shown in Figure 3) suggests that interaction was significant at low levels of situational factors and insignificant at high levels of situational factors. The slope test shows that the presence of situational factors suppressed the positive impact of SM on QOLL.
This investigation has identified factors that help ensure the quality of online learning. The results of the study have highlighted that the role of the faculty, university support, and motivational factors positively counted towards the quality of students’ online learning experiences. Moreover, it was also found that the situational factors significantly moderated the relationship between the faculty support, motivational factors and the quality of the online learning relationship. Our results support H1, H2, and H3 postulated in this study. These results are in line with those of earlier studies that have reported a positive relationship between the instructors’ support (Ward et al., 2010; Abbasi et al., 2020; Chirikov et al., 2020; Mishra et al., 2020), university support (Selim, 2007; Lee et al., 2011; Daumiller et al., 2021; Ibrahim et al., 2021), motivational factors (Koca, 2016; Aristika and Juandi, 2021; Chen et al., 2021; Walker and Koralesky, 2021) and the quality of the online learning.
The first important finding of the present study is regarding the positive impact of university support on the quality of online learning, which is consistent with the findings of earlier studies (Selim, 2007; Lee et al., 2011; Daumiller et al., 2021; Ibrahim et al., 2021). The universities generally provide guidance and tutorials to the students that guide them on how to use learning management systems, thus helping them to learn easily (Ibrahim et al., 2021). Our results have identified the importance of support from the university. The universities guide students about using different modes of learning given in the learning management system, such as using the available materials, consulting the recorded lectures, submitting the assignments and examination papers, looking at one’s results, etc., Moreover, the universities provide support in dealing with any technical issues, time management problems and challenges related to computer knowledge, and so forth (Azzi et al., 2021).
The second important finding of the current investigation is the importance of instructors’ support in the online learning environment. Our results generalize the findings of earlier studies, stating that a positive relationship existed between the faculty contributions towards students and their quality of learning (Ward et al., 2010; Abbasi et al., 2020; Chirikov et al., 2020; Mishra et al., 2020). The faculty members’ willingness to cooperate with students and resolve their technology problems adds to the student’s quality of online learning (Abbasi et al., 2020). Moreover, the teachers provide students with the opportunities to ask questions, provide time flexibility in assignments submissions, and provide timely feedback on their assessments (Frymier and Houser, 2000; Walker and Graham, 2021). This contributes to the quality of online learning, which may not be possible in face-to-face teaching due to faculty members’ workload, time commitments, and other administrative duties. At the same time, the convenient exam schedules developed in consultation with students also add to their confidence and motivate them to do their best in exams (Aristika and Juandi, 2021).
The third finding highlights the positive relationship between the motivational factors and the quality of online learning. Motivational factors also add to the quality of the online learning experiences (Koca, 2016; Aristika and Juandi, 2021; Chen et al., 2021; Walker and Koralesky, 2021). While being engaged in online education, one saves on the time to travel and the traveling costs, both of which can be then used for study purposes. Many students have developed their computer skills, learned the effective use of the internet and smartphones for study purposes. This has added to their skills set, and they have become comfortable in acquiring education using online technologies.
The moderation results have shown that the negative consequences of situational factors significantly moderated the relationship between the faculty support and the quality of online learning and the motivational factors and the quality of online learning. However, the university support and the quality of online learning relationships were not moderated by situational factors. It is noted that despite the efforts and contributions of the faculty members, the situational factors have affected this relationship. The students felt that a lack of hands-on training and a lack of student-student interaction hampered their online learning (Cho et al., 2009). Moreover, the students with deficient communication and technical skills also faced several problems with online learning. People faced with slow internet and interrupted electricity supply also faced difficulties in learning. At times, the students experienced problems in managing their workload that counted towards their wasting time in managing their problems and further slowed their learning (Chen et al., 2021). The student’s sense of being out of the place of learning had a negative impact on their psychological states and hampered their online learning. At times the technological interruptions restricted the students from grasping new concepts and prevented them from attaining their learning objectives effectively, thus further adding to their online learning difficulties. Furthermore, many respondents have reported feeling overwhelmed from work overload and the problems they had to manage resulting in disrupted sleep patterns leading to erratic learning patterns (Daumiller et al., 2021). Similarly, the students felt all benefits of online learning such as their ability to save their transportation costs, manage their learning, and maintain self-discipline were influenced by situational factors and thus resulted in non-uniform online learning experiences (Chen et al., 2021). A lack of student-student and student-teacher relationships restricted them from clearing their concepts by collaborating freely with others and greatly disadvantaged their learning (Borup et al., 2019; Aliyyah et al., 2020). Lastly, the results showed that the university support was very significant for the students’ online learning experiences as this relationship was not affected by the negative consequences of situational factors.
The results of the study highlight the fact that higher education institutions, both federal and provincial, in Pakistan, must address the critical aspects of online teaching and learning found in our study. There is a need to implement innovative changes in the online education system and achieve versatility and sustainability over time. The results highlighted that the support from the university and the course instructors as well as the various motivational factors are essential for ensuring the quality of online learning. Furthermore, there is a need to address the negative consequences of situational factors. The education policymakers must consider these factors while developing an online learning policy for students. Moreover, the negative consequences of situational factors are of high importance, especially in a country like Pakistan with limited ICT resources and should be addressed and minimized.
The education ministries and committees at the federal and provincial levels can look into training the instructors so that they can provide the required support to their students. Similarly, the universities should also use learning management systems (LMS) for the timely delivery of course material and for providing secure online discussion platforms. Similarly, collaboration among all stakeholders, i.e., parents, students, and university management is key to improving the quality of online education. The faculty with deficient skills should be trained for better online course delivery. Pre-developed modules for course delivery may help teachers and students understand the material before conducting online classes. Lastly, any reservations that the instructors and learners may still have despite the many innovative technological and content-related features in online education should be addressed and resolved.
Like all investigations, this study also has some limitations to report. Firstly, we used stepwise linear regression and PROCESS Model 1 for hypothesis testing. It may raise the issue of causality, so we recommend that future studies use qualitative and experimental settings to get more comprehensive insights. Secondly, we have studied antecedents of the quality of online learning. However, future studies need to go beyond it. There should be studies conducted to investigate the consequences of the quality of online learning on students’ satisfaction, their academic performance/scores, job-seeking opportunities/difficulties, etc. Thirdly, for the validation of the model, the same framework can be tested in other settings like primary education vs. higher education. Lastly, as the convenience sampling technique was used for data collection, the selection bias may raise issues. Caution is required for the generalizability of the findings as the sample may not have been fully representative. However, despite these limitations, this study still provides additional theoretical and practical support for a deeper understanding of how the quality of online learning is affected by the many factors considered in this study. The future studies may consider direct impact of additional factors like socioeconomic status, family dynamics (single vs. joint family), space availability, parental upbringing, parental education and their moderating and mediating implications for quality of online learning. Also, considering demographic factors such as age and location (urban/rural) may also provide insightful findings.
This research aimed to investigate the impact of university support, instructor’s support and motivational factors on the quality of online learning and the moderating role of situational factors on all proposed hypotheses. Hypotheses H1, H2 and H3 were accepted. Similarly, moderating hypotheses H4b, H4c were also accepted while H4a (moderating role of IS on the US and QOL relationship) was rejected.
Moving from face-to-face classes to online education requires the cooperation of all of the stakeholders. The current study finds that the contributions of all stakeholders, such as the faculty members, educational institutions, and the students themselves have been vital for ensuring the quality of online learning during the COVID-19 pandemic in Pakistan. Online education is at its infancy stage in Pakistan. Given the difficulties inherent to this stage, it is necessary to adopt the technology innovations that can help in the shift from the traditional to the online mode of education during this pandemic.
The raw data supporting the conclusions of this article will be made available by the authors, without undue reservation.
FS developed the overall manuscript and performed the data analysis. WA worked on the literature review and performed proofreading. MM worked on the literature review and discussion section for the manuscript. SR assisted in the data collection and data analysis of this manuscript. All authors contributed to the article and approved the submitted version.
The authors declare that the research was conducted in the absence of any commercial or financial relationships that could be construed as a potential conflict of interest.
All claims expressed in this article are solely those of the authors and do not necessarily represent those of their affiliated organizations, or those of the publisher, the editors and the reviewers. Any product that may be evaluated in this article, or claim that may be made by its manufacturer, is not guaranteed or endorsed by the publisher.
The researchers want to acknowledge Prince Sultan University for providing APC for this manuscript.
Abbasi, S., Ayoob, T., Malik, A., and Memon, S. I. (2020). Perceptions of students regarding E-learning during Covid-19 at a private medical college. Pak. J. Med. Sci. 36:S57. doi: 10.12669/pjms.36.COVID19-S4.2766
Aliyyah, R. R., Rachmadtullah, R., Samsudin, A., Syaodih, E., Nurtanto, M., and Tambunan, A. R. S. (2020). The perceptions of primary school teachers of online learning during the COVID-19 pandemic period: a case study in Indonesia. J. Ethn. Cult. Stud. 7, 90–109. doi: 10.29333/ejecs/388
Allen, I. E., and Seaman, J. (2006). Growing by Degrees: Online Education in the United States, 2005. Wellesley, MA: Sloan Consortium (NJ1).
Andel, S. A., de Vreede, T., Spector, P. E., Padmanabhan, B., Singh, V. K., and De Vreede, G. J. (2020). Do social features help in video-centric online learning platforms? A social presence perspective. Comput. Hum. Behav. 113:106505. doi: 10.1016/j.chb.2020.106505
Aristika, A., and Juandi, D. (2021). The effectiveness of hybrid learning in improving of teacher-student relationship in terms of learning motivation. Emerg. Sci. J. 5, 443–456. doi: 10.28991/esj-2021-01288
Azzi, D. V., Melo, J., Neto, A. D. A. C., Castelo, P. M., Andrade, E. F., and Pereira, L. J. (2021). Quality of life, physical activity and burnout syndrome during online learning period in Brazilian university students during the COVID-19 pandemic: a cluster analysis. Psychol. Health Med. 27, 466–480. doi: 10.1080/13548506.2021.1944656
Baturay, M. H., and Yükseltürk, E. (2015). The role of online education preferences on student’s achievement. Turkish Online J. Dist. Educ. 16, 3–12.
Beard, L. A., and Harper, C. (2002). Student perceptions of online versus on campus instruction. Education 122, 658–663.
Borup, J., Chambers, C. B., and Stimson, R. (2019). K-12 student perceptions of online teacher and on-site facilitator support in supplemental online courses. Online Learn. 23, 253–280.
Campbell, T. A., and Campbell, D. E. (1997). Faculty/student mentor program: effects on academic performance and retention. Res. High. Educ. 38, 727–742.
Chang, K. E., Chen, Y. L., Lin, H. Y., and Sung, Y. T. (2008). Effects of learning support in simulation-based physics learning. Comput. Educ. 51, 1486–1498. doi: 10.1016/j.compedu.2008.01.007
Chang, T. Y., Hong, G., Paganelli, C., Phantumvanit, P., Chang, W. J., Shieh, Y. S., et al. (2020). Innovation of dental education during COVID-19 pandemic. J. Dent. Sci. 16, 15–20. doi: 10.1016/j.jds.2020.07.011
Chen, C., Landa, S., Padilla, A., and Yur-Austin, J. (2021). Learners’ experience and needs in online environments: adopting agility in teaching. J. Res. Innov. Teach. Learn. 14, 18–31. doi: 10.1108/JRIT-11-2020-0073
Chirikov, I., Shmeleva, E., and Loyalka, P. (2020). The role of faculty in reducing academic dishonesty among engineering students. Stud. High. Educ. 45, 2464–2480. doi: 10.1080/03075079.2019.1616169
Cho, V., Cheng, T. C. E., and Hung, H. (2009). Continued usage of technology versus situational factors: an empirical analysis. J. Eng. Technol. Manag. 26, 264–284. doi: 10.1016/j.jengtecman.2009.10.003
Cobb, S. C. (2009). Social presence and online learning: a current view from a research perspective. J. Interact. Online Learn. 8, 241–254.
Cull, S., Reed, D., and Kirk, K. (2010). “Student motivation and engagement in online courses,” in Authored as part of the 2010 workshop, Teaching Geoscience Online-A Workshop for Digital Faculty, 28–29. Available online at: http://serc.carleton.edu/NAGTWorkshops/online/motivation.html
Curley, R. G., and Strage, A. A. (1996). Instructional support and demands: helping teachers help students meet increasing academic standards. Education 117:128. doi: 10.4324/9780203428023-13
Daumiller, M., Rinas, R., Hein, J., Janke, S., Dickhäuser, O., and Dresel, M. (2021). Shifting from face-to-face to online teaching during COVID-19: the role of university faculty achievement goals for attitudes towards this sudden change, and their relevance for burnout/engagement and student evaluations of teaching quality. Comput. Hum. Behav. 118:106677. doi: 10.1016/j.chb.2020.106677
Djidu, H., Mashuri, S., Nasruddin, N., Sejati, A. E., Rasmuin, R., and La Arua, A. (2021). Online learning in the post-Covid-19 pandemic era: is our higher education ready for it? J. Penelitian Pengkajian Ilmu Pendidikan 5, 139–151. doi: 10.36312/esaintika.v5i2.479
Dong, C., Cao, S., and Li, H. (2020). Young children’s online learning during COVID-19 pandemic: Chinese parents’ beliefs and attitudes. Child. Youth Serv. Rev. 118:105440. doi: 10.1016/j.childyouth.2020.105440
Eltaybani, S., Abdelwareth, M., Abou-Zeid, N. A., and Ahmed, N. (2020). Recommendations to prevent nursing errors: content analysis of semi-structured interviews with intensive care unit nurses in a developing country. J. Nurs. Manag. 28, 690–698. doi: 10.1111/jonm.12985
Fornell, C., and Larcker, D. F. (1981). Evaluating structural equation models with unobservable variables and measurement error. J. Mark. Res. 18, 39–50. doi: 10.2307/3151312
Franklin, T., Turner, S., Kariuki, M., and Duran, M. (2001). Mentoring overcomes barriers to technology integration. J. Comput. Teach. Educ. 18, 26–31.
Frymier, A. B., and Houser, M. L. (2000). The teacher-student relationship as an interpersonal relationship. Commun. Educ. 49, 207–219. doi: 10.1080/03634520009379209
Gerbing, D. W., and Hamilton, J. G. (1996). Viability of exploratory factor analysis as a precursor to confirmatory factor analysis. Struct. Equ. Model. 3, 62–72. doi: 10.1080/10705519609540030
Hasibuan, N. (2016). Development of islamic education with educational technology implications. FITRAH J. Study Islamic Sci. 1:189. doi: 10.24952/fitrah.v1i2.313
Holder, B. (2007). An investigation of hope, academics, environment, and motivation as predictors of persistence in higher education online programs. Intern. High. Educ. 10, 245–260. doi: 10.1016/j.iheduc.2007.08.002
Hwang, G. J., Wang, S. Y., and Lai, C. L. (2021). Effects of a social regulation-based online learning framework on students’ learning achievements and behaviors in mathematics. Comput. Educ. 160:104031. doi: 10.1016/j.compedu.2020.104031
Ibrahim, N. K., Al Raddadi, R., AlDarmasi, M., Al Ghamdi, A., Gaddoury, M., AlBar, H. M., et al. (2021). Medical students’ acceptance and perceptions of e-learning during the Covid-19 closure time in King Abdulaziz University, Jeddah. J. Infect. Public Health 14, 17–23. doi: 10.1016/j.jiph.2020.11.007
Johnson, D. W. (1981). Student-student interaction: the neglected variable in education. Educ. Res. 10, 5–10. doi: 10.1016/j.adolescence.2021.01.005
Jung, I., Kudo, M., and Choi, S. K. (2012). Stress in Japanese learners engaged in online collaborative learning in English. Br. J. Educ. Technol. 43, 1016–1029. doi: 10.1111/j.1467-8535.2011.01271.x
Kearsley, G. (2000). Online Education: Learning and Teaching in Cyberspace. Wadsworth, ON: Wadsworth Publishing Company.
Khachfe, H. H., Chahrour, M., Sammouri, J., Salhab, H. A., Makki, B. E., and Fares, M. Y. (2020). An epidemiological study on COVID-19: a rapidly spreading disease. Cureus 12:e7313. doi: 10.7759/cureus.7313
Khalil, R., Mansour, A. E., Fadda, W. A., Almisnid, K., Aldamegh, M., Al-Nafeesah, A., et al. (2020). The sudden transition to synchronized online learning during the COVID-19 pandemic in Saudi Arabia: a qualitative study exploring medical students’ perspectives. BMC Med. Educ. 20:285. doi: 10.1186/s12909-020-02208-z
Khan, I. M. (2021). An Analysis of the Motivational Factors in Online Learning. Ph.D. thesis. Tempe, AZ: University of Phoenix.
Kikuchi, H. (2006). Motivational factors affecting online learning by Japanese MBA students. Australas. J. Educ. Technol. 22, 389–415.
Laksana, D. N. L. (2021). Implementation of online learning in the pandemic covid-19: student perception in areas with minimum internet access. J. Educ. Technol. 4, 502–509. doi: 10.23887/jet.v4i4.29314
Lao, T., and Gonzales, C. (2005). Understanding online learning through a qualitative description of profesors and students’ experiences. J. Technol. Teach. Educ. 13, 459–474.
Lee, S. J., Srinivasan, S., Trail, T., Lewis, D., and Lopez, S. (2011). Examining the relationship among student perception of support, course satisfaction, and learning outcomes in online learning. Intern. High. Educ. 14, 158–163. doi: 10.1016/j.iheduc.2011.04.001
Lim, D. H., and Kim, H. J. (2003). Motivation and learner characteristics affecting online learning and learning application. J. Educ. Technol. Syst. 31, 423–439. doi: 10.1186/s12913-016-1423-5
Lolli, J. C. (2013). Interpersonal communication skills and the young hospitality leader: are they prepared? Int. J. Hosp. Manag. 32, 295–298. doi: 10.1016/j.ijhm.2012.02.010
Mishra, L., Gupta, T., and Shree, A. (2020). Online teaching-learning in higher education during lockdown period of COVID-19 pandemic. Int. J. Educ. Res. Open 1:100012. doi: 10.1016/j.ijedro.2020.100012
Moisey, S. D., and Hughes, J. A. (2008). “Supporting the online learner,” in The Theory and Practice of Online Learning, ed. T. Anderson (Edmonton, AB: AU Press), 419–439.
Mokter, H. C. (2020). The effect of the Covid-19 on sharing economy activities. J. Clean. Prod. 280, 124782. doi: 10.1016/j.jclepro.2020.124782
Moore, M. G., and Kearsley, G. (2005). Distance Education: A Systems View. Belmont, CA: Thomson Wadsworth.
Mukhtar, K., Javed, K., Arooj, M., and Sethi, A. (2020). Advantages, limitations and recommendations for online learning during COVID-19 pandemic era. Pak. J. Med. Sci. 36:S27. doi: 10.12669/pjms.36.COVID19-S4.2785
Mustakim. (2020). The effectiveness of e-learning using online media during the Covid-19 pandemic in mathematics. Al ASMA 2, 1–12. doi: 10.19166/johme.v5i1.3811
Onwuegbuzie, A. J., Witcher, A. E., Collins, K. M., Filer, J. D., Wiedmaier, C. D., and Moore, C. W. (2007). Students’ perceptions of characteristics of effective college teachers: a validity study of a teaching evaluation form using a mixed-methods analysis. Am. Educ. Res. J. 44, 113–160. doi: 10.3102/0002831206298169
Paechter, M., Maier, B., and Macher, D. (2010). Students’ expectations of, and experiences in e-learning: their relation to learning achievements and course satisfaction. Comput. Educ. 54, 222–229. doi: 10.1016/j.compedu.2009.08.005
Panesar, K., Dodson, T., Lynch, J., Bryson-Cahn, C., Chew, L., and Dillon, J. (2020). Evolution of COVID-19 guidelines for university of washington oral and maxillofacial surgery patient care. J. Oral Maxillofac. Surg. 78, 1136–1146. doi: 10.1016/j.joms.2020.04.034
Patricia, A. (2020). College students’ use and acceptance of emergency online learning due to COVID-19. Int. J. Educ. Res. Open 1:100011. doi: 10.1016/j.ijedro.2020.100011
Pittenger, A., and Doering, A. (2010). Influence of motivational design on completion rates in online self-study pharmacy-content courses. Dist. Educ. 31, 275–293. doi: 10.1080/01587919.2010.513953
Pratiwi, E. W. (2020). The impact of Covid-19 on online learning activities at Christian Universities in Indonesia. Educ. Sci. Perspect. 34, 1–8. doi: 10.31334/abiwara.v2i1.1049
Rovai, A. P., and Downey, J. R. (2010). Why some distance education programs fail while others succeed in a global environment. Intern. High. Educ. 13, 141–147. doi: 10.1016/j.iheduc.2009.07.001
Rusli, R., Rahman, A., and Abdullah, H. (2020). Student perception data on online learning using heutagogy approach in the Faculty of Mathematics and Natural Sciences of Universitas Negeri Makassar. Indonesia. Data Brief 29:105152. doi: 10.1016/j.dib.2020.105152
Selim, H. M. (2007). Critical success factors for e-learning acceptance: confirmatory factor models. Comput. Educ. 49, 396–413. doi: 10.1016/j.compedu.2005.09.004
Shehzadi, S., Nisar, Q. A., Hussain, M. S., Basheer, M. F., Hameed, W. U., and Chaudhry, N. I. (2020). The role of digital learning toward students’ satisfaction and university brand image at educational institutes of Pakistan: a post-effect of COVID-19. Asian Educ. Dev. Stud. 10, 276–294. doi: 10.1108/aeds-04-2020-0063
Shieh, D. (2009). These lectures are gone in 60 seconds. Chron. High. Educ. 26, 1–13. doi: 10.1016/j.ijindorg.2013.12.001
Soffer, T., Kahan, T., and Nachmias, R. (2019). Patterns of students’ utilization of flexibility in online academic courses and their relation to course achievement. Int. Rev. Res. Open Distrib. Learn. 20, 202–220. doi: 10.1016/j.nedt.2004.09.006
Suprianto, S., Arhas, S. H., Mahmuddin, M., and Siagian, A. O. (2020). The effectiveness of online learning amid the COVID-19 pandemic. J. Adm. 7, 321–330.
Suryaman, M., Cahyono, Y., Muliansyah, D., Bustani, O., Suryani, P., Fahlevi, M., et al. (2020). COVID-19 pandemic and home online learning system: does it affect the quality of pharmacy school learning. Syst. Rev. Pharm. 11, 524–530.
Thorpe, M. (2002). Rethinking learner support: the challenge of collaborative online learning. Open Learn. 17, 105–119. doi: 10.1080/02680510220146887a
Tj, H. W., and Tanuraharjo, H. H. (2020). The effect of online learning service quality on student satisfaction during COVID19 pandemic In 2020. J. Manajemen Indonesia 20:240. doi: 10.25124/jmi.v20i3.3520
Walker, K. A., and Koralesky, K. E. (2021). Student and instructor perceptions of engagement after the rapid online transition of teaching due to COVID-19. Nat. Sci. Educ. 50:e20038.
Walker, S., and Graham, L. (2021). At risk students and teacher-student relationships: student characteristics, attitudes to school and classroom climate. Int. J. Inclusive Educ. 25, 896–913. doi: 10.1080/13603116.2019.1588925
Wanders, F. H., Dijkstra, A. B., Maslowski, R., and Van der Veen, I. (2020). The effect of teacher-student and student-student relationships on the societal involvement of students. Res. Pap. Educ. 35, 266–286. doi: 10.1080/02671522.2019.1568529
Ward, M. E., Peters, G., and Shelley, K. (2010). Student and faculty perceptions of the quality of online learning experiences. Int. Rev. Res. Open Distrib. Learn. 11, 57–77. doi: 10.19173/irrodl.v11i3.867
Wong, G. L. H., Wong, V. W. S., Thompson, A., Jia, J., Hou, J., Lesmana, C. R. A., et al. (2020). Management of patients with liver derangement during the COVID-19 pandemic: an Asia-Pacific position statement. Lancet Gastroenterol. Hepatol. 5, 776–787. doi: 10.1016/S2468-1253(20)30190-4
Woo, Y., and Reeves, T. C. (2007). Meaningful interaction in web-based learning: a social constructivist interpretation. Intern. High. Educ. 10, 15–25. doi: 10.1016/j.iheduc.2006.10.005
Yang, Y., and Cornelius, L. F. (2004). Students’ Perceptions Towards the Quality of Online Education: A Qualitative Approach. Bloomington, IN: Association for Educational Communications and Technology.
Young, A., and Norgard, C. (2006). Assessing the quality of online courses from the students’ perspective. Intern. High. Educ. 9, 107–115. doi: 10.1016/j.iheduc.2006.03.001
Zhafira, N. H., Ertika, Y., and Chairiyaton. (2020). Student perceptions of online lectures as learning facilities during the Covid-19 quarantine period. J. Bus. Manag. Strategy Stud. 4, 37–45.
University Support
(1). Guidance/tutorials on the access and use of learning management systems or other modes of online education.
(2). Clear guidelines/policies on shifting from traditional to the online mode of education.
(3). Availability of learning material and library resources for course completion.
(4). Technical support to overcome any technical difficulties.
(5). Provision for registering any grievances or complaints.
Scale: 1 = not at all satisfied, 2 = slightly satisfied, 3 = moderately satisfied, 4 = very satisfied, 5 = extremely satisfied.
Instructor’s Support
(1). Opportunities given to ask questions.
(2). Flexibility in following assignment deadlines.
(3). Clear guidelines about assignments and assessments.
(4). Availability for consultation and guidance after class hours (WhatsApp, Messages, E-mails, etc.).
(5). In-time delivery of course content.
(6). Appropriate pace of lectures.
(7). The overall quality of course content.
(8). Follow-up and motivation to improve class progress.
(9). Timely and continuous feedback on class progress.
(10). Convenient exam schedules.
Scale: 1 = Not at all Satisfied, 2 = Slightly Satisfied, 3 = Moderately Satisfied, 4 = Very Satisfied, 5 = Extremely Satisfied.
Motivational Factors
(1). My ability to practice self-responsibility has increased.
(2). My ability to practice self-discipline has increased.
(3). I was able to manage my time effectively (completing assignments, assessments, etc.).
(4). I was able to cut down transportation costs.
(5). My command in using the relevant software and required tools has increased.
(6). I was quite successful in using the relevant software and required tools.
(7). I was able to focus on additional things (part-time job, hobbies, etc.).
(8). My time-management skills have improved.
(9). I was able to maintain motivation and enthusiasm while taking online classes.
(10). I found online learning to be more convenient and flexible.
Scale: 1 = strongly disagree, 2 = disagree, 3 = neutral, 4 = agree, 5 = strongly agree.
Situational Factors
(1). Lack of hands-on/practical training.
(2). Lack of student-student interaction.
(3). Lack of interpersonal and communication skills.
(4). Lack of student-teacher interaction.
(5). Online learning boredom/difficulty in concentrating.
(6). Lack of sense of time/irregular routine.
(7). Feeling overburdened with academic tasks and responsibilities.
(8). Lack of sense of place.
(9). Inability to grasp concepts, learning objectives effectively.
(10). Disruption in sleeping pattern.
Scale: 1 = Not at all Influential, 2 = Slightly Influential, 3 = Somewhat Influential, 4 = Very Influential, 5 = Extremely Influential.
Quality of Online Learning
(1). I was able to learn from the online discussions and online class activities.
(2). I was able to learn from the online course offered by my university.
(3). As a result of my experience with the online courses offered by my university, I would like to participate in other online courses in the future.
(4). The online courses offered by my university were useful learning experience for me.
Scale: 1 = strongly disagree, 2 = disagree, 3 = neutral, 4 = agree, 5 = strongly agree.
Keywords: university support, instructor’s support, motivational factors, situational factors, quality of online learning, developing economy
Citation: Saleem F, AlNasrallah W, Malik MI and Rehman SU (2022) Factors Affecting the Quality of Online Learning During COVID-19: Evidence From a Developing Economy. Front. Educ. 7:847571. doi: 10.3389/feduc.2022.847571
Received: 02 January 2022; Accepted: 30 March 2022;
Published: 28 April 2022.
Edited by:
Bulent Tarman, Turan University, KazakhstanReviewed by:
Teguh Budiharso, State Islamic University (UIN) Raden Mas Said, IndonesiaCopyright © 2022 Saleem, AlNasrallah, Malik and Rehman. This is an open-access article distributed under the terms of the Creative Commons Attribution License (CC BY). The use, distribution or reproduction in other forums is permitted, provided the original author(s) and the copyright owner(s) are credited and that the original publication in this journal is cited, in accordance with accepted academic practice. No use, distribution or reproduction is permitted which does not comply with these terms.
*Correspondence: Farida Saleem, ZmFyaWRhLXNhbGVlbUBob3RtYWlsLmNvbQ==
Disclaimer: All claims expressed in this article are solely those of the authors and do not necessarily represent those of their affiliated organizations, or those of the publisher, the editors and the reviewers. Any product that may be evaluated in this article or claim that may be made by its manufacturer is not guaranteed or endorsed by the publisher.
Research integrity at Frontiers
Learn more about the work of our research integrity team to safeguard the quality of each article we publish.