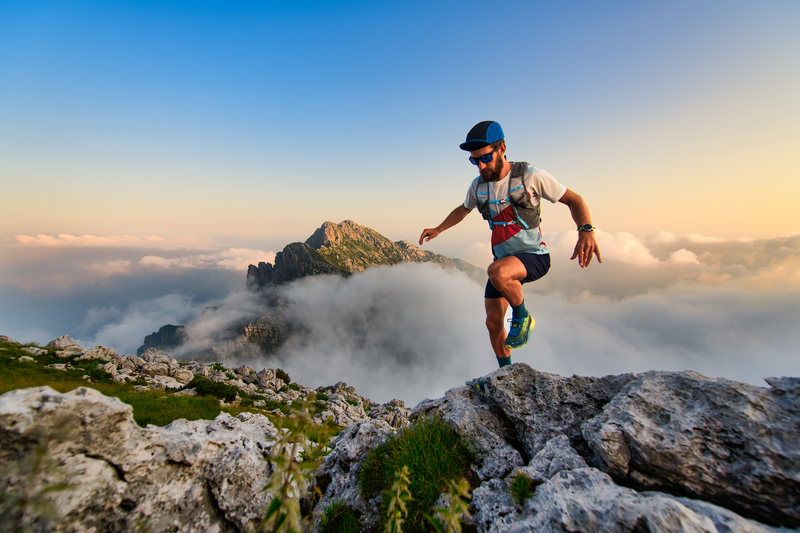
94% of researchers rate our articles as excellent or good
Learn more about the work of our research integrity team to safeguard the quality of each article we publish.
Find out more
ORIGINAL RESEARCH article
Front. Educ. , 19 May 2022
Sec. Digital Learning Innovations
Volume 7 - 2022 | https://doi.org/10.3389/feduc.2022.845647
This article is part of the Research Topic Open Education for Sustainable Development: Contributions from Emerging Technologies and Educational Innovation View all 11 articles
The popularity of educational videos has increased in recent years. In 2018, YouTube announced a $20 million investment to support educational video creators and organized the YouTube EduCon event to bring them together and form a community. The COVID-19 pandemic pushed educational institutions to use resources contained in public repositories, such as YouTube. The objective of this research study was to explore the dynamics of EduTubers to understand the motivations for their interactions. We used social network analysis (SNA) with YouTube data to analyze the dynamics of EduTubers’ recommendation networks. Meaningful insights reveal a relationship between the level of digital engagement and the level of out-degree. Additionally, we confirm that educhannel homophily has a positive effect on the level of reciprocity. The main contribution lies in the use of theoretical concepts (reciprocity and homophily), focusing on the intrinsic motivations of EduTubers to recommend other channels. The practical implications of the results suggest that educhannels that initiate and grow digital engagement are more likely to participate in a recommendation network.
The popularity of educational videos has increased recently. In 2018, YouTube announced a $20 million investment to support educational video creators, now called EduTubers (López, 2019; López et al., 2020; Martínez and Cedillo, 2020). YouTube also organized the annual YouTube EduCon event. In addition to providing training and development for EduTubers, this event aimed to bring them together and form a community (YouTube, 2018). Google (2019) highlights the increasing popularity of YouTube; the platform contains more than one million educational videos, which have more than one billion daily views.
Digital social networks are applications that young people use the most, with videos constituting most of their digital consumption, including videos to support their learning objectives. In Mexico, accessing social media apps is the principal activity of users (Asociación de Internet, 2021). Rangarajan et al. (2019) indicate that the innovative approach offered by social platforms, such as YouTube, has led to the increased use of videos for training. In this case, given the availability of this type of videos on the Internet and the prominence of the technology in everyday life, seeking out this type of complementary resources is becoming increasingly common for students.
The COVID-19 pandemic pushed educational institutions to use resources contained in public repositories, such as YouTube. The use of public educational content by teachers drove the increase in the possibilities in formal and informal settings (Pattier, 2021a). To address these needs, YouTube launched four strategies focused on finding learning resources more easily, including YouTube Learning Destination, which has high-quality educational content for students, and #StudyWithMe, purposed with sharing study experiences (YouTube Official Blog, 2020). Given the evident importance of open educational content and the popularity of YouTube among the student population, the social network dynamics among EduTubers is a topic that has been increasing its presence in academic research.
YouTube channels are looking for strategies to capture consumers and grow in popularity; in this competition dynamic, measuring digital engagement is important. The literature features some studies focused on the influence and digital engagement of YouTube channels. Abdelkader (2021) proposed a model to determine the level of engagement with content published through YouTube channels. Paolillo (2008) analyzed the social structure of YouTube by studying friendship relationships and their correlation with the tags applied to the videos. Tur-Viñes et al. (2018) identified the practices of influencers on YouTube and the presence of brands in their content.
Another line of inquiry has focused on the communities formed on YouTube and the key factors for success in those communities. For example, scholars have studied the recommendations made by YouTube through its algorithms (Abul-Fottouh et al., 2020; Kaiser and Rauchfleisch, 2020), as well as the mutually beneficial behaviors and the effect that the number of subscribers and views can have (Song et al., 2019). Some scholars (Pérez-Escoda and García-Ruiz, 2020; Arroyo et al., 2021; Fortaleza, 2021; Pattier, 2021a,b,c) studied the practices and characteristics that classify educational channels among YouTube’s top channels. Two key factors for our research emerge from the results: (1) the data and statistics of the channel, considering the frequency of video uploads and the average duration of the videos, and (2) the use of the YouTube platform, adding the “Community” section and offering links to other YouTube channels. To our knowledge, prior research included only a limited number of studies on the dynamics of the EduTubers’ interactions and community formation.
Although YouTube is focused on content sharing, it also offers users certain socially oriented functionalities. The “Community” section extends the possibilities for creators and consumers to interact on the same platform. Thus, for the creators (in this context EduTubers), it is an additional source of the traffic to their channel that allows them to strengthen their ties with other EduTubers and create a community. Therefore, EduTubers can use strategies related to reciprocity (a behavioral response of mutual benefit) and homophily (propensity of individuals to connect with similar others) to increase traffic to their channel and their level of digital engagement because engagement in a social network is reflected in the publications and interactions shared by users (Pletikosa Cvijikj and Michahelles, 2013). In sum, content producers on YouTube benefit from social relationships for mutual support, for example, reciprocated recommendations (Paolillo, 2008), which can benefit the channel’s engagement rate. Therefore, exploring mechanisms of social interaction in the context of EduTubers and educhannels is relevant.
The objective of this research is to explore the dynamics of the EduTubers’ recommendation network to understand the nature of the connection between them. We used as theoretical bases (a) digital engagement to understand successful educhannels, (b) social exchange theory (SET) to understand the reciprocity phenomena, and (c) the mechanism of homophily to understand the connection mechanism between similar members of a group. The result of this research expands scientific knowledge as they allow a better understanding of the dynamics generated in the recommendation network of EduTubers and, above all, by implementing a technique that is widely used in the social sciences, such as SNA, to study a phenomenon of life on the digital plane. To do so, we created a recommendation network of 412 channels of EduTubers, gathered their main attributes, analyzed their centrality and reciprocity, and used Multiple regression quadratic assignment procedures (MRQAP) to measure their interrelation.
The main contribution of our results is the use of theoretical concepts (reciprocity and homophily), which focuses on the intrinsic motivations of EduTubers to recommend other channels. Our findings indicate that EduTubers’ channels that have a higher level of digital engagement tend to recommend a higher number of other educhannels. Another detail that is important to note is that each online social network is different in nature and behavior (i.e., Facebook and Twitter). The results of this study suggest that on YouTube, and specifically among EduTubers, recommending other educhannels is a relevant factor that contributes to their digital engagement.
In this section, we present the theoretical bases of the research that will help provide insights to understand the phenomena: digital engagement, SET, and homophily.
The concept of engagement can take on different connotations depending on the context in which it is used. Digital engagement on a social network is reflected in the level of audience involvement based on the characteristics and content shared on the network (Pletikosa Cvijikj and Michahelles, 2013). The concept in social media is also understood as a psychological state of motivation that results in the act of following (Pérez-Escoda et al., 2020). Different perspectives and models of digital engagement can be found in the literature according to the social network studied.
Some scholars have proposed models to measure digital engagement. However, the mechanisms vary from one technological platform to another. Bonsón and Ratkai (2013) consider likes, comments, and shares as elements for measuring popularity, commitment, and virality associated with social media content. Authors such as Abdelkader (2021) point out that the content published, the number of subscribers, the number of uploaded videos, the years of experience, the length of the video, the country, and the category are the factors to determine engagement. In general, the proposals agree that these actions include (1) giving a like and (2) commenting, which are direct manifestations of engagement (Pletikosa Cvijikj and Michahelles, 2013; Sabate et al., 2014). When measuring engagement, considering both content exposure and user participation is important.
In this sense, scholars have proposed mechanisms to measure fans’ digital engagement on YouTube. Xie (2017) developed an engagement index to measure three layers of engagement in YouTube: (1) shallow engagement is calculated by four quantitative variables: view count, like count, dislike count, and comment count; (2) medium engagement is measured by the frequency of three types of interactions: conversations between the community members, comments intended to speak to the others who are watching the video and the vocabulary in the comment indicate an emotional expression; and finally, (3) deep engagement is measured by three variables related to contributing labor and money: audiences contributing subtitles, leaving long comments, and donating money to the channel. Another indicator for calculating engagement in YouTube channels is by considering likes, dislikes, and views (López-Navarrete et al., 2021). Although some of the proposals are recent, an important detail to emphasize is that YouTube has currently changed the interaction options and the number of dislikes is not available to the public. Some services on the web, such as SocialBlade, track user statistics for social media. SocialBlade provides some metrics to understand the growth and trends of accounts on social media; these statistics have also been used in other studies (Pérez-Escoda and García-Ruiz, 2020; Abdelkader, 2021; Fortaleza, 2021). According to SocialBlade (nd), they initially ranked based on the numbers of subscribers and visits, but they quickly realized that these were not very reliable indicators. Now, their algorithm aims to measure the influence of a channel based on several metrics, including the average number of visits.
Social exchange theory (SET) is a family of conceptual models (Cropanzano and Mitchell, 2005) that are based on the assumption that any interaction between individuals is an exchange of tangible or intangible resources (Homans, 1958). The main premise of SET is that people enter and maintain relationships with the expectation that doing so will be rewarding (Homans, 1958; Blau, 1968). Scholars have found that the level of commitment in the relationship is proportional to the number of benefits that will be obtained (Thibaut and Kelley, 1959), that interdependence is crucial to the continuance of such relationship (Emerson, 1962), and that positive economic and social outcomes increase the partners’ trust over time.
Given these patterns of social behaviors, individuals who develop mutual and beneficial exchanges over time engender trust, loyalty, and commitment among the parties (Mitchell et al., 2012). When providing another with a benefit, one must trust that the other will return the benefit in time (Homans, 1958; Blau, 1964). Furthermore, SET predicts that in reaction to positive initiating actions, targets will tend to reply in kind by engaging in reciprocity (Cropanzano and Mitchell, 2005).
Reciprocity is a powerful determinant of human behavior. It is modeled as the behavioral response to an action that is perceived as either kind or unkind, and it is assessed in terms of the consequence of an action and the intentions involved (Eisenberger et al., 2001). Reciprocity has been studied in dyads and groups or networks. When a person does another a favor and receives a benefit in return directly from the same person (dyadic level), it is called direct reciprocity (Axelrod and Hamilton, 1981). When reciprocity involves a group or a network, it is referred to as indirect reciprocity (Nowak, 2006) or generalized exchange (Takahashi, 2000). In the social media arena, scholars have found reciprocity among content providers in social media (Song et al., 2019). Cheng et al. (2008) studied videos as nodes and recommendations to other videos as ties. Moreover, although Torres and Conrad (2015) studied YouTube channels that recommend other channels and analyzed the structure of the network created by such recommendations, they suggest that additional research is needed.
For instance, the ties between content providers have not been well understood, especially for their reciprocity (Song et al., 2019). The scarce literature on ties between providers has studied how ties induce the linked providers to change their content (Zeng and Wei, 2013; Wang et al., 2018) and how one-way ties benefit the initiators (Katona and Sarvary, 2008; Mayzlin and Yoganarasimhan, 2012) and the providers to which they link (Stephen and Toubia, 2010; Jabr and Zheng, 2014). Commonly, researchers rely on SNA measures in their studies to analyze their data.
Social network analysis enables the study of social systems from a structural perspective through the identification of behavioral patterns based on node and tie attributes (Freeman, 1979). In particular, degree centrality (Freeman, 1979; Borgatti, 2005) is a concept that describes how connected a node is with others in the network. It is understood as the number of direct edges a node has, such as the number of incoming recommendations and outgoing recommendations of a YouTube channel.
Given the patterns of reciprocity and how the level of digital engagement is obtained, we propose that YouTube content providers recommend other channels with the aim that the others will reciprocate their action (Homans, 1958; Blau, 1964; Mitchell et al., 2012), which in turn will increase their digital engagement on the network. We state our first research question as follows: Is there a relationship between the centrality in EduTubers’ recommendation network and their digital engagement on YouTube? However, it is interesting as well to explore whom those EduTubers recommend. YouTube content creators are strongly linked to others producing similar content (Paolillo, 2008). Therefore, we find in homophily one possible answer.
Homophily is one of the main patterns underlying human relationships (Lawrence and Shah, 2020). It refers to the propensity of individuals to connect with similar others, that is, with those who have common attributes and values (Lazarsfeld and Merton, 1954); who are like-minded; and who share the same habits, behaviors, and beliefs (McPherson et al., 2001). A variety of lines of reasoning support the homophily principle. One of them is the similarity-attraction hypothesis (Byrne, 1971) which was presented by Heider (1958), who proposed that psychological discomfort that emerges from cognitive or emotional inconsistency can be reduced by homophily. Therefore, individuals tend to select similar others to reduce the potential areas of conflict in the relationship (Sherif, 1958), and there is an a priori notion that they are more likely to be accepted (Easley and Kleinberg, 2010). Consequently, trust and solidarity are expected to be more likely to be built with similar than with dissimilar counterparts (Mollica et al., 2003). Furthermore, ties among similar ones reduce the risks associated with their formation and the cost of maintaining them, and are more permanent and durable (Kossinets and Watts, 2009).
Homophily has been frequently observed in social networks, where people with similar contexts connect with each other naturally and constantly (Ma et al., 2015; Halberstam and Knight, 2016). The effect of homophily has been widely studied in social media data, from textual data to follower lists of accounts (Pan et al., 2019; Khanam et al., 2020). Most of the studies focused on textual posts, hashtags, mentions, or users’ network connections. However, a research gap exists, because studies on YouTube recommendations are scarce (Khanam et al., 2020). As an exception, Kaiser and Rauchfleisch (2020) analyzed the algorithmic homophily on YouTube’s channel recommendations. These authors found that the algorithm fosters the creation of highly homophilous communities. In the same line, Paolillo (2008) found that YouTube content creators are strongly linked to others that produce similar content, also based on the recommendation network. In sum, previous studies in a variety of contexts consistently found that homophilous ties are more likely to act similarly, to reciprocate, and to cooperate (Ma et al., 2015; Wang et al., 2018; Song et al., 2019). Therefore, our second research question is as follows: Is there a relationship between educhannels’ homophily (channel attributes) and the level of reciprocity in EduTubers’ recommendation network?
This research aims to explore the dynamic of EduTubers’ recommendation network to understand the nature of the connections between them. The research follows a design using digital methods and SNA. Digital methods are techniques for the study of social phenomena employing data available on the network (Rogers, 2015). This technique uses digital objects (links, comments, etc.) created on Internet platforms. SNA provides a means to understand the structure and information flows of social networks in both social media and academic media (Carmichael and Archibald, 2019). SNA’s purpose is to examine connections between individuals and groups via the study of the structure of nodes and edges, which represent entities and relationships, respectively. The use of SNA is vast, being the common context of study knowledge sharing networks (Han et al., 2020), interlocking directorates (Wang et al., 2021), and collaboration and advice-seeking networks (Sinnema et al., 2020). Although a recommendation network analyzes the interaction of content creators, it differs somehow from the rest due to the lack of evidence of face-to-face contact and the difficulty of measuring the intensity of such interactions.
In SNA, one of the sampling methods is snowball sampling, in which relationships between participants are iteratively traced to identify new participants in the network (Paolillo, 2008). We used this approach because it has a particular value in network circumstances with unavailable pre-existing lists (Doreian and Woodard, 1992). For this study, as entry points, 37 educhannels owned by the most recommended EduTubers were identified and selected. To avoid bias, the selected educhannels were about different topics, targeted different audiences, and were created in different countries. Entry points were identified through a Google search. For the search, we use the terms “top 10 edutubers” and “top edutubers,” and we found seven web pages with a list of EduTubers1 ,2 ,3 ,4 ,5 ,6 ,7, which meets the exploratory nature of this study.
The relational data were collected based on information gathered from each channel in the section “Channels,” which contains the channel recommendations of the owner. The collected data allowed the creation of an ego network that differs from a full network in the sense that it is created from first-degree connections and the interlinkage among them. The full network requires a full list of actors from the beginning of an SNA study (Stolz and Schlereth, 2021). The ego network data were integrated by using the information of recommended channels in an iterative process to find new educhannels. Notably, for the owners, the “Channels” section is an additional source of traffic to their channel and strengthens their ties with other creators, hence creating a community and increasing their digital engagement. Furthermore, channel recommendations are not personalized on a user level. Thus, every time a channel page is opened even by different individual users, the same set of channels is recommended (Kaiser and Rauchfleisch, 2020). At the end of the third iterative process, 412 educhannels were selected to integrate the recommendation network with 1,303 edges.
The data for the analysis were collected during October 2021. The following attributes were obtained for each channel: year of creation, number of subscribers, number of videos, number of channel views, category of the topic covered in the videos based on OECD’s classification (Organization for Economic Co-operation and Development, 2007), target audience, type of content developed, country, and level of digital engagement. The 412 educhannels considered for this study were created between 2005 (foundation year of YouTube) and 2021. Table 1 shows the attributes and categories used in this study.
The format of 46.6% of the educhannels is as a class and 40.3% is as science communication. Eighteen percent of the educhannels are from Latin America, 23.1% are from Spain, and 37.6% are from non-Spanish-speaking countries. Furthermore, most of the channels are rated B (9.2% B+, 35.2% B, 29.1% B−), and 17.7% are rated C+. Finally, 38.1% of the channels talk about natural science, 16.7% talk about humanities, and 29.1% discuss a variety of subjects.
To build the YouTube Channel Recommendation Network, we directed a graph where the nodes are all channels found in the data collection process and the edges are the creators’ recommendations to other channels. Our unit of analysis is the relationship between pairs of educhannels, meaning that all the variables are dyadic (Borgatti and Cross, 2003).
For the first research question, our dependent variable is the level of digital engagement. As previously mentioned in this document, the metrics commonly used for digital engagement analysis are based on videos. However, in our study, we analyzed channels and their connectivity. Therefore, we could not apply those metrics. As a result, to triangulate the sources of information and strengthen the results, we obtained this metric from SocialBlade, which is a trustable Internet site and used in other studies (Pérez-Escoda and García-Ruiz, 2020; Abdelkader, 2021; Fortaleza, 2021; SocialBlade, nd). It is useful to understand the growth and trends of accounts on social media. The level of digital engagement is given from A+ to D−, where A+ is the higher level and D− is the lower level (To see how this level is calculated, visit https://socialblade.com/youtube/help). For the independent variables, we calculated the degree centrality (in-degree and out-degree) and the reciprocity of each educhannel. Out-degree measures the number of ties or recommendations from a focal node or YouTube channel to others, and in-degree measures the ties or recommendations into the given node. Reciprocity measures the number of reciprocated ties in a network. A tie is reciprocated if a recommendation is made from educhannel B to educhannel A whenever a recommendation is made from educhannel A to educhannel B (UCINET 6.0).
For the second research question, the dependent variable is the reciprocity of each educhannel. The independent variables are four of the attributes collected for each educhannel (Table 1): country, audience, topic, and format.
The analysis consisted of two main steps. First, matrices were created to obtain the SNA measures. Second, correlations and regressions to test the proposed relationship within our research questions.
First, the recommendation matrix was constructed by 412 columns and 412 rows, with a number “1” being assigned when a recommendation from one educhannel to another was present and “0” when such recommendation was absent. For each educhannel attribute (level of digital engagement, in-degree, out-degree, reciprocity, country, audience, topic, and format), we transformed the 412 rows and 1 column into a matrix with 412 rows and 412 columns by using a procedure called attribute to matrix-exact matches from the statistical package UCINET 6.0 (Borgatti, 2002). Here, “1” means that one educhannel shares the same attribute with another educhannel, and “0” is given when it differs. For example, two educhannels from Latin America will have a value of “1” in the row and column where they are related and a “0” with an educhannel from Spain. Notably, to avoid possible non-agreement because of using numeric variables such as in-degree, non-degree, and reciprocity, we categorize these three into low, medium, and high values. We applied the same categorization for digital engagement. The frequency of educhannels for each category and variable is shown in Table 2. We ended up with eight attribute matrices.
Second, a quadratic assignment procedure – correlation and regression (QAP/MRQAP) (Hubert, 1987; Krackhardt, 1988; Borgatti and Cross, 2003)—was used to analyze the data and to test the proposed relationships. These procedures are commonly used to analyze the association between networks (Han et al., 2020; Rivera et al., 2020). In QAP, one is an observed network, while the other is a model or expected network. In the first step, the algorithm computes Pearson’s correlation coefficient between corresponding cells of the two matrices. In the second step, it randomly permutes the rows and columns of one matrix synchronously and recomputes the correlation. The second step is performed hundreds of times to compute the proportion of times that a random measure is larger than or equal to the observed measure calculated in step 1. QAP and MRQAP methods generate significance levels (p-values) of the matrix relationship. P-values that are less than <0.05 suggest a strong relationship between the matrices that is unlikely to have occurred by chance (Dekker et al., 2003; Borgatti et al., 2013). In other words, p-values higher than 0.05 can be interpreted as not significant. In particular, in MRQAP, one matrix is the dependent network and is regressed on one or more independent matrices. MRQAP generates a pseudo R2 that may be interpreted analogously to the R2 statistic in ordinary least squares regression (Long and Chen, 2021). A high R2 corresponds to better model fit. The statistical package UCINET 6.0 (Borgatti, 2002) was used to conduct both statistical procedures. Results are presented and described in the following section.
To facilitate the presentation and interpretation of the results, we divided the section into two parts, each focused on a research question.
RQ1: Does a relationship exist between the centrality and reciprocity in an EduTuber’s recommendation network and their level of digital engagement on YouTube?
Table 3 shows the results for the QAP correlations. Out-degree and digital engagement (p < 0.01; β = 0.038) are positively and significantly correlated. However, in-degree (p < n.s.; β = 0.011) and reciprocity (p < n.s.; β = -0.007) do not have a significant correlation with the level of digital engagement. Moreover, out-degree and in-degree (p < 0.01; β = 0.428), as well as reciprocity and out-degree (p < 0.01; β = 0.124) have a significant, positive, and strong relationship. β indicates the strength and direction of the relationship and proportionality between the two matrices. Then, the strongest relationship between the variables is with the out-degree and in-degree. Notably, in Table 3, these results refer to exact matched attributes with low, medium, and high values. Thus, the relationships can be interpreted as follows: Channels with a similar level of digital engagement (higher levels) have a significant correlation with channels with similar levels of out-degree (higher levels). In other words, channels with higher levels of digital engagement tend to have higher levels of out-degree. In addition, channels with similar levels of out-degree (higher levels) significantly correlate to channels with similar levels of reciprocity (higher levels). That is, channels that recommend more channels tend to have higher levels of reciprocity.
Table 4 shows the MRQAP results. To test for causality, we ran different models to compare the adjusted R2, which is the best indicator of the model fit. Model 1 includes the in-degree, Model 2 includes the out-degree, Model 3 includes the reciprocity, and Model 4 includes all three independent variables. As a result, only the out-degree has a significant and positive effect on the digital engagement in Model 2 (p < 0.01; β = 0.03784) and in Model 4 (p < 0.01; β = 0.04161), because the p-values are lower than 0.05. β is the standardized regression coefficient and represents the ratio in which the independent variable affects the dependent one. Then, the strongest beta corresponds to the out-degree in Model 4.
We compared the models and found that the best fit is for Model 4 (Adj. R2 = 0.008). Perhaps the adjusted R2 is small because other variables are not included in this study and can explain the variance of the dependent variable better. Another possibility is the manner in which the relational data and the digital engagement were collected; all of them came from a database and were not obtained through questionnaires. Lastly, 5,000 permutations were run, which can reduce the size of the adjusted R2. The in-degree and reciprocity do not have a statistically significant effect on digital engagement. Therefore, the first research question is partially supported by the results. In particular, a high level of out-degree in EduTubers’ channels correspond to their higher digital engagement.
In sum, considering the results of the analyses, we find a statistically significant and positive relationship between the level of digital engagement and the level of out-degree, but not with the in-degree and reciprocity. In the next section, we present the results of the analysis to answer research question 2.
RQ2: Does a relationship exist between educhannel homophily (same country, audience, topic, and format) and the level of reciprocity in an EduTuber’s recommendation network?
Table 5 shows the QAP results and shows that same country (p < 0.01; β = 0.080), same audience (p < 0.05; β = 0.027), and same topic (p < 0.01; β = 0.039) have a significant and positive correlation with the level of reciprocity, whereas channels with the same format do not (n.s.; β = 0.004). Thus, homophily (same country, same audience, and same topic) can be interpreted to be related to the same level of reciprocity. Notably, the strongest correlation is between the same topic and the same audience (p < 0.01; β = 0.149), which is expected in the EduTubers’ recommendation network. This result occurred because collaboration and cooperation, instead of competition, seem to be particular attributes of EduTubers’ behavior. In sum, for the second research question based on the QAP analysis, results suggest a statistically significant relationship between homophily and the level of reciprocity.
To test for causality, we ran different models to compare the adjusted R2. Table 6 displays the MRQAP results. Model 1 includes the same country, Model 2 includes the same audience, Model 3 includes the same topic, Model 4 includes the same format, and Model 5 includes all the independent variables. As a result, the same country (p < 0.01; β = 0.079), same audience (p < 0.05; β = 0.026), and same topic (p < 0.01; β = 0.039) have a statistically significant and positive effect on the level of reciprocity. However, the same format (n.s.; β = 0.0038) has no effect. Model 4 shows the best fit (Adj. R2 = 0.008). Therefore, for the second research question, we statistically confirm based on the MRQAP results that homophily in terms of the same country, same audience, and same topic has a positive effect on the level of reciprocity. Thus, homophily influences the level of reciprocity in the recommendation network of the studied EduTubers.
To explore this answer in a more in-depth manner, finding which categories of the independent variables have a higher level of reciprocity is relevant. Table 7 shows the level of reciprocity between and within groups of channels that share the same attributes.
Table 7A shows that channels from Spain (R = 0.50) have a higher level of reciprocity within the group compared with the other groups. Between groups, the higher level of reciprocity is in channels from Spain and channels from Latin America (R = 0.47). Such results suggest that language is a relevant aspect of the EduTubers’ recommendation network. As expected, in Table 7B, the channels focused on teenagers (R = 0.63) have a higher level of reciprocity within, followed by the channels focused on children (R = 0.48). This result occurred perhaps because the number of specialized educhannels focused on these audiences is small. Table 7C shows that channels focused on humanities (R = 0.49) have a higher level of reciprocity among them compared with the other groups. Interestingly, channels related to health and medical sciences have a strong reciprocity with channels of agricultural sciences (R = 1.0) and social sciences (R = 1.0) but have non-reciprocity with all with channels related to engineering and technology (R = 0). Finally, Table 7D shows that DIY channels have strong reciprocity within their group (R = 0.50).
In sum, we find that a statistically significant relationship exists between educhannels homophily (same country, audience, and topic) and the level of reciprocity, but not with the same format. For instance, an increase in the level of homophily will increase the level of reciprocity. Furthermore, the greatest reciprocity occurs within the Spanish educhannels and between these and the Latin American educhannels. This finding suggests that language is a relevant aspect of the recommendation network. Finally, educhannels focused on the same audience also have higher levels of reciprocity.
The main objective of the research is to explore the dynamics of EduTubers’ recommendation network to understand the nature of the connection between them. In this regard, to answer the research questions that guided this study, we created a recommendation network of EduTubers’ channels, gathered their main attributes, analyzed their centrality and reciprocity, and measured their interrelation. We found that on YouTube, and specifically among EduTubers, cooperation and collaboration are essential.
RQ1: Does a relationship exist between the centrality and reciprocity in an EduTuber’s recommendation network and their level of digital engagement on YouTube?
The results suggest that a relationship exists between the level of digital engagement and the level of out-degree. In this sense, EduTubers’ channels that have a higher level of digital engagement tend to give a higher number of recommendations. This idea seems logical; while increasing the collaboration between channels, the traffic on the channel could be increasing too. However, Song et al. (2019) suggest that current policies of various digital platforms that encourage creators to connect with other channels are not optimal because they cause subscriber growth to decrease, thus compromising digital engagement. Even though, we found similar results as Sanders et al. (2019) in a retweet network, where results indicate that a twitter account with higher out-degree has also a higher digital engagement. Yet the data obtained from the network under study do not indicate any significant relationship between digital engagement and in-degree or reciprocity. However, some researchers report different results; for example, Wu et al. (2020) found positive effects of direct reciprocity when the channels have the same level of popularity. In addition, Wattenhofer et al. (2021) found a low level of reciprocity on channels with many subscribers. As Rowe and Alani (2014) demonstrated, the effect on the engagement varies in different social media platforms, or across different non-random datasets from the same. Thus, the causes perhaps are related to idiosyncrasies of the used datasets or applied analysis, which remarks the need for reproducing these types of studies over multiple datasets and social media platforms.
RQ2: Does a relationship exist between educhannel homophily (channel attributes) and the level of reciprocity in an EduTuber’s recommendation network?
The results confirm the positive effect of homophily (country, audience, and topic) on the level of reciprocity, i.e., an increase in the level of homophily will increase the level of reciprocity. The results suggest that language is a relevant aspect of the EduTubers’ recommendation network. The highest reciprocity occurs within the Spanish educhannels and between these and the Latin American educhannels. This finding suggests that language is a relevant aspect of the recommendation network. In addition, educhannels that focus on the same audience also present higher levels of reciprocity, perhaps because of the fewer specialized educhannels that focus on these audiences. In line with these results, Kaiser and Rauchfleisch (2020) found that the algorithm encourages the creation of highly homophilic communities and the factors of such recommendations are topics, language, and location. Another study that also found homophily in the results is that of Gruzd and Hodson (2021), who found the presence of gender homophily in channel recommendations. In addition, Wu et al. (2020) found that content creators who prefer to collaborate with smaller or larger creators would be more likely to choose others in the same content genre. Moreover, content creators who prefer to cooperate with others with similar popularity were more likely to collaborate with ones that specialize in different content genres. Our results are also similar to those of studies such as Song et al. (2019), who found that content similarity and common links increase the probability of reciprocity.
Our research makes key contributions to the literature. As Arora et al. (2019) suggested our research integrated network metrics to better understand the influence of the actors on a network. Khanam et al. (2020) pointed out, the lack of academic research based on SNA leads to a lack of knowledge about the effects of the degree of homophily on online platforms of images and videos. The main contribution of our results consists of the use of theoretical concepts (reciprocity and homophily), which focuses on the intrinsic motivations of EduTubers to recommend other channels. Another important detail to note is that each online social network is different in nature and behavior (i.e., Facebook and Twitter), which implies that dynamics in their interactions will differ (Rowe and Alani, 2014; Khanam et al., 2020). In this study, the results suggest that on YouTube and specifically among EduTubers, cooperation and collaboration are essential, unlike in other types of digital networks, which are by nature more competitive. Wu et al. (2020) proposed that cooperative behavior could help creators diversify their content, blur the community boundary, increase in size, and strengthen one’s own identity.
With regard to the practical implications, our results suggest that educhannels that are initiating and growing their digital engagement are more likely to participate in a recommendation network, i.e., make sure to recommend channels, especially channels with similar characteristics. This idea is based on our results, which indicate that a high number of channels recommended corresponds to high levels of digital engagement. This strategy may increase content exposure and discoverability, which could affect the growth of the channel (Wu et al., 2020). Furthermore, Wattenhofer et al. (2021) indicate that homophily can help information reach like-minded individuals more quickly. Thus, the recommended channels must be channels with similar content and common links (Song et al., 2019). In addition, from the content, channels are recommended to form a community among channels from the same country and with the same type of audience.
Despite the differences in the nature of today’s online social media, content creators must identify those strategies that help them capture the audience’s attention. This way, they can be recognized for their popularity, stand out from other creators, and increase digital engagement. This study finds that on YouTube, specifically in the EduTubers network, cooperation and collaboration are fundamental strategies. For instance, educhannels that are initiating and growing their digital engagement tend to participate in a recommendation network. Thus, the higher the number of channels recommended by an EduTuber, the higher the probability of increasing their digital engagement. These tactics can help them increase content exposure and discoverability, which could affect the growth of the channel (Wu et al., 2020). In addition, our results suggest that in the recommendation network, an increase in educhannel homophily will increase the level of reciprocity. Then, EduTubers should recommend similar educhannels in the same country and with the same audience and topics to increase their reciprocity. Therefore, although homophily is a fundamental phenomenon underlying human relationships in social networks, recognizing those specific attributes that enhance reciprocity and ultimately affect digital engagement will be important.
This research has a few limitations in terms of data. First, the sample of collected entry points is likely not representative of YouTube channels in general, and the resulting crawl is potentially biased by this approach. In addition, it could be considered a bias effect in some of the results of the studied indicators. Although our sample size is larger than that of other studies of EduTubers (Kaiser and Rauchfleisch, 2020; Pérez-Escoda et al., 2020; Pérez-Escoda and García-Ruiz, 2020; Arroyo et al., 2021; Fortaleza, 2021; Marcelo and Marcelo, 2021; Pattier, 2021a), we recommend future research to collect more data to analyze a more complete network of EduTubers. Second, this work is a cross-sectional study conducted at one time point. The nature of YouTube, as a dynamic platform, poses complication characteristics for academics who want to avoid this limitation. Future research could examine the evolution of the network over time to analyze changes in the community structure.
Some of the results of this study motivate us to further study the topic of the EduTuber community. Future research could explore the possibility that newer channels rely more on recommendations to increase their digital engagement rate compared consolidated channels that may use another type of strategy. Our results also suggest that some characteristics of specific conditions, such as the same country, audience, and topic, are needed to increase the reciprocity level. Future research may study this issue to confirm the thesis, find these factors, and compare it with other social networks such as Twitter or Facebook. Furthermore, future research could extend the analysis to other relevant factors such as gender, for example, as in Pattier (2021d).
The raw data supporting the conclusions of this article will be made available by the authors, without undue reservation.
All authors listed have made a substantial, direct, and intellectual contribution to the work, and approved it for publication.
The authors declare that the research was conducted in the absence of any commercial or financial relationships that could be construed as a potential conflict of interest.
All claims expressed in this article are solely those of the authors and do not necessarily represent those of their affiliated organizations, or those of the publisher, the editors and the reviewers. Any product that may be evaluated in this article, or claim that may be made by its manufacturer, is not guaranteed or endorsed by the publisher.
We acknowledge the technical support of Writing Lab, Institute for the Future of Education, Tecnologico de Monterrey, Mexico, in the production of this work.
Abdelkader, O. A. (2021). A proposed benchmark guide for customer engagement rating via YouTube channels. Turk. J. Comput. Mathemat. Educ. 12, 2781–2791.
Abul-Fottouh, D., Song, M. Y., and Gruzd, A. A. (2020). Examining algorithmic biases in YouTube’s recommendations of vaccine videos. Int. J. Med. Inform. 140:104175. doi: 10.1016/j.ijmedinf.2020.104175
Arroyo, D. G. C., Peralta, S. M., and Lugo, I. V. (2021). Estudio de caso de los canales de edutubers mexicanos especializados en contenidos para profesores. Rev. Electr. de Invest. e Innov. Educ. 6, 25–37.
Arora, A., Bansal, S., Kandpal, C., Aswani, R., and Dwivedi, Y. (2019). Measuring social media influencer index- insights from facebook, Twitter and Instagram. J. Retail. Consum. Serv. 49, 86–101. doi: 10.1016/j.jretconser.2019.03.012
Asociación de Internet, M. X. (2021). 16° Estudio sobre los Hábitos de los Usuarios de Internet en México 2020. Available online at https://www.asociaciondeinternet.mx/estudios/asociacion (accessed March 09, 2022).
Bonsón, E., and Ratkai, M. (2013). A set of metrics to assess stakeholder engagement and social legitimacy on a corporate Facebook page. Onl. Inform. Rev. 37, 787–803. doi: 10.1108/OIR-03-2012-0054
Borgatti, S. P. (2002). A statistical method for comparing aggregate data across a priori groups. Field Methods 14, 88–107. doi: 10.1177/1525822x02014001006
Borgatti, S. P. (2005). Centrality and network flow. Soc. Net. 27, 55–71. doi: 10.1016/j.socnet.2004.11.008
Borgatti, S. P., and Cross, R. (2003). A relational view of information seeking and learning in social networks. Manage. Sci. 49, 432–445. doi: 10.1287/mnsc.49.4.432.14428
Borgatti, S. P., Everett, M. G., and Johnson, J. C. (2013). Analyzing Social Networks. London: SAGE Publication.
Carmichael, D., and Archibald, J. (2019). A Data Analysis of the Academic use of Social Media. Int. J. Inform. Technol. Comput. Sci. 5, 1–10. doi: 10.5815/ijitcs.2019.05.01
Cropanzano, R., and Mitchell, M. S. (2005). Social exchange theory: an interdisciplinary review. J. Manage. 31, 874–900. doi: 10.1177/0149206305279602
Cheng, X., Dale, C., and Liu, J. (2008). “Statistics and social network of youtube videos,” in 2008 16th International Workshop on Quality of Service, (Enschede, Netherlands: IEEE), 229–238.
Dekker, D., Krackhardt, D., and Snijders, T. (2003). “Multicollinearity robust QAP for multiple regression,” in 1st annual conference of the North American Association for Computational Social and Organizational Science, (Pittsburgh, PA). 22–25.
Doreian, P., and Woodard, K. L. (1992). Fixed list versus snowball selection of social networks. Soc. Sci. Res. 21, 216–233. doi: 10.1016/0049-089x(92)90016-a
Easley, D., and Kleinberg, J. (2010). Positive and negative relationships. Networks, Crowds, and Markets: Reasoning about a Highly Connected World. Cambridge: Cambridge University Press: UK.
Eisenberger, R., Armeli, S., Rexwinkel, B., Lynch, P. D., and Rhoades, L. (2001). Reciprocation of perceived organizational support. J. Appl. Psychol. 86:42. doi: 10.1037/0021-9010.86.1.42
Freeman, L. C. (1979). Centrality in social networks conceptual clarification. Soc. Netw. 1, 215–239. doi: 10.1016/0378-8733(78)90021-7
Fortaleza, M. (2021). “La educación a través de YouTube: Análisis de los canales de edutubers españoles,” in Docencia, ciencia y humanidades: hacia un enseñanza integral en la universidad del siglo XXI. 1st ed, eds M. Pallarés, J. Quintana, and A. Santisteban (Spain: Dykinson), 71–87.
Google. (2019). La formación se vuelve tendencia en YouTube. Available online at https://espana.googleblog.com/2019/02/la-formacion-se-vuelve-tendencia-en.html. (accessed November 25, 2021).
Gruzd, A., and Hodson, J. (2021). Making Sweet Music Together: the Affordances of Networked Media for Building Performance Capital by YouTube Musicians. Soc. Media Soc. 7, 1–15. doi: 10.1177/20563051211025511
Halberstam, Y., and Knight, B. (2016). Homophily, group size, and the diffusion of political information in social networks: evidence from Twitter. J. Pub. Econ. 143, 73–88. doi: 10.1016/j.jpubeco.2016.08.011
Han, S. H., Yoon, S. W., and Chae, C. (2020). Building social capital and learning relationships through knowledge sharing: a social network approach of management students’ cases. J. Knowled. Manage. 24, 921–939. doi: 10.1108/jkm-11-2019-0641
Hubert, L. J. (1987). Assignment Methods in Combinatorial Data Analysis, Dekker. New York: CRC Press.
Kaiser, J., and Rauchfleisch, A. (2020). Birds of a Feather Get Recommended Together: algorithmic Homophily in YouTube’s Channel Recommendations in the United States and Germany. Soc. Media Soc. 4, 1–15. doi: 10.1177/2056305120969914
Katona, Z., and Sarvary, M. (2008). Network formation and the structure of the commercial world wide web. Market. Sci. 27, 764–778. doi: 10.1287/mksc.1070.0349
Khanam, K. Z., Srivastava, G., and Mago, V. K. (2020). The Homophily Principle in Social Network Analysis. Anal. Comput. Syst 37, 111. doi: 10.1145/1122445.1122456
Kossinets, G., and Watts, D. J. (2009). Origins of homophily in an evolving social network. American J. Sociol. 115, 405–450. doi: 10.1086/599247
Krackhardt, D. (1988). Predicting with networks: nonparametric multiple regression analysis of dyadic data. Soc. Netw. 10, 359–381. doi: 10.1016/0378-8733(88)90004-4
Lawrence, B. S., and Shah, N. P. (2020). Homophily: measures and meaning. Acad. Manage. Ann. 14, 513–597. doi: 10.5465/annals.2018.0147
Lazarsfeld, P. F., and Merton, R. K. (1954). Friendship as a social process: a substantive and methodological analysis. Freedom Control Moder. Soc. 18, 18–66.
López, A. (2019). El fenómeno de los EduTubers. Estudio sobre las habilidades comunicativas de los YouTubers educativos más populares. Argentina: Universidad Austral, Argentina. [Ph.D. Thesis].
López, J., Maza-Cordova, J., and y Tusa, F. (2020). Educar en el contexto digital: el reto de ser EduTuber. Risti 25, 188–200.
López-Navarrete, A. J., Cabrera-Méndez, M., Díez-Somavilla, R., and Calduch-Losa, Á (2021). Formula for measuring the engagement of the viewer on YouTube: exploratory research on the main Spanish youtubers. Mediterr. J. Commun. 12, 143–156. doi: 10.14198/MEDCOM000013
Long, Y., and Chen, Y. (2021). Impact of structural embeddedness on the performance of tourism supply chain integration: a social network perspective. Ann. Operations Res. 1–17.
Ma, L., Krishnan, R., and Montgomery, A. L. (2015). Latent homophily or social influence? An empirical analysis of purchase within a social network. Manage. Sci. 61, 454–473. doi: 10.1287/mnsc.2014.1928
Marcelo, C., and Marcelo, P. (2021). Educational influencers on Twitter. Anal. Hashtags Relat. struct. 68, 73–83. doi: 10.3916/C68-2021-06
Martínez, S., and Cedillo, J. (2020). ¿Así que quieres ser YouTuber divulgador/a de la ciencia?. Cienciorama. Available online at http://cienciorama.unam.mx/a/pdf/656_cienciorama.pdf (accessed April 29, 2021).
McPherson, M., Smith-Lovin, L., and Cook, J. M. (2001). Birds of a feather: homophily in social networks. Ann. Rev. Sociol. 27, 415–444. doi: 10.1146/annurev.soc.27.1.415
Mayzlin, D., and Yoganarasimhan, H. (2012). Link to success: how blogs build an audience by promoting rivals. Manage. Sci. 58, 1651–1668. doi: 10.1287/mnsc.1110.1510
Mitchell, M. S., Cropanzano, R. S., and Quisenberry, D. M. (2012). “Social exchange theory, exchange resources, and interpersonal relationships: A modest resolution of theoretical difficulties,” in Handbook of social resource theory, eds K. Törnblom and A. Kazemi (New York, NY: Springer), 99–118. doi: 10.1007/978-1-4614-4175-5_6
Mollica, K. A., Gray, B., and Trevino, L. K. (2003). Racial homophily and its persistence in newcomers’ social networks. Organ. Sci. 14, 123–136. doi: 10.1287/orsc.14.2.123.14994
Nowak, M. A. (2006). Five rules for the evolution of cooperation. Science 314, 1560–1563. doi: 10.1126/science.1133755
Organization for Economic Co-operation and Development. (2007). Revised fields of science and technology (FOS) classification in the FRACATI manual. Available online at https://docs.google.com/viewer?a=v&pid=sites&srcid=Y29uY3l0ZWMuZ29iLnBlfG1hbnVhbC11c28tZGluYS10ZXN0fGd4OjFjMjk3MTU4ZTNkMDA3NDg (accessed on 26 Feb 2007).
Pan, J., Bhardwaj, R., Lu, W., Chieu, H. L., Pan, X., and Puay, N. Y. (2019). “Twitter homophily: Network based prediction of user’s occupation,” in Proceedings of the 57th Annual Meeting of the Association for Computational Linguistics, (Florence: Association for Computational Linguistics), 2633–2638.
Paolillo, J. C. (2008). “Structure and Network in the YouTube Core,” in Proceedings of the 41st Annual Hawaii International Conference on System Sciences (HICSS 2008), (Piscataway: IEEE), 156–156. doi: 10.1109/HICSS.2008.415
Pattier, D. (2021a). Referentes educativos durante la pandemia de la COVID-19. Publicaciones 51, 533–548. doi: 10.30827/publicaciones.v51i3.18080
Pattier, D. (2021b). Educating in Art and Culture Through YouTube: the Impact of Edutubers. Komunikacija i kultura online 12, 167–181. doi: 10.18485/kkonline.2021.12.12.10
Pattier, D. (2021c). Science on YouTube: successful EduTubers. TECHNO REVIEW.. Int. Technol., Sci. Soc. Rev. 10, 1–15. doi: 10.37467/gka-revtechno.v10.2696
Pattier, D. (2021d). The Gender Gap Among EduTubers and the Factors Significantly Influencing It. J. N. Approach. Educ. Res. 10, 313–329. doi: 10.7821/naer.2021.7.732
Pérez-Escoda, A., and García-Ruiz, R. (2020). “El engagement del influencer en YouTube como recurso educativo para la innovación en el aula,” in (Presidencia). I Congreso Internacional de Comunicación y Redes Sociales, ed. F. García García (New York: McGrawHill).
Pérez-Escoda, A., Jiménez-Narros, C., Perlado-Lamo-de-Espinosa, M., and Pedrero-Esteban, L. M. (2020). Social Networks’ Engagement During the COVID-19 Pandemic in Spain: health Media vs. Healthcare Professionals. Int. J. Environ. Res. Public Health 17, 5261. doi: 10.3390/ijerph17145261
Pletikosa Cvijikj, I., and Michahelles, F. (2013). Online engagement factors on Facebook brand pages. Soc. Netw. Anal. Minin. 3, 843–861. doi: 10.1007/s13278-013-0098-8
Rangarajan, K., Begg, K., and Somani, B. (2019). Online Digital Media: the Uptake of YouTube-based Digital Clinical Education (DCE). Am. J. Distance Educ. 33, 142–150. doi: 10.1080/08923647.2019.1582308
Rivera, A. E., Rodríguez-Aceves, L., and Mojarro-Duran, B. I. (2020). Enabling knowledge sharing through psychological safety in inter-organisational arrangements. J. Knowledg. Manage. 25, 1170–1193. doi: 10.1108/JKM-04-2020-0241
Rogers, R. (2015). “Digital methods for web research,” in Emerging Trends in the Social and Behavioral Sciences: An Interdisciplinary, Searchable, and Linkable Resource, eds R. Scott and S. Kosslyn (Hoboken, NJ, USA: John Wiley & Sons, Inc).
Rowe, M., and Alani, H. (2014). “Mining and comparing engagement dynamics across multiple social,” in Proceedings of the 2014 ACM conference on Web science (WebSci ’14), (New York, NY, USA: Association for Computing Machinery), 229–238. doi: 10.1145/2615569.2615677
Sabate, F., Berbegal-Mirabent, J., Cañabate, A., and Lebherz, P. (2014). Factors influencing popularity of branded content in Facebook fan pages. Euro. Manage. J. 32, 1001–1011. doi: 10.1016/j.emj.2014.05.001
Sanders, W., Wang, Y., and Zheng, Q. (2019). Brand’s Social Media Presence as Networks: the Role of Interactivity and Network Centrality on Engagement. Commun. Res. Rep. 36, 1–11. doi: 10.1080/08824096.2019.1590192
Sherif, M. (1958). Superordinate goals in the reduction of intergroup conflict. Am. J. Sociol. 63, 349–356. doi: 10.1086/222258
Sinnema, C., Daly, A. J., Liou, Y. H., and Rodway, J. (2020). Exploring the communities of learning policy in New Zealand using social network analysis: a case study of leadership, expertise, and networks. Int. J. Educ. Res. 99:101492. doi: 10.1016/j.ijer.2019.10.002
Song, T., Tang, Q., and Huang, J. (2019). Triadic closure, homophily, and reciprocation: an empirical investigation of social ties between content providers. Inform. Syst. Res. 30, 912–926. doi: 10.1287/isre.2019.0838
SocialBlade (n.d.). Frequently Asked Questions. Available online at: https://socialblade.com/youtube/help (accessed November 25, 2021).
Stephen, A. T., and Toubia, O. (2010). Deriving value from social commerce networks. J. Market. Res. 47, 215–228. doi: 10.1509/jmkr.47.2.215
Stolz, S., and Schlereth, C. (2021). Predicting tie strength with ego network structures. J. Interact. Market. 54, 40–52. doi: 10.1016/j.intmar.2020.10.001
Takahashi, N. (2000). The emergence of generalized exchange. Am. J. Soc. 105, 1105–1134. doi: 10.1086/210400
Torres, I., and Conrad, J. (2015). CS 224 W Project Milestone Analysis of the YouTube Channel Recommendation Network. Available online at http://snap.stanford.edu/class/cs224w-2015/projects_2015/Analysis_of_the_YouTube_Channel_Recommendation_Network.pdf (accessed November 02, 2021).
Tur-Viñes, P., Núñez-Gómez, M. J., and González-Río. (2018). Kid influencers on YouTube. A space for responsibility. Revista Latina de Comunicación Social 73, 1211–1230. doi: 10.4185/RLCS-2018-1303
Wattenhofer, M., Wattenhofer, R., and Zhu, Z. (2021). “The YouTube social network”, in Proceedings of the 6th International AAAI Conference on Web and Social Media, Dublin.
Wang, W. K., Lu, W. M., Kweh, Q. L., Nourani, M., and Hong, R. S. (2021). Interlocking directorates and dynamic corporate performance: the roles of centrality, structural holes and number of connections in social networks. Rev. Manage. Sci. 15, 437–457. doi: 10.1007/s11846-019-00347-2
Wang, C., Zhang, X., and Hann, I. H. (2018). Socially nudged: a quasi-experimental study of friends’ social influence in online product ratings. Inform. Syst. Res. 29, 641–655. doi: 10.1287/isre.2017.0741
Wu, B., Liu, C. Z., and Zhu, H. (2020). “Will cooperation help content creators grow? Empirical evidence from twitch.tv,” in Proceedings of the 41st International Conference on Information Systems, ICIS 2020, Hyderabad.
YouTube. (2018). A final update on our priorities for 2018. Available Online at https://blog.YouTube/inside-YouTube/a-final-update-on-our-priorities-for (accessed on Oct.22.2018).
YouTube Official Blog. (2020). COVID-19: Resources to help people learn on YouTube. Available online at https://blog.YouTube/news-and-events/covid-19-resources-to-help-people-learn/ (accessed on 20 Mar 2020).
Xie, L. (2017). Subscriber Number is Not Everything: YouTube Community Engagement Measurement. St, Murfreesboro: Middle Tennessee State University. [Ph D thesis].
Keywords: EduTuber, educational videos, YouTube, social network analysis, educational innovation, communities
Citation: Pasquel-López C, Rodríguez-Aceves L and Valerio-Ureña G (2022) Social Network Analysis of EduTubers. Front. Educ. 7:845647. doi: 10.3389/feduc.2022.845647
Received: 30 December 2021; Accepted: 06 April 2022;
Published: 19 May 2022.
Edited by:
Diana Hernández Montoya, Universidad Estatal a Distancia, Costa RicaReviewed by:
Christian Bokhove, University of Southampton, United KingdomCopyright © 2022 Pasquel-López, Rodríguez-Aceves and Valerio-Ureña. This is an open-access article distributed under the terms of the Creative Commons Attribution License (CC BY). The use, distribution or reproduction in other forums is permitted, provided the original author(s) and the copyright owner(s) are credited and that the original publication in this journal is cited, in accordance with accepted academic practice. No use, distribution or reproduction is permitted which does not comply with these terms.
*Correspondence: Cynthia Pasquel-López, YTAwNjA5NzEwQHRlYy5teA==
Disclaimer: All claims expressed in this article are solely those of the authors and do not necessarily represent those of their affiliated organizations, or those of the publisher, the editors and the reviewers. Any product that may be evaluated in this article or claim that may be made by its manufacturer is not guaranteed or endorsed by the publisher.
Research integrity at Frontiers
Learn more about the work of our research integrity team to safeguard the quality of each article we publish.