- Department of Biology, Bioscience Education and Zoo Biology, Goethe University, Frankfurt, Germany
Out-of-school laboratories, also called student labs, are an advantageous opportunity to teach biological subjects. Particularly in the case of complex fields such as neurobiology, student labs offer the opportunity to learn about difficult topics in a practical way. Due to numerous advantages, digital student labs are becoming increasingly popular nowadays. In this study, we investigated the effect of an electrophysiological setup for a virtual experiment with and without hands-on elements on participant motivation and technology acceptance. For this purpose, 235 students were questioned during a student laboratory day. The surveyed students showed high motivation and technology acceptance for the virtual experiment. In the comparison, the electrophysiological setup with hands-on elements performs better in the intrinsic components than the setup without hands-on elements: Thus, the hands-on approach is rated as more interesting and the perceived enjoyment scores higher. Nevertheless, both experimental groups show high values, so that the results of the study support the positive influence of digital laboratory as well as a positive influence of hands-on elements.
Introduction
Neurobiology has become an integral part of biology curricula, both in middle and high school (Gage, 2019). However, students still encounter problems in understanding neuroscience topics. Even university students have problems understanding abstract concepts such as the emergence of the resting potential (Manalis and Hastings, 1974; Barry, 1990). However, more recent studies also reveal existing misconceptions, for example, regarding membrane potential (Silverthorn, 2002) or synaptic transmission (Montagna et al., 2010). There are diverse explanations for the problems. One of the reasons for the misconceptions could be that students often memorize facts about neurobiology without understanding the underlying concepts (Krontiris-Litowitz, 2003). Griff (2018) believes the problems include a lack of basic understanding of physical and chemical principles.
Teaching Neuroscience
It is widely known that more active approaches can improve understanding of a topic (Michael, 2006). Experimental approaches in neurobiology have shown that problems of understanding can be addressed (Marzullo and Gage, 2012; Shannon et al., 2014). Thereby, the exact type of practical work is less important (Lewis, 2014). But active approaches are often impossible to implement in schools due to limited resources (Dagda et al., 2013), and participation in hands-on laboratory work on the topic is often not possible (Albarracín et al., 2016). Schools lack simple, compelling, and inexpensive tools with which to conduct neurobiology topics, such as electrophysiological measurements (Marzullo and Gage, 2012).
To address the problem that neurophysiological processes are often not visible and difficult to understand, there is a whole range of teaching approaches and methods. For example, structural models for a better illustration of the neurobiological structures (Krontiris-Litowitz, 2003; Keen-Rhinehart et al., 2009) or functional models in which bioelectric potentials can be traced with simplified replicas of neurons (Dabrowski et al., 2013; Shlyonsky, 2013) can be used. However, visualization of non-visible processes through analogies (Procopio, 1994; Milanick, 2009; Griff, 2018), explanatory models (Wright, 2004; Cardozo, 2016), or even role-playing (Carvalho, 2011; Holloway, 2013) are also part of the repertoire of neurobiological teaching approaches. Direct access to authentic neuroscience research methods can be provided by electrophysiological experiments and preparations (e.g., Le Guennec et al., 2002; Dagda et al., 2013; Shannon et al., 2014). However, conducting experiments in electrophysiology is extremely challenging and costly (Diwakar et al., 2014; Lewis, 2014).
Computer-Based Teaching Approaches
To address this problem, the use of neurosimulations in biology classes offers a number of advantages and teaching possibilities, especially in comparison to the practical application of research methods. One of the main arguments for neurosimulations is better resource availability (Chinn and Malhotra, 2002; Hofstein and Lunetta, 2004). While laboratory equipment for electrophysiological experiments costs thousands of dollars (Diwakar et al., 2014), virtual experiments often only require computers and thus no special equipment or rooms (Grisham, 2009). Another reason for conducting neurobiology experiments virtually is the increasing concern for animal welfare (e.g., Dewhurst, 2006; Knight, 2007; Kaisarevic et al., 2017).
In addition, computer-based approaches can be designed specifically to contribute to a better understanding of the topic (Meuth et al., 2005; Ma and Nickerson, 2006; de Jong et al., 2013; Lewis, 2014). Virtual experiments can be seen as an effective tool to help learners understand the basic concepts of neurophysiology (Bish and Schleidt, 2008; Stuart, 2009) and often provide learning outcomes that are comparable to or exceed those of traditional laboratories (e.g., Dewhurst, 2006; Knight, 2007; Sheorey and Gupta, 2011; Brinson, 2015; Quiroga and Choate, 2019). Over the years, various neurosimulations have therefore been developed with different emphases and special features (e.g., Barry, 1990; Schwab et al., 1995; Braun, 2003; Crisp, 2012; Wang et al., 2018). Nevertheless, it is often argued that learners do not practice using certain tools with mouse clicks and thus important experimental or technical skills cannot be learned (Braun, 2003; Lewis, 2014). Research on combining virtual setups with hands-on elements in student labs currently proves to have significant gaps. In addition, the approaches described are primarily designed for higher education, so that a teaching concept and its effectiveness for the school sector are in particular need of research. Two factors that are of particular importance in this context are the motivation for the experiments, as a predictor of learning success, and the technology acceptance to determine whether the setup used is the appropriate tool. Estriegana et al. (2019) explain that innovative learning technologies offer opportunities to improve learners’ understanding. However, these expectations are only true if learners are interested in using the technology. The best tool fails if it does not capture learners’ interest or motivate them to use it. Therefore, it is necessary to understand learners’ potential acceptance or rejection and determine the influencing factors. This was investigated using two test constructs (motivation and technology acceptance).
Motivation
According to the classical definition, motivation means being moved to do something (Ryan and Deci, 2000). Motivation is a central concept to explain behavior and the duration and intensity of engagement with something (Schiefele and Schaffner, 2015). It is considered one of the foundations for willingness to learn (Krapp, 2003; Pintrich, 2003). A distinction is often made between intrinsic and extrinsic motivation. The extrinsic motivation on the one hand leads to an activity of a person because it is perceived as serving to achieve valued outcomes. Intrinsic motivation, on the other hand, leads to an activity for no apparent reinforcement other than the performance of the activity itself, making it purpose and reward (Ryan and Deci, 2000). According to the person-object theory, the quality of intrinsic motivation is one of the main reasons for performing an interest-related action (Krapp, 2007). Accordingly, motivation plays an important role in addressing the deficits in learners’ neurobiological knowledge and skills. Empirical studies support the assumption that performing practical activities increases motivation (Dohn et al., 2016). According to the model of Betz (2018), a motivational effect can result from the perception of scientific authenticity.
Technology Acceptance
Due to the growing dependence on computer-based systems and the increase of new technologies, user acceptance of technologies still has a high relevance today. Acceptance is important for the motivational and action-oriented components, which in turn influence how intensively an object is engaged with (Müller-Böling and Müller, 1986). A number of models have been developed to examine how individuals adopt new technologies, with the prevalent ones being the Technology Acceptance Model (TAM; Taherdoost, 2018). The TAM was developed by Davis (1985) to examine the reasons why new technologies are adopted or rejected. The theoretical basis of the model is based on the social psychological theory of reasoned action, which describes a model for predicting behavior that is related to attitude (Ajzen and Fishbein, 1980). Various explanatory factors are used to explain user acceptance in the TAM (Davis, 1989; Davis et al., 1989). The two core components for predicting acceptance are Perceived Usefulness (PU) and Perceived Ease of Use (PEOU). PU is the degree to which a person believes that using a particular system is improving their work performance, and PEOU is the degree to which a person believes that using a particular system is free of physical and mental effort and strain (e.g., Davis, 1989; Venkatesh and Bala, 2008). While PU and PEOU can be seen more as extrinsic influencing factors, other work inserts Perceived Enjoyment (PE) as an intrinsic explanatory component in the model (Davis et al., 1992; Yi and Hwang, 2003; Lee et al., 2005). Accordingly, PE is the degree to which an activity using a technology is perceived as enjoyable or pleasurable to itself (Davis et al., 1992).
Research Question and Purpose
The TAM is frequently used in educational contexts for research purposes (Granić and Marangunić, 2019), such as in the e-learning field (Šumak et al., 2011). In the school context, a special need for research emerges due to the increasing involvement of technologies - however, in the review by Granić and Marangunić (2019), only 4% of studies in the educational context show an investigation of technology acceptance among students. Many studies on neurosimulations can demonstrate the acceptance for computer-based experiments by learners (e.g., Braun, 2003; Demir, 2006; Newman and Newman, 2013).
The two main research questions of this study are: Do students show acceptance and motivation towards a neurosimulation and can additional hands-on elements increase this effect? In this context, the structure of the two measurement instruments used will also be examined.
Materials and Methods
The study was carried out as part of the neuroscience student lab at the Goethe University Frankfurt am Main. The lab is an extracurricular school laboratory in which school classes can experience neurobiological topics in a practical way. The topics of the lab days are designed to fit the school curricula. For this study, a student lab day was conducted on the topic of electrophysiology, with a focus on the functional properties of neurons. To investigate the impact of supplementary hands-on elements when conducting the virtual experiments, two different setups were created: A computer-based neurosimulation (NS) and the same neurosimulation but with supplementary hands-on elements (NS-HO).
Neurosimulation
For the laboratory day, the ganglion of the medicinal leech (Hirudo medicinalis) was used as the model nervous system. The electrophysiological measurements used were authentic recordings previously conducted and recorded by scientists, what distinguishes our neurosimulation from most others. For the monitoring and execution of the experiments, a neurosimulation was developed that was adapted to the needs of the students and had a simple and easy to understand navigation menu (Figure 1). The students controlled the experiments independently using a graphical user interface (GUI): They activate a microelectrode and select the neuron by mouse click on a picture of the ganglion. During the laboratory day, the students applied different agonists. Depending on the selected neuron and the experiment protocol, the measurement data of the respective experiment were imported from a database and dynamically displayed.
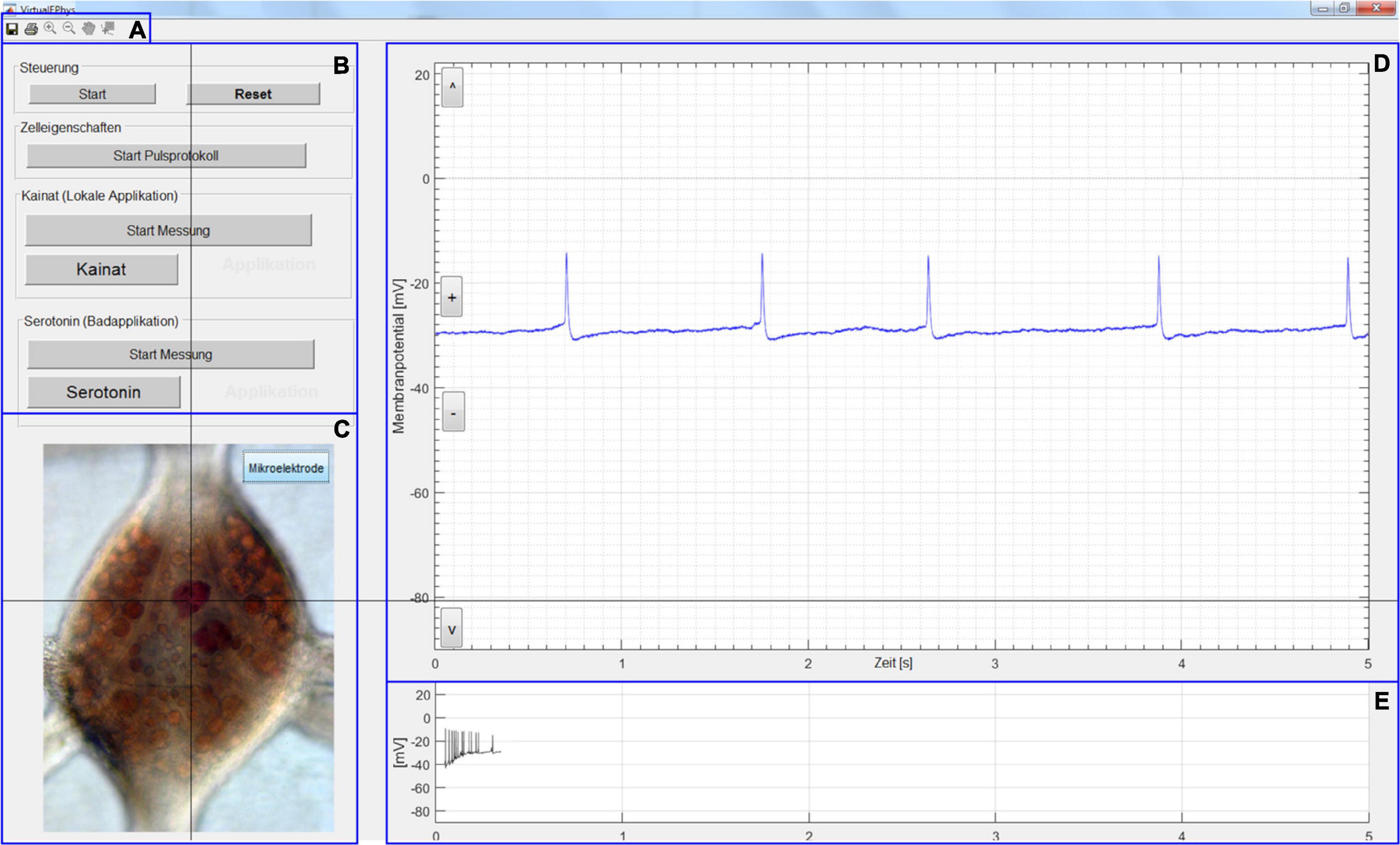
Figure 1. The graphical user interface (GUI) of the neurosimulation to control the experiments and display the measurement traces. The GUI includes: (A) a toolbar to zoom in the measurement trace, (B) buttons to control the experiments and applications, (C) a photo of the leech ganglion where the model neuron can be selected after activating the microelectrode button (crosshair), (D) a main measurement window that displays the membrane potential of the measurement traces live; in this example, the application of kainate (E) a second measurement window that allows to see the complete measurement.
Neurosimulation With Hands-On Elements
The difference in operation compared to the NS is that in the NS-HO the insertion of the electrode into the ganglion is not done by mouse click in the GUI, but with a quasi-real measurement setup (hands-on elements, Figure 2). To implement the research method as authentically as possible for the students, the hands-on elements were based on a real electrophysiological measurement setup, including a binocular for viewing a virtual ganglion preparation and a micromanipulator for controlling the position of the electrode. The virtual preparation was a picture of the leech ganglion, placed on a circuit board. Four pairs of contacts on the board represented different types of neurons found in the leech ganglion. The student used the electrode to make contact with the neuron on the board (Figure 3). To import the measurement from database, the circuit board and the electrode were connected to a computer via an Input/Output board.
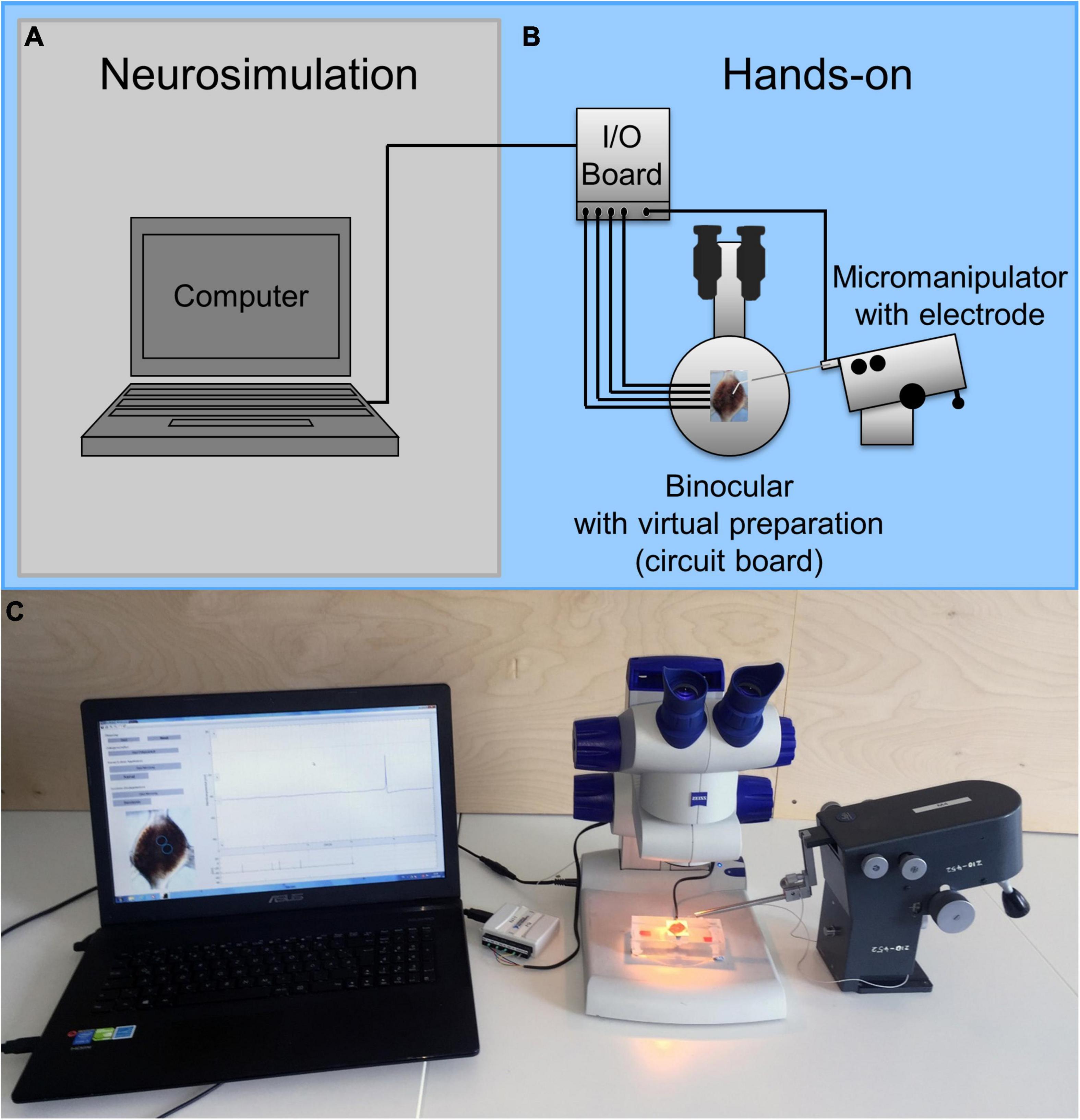
Figure 2. Structure of (A) the Neurosimulation (NS) and (B) the Neurosimulation with Hands-On Elements (NS-HO). The developed neurosimulation was run on the computer. Using a binocular and micromanipulator with fixed microelectrode, the students were able to insert the electrode into the preparation and observe the dynamic results on the laptop (C).
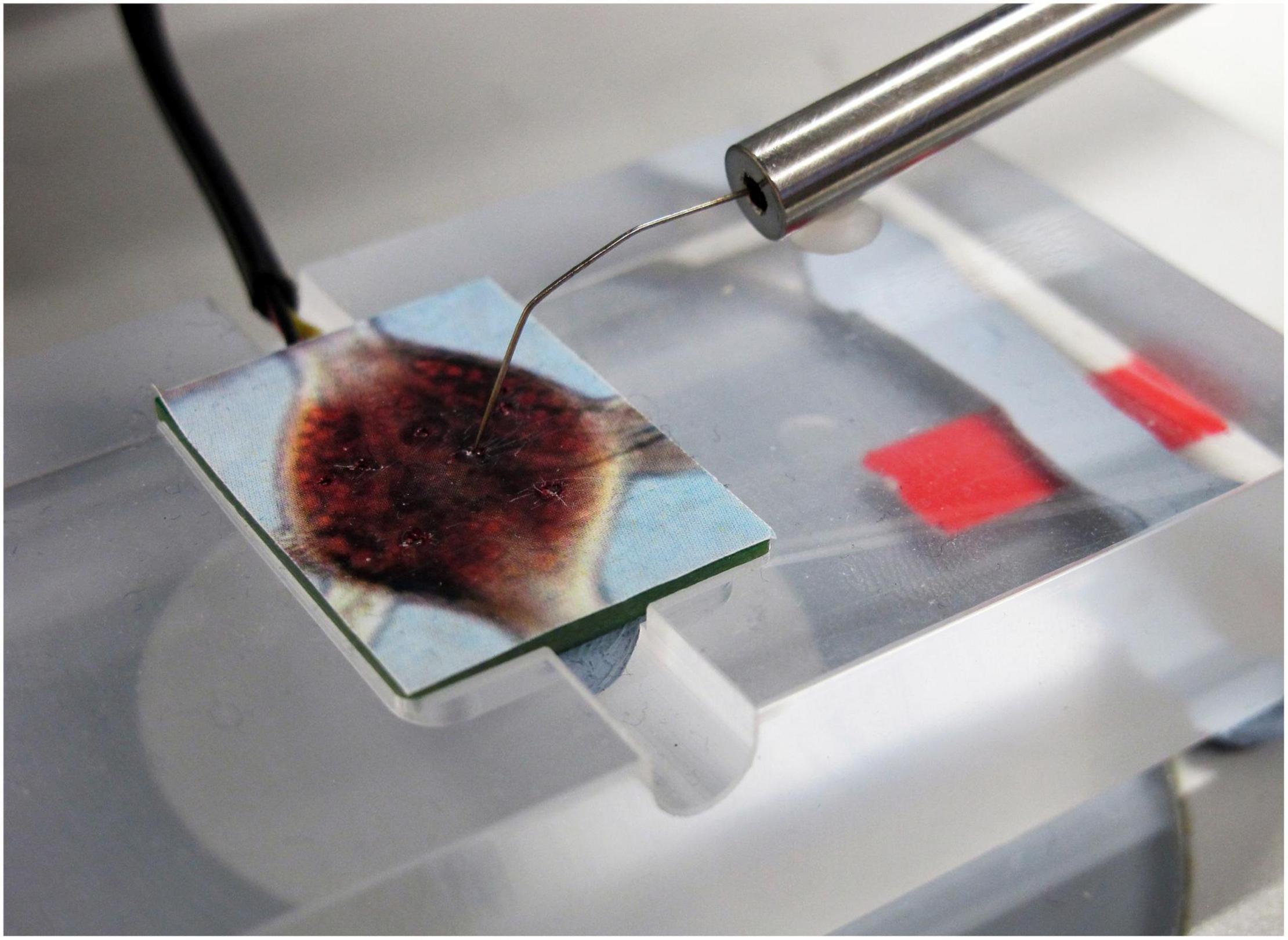
Figure 3. Virtual preparation (picture of the leech ganglion on circuit board) on microscope slide with attached microelectrode.
Procedure of the Laboratory Day
The lab day of this study focused on electrophysiology. During the lab day, the students became familiar with research and methods in neurobiology, learned about important morphological and functional properties of nerve cells, and performed electrophysiological measurements themselves. The course day began with a thematic introductory presentation. In this presentation, important basics such as the resting potential, the generation of an action potential, and the transmission and forwarding of action potentials were recalled. The leech as an experimental organism was also presented. Following the introduction, participants were randomly divided into two groups. Group one performed the neurosimulation (NS) only with a computer and the installed user interface, while group two performed the neurosimulation with the supplementary hands-on elements (NS-HO). After performing the simulations, the lab day was evaluated using a paper-pencil survey.
Participants
In this study, 235 high school students (63.4% female, 36.6% male), who visited the student lab with their teachers, were surveyed. The majority of the sample (85.1%) had chosen biology as an advanced course in school and participants were between 17 and 20 years old. Prior to the survey, all participants, and additionally their parents in the case of minors, were informed in writing about the purpose of the study, the voluntary nature of participation and the anonymity of responses. Before participating in the study, the students had to give their written consent. For underage study participants, parental consent was also obtained. Classes received a reduced participation fee for taking the survey. If individuals did not participate in the survey, all students still received the reduced fee. The survey took place between August 2017 and February 2018. A total of 160 individuals participated in the NS, and 75 individuals participated in the NS-HO group.
Measuring Instruments
The Lab Motivation Scale (LMS) was used to survey motivation for the electrophysiological experiments of the lab day, and components of the Technology Acceptance Model (TAM) were used to evaluate corresponding acceptance.
Lab Motivation Scale
A questionnaire aligned with the LMS was used for the comparative survey on the motivation for simulation with and without hands-on elements. The LMS was developed in 2016 by Dohn et al. (2016) to examine how laboratory work influences motivation regarding topics in physiology among students. The original scale consists of 21 items divided into three components: situational interest (Interest; e.g., “Working with the setup was fun to do”), willingness to engage (Effort; e.g., “I think I did pretty well working with the setup”), and confidence in understanding (Self-Efficacy; e.g., “I feel confident to explain electrophysiological measurements”). The 14 applicable items were adapted to the neurosimulation and in this study rated on a 4-point Likert scale.
Technology Acceptance Model
For the comparative survey on the acceptance a questionnaire aligned with the TAM was developed (Davis, 1989; Davis et al., 1989). From four published papers that conducted a survey using TAM items, the ones applicable to the setup were adopted (Davis et al., 1989; Venkatesh and Bala, 2008; Park, 2009; Diwakar et al., 2014). In addition to the two standard components PU (e.g., “The virtual setup will make it easier to do my studies”) and PEOU (e.g., “I find the virtual setup easy to use”) introduced by Davis in 1989, this study also investigated PE (Liaw and Huang, 2003; Yi and Hwang, 2003; Serenko, 2008; Venkatesh and Bala, 2008) with items such as “I find using the virtual setup to be enjoyable.” The scale used here consists of 18 items rated on a 5-point Likert scale in this study. The detailed scale documentation can be found in the Appendix Table 1.
Analysis
To verify the factor structure of the two instruments used, exploratory factor analyses with varimax rotation were performed. Previously, the sampling adequacy was examined using the Barlett test and the Kaiser-Meyer-Olkin test (KMO). Our sample size was adequate according to the common rule of thumb that at least 10 people per variable should be surveyed (Field, 2009). The examination of the factor structure is particularly important in the case of the LMS because, on the one hand, it is a shortened version of the original scale and, on the other hand, a different age group was surveyed than in the original study by Dohn et al. (2016). Cronbach’s alpha was calculated for each factor to evaluate reliability and internal consistency. Normal distribution was tested using the Kolmogorov-Smirnov test. Because the Kolmogorov-Smirnov test showed a significant result for all data (p < 0.05), a normal distribution cannot be assumed. Therefore, to examine the difference of the TAM and the LMS between the NS and NS-HO groups, the Mann-Whitney U test was used. For significant results the effect size r was calculated using the formula (Fritz et al., 2012).
Results
For the 14 items of the LMS, the Barlett test (p < 0.001) and the KMO (KMO = 0.885) confirmed the applicability of a factor analysis. The factor analysis of the LMS items showed a three factor solution. The three factors together explained 64.82% of the variance. The factor distribution of the items is consistent with the theoretical assumptions and item distribution of Dohn et al. (2016). The items loaded on their respective components with factor loadings above 0.5 and the Cronbach α values were above 0.7 and thus in the good to very good range (Table 1). The comparison between the NS and the NS-HO showed no significant difference between the groups for the Effort (p = 0.485; MedianNS = 3.5; IQRNS = 1.00; MedianNS–HO = 3.25; IQRNS–HO = 0.75) and Self-Efficacy (p = 0.565; MedianNS = 3.00; IQRNS = 1.00; MedianNS–HO = 3.00; IQRNS–HO = 0.80) components. For the Interest component, a significant difference with a small effect size was observed between the two study setups (p = 0.018; r = 0.16; MedianNS = 3.00; IQRNS = 0.85; MedianNS–HO = 3.20; IQRNS–HO = 0.80; Figure 4). Self-Efficacy had a lower score in both groups (mean = 2.97) with a great variance (IQRNS = 1.00; IQRNS–HO = 0.80). All components of the LMS had medians greater than or equal to 3, which represents a positive evaluation range.
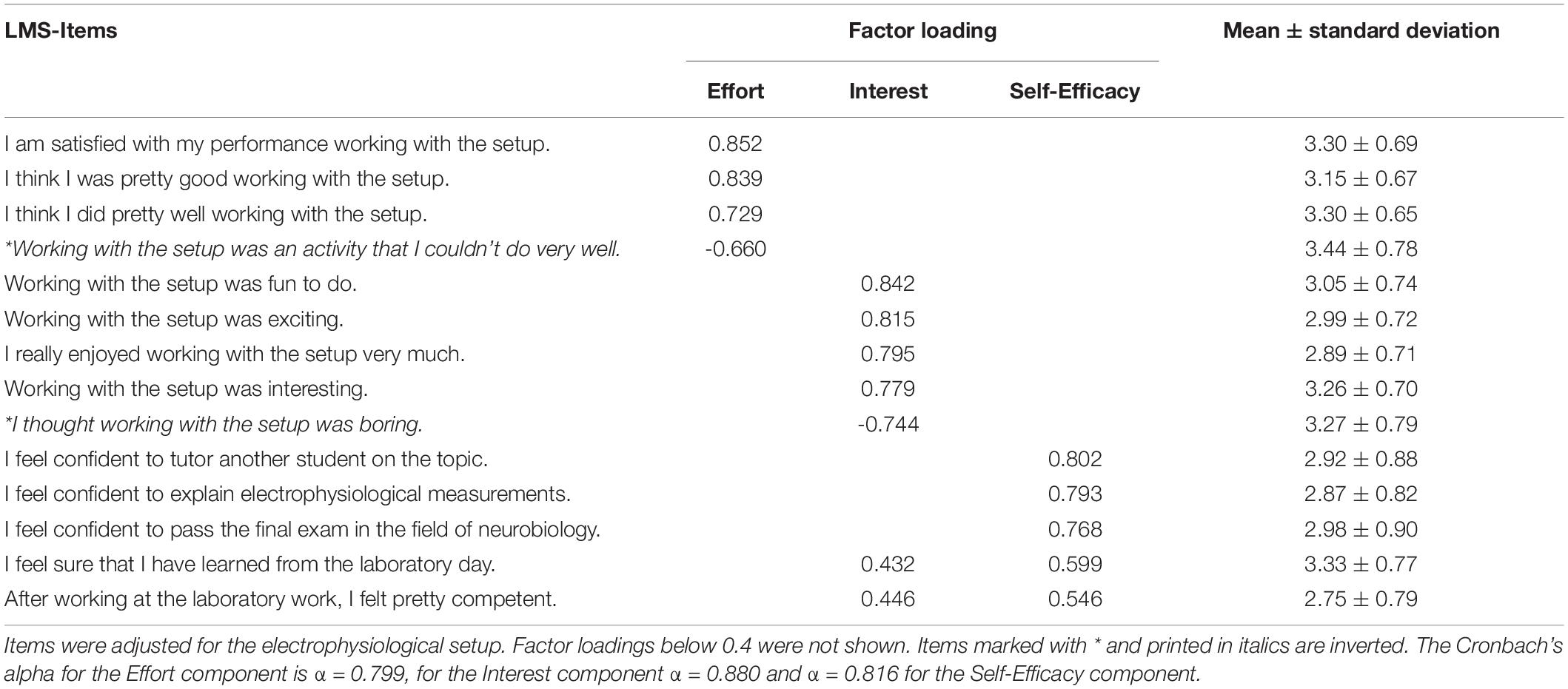
Table 1. Results of factor analysis with varimax rotation of the 14 items of the Lab Motivation Scale (LMS).
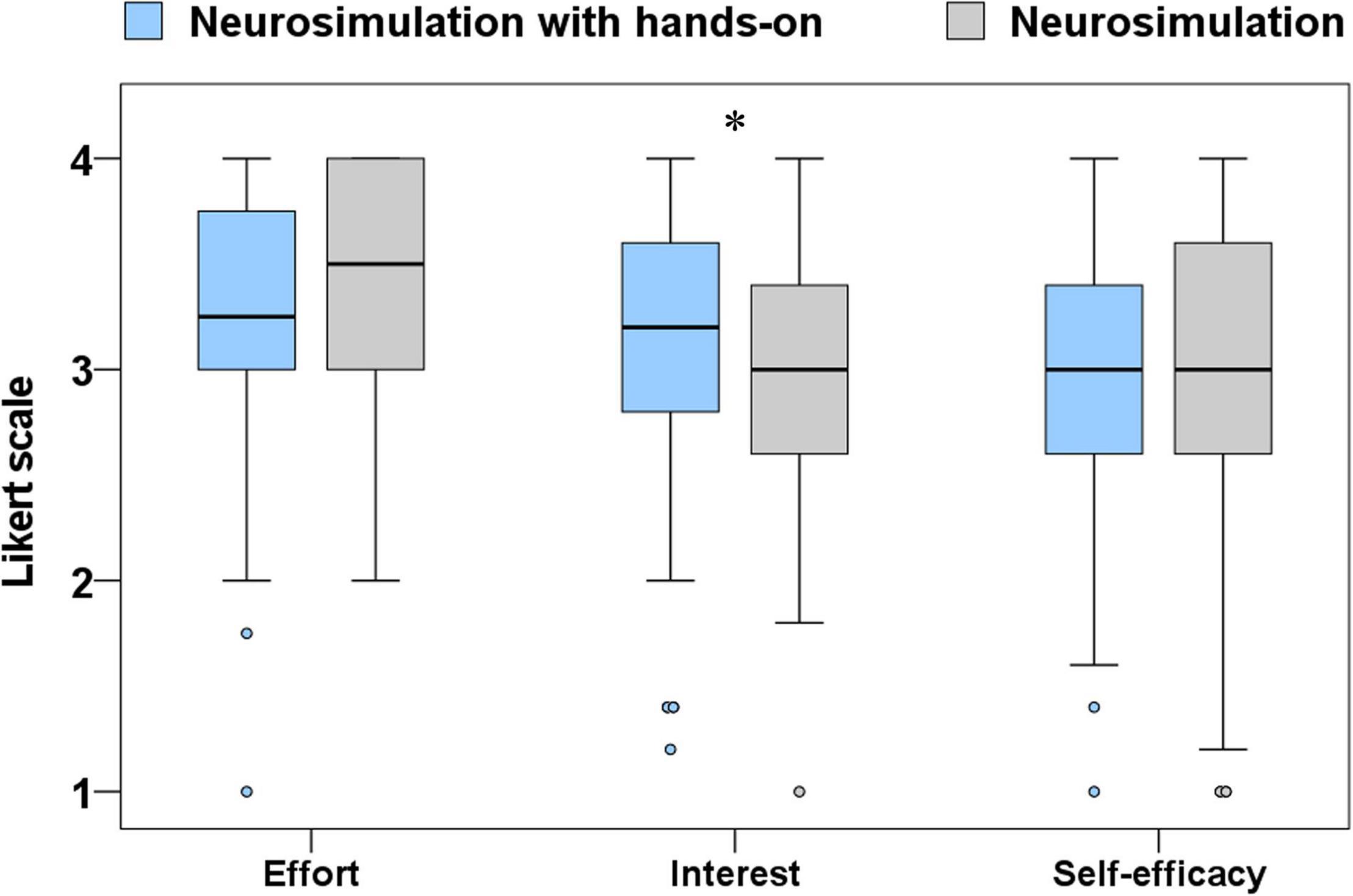
Figure 4. Comparison of the 3 components of Lab Motivation Scale (LMS) between the two test groups. Only significant effects were marked (* p < 0.05). Additional values for the boxplots can be found in the Appendix Table 2.
For the 18 items of the TAM, the Barlett test (p < 0.001) and the KMO (KMO = 0.889) also confirmed the applicability of a factor analysis. The factor analysis of the TAM items shows a division into three factors: PEOU, PU and PE. The three factors together explained 60.84% of the variance. All items used loaded on the appropriate component with factor loadings > 0.6 and the alpha scores of the components are in a good to very good range with α > 0.7, indicating the internal consistency of the scale. The results of the factor analysis and the calculated Cronbach alpha values for the three components are shown in Table 2. The comparison between the NS and NS-OH showed a significant difference between the groups in the component PE with a small effect size (p = 0.003; r = 0.21). No significant difference was found between the groups for PEOU (p = 0.761) and PU (p = 0.092; Figure 5). PEOU showed the highest mean scores compared to the other components of the TAM (MeanNS = 4.21; MeanNS–HO = 4.23). PU always had the highest standard deviation (SDNS = 0.92; SDNS–HO = 0.83).
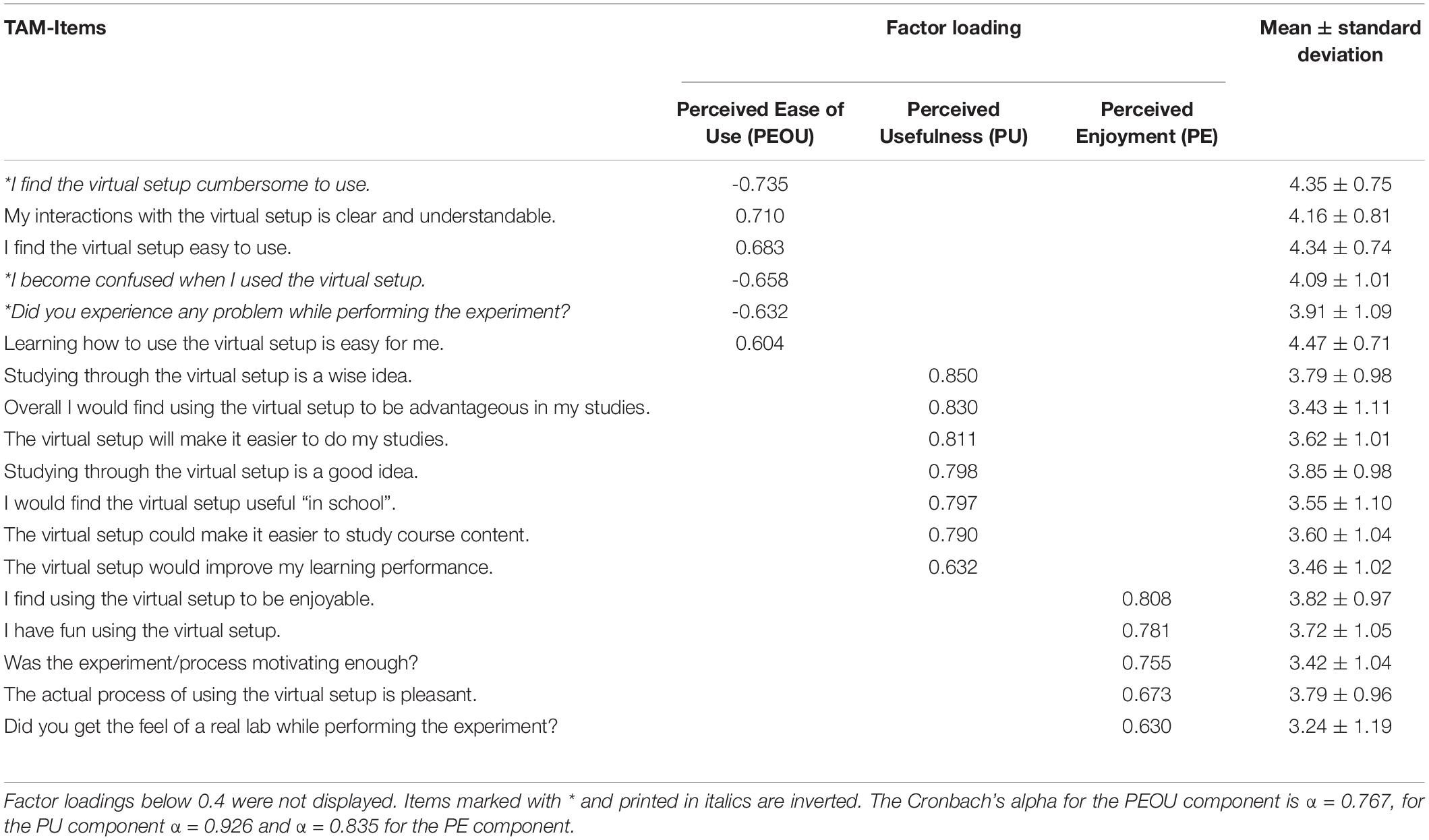
Table 2. Results of factor analysis of the 18 items of Technology Acceptance Model (TAM) with varimax rotation.
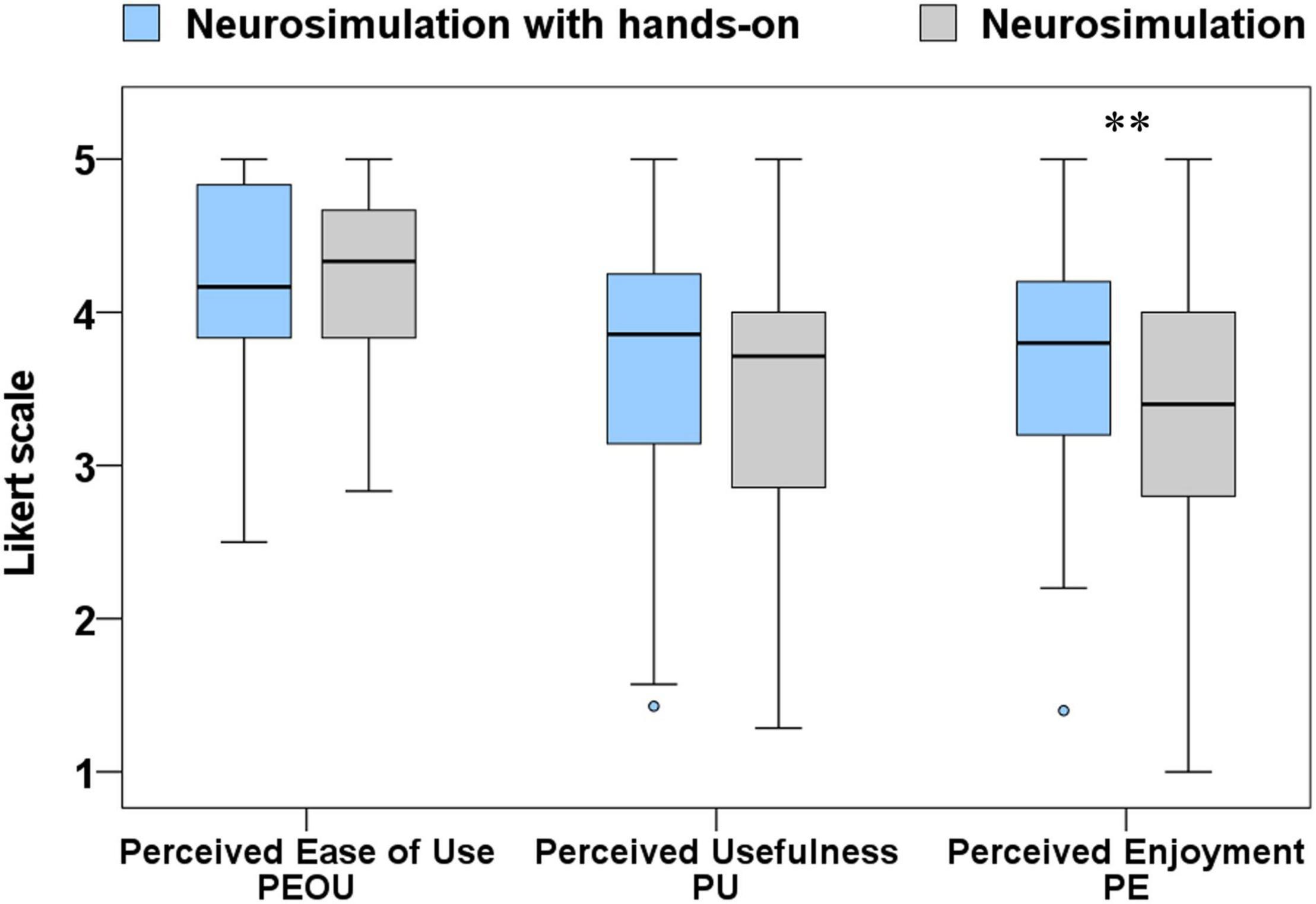
Figure 5. Comparison of the 3 components of Technology Acceptance Model (TAM) between the two test groups. Only significant effects were marked (** p < 0.01). Additional values for the boxplots can be found in the Appendix Table 2.
Discussion
It is well documented that there are widespread problems in students’ understanding of neurobiology (Cardozo, 2016). These problems can be addressed with experimental and novel teaching approaches (Marzullo and Gage, 2012; Shannon et al., 2014). For an approach to work, it is important that there is acceptance and motivation toward the tool as a basis for knowledge generation (Müller-Böling and Müller, 1986; Estriegana et al., 2019).
In the conducted study, the lab motivation and acceptance for simulation was measured after the laboratory day. A baseline survey before the laboratory day or the implementation of a control group was not possible in this study. The two instruments used directly ask about technology acceptance and motivation for the simulation performed. Since the students do not know the simulation before the event, they cannot make a statement about the acceptance or motivation for it. The same applies to a possible control group. Therefore, in this and comparable studies (Liu et al., 2010; Diwakar et al., 2014; Kilic et al., 2015) only a post-intervention survey was conducted. Even though this does not allow a before-after comparison, the results can still provide information about how high the technology acceptance and motivation for the simulation is and what influence the hands-on components might have in this context. In future studies, other more general instruments could be used to further assess motivation and technology acceptance, making the use of a control group or baseline analysis possible.
Lab Motivation
Two items of the Self-Efficacy component show cross-loadings on the Interest component that are higher than the common 0.4 threshold. These cross-loadings should not go ignored for future studies. For the analysis in this paper, the items were not excluded from the calculations and assigned to the SE component, because the factor loadings on this component are higher and in line with the results of the original scale by Dohn et al. (2016). The students rated the components Effort and Interest very highly, so that both experimental setups had an overall motivating effect. The motivational and interest-enhancing effect of student experiments is well known (Euler, 2005; Glowinski, 2007). The independent and science-oriented design of the experiments (whether it was NS or NS-HO) may have contributed to the reinforcement of these components. Corter et al. (2011) show in a comparative study of hands-on labs, remote labs, and simulations that working with real data particularly promotes motivation, what is given in our neurosimulation by the authentic measurements from a leech ganglion recorded by scientist.
Depending on the type of setup used, there is a significant difference for the Interest component, with the NS-HO group rating the component higher than the NS group. Students in the NS-HO group felt more “pleasure,” which corresponds to intrinsic motivation. Accordingly, situational interest is fostered by hands-on experience rather than pure simulation. It is assumed that hands-on activities have a positive effect on the development of interest (Bergin, 1999). The higher rating of interest can also be explained by a greater perception of authenticity of the electrophysiological setup elements. Similar findings are described in the work of Engeln (2004), Glowinski (2007), and Pawek (2009), who demonstrate a positive effect of authenticity on interest. A higher rating of the component would have been desirable, but induced sustained motivation can lead to a maintenance of learning activities, which can increase knowledge and skills regarding the content in the long term (Krapp, 2003; Pintrich, 2003).
The difference between the tested groups for the LMS turns out to be smaller than expected, the hands-on elements seem to play only a minor role for motivation and the sole neurosimulation has a motivating effect on the students as well. Holstermann et al. (2010) show for several hands-on activities that they frequently promote interest, but for individual hands-on activities no promoting influence could be proven.
Technology Acceptance
The TAM is a very widely spread tool that is repeatedly used in various studies. While the implementation of new variables to explain technology acceptance is frequently performed in the TAM (Park et al., 2007; Melas et al., 2011; Son et al., 2012; Cheung and Vogel, 2013), group comparisons as in this study are rather rare (Venkatesh and Morris, 2000). The factor analysis performed and alpha values confirm the applicability of the TAM items used in this study.
The high scores for all three components of the TAM in this study indicate that students have high acceptance for the NS, as well as for the NS-HO. Many studies on neurosimulations can demonstrate learner acceptance of computer-based experiments (e.g., Braun, 2003; Demir, 2006; Newman and Newman, 2013). In previous studies, PU and PEOU were often rated in a similar range (Hu et al., 1999; Lee and Kim, 2009; Tao et al., 2009; Pai and Huang, 2011). In this study, it appears that PEOU was rated higher on average, which indicates that the designed user interface of the neurosimulation as well as the handling of the hands-on elements were perceived user-friendly by the students, which is elementary for a successful application. PU, which in this context refers to increased learning success, has large variance, what corresponds to the assessment of the “self-efficacy in terms of learning success” of the LMS.
When comparing NS and NS-HO, PEOU and PU show no significant difference. PEOU and PU have been proven to be influencing factors on the acceptance for learning with technologies (Granić and Marangunić, 2019). The high scores of these components in both test groups indicate that technology acceptance is present. The PE component was scored higher in the NS-HO group than in the NS group. Even though it is a small effect size, it could be assumed that the hands-on elements may have had a particularly positive effect on this component. PE, Perceived Enjoyment, can be seen as an intrinsic influencing component (Davis et al., 1992). Thus, the results of the TAM are consistent with those of the LMS: in both models, the intrinsic component was rated higher by the NS-HO group than by the NS group. Therefore, it can be concluded that the hands-on elements have a positive influence on the intrinsic motivation and is therefore a positive extension to the pure simulation.
Although this study has made an important contribution to the application of a neurosimulation in a school context, there are still research gaps in this area, as a large number of studies regarding these teaching concepts have focused on university students rather than high school students (Barry, 1990; Schwab et al., 1995; Crisp, 2012; Wang et al., 2018). A particularly interesting further approach would be to examine teachers’ technology acceptance in more detail. PU and PEOU show a significant influence on teachers’ intention to use a technology (Scherer et al., 2019). Since teachers are often insecure in teaching neurobiological topics (MacNabb et al., 2006), examining and increasing their technology acceptance would be of particular benefit.
Conclusion
To address the difficulties in teaching and understanding electrophysiology, a computer-based neurosimulation was developed to provide students with experimental access to electrophysiological measurements. In order to investigate the potential effect of hands-on experiences, electrophysiological setup elements and a virtual preparation (circuit board) were added to the neurosimulation for some students. Small significant differences between the NS and the NS-HO were found especially in the intrinsic components: The students who worked on the NS-HO rated the simulation as more interesting and enjoyed their work more than the students in the NS group.
Overall, this research confirms a general positive perception of the neurosimulation. The students showed high motivation and technology acceptance for both the NS and the NS-HO, so that the use of neurosimulation on its own can also be considered beneficial. Nevertheless, neurosimulations should not be seen as a replacement of the actual research experiment, but rather represents a unique access opportunity in the context of student education that usually could not be offered to students in school.
We have made the neurosimulation (BrainTrack) freely accessible to all educators and students across the world through the url: https://virtualbrainlab.de.
Data Availability Statement
The raw data supporting the conclusions of this article will be made available by the authors, without undue reservation.
Ethics Statement
The studies involving human participants were reviewed and approved by the ethics committee of the science didactic institutes and departments (FB 13, 14, 15) of the Goethe University Frankfurt am Main. Written informed consent to participate in this study was provided by the participants’ legal guardian/next of kin.
Author Contributions
SF-Z and PD: conceptualization and methodology. SF-Z: data collection, validation, formal analysis, and investigation. SF-Z and MK: writing—original draft. SF-Z, PD, and MK: writing—review and editing and visualization. PD: funding acquisition and supervision. All authors contributed to the article and approved the submitted version.
Funding
This study was financially supported by the Hertie Foundation Frankfurt (project funding number P1180065).
Conflict of Interest
The authors declare that the research was conducted in the absence of any commercial or financial relationships that could be construed as a potential conflict of interest.
Publisher’s Note
All claims expressed in this article are solely those of the authors and do not necessarily represent those of their affiliated organizations, or those of the publisher, the editors and the reviewers. Any product that may be evaluated in this article, or claim that may be made by its manufacturer, is not guaranteed or endorsed by the publisher.
Acknowledgments
We would like to thank the Hertie Foundation Frankfurt, which contributed to the success of the project through its financial support and also with great personal effort.
References
Ajzen, I., and Fishbein, M. (1980). Understanding Attitudes and Predicting Social Behavior. Hoboken, NJ: Prentice-Hall.
Albarracín, A. L., Farfán, F. D., Coletti, M. A., Teruya, P. Y., and Felice, C. J. (2016). Electrophysiology for biomedical engineering students: a practical and theoretical course in animal electrocorticography. Adv. Physiol. Educ. 40, 402–409. doi: 10.1152/advan.00073.2015
Barry, P. H. (1990). Membrane potential simulation program for IBM-PC-compatible equipment for physiology and biology students. Am. J. Physiol. 259(6 Pt 3), S15–S23. doi: 10.1152/advances.1990.259.6.S15
Bergin, D. A. (1999). Influences on classroom interest. Educ. Psychol. 34, 87–98. doi: 10.1207/s15326985ep3402_2
Betz, A. (2018). Der Einfluss der Lernumgebung auf die (wahrgenommene) Authentizität der linguistischen Wissenschaftsvermittlung und das Situationale Interesse von Lernenden. Unterrichtswissenschaft 43, 261–278. doi: 10.1007/s42010-018-0021-0
Bish, J. P., and Schleidt, S. (2008). Effective use of computer simulations in an introductory neuroscience laboratory. J. Undergrad. Neurosci. Educ. 6, A64–A67.
Braun, H. A. (2003). “Virtual versus real laboratories in life science education: concepts and experiences,” in From Guinea Pig to Computer Mouse. Alternative Methods for a Progressive, Humane Education, eds N. Jukes and M. Chiuia (Leicester: InterNICHE), 81–87.
Brinson, J. R. (2015). Learning outcome achievement in non-traditional (virtual and remote) versus traditional (hands-on) laboratories: a review of the empirical research. Comp. Educ. 87, 218–237. doi: 10.1016/j.compedu.2015.07.003
Cardozo, D. (2016). An intuitive approach to understanding the resting membrane potential. Adv. Physiol. Educ. 40, 543–547. doi: 10.1152/advan.00049.2016
Carvalho, H. (2011). A group dynamic activity for learning the cardiac cycle and action potential. Adv. Physiol. Educ. 35, 312–313. doi: 10.1152/advan.00128.2010
Cheung, R., and Vogel, D. (2013). Predicting user acceptance of collaborative technologies: an extension of the technology acceptance model for e-learning. Comp. Educ. 63, 160–175. doi: 10.1016/j.compedu.2012.12.003
Chinn, C. A., and Malhotra, B. A. (2002). Epistemologically authentic inquiry in schools: a theoretical framework for evaluating inquiry tasks. Sci. Educ. 86, 175–218. doi: 10.1002/sce.10001
Corter, J. E., Esche, S. K., Chassapis, C., Ma, J., and Nickerson, J. V. (2011). Process and learning outcomes from remotely-operated, simulated, and hands-on student laboratories. Comp. Educ. 57, 2054–2067. doi: 10.1016/j.compedu.2011.04.009
Crisp, K. M. (2012). A structured-inquiry approach to teaching neurophysiology using computer simulation. J. Undergraduate Neurosci. Educ. 11, A132–A138.
Dabrowski, K. M., Castaño, D. J., and Tartar, J. L. (2013). Basic neuron model electrical equivalent circuit: an undergraduate laboratory exercise. J. Undergraduate Neurosci. Educ. 15, A49–A52.
Dagda, R. K., Thalhauser, R. M., Dagda, R., Marzullo, T. C., and Gage, G. J. (2013). Using crickets to introduce neurophysiology to early undergraduate students. J. Undergraduate Neurosci. Educ. 12, A66–A74.
Davis, F. D. (1985). A Technology Acceptance Model for Empirically Testing New End-user Information Systems: Theory and Results. thesis Ph. D, Cambridge, MA: Massachusetts Institute of Technology.
Davis, F. D. (1989). Perceived usefulness, perceived ease of use, and user acceptance of information technology. MIS Quarterly 13:319. doi: 10.2307/249008
Davis, F. D., Bagozzi, R. P., and Warshaw, P. R. (1989). User acceptance of computer technology: a comparison of two theoretical models. Manag. Sci. 35, 982–1003.
Davis, F. D., Bagozzi, R. P., and Warshaw, P. R. (1992). Extrinsic and intrinsic motivation to use computers in the workplace. J. Appl. Soc. Psychol. 22, 1111–1132. doi: 10.1111/j.1559-1816.1992.tb00945.x
de Jong, T., Linn, M. C., and Zacharia, Z. C. (2013). Physical and virtual laboratories in science and engineering education. Science 340, 305–308. doi: 10.1126/science.1230579
Demir, S. S. (2006). Interactive cell modeling web-resource, iCell, as a simulation-based teaching and learning tool to supplement electrophysiology education. Ann. Biomed. Eng. 34, 1077–1087. doi: 10.1007/s10439-006-9138-0
Dewhurst, D. (2006). Computer-based alternatives in higher education - past, present and future. Alternatives Animal Exp. 23, 197–201.
Diwakar, S., Parasuram, H., Medini, C., Raman, R., Nedungadi, P., Wiertelak, E., et al. (2014). Complementing neurophysiology education for developing countries via cost-effective virtual labs: case studies and classroom scenarios. J. Undergrad. Neurosci. Educ. 12, A130–A139.
Dohn, N. B., Fago, A., Overgaard, J., Madsen, P. T., and Malte, H. (2016). Students’ motivation toward laboratory work in physiology teaching. Adv. Physiol. Educ. 40, 313–318. doi: 10.1152/advan.00029.2016
Engeln, K. (2004). Schülerlabors. Authentische, Aktivierende Lernumgebungen als Möglichkeit, Interesse an Naturwissenschaften und Technik zu Wecken. Dissertation, Kiel: Christian-Albrechts-University.
Estriegana, R., Medina-Merodio, J.-A., and Barchino, R. (2019). Student acceptance of virtual laboratory and practical work: an extension of the technology acceptance model. Comp. Educ. 135, 1–14. doi: 10.1016/j.compedu.2019.02.010
Euler, M. (2005). Schülerinnen und schüler als forscher: informelles lernen im schülerlabor. Naturwissenschaften Im Unterricht. Physik 16, 4–12.
Field, A. (2009). Discovering Statistics Using SPSS: (and sex and drugs and rock ‘n’ roll). Newcastle upon Tyne: Sage.
Fritz, C. O., Morris, P. E., and Richler, J. J. (2012). Effect size estimates: current use, calculations, and interpretation. J. Exp. Psychol. General 141, 2–18. doi: 10.1037/a0024338
Gage, G. J. (2019). The case for neuroscience research in the classroom. Neuron 102, 914–917. doi: 10.1016/j.neuron.2019.04.007
Glowinski, I. (2007). Schülerlabore im Themenbereich Molekularbiologie als Interesse fördernde Lernumgebung. Kiel: Christian-Albrechts-University.
Granić, A., and Marangunić, N. (2019). Technology acceptance model in educational context: a systematic literature review. Br. J. Educ. Technol. 50, 2572–2593. doi: 10.1111/bjet.12864
Griff, E. (2018). The leaky neuron: understanding synaptic integration using an analogy involving leaky cups. CourseSource 5, 1–11. doi: 10.24918/cs.2018.11
Grisham, W. (2009). Modular digital course in undergraduate neuroscience education (MDCUNE): a website offering free digital tools for neuroscience educators. J. Undergraduate Neurosci. Educ. 8, A26–A31.
Hofstein, A., and Lunetta, V. N. (2004). The laboratory in science education: foundations for the twenty-first century. Sci. Educ. 88, 28–54. doi: 10.1002/sce.10106
Holloway, S. R. (2013). Three colossal neurons: a new approach to an old classroom demonstration. J. Undergrad Neurosci. Educ. 12, A1–A3.
Holstermann, N., Grube, D., and Bögeholz, S. (2010). Hands-on activities and their influence on s interest. Res. Sci. Educ. 40, 743–757. doi: 10.1007/s11165-009-9142-0
Hu, P. J., Chau, P. Y. K., Sheng, O. R. L., and Tam, K. Y. (1999). Examining the technology acceptance model using physician acceptance of telemedicine technology. J. Manag. Inform. Systems 16, 91–112. doi: 10.1080/07421222.1999.11518247
Kaisarevic, S. N., Andric, S. A., and Kostic, T. S. (2017). Teaching animal physiology: a 12-year experience transitioning from a classical to interactive approach with continual assessment and computer alternatives. Adv. Physiol. Educ. 41, 405–414. doi: 10.1152/advan.00132.2016
Keen-Rhinehart, E., Eisen, A., Eaton, D., and McCormack, K. (2009). Interactive methods for teaching action potentials, an example of teaching innovation from neuroscience postdoctoral fellows in the fellowships in research and science teaching (FIRST) program. J. Undergraduate Neurosci. Educ. 7, A74–A79.
Kilic, E., Güler, Ç, Çelik, H. E., and Tatli, C. (2015). Learning with interactive whiteboards: determining the factors on promoting interactive whiteboards to students by technology acceptance model. Interact. Technol. Smart Educ. 12, 285–297. doi: 10.1108/ITSE-05-2015-0011
Knight, A. (2007). Humane teaching methods prove efficacious within veterinary and other biomedical education. Alternatives Animal Testing Exp. 14, 213–220.
Krapp, A. (2003). Die bedeutung der lernmotivation für die optimierung des schulischen bildungssystems. Politische Stud. 54, 91–105.
Krapp, A. (2007). An educational–psychological conceptualisation of interest. Int. J. Educ. Vocational Guidance 7, 5–21. doi: 10.1007/s10775-007-9113-9
Krontiris-Litowitz, J. (2003). Using manipulatives to improve learning in the undergraduate neurophysiology curriculum. Adv. Physiol. Educ. 27, 109–119. doi: 10.1152/advan.00042.2002
Le Guennec, J.-Y., Vandier, C., and Bedfer, G. (2002). Simple experiments to understand the ionic origins and characteristics of the ventricular cardiac action potential. Adv. Physiol. Educ. 26, 185–194. doi: 10.1152/advan.00061.2001
Lee, M. K. O., Cheung, C. M. K., and Chen, Z. (2005). Acceptance of internet-based learning medium: the role of extrinsic and intrinsic motivation. Inform. Manag. 428, 1095–1104. doi: 10.1016/j.im.2003.10.007
Lee, S., and Kim, B. G. (2009). Factors affecting the usage of intranet: a confirmatory study. Comp. Hum. Behav. 25, 191–201. doi: 10.1016/j.chb.2008.08.007
Lewis, D. I. (2014). The Pedagogical Benefits and Pitfalls of Virtual Tools for Teaching and Learning Laboratory Practices in the Biological Sciences. Heslington: The Higher Education Academy.
Liaw, S.-S., and Huang, H.-M. (2003). An investigation of user attitudes toward search engines as an information retrieval tool. Comp. Hum. Behav. 19, 751–765.
Liu, I.-F., Chen, M. C., Sun, Y. S., Wible, D., and Kuo, C.-H. (2010). Extending the TAM model to explore the factors that affect intention to use an online learning community. Comp. Educ. 54, 600–610.
Ma, J., and Nickerson, J. V. (2006). Hands-on, simulated, and remote laboratories: a comparative literature review. ACM Comp. Surveys 38, 1–24.
MacNabb, C., Schmitt, L., Michlin, M., Harris, I., Thomas, L., Chittendon, D., et al. (2006). Neuroscience in middle schools: a professional development and resource program that models inquiry-based strategies and engages teachers in classroom implementation. CBE Life Sci. Educ. 5, 144–157. doi: 10.1187/cbe.05-08-0109
Manalis, R. S., and Hastings, L. (1974). Electrical gradients across an ion-exchange membrane in student’s artificial cell. J. Appl. Physiol. 36, 769–770. doi: 10.1152/jappl.1974.36.6.769
Marzullo, T. C., and Gage, G. J. (2012). The SpikerBox: a low cost, open-source bioamplifier for increasing public participation in neuroscience inquiry. PLoS One 7:e30837. doi: 10.1371/journal.pone.0030837
Melas, C. D., Zampetakis, L. A., Dimopoulou, A., and Moustakis, V. (2011). Modeling the acceptance of clinical information systems among hospital medical staff: an extended TAM model. J. Biomed. Inform. 44, 553–564. doi: 10.1016/j.jbi.2011.01.009
Meuth, P., Meuth, S. G., and Jacobi, D. (2005). Get the rhythm: modeling neuronal activity. J. Undergraduate Neurosci. Educ. 4, A1–A11.
Michael, J. (2006). Where’s the evidence that active learning works? Adv. Physiol. Educ. 30, 159–167.
Milanick, M. (2009). Changes of membrane potential demonstrated by changes in solution color. Adv. Physiol. Educ. 33:230. doi: 10.1152/advan.00052.2009
Montagna, E., Azevedo, A. M. S., de, Romano, C., and Ranvaud, R. (2010). What is transmitted in “synaptic transmission”? Adv. Physiol. Educ. 34, 115–116. doi: 10.1152/advan.00006.2010
Müller-Böling, D., and Müller, M. (1986). Akzeptanzfaktoren der Bürokommunikation. Munich: Oldenbourg.
Newman, M. H., and Newman, E. A. (2013). MetaNeuron: a free neuron simulation program for teaching cellular neurophysiology. J. Undergraduate Neurosci. Educ. 12, A11–A17.
Pai, F.-Y., and Huang, K.-I. (2011). Applying the technology acceptance model to the introduction of healthcare information systems. Technol. Forecasting Soc. Change 78, 650–660. doi: 10.1016/j.techfore.2010.11.007
Park, N., Lee, K. M., and Cheong, P. H. (2007). University instructors’ acceptance of electronic courseware: an application of the technology acceptance model. J. Computer-Med. Commun. 13, 163–186. doi: 10.1111/j.1083-6101.2007.00391.x
Park, S. Y. (2009). An analysis of the technology acceptance model in understanding university students’ behavioral intention to use e-learning. Educ. Technol. Soc. 12, 150–162.
Pawek, C. (2009). Schülerlabore als Interessefördernde außerschulische Lernumgebungen für Schülerinnen und Schüler aus der Mittel- und Oberstufe. Dissertation, Kiel: Christian-Albrechts-University.
Pintrich, P. R. (2003). A Motivational science perspective on the role of student motivation in learning and teaching contexts. J. Educ. Psychol. 95, 667–686. doi: 10.1037/0022-0663.95.4.667
Procopio, J. (1994). Hydraulic analogs as teaching tools for bioelectric potentials. Am. J. Physiol. 267(6 Pt 3), S65–S76. doi: 10.1152/advances.1994.267.6.S65
Quiroga, M. D. M., and Choate, J. K. (2019). A virtual experiment improved students’ understanding of physiological experimental processes ahead of a live inquiry-based practical class. Adv. Physiol. Educ. 43, 495–503. doi: 10.1152/advan.00050.2019
Ryan, R. M., and Deci, E. L. (2000). Intrinsic and extrinsic motivations: classic definitions and new directions. Contemporary Educ. Psychol. 25, 54–67. doi: 10.1006/ceps.1999.1020
Scherer, R., Siddiq, F., and Tondeur, J. (2019). The technology acceptance model (TAM): a meta-analytic structural equation modeling approach to explaining teachers’ adoption of digital technology in education. Comp. Educ. 128, 13–35. doi: 10.1016/j.compedu.2018.09.009
Schiefele, U., and Schaffner, E. (2015). “Motivation,” in Springer-Lehrbuch. Pädagogische Psychologie, 2nd Edn, eds E. Wild and J. Möller (Berlin: Springer).
Schwab, A., Kersting, U., Oberleithner, H., and Silbernagl, S. (1995). Xenopus laevis oocyte: using living cells to teach the theory of cell membrane potential. Am. J. Physiol. 268(6 Pt 3), S26–S31. doi: 10.1152/advances.1995.268.6.S26
Serenko, A. (2008). A model of user adoption of interface agents for email notification. Interact. Comp. 20, 461–472.
Shannon, K. M., Gage, G. J., Jankovic, A., Wilson, W. J., and Marzullo, T. C. (2014). Portable conduction velocity experiments using earthworms for the college and high school neuroscience teaching laboratory. Adv. Physiol. Educ. 38, 62–70. doi: 10.1152/advan.00088.2013
Sheorey, T., and Gupta, V. K. (2011). Effective virtual laboratory content generation and accessibility for enhanced skill development through ICT. Proc. Comp. Sci. Inform. 12, 33–39.
Shlyonsky, V. (2013). Ion permeability of artificial membranes evaluated by diffusion potential and electrical resistance measurements. Adv. Physiol. Educ. 37, 392–400. doi: 10.1152/advan.00068.2013
Silverthorn, D. U. (2002). Uncovering misconceptions about the resting membrane potential. Adv. Physiol. Educ. 26, 69–71.
Son, H., Park, Y., Kim, C., and Chou, J.-S. (2012). Toward an understanding of construction professionals’ acceptance of mobile computing devices in South Korea: an extension of the technology acceptance model. Automation Construct. 28, 82–90.
Stuart, A. E. (2009). Teaching neurophysiology to undergraduates using neurons in action. J. Undergraduate Neurosci. Educ. 8, A32–A36.
Šumak, B., Herièko, M., and Pušnik, M. (2011). A meta-analysis of e-learning technology acceptance: the role of user types and e-learning technology types. Comp. Hum. Behav. 27, 2067–2077.
Taherdoost, H. (2018). A review of technology acceptance and adoption models and theories. Proc. Manufacturing 22, 960–967.
Tao, Y.-H., Cheng, C.-J., and Sun, S.-Y. (2009). What influences college students to continue using business simulation games? the Taiwan experience. Comp. Educ. 53, 929–939.
Venkatesh, V., and Bala, H. (2008). Technology acceptance model 3 and a research agenda on interventions. Decision Sci. 39, 273–315.
Venkatesh, V., and Morris, M. G. (2000). Why don’t men ever stop to ask for directions? gender, social influence, and their role in technology acceptance and usage behavior. MIS Quarterly 24:115.
Wang, R., Liu, C., and Ma, T. (2018). Evaluation of a virtual neurophysiology laboratory as a new pedagogical tool for medical undergraduate students in China. Adv. Physiol. Educ. 42, 704–710. doi: 10.1152/advan.00088.2018
Yi, M. Y., and Hwang, Y. (2003). Predicting the use of web-based information systems: self-efficacy, enjoyment, learning goal orientation, and the technology acceptance model. Int. J. Human-Computer Stud. 59, 431–449.
Appendix
Keywords: hands-on elements, lab motivation scale (LMS), technology acceptance model (TAM), digital student lab, neurobiology, neurosimulation, teaching tool, learning technology
Citation: Formella-Zimmermann S, Kleespies MW and Dierkes PW (2022) Motivation and Technology Acceptance in a Neuroscience Student Lab—An Empirical Comparison Between Virtual Experiments With and Without Hands-on Elements. Front. Educ. 7:817598. doi: 10.3389/feduc.2022.817598
Received: 18 November 2021; Accepted: 04 February 2022;
Published: 25 February 2022.
Edited by:
Mohammed Saqr, University of Eastern Finland, FinlandReviewed by:
Chia-Lin Tsai, University of Northern Colorado, United StatesTzung-Jin Lin, National Taiwan Normal University, Taiwan
Copyright © 2022 Formella-Zimmermann, Kleespies and Dierkes. This is an open-access article distributed under the terms of the Creative Commons Attribution License (CC BY). The use, distribution or reproduction in other forums is permitted, provided the original author(s) and the copyright owner(s) are credited and that the original publication in this journal is cited, in accordance with accepted academic practice. No use, distribution or reproduction is permitted which does not comply with these terms.
*Correspondence: Sandra Formella-Zimmermann, cy56aW1tZXJtYW5uQGVtLnVuaS1mcmFua2Z1cnQuZGU=