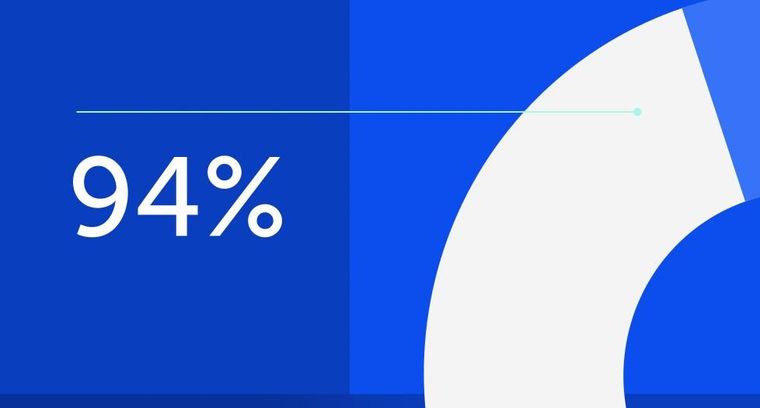
94% of researchers rate our articles as excellent or good
Learn more about the work of our research integrity team to safeguard the quality of each article we publish.
Find out more
ORIGINAL RESEARCH article
Front. Educ., 17 May 2022
Sec. Language, Culture and Diversity
Volume 7 - 2022 | https://doi.org/10.3389/feduc.2022.758736
We examined the linguistic features of texts in twenty-nine picture books used in an early English as a Foreign Language program in China. We used the software CLAN to automatically extract indices of linguistic complexity that are typically used to analyze child-directed speech and tested if these indices aligned with expert judgments on the books’ appropriate grade level (Kindergarten-1 through Kindergarten-3). Of the eleven characteristics investigated, seven showed significant between-level differences with moderate effect sizes. Across all levels, vocabulary complexity (i.e., frequency of types, frequency of tokens, and vocabulary diversity) and syntactic complexity (i.e., number of verbs per utterance, number of Developmental-Sentence-Scoring-eligible utterances, mean length of utterance in morphemes, and total number of non-zero morphemes) increased, also in alignment with experts’ judgments. Indices of child language development can thus be used to estimate text complexity in picture books. The study contributes to a better understanding of children’s picture book difficulty and has methodological implications for investigating text characteristics for very young children learning English as a foreign language.
The term “children’s picture books” generally refers to books in which texts and illustrations complete one another to tell a story, usually targeting beginning readers (Al Khaiyali, 2014). Picture books are widely used in at-home reading and in early childhood classrooms to promote early language competence. With a wide range of topics, easy-to-understand illustrations and interesting contents, picture books can create a rich, context-based learning environment that encourages more teacher-child interaction and leads to deeper levels of conversation (Massey, 2004; Wasik et al., 2006). Mounting evidence suggests that training kindergarten teachers on book reading, such as choosing appropriate books or showing connections between the book and children’s own experiences, can help teachers promote children’s expressive vocabulary (Biemiller and Boote, 2006; Hindman et al., 2012), phonological awareness (Elmonayer, 2013), narrative skills (Zevenbergen et al., 2003) and social-emotional development such as formal schooling readiness skills (Cutler and Slicker, 2020).
With the development of readers’ capacities, texts should become more complex (Snow, 2002). Researchers have argued that reading materials can provide readers with comprehensible input and facilitate learning of a language when they are based on the concept of the zone of proximal development proposed by Vygotsky (Berendes et al., 2017). That is, what the readers can do with some adult or expert guidance. Conversely, inappropriate reading materials that demand too much or too little can negatively affect the reader’s experience; they may be either too boring or too difficult and therefore lead to frustration (Rog and Burton, 2001). Experiences of failure at the very beginning of a reading stage can lead to later reading difficulties (Snow et al., 1998) or a reluctance to read independently (Torgesen, 2004).
Therefore, matching appropriate reading books to different ages and school grades has attracted the attention of numerous researchers (Rog and Burton, 2001; Sierschynski et al., 2014; Denning et al., 2016) and educators. According to Hiebert (2009), text difficulty is usually determined using either expert judgment or readability formulas. In addition, the criteria upon which picture books are leveled are unclear and vary from publisher to publisher. Nor are the characteristics of texts at each level described (Rog and Burton, 2001).
Shared book reading during the preschool years not only connects to young children’s literacy development but also future school success (Wasik et al., 2006; Beauchat et al., 2009). Zevenbergen and Whitehurst (2003) found that dialogic reading of picture books had substantial positive effects on preschool-aged childrens’ language development and literacy skills. Similarly, picture book reading has been found to improve vocabulary and syntax development not only in first language (L1) contexts, but also in English as foreign language (EFL) contexts. Hui et al. (2020) found that picture book reading led to significant vocabulary and syntax development (in English) in young Chinese EFL children. Picture books have also been found to be useful materials for explicit reading comprehension, which is an effective language teaching method.
Although the matching of linguistic complexity and grade levels has been studied extensively (e.g., Rog and Burton, 2001; Mesmer et al., 2012; Berendes et al., 2017; Holster et al., 2017; Jin et al., 2020), relatively few studies have targeted Chinese EFL students and even fewer targeted very young EFL children’s reading materials. Jin et al. (2020) analyzed a large corpus of teaching materials for Chinese EFL students from Grade 1 to 12. They found that five syntactic complexity measures generated by the Syntactic Complexity Analyzer (SCA, Lu, 2010) were predictive of grade levels. However, it is unclear whether these linguistic indicators are also applicable to the prediction of the difficulty level of children’s picture books.
The objective of this manuscript is to conduct an analysis of texts in children’s picture books to examine the characteristics of the texts and their relationship with holistic expert judgments of difficulty.
When speaking about grading reading materials, a distinction should first be drawn between the concepts of “difficulty” and “complexity.” Using the terms synonymously conflates causes and effects (Mesmer et al., 2012). “Difficulty” emphasizes the role of the reader, pointing to their perceptions and judgments of the text, whereas “complexity” better represents the nature of texts, such as sentence structure and vocabulary (Sierschynski et al., 2014). The same text can be of different levels of difficulty due to a number of reasons such as readers’ varying reading experience, prior learning, or knowledge of concepts. In such cases, text difficulty is usually measured qualitatively since the method recognizes books as an integral structure. Studies that focus on text complexity, on the other hand, investigate characteristics of the text itself (e.g., syntactic complexity and lexical coverage or density) and commonly resort to computerized systems. The two concepts are intertwined in the study of leveled reading, but the current study analyzes “text complexity,” since the aim is to investigate the relationship between linguistic characteristics of texts and their recommended grade level.
Due to advances in technology, early analyses of text complexity that describe in detail text features that would likely affect text comprehensibility or readability have now been replaced by quantitative approaches (Hiebert and Pearson, 2014). Computational tools, such as Lexile (Smith, 1989) and ATOS (School Renaissance Institute, 2000), differ in terms of the indices used to compute reading complexity but mainly rely on word and sentence lengths and numbers of modifiers to measure syntactic complexity (Hiebert, 2002), and word familiarity or word difficulty to assess semantic complexity. The user-friendliness of these tools makes them popular among many parents and teachers. However, because these tools take only a few text features into account, some researchers have voiced the concern that these tools tend to underestimate text comprehensibility (Crossley et al., 2011).
Furthermore, other disciplines such as psycholinguistics have meanwhile made progress in better understanding the cognitive process of reading. Their insights are used in newer readability analysis approaches and include additional factors that affect comprehensibility such as textual cohesion. Typical examples are Coh-Metrix (Graesser et al., 2004) and TextEvaluator (Sheehan et al., 2014). By including more variables that go beyond surface features, these new systems permit a more thorough and productive analysis of texts (Hiebert and Pearson, 2014). However, none of these metrics specifically relate to or provide explanations of what constitutes text complexity in early grades (Fitzgerald et al., 2015). Different from more experienced readers like teenagers and adults, young children are still in the process of acquiring language, and what is complex and difficult for young children is likely to be different.
In this manuscript, we explore the idea of using linguistic indices that have been specifically developed for analyzing children’s language development as a tool for assessing text complexity in books aimed at young children. Specifically, we are using the software CLAN (Computerized Language Analysis; MacWhinney, 2000) to automatically extract several linguistic measures that are used in language acquisition research. In the next section, we explain why CLAN is particularly suited for our purposes, and provide an overview of the most important measures that can be extracted using CLAN.
Computerized Language Analysis is a powerful annotation tool, and a free and fully automatic analyzer for written texts. It uses a specific transcription and coding system (CHAT, Codes for the Human Analysis of Transcripts) that makes it possible to analyze playful words and neologisms that cannot be read by other text analyzers, such as “slapity,” “clickity,” “tippity,” and “creepity,” which are often found in children’s books (in this case “Dancing Feet!”). More importantly, it can be used to automatically analyze a broad range of linguistic features to calculate indicators of children’s early language development that child language researchers have developed over the years.
These indicators can be roughly put into two groups: those pertaining to the syntactic complexity of utterances, and those pertaining to the complexity of children’s vocabulary. Already in, Nice (1925) suggested that “average sentence length may well prove to be the most important single criterion for judging a child’s progress in the attainment of adult language” (p. 378). In 1973, Brown introduced the mean length of utterance (MLU) as a measure. MLU is used for longitudinal (intra-individual) and cross-sectional (inter-individual) comparisons in monolingual language acquisition, for cross-linguistic studies, for comparison of bilinguals’ early language development (Marchman et al., 2004; Meisel, 2011; Thordardottir, 2011; Hoff et al., 2014). There are two MLU measurements: MLUw refers to the mean number of words in a child’s utterances, whereas MLUm refers to the mean number of morphemes in a child’s utterances. Morphemes are the smallest units in language that carry a meaning. For example, the sentence “He sings loudly” has five morphemes (he, sing, -s, loud, -ly), but only three words. Both MLUw and MLUm are reliable estimates of children’s language development (Rice et al., 2010; Wieczorek, 2010) and they are highly correlated (Hickey, 1991; Parker and Brorson, 2005). It has also been argued that they are indicators of different aspects of language development. Specifically, it has been suggested that MLUw provides information about the lexicon, whereas MLUm is an indicator of morphosyntactic development (Wieczorek, 2010). Further measures for syntactic complexity available in CLAN are the Number of Verbs per Utterance (Verbs_Utts), the Number of DSS-eligible Utterance (DSS_Utts; see below for a definition) and the Total Number of Non-zero Morphemes (Total_non_zero_mors).
The Number of Verbs per Utterance is seen as an indicator of language development, because nouns are considered to be easier to learn (Ellis and Beaton, 1993). Conversely, if a text contains more verbs, it would be expected to be more difficult. The indicator is calculated by dividing the number of verbs by the total number of sentences in text.
DSS stands for Developmental Sentence Score, and is based on a developmental scale of syntax acquisition (Lee and Canter, 1971). A child’s ability to formulate “advanced” sentences is estimated by assigning weighted scores to certain elements (e.g., pronouns, conjunctions, WH-questions). Elements that typically occur later in children’s language development are given higher scores. For example, a score of 1 is given for the use of pronouns like it, this, and that, but a score of 5 for pronouns like anything, every or everybody. In a similar vein, the Total Number of Zero Morphemes counts the number of Brown (1973) 14 grammatical morphemes, which include things like regular past tense (e.g., he jump-ed) or plural -s (e.g., car-s). In other words, it shows how many “advanced” grammatical morphemes occur in a child’s speech (or a text).
Next to syntactic complexity, vocabulary diversity is considered a crucial measure of child language development (MacWhinney, 2000), and has been widely studied in both first and second language development (Malvern et al., 2004). It is also considered to be an important measurement of language input for young children (Huttenlocher et al., 2010; Montag, 2019), and therefore a strong candidate for an indicator of text complexity in books aimed at young children. Widely used measures include the Number of Different Words (NDW), Type-Token Ratio (TTR) and Diversity (D or VocD). NDW is the most direct measurement of lexical diversity due to its simple calculation (Miller, 1991). TTR refers to the number of unique words (types) to the overall number of words (tokens). TTR is used to examine the extent to which the vocabulary use is repetitive and thus reflects changes over age (Klee, 1992). The closer the TTR is to 1, the more lexical variety a segment of speech (or a text) has; the smaller the TTR is, the more repetitive a text is. D (Malvern and Richards, 1997), or VocD in CLAN, is similar to both NDW and TTR, but is said to be less affected by differences in sample size than NDW and TTR (McKee et al., 2000). The higher VocD, the more lexical variety a text has. In addition to these indices, CLAN also allows extracting other basic summary measures, such as the total number of utterances or the total number of different word types.
Young EFL children in China are a highly heterogeneous group and there is large individual variation in these children’s L2-English development. Sun et al. (2015) documented the development of four Chinese preschoolers (age three) in an English classroom and found that they progressed from non-verbal to verbal phases at different times, and that their progress was related to their temperament characteristics. English is officially taught as a school subject starting from 3rd grade in China. Before 3rd grade, English instruction is informal and organized in homes and private kindergartens (Chen et al., 2020). Sun et al. (2016) investigated 71 kindergarteners learning English in China and found that the individual variation in their English proficiency measures was largely explained by external factors such as English use at home or the quantity of instruction at school.
In this study, we automatically extract well-established measures of children’s language development from young children’s picture books in order to determine if these measures align with experts’ rating of the books’ difficulty. More specifically, we are focusing our analysis on picture books for children learning English or learning through English in kindergartens in China. Kindergartens in China means full-day programs (government-licensed, private, or community-based) providing childcare and educational preparation for children from 3 to 6 years of age. To be specific, the study aims to address the following question:
How predictive are automatically generated linguistic indicators of expert leveling of picture books?
This study uses materials used in a kindergarten EFL curriculum that has been implemented across 25 private kindergartens in a metropolitan city in China. This curriculum is designed around 30 picture books that are either classic (i.e., The Very Hungry Caterpillar) and/or widely available (i.e., Brown Bear Brown Bear What Do You See) and/or closely related to children’s immediate life experience (i.e., The Last Day of Kindergarten). These picture books were also chosen to help children gain rhyme awareness or other types of phonological awareness, as phonics instruction is an important part of the kindergarten curriculum.
The books were assigned to three levels by 30 experienced early childhood educators. These experts included professors of early childhood education, researchers at various institutions, officers at early reading-related non-governmental organizations (NGOs), and postgraduate students in Early Childhood Education (ECE) programs at universities in China and the United States. These experts had experience in working with young Chinese children (0–6 years old) and their families. Twenty percent (20%) of the experts had more than 10 years of ECE work experience; 50% of the experts had than 5–10 years of ECE work experience; and 30% of the experts had 3–5 years of ECE work experience. Among them, 60% had direct teaching experience in preschools and kindergartens, 20% worked at universities, and 20% of the experts worked with young children’s parents and families.
The selection criteria for the 30 books included appropriateness (i.e., the book is written and illustrated for children aged 3–6 years old and is connected with children’s life experience), literary quality (i.e., the book is written using language that is easy to understand for children and encourages comprehension and creativity), interactivity (i.e., the book has playful elements that allow interaction between children and the book and between children and teachers), artistic quality (i.e., the book uses symbolic messages that connect the story and children’s cognitive and emotional growth), and availability (i.e., the books need to be imported and easily purchased from the Chinese book market). Ten books were categorized as first grade in kindergarten (K1) (e.g., Brown Bear, Brown Bear What Do You See), ten as second grade (K2) (e.g., Pete the Cat-I Love My White Shoes), and ten as third grade (K3) (e.g., The Day the Crayons Quit). When categorizing the books into three grade levels, experts used holistic assessment and considered a range of other factors. These included book length, vocabulary familiarity, sentence structure, and illustration support, and a balanced mix of the content area support (i.e., health, language, science, and art). The educators read the recommended reading ages of each of these books, but this information did not decide the category. Judges were more concerned about the books’ appropriateness for each grade level according to their experience. For example, The Going to Bed Book was most appropriate for K1 because daily routine is an emphasis in the first year of kindergarten.
The expert judges were provided with Fountas and Pinnell Guided Reading Text Level Descriptions for level A, B, and C (Fountas and Pinnell, 1996) when leveling the books1. Characteristics for level A include “simple factual texts, animal fantasy and realistic fiction; text and concepts highly supported by pictures; and almost all vocabulary familiar to children.” Characteristics for level B include “two or more lines of text on each page; repeating language patterns; very familiar themes and ideas.” Characteristics for level C include “two to five lines of text on each page, many sentences with prepositional phrases and adjectives, amusing one-dimensional characters.” Any disagreement among the experts was resolved by discussion among the group. Out of the 30 picture books, we selected 29 (see Appendix 1) to perform our analysis. One book (The Snowman) has no words in it. In total, 9,687 words were included and the number of words in each book ranged from 50 to 1,406.
At the beginning of the analysis, the 29 picture books were transcribed into CHAT format according to a set of standard rules specified in CLAN and sorted in terms of their grade levels. After the transcription, the command of MOR (short for morphology) was run to assign morphological and grammatical features to the words. Next, the command of KIDEVEAL was executed to calculate several indices that are important indicators of children’s language development (MacWhinney, 2000), including traditional measurements such as Mean Length of Utterance (MLU) and Type/Token Ratio (TTR) (See Supplementary Material for a sample chat file).
In total, KIDEVAL generated 42 different indices. However, not all of these could be used. For example, some texts do not contain a sufficient number of sentences for a given index to be calculated (e.g., “MLU of the first 100 utterance in morphemes”), and some indices are identical due to the nature of the texts (e.g., “total number of words for each speaker” and “frequency of tokens”). After removing these uninformative indices, 11 indices remained. The indices were further divided into six syntactic indicators (total number of utterances, number of verbs per utterance, number of DSS-eligible utterances, mean length of utterance in words, mean length of utterance in morphemes and number of Brown’s 14 grammatical morphemes) and five vocabulary indicators (frequency of types, frequency of tokens, type/token ratio, number of different words in the first 100 words, and Diversity).
We first analyzed indicators across the grade levels so that we have a general idea of text features across the three grade levels. Figure 1 shows the means of the z-scores of syntactic indices across the three grade levels (K1–K3). It can be seen that the majority of the variables increase linearly, but Verbs per Utterance decrease slightly from K2 to K3. The largest changes were found in the Number of DSS-eligible Utterances (DSS_Utts).
Figure 1. Means of the Z-score of syntactic indicators among the three levels. TOT_UTT, total number of utterance; DSS_utts, number of DSS-eligible utterances; Verbs_Utt, number of verbs per utterance; MLU_words, mean length of utterance in words; MLU_morphemes, mean length of utterance in morphemes; Total Non-zero Mors, total number of non-zero morphemes.
Figure 2 shows the means of the z-scores of vocabulary indices across the three grade levels (K1–K3). Frequency of Types (FREQ_types) and vocabulary diversity (VocD) showed the most distinctive changes across grade levels.
Figure 2. Means of the Z-score of vocabulary indicators among the three levels. FREQ_types, total number of different words; FREQ tokens, total number of words; FREQ_TTR, type/token ratio; NDW_ 100, number of different words in the first 100 words; VocD, vocabulary diversity (D).
To identify any differences between grade levels, we ran a one-way ANOVA, with grade level as the independent variable, and the 11 indices as dependent variables. Table 1 summarizes the results. We found significant between-level differences for seven of the eleven indices. There were no significant differences between grades for Total number of utterance (TOT-UTT), MLU in words (MLUw), Type/token ratio (TTR) and Number of different words in 100 words (NDW_100). For those seven indices that showed a significant difference, the effect sizes ranged from 0.25 to 0.5, suggesting an overall moderate effect size. A post hoc Scheffé Test and Games-Howell Test further showed higher linguistic complexity for K3 compared to K1, some for K2 compared to K1, but no significant difference between K2 and K3.
Table 1. One-way analysis of variance summary table comparing different difficulty levels in regard to the eleven linguistic characteristics.
Tables 2, 3 summarize the correlations between the 11 indices and grade level. All indices except Type/Token Ratio and NDW_100 (Number of different words in 100 words) showed a significant correlation with grade level, five of which can be considered as moderately correlated (r > 0.50). Many indices also strongly correlated with each other. For example, the correlation coefficient between MLUw and MLUm reached r = 0.99 (p < 0.01), and it is remarkably high between Verb_Utt and MLUw (r = 0.91, p < 0.01) and MLUm (r = 0.90, p < 0.01) as well.
To find out what linguistic characteristics are predictive of the expert leveling of the picture books, we ran an ordinal logistic regression twice, once at the vocabulary level and once at the syntactic level. To avoid the issue of collinearity, four indices (Verb_Utt, MLUw, FREQ_types, and NDW_100) were excluded. Grade level was used as the dependent variable while the indices were used as the independent variables. None of the four syntactic indices (TOT_UTT, DSS_Utts, MLUm, and Total non-zero mors) was predictive. With respect to vocabulary complexity, only vocabulary diversity (D) was a significant predictor (see Table 4). That accounted for approximately 27.2% of the variance in the outcome (McFadden’s pseudo - R2 = 0.272).
We found significant differences for seven of the eleven indices among the texts for the three grade levels, with moderate effect sizes. Although there was no significant difference for three features between K2 and K3, the means of each index indicate that in general, the difficulty level of the texts increases from K1 to K2, and from K2 to K3, in line with the experts’ leveling of books. However, when trying to predict expert rating from those indices, we found only vocabulary diversity (D) to be a statistically significant predictor. Readability formulas can be problematic for two reasons. First, different formulas yield different text difficulty scores, making it difficult to determine a text’s difficulty level with certainty. Second, readability formulas do not go beyond surface features such as word frequency and sentence length. However, discourse level information (i.e., cohesion and coherence of the text) and other cognitive factors (e.g., level of abstraction of illustrations) also affect the difficulty level of book. It is therefore advisable to combine quantitative and qualitative approaches of text leveling when assigning children’s picture books. That suggests that other factors (not captured by the indices) play a role when experts determine what is appropriate for a given grade level. In other words, experts tend to evaluate the picture book complexity and appropriateness from a broader perspective.
In the following, we discuss in more detail first our findings for measures of syntactic complexity and then for vocabulary complexity. We then go on to evaluate the usefulness of these indicators for assessing text complexity in children’s picture books.
Syntactic complexity is widely discussed in the field of leveled reading (Mesmer et al., 2012; Frantz et al., 2015; Berendes et al., 2017; Jin et al., 2020). Traditional measurements are Mean length of utterance (MLU), Total number of utterance (TOT-UTT), average sentence length, and number of modifiers. As mentioned above, MLU in word and in morphemes have been two extensively used indices of language development, measured with number of words (or morphemes) divided by number of sentences. Similar to Hickey (1991) study, we found a remarkably high correlation between the two indices. However, only MLUm was significantly different between K1 and K3, while MLUw was not. Although differences between the two different MLU measurements are assumed to be small (Hickey, 1991; Parker and Brorson, 2005), our study indicates that MLUm is more predictive in complexity of picture books than MLUw. Our finding supports Wieczorek (2010) argument that MLUw and MLUm cannot be used interchangeably as indicators of children’s language development. According to Wieczorek, MLUw is more indicative of vocabulary development, while MLUm says more about morphosyntactic development. In their analysis of Chinese EFL teaching materials for primary and secondary grades, Jin et al. (2020) found that sentence length, measured in MLUw, was the best predictor of grade level. We found that in kindergartens, MLUm is a more fine-grained indicator of text complexity. The difference between kindergarten and later school years indicates that there are no “one size fits all” measures.
Books for different grades differed also significantly from each other in the Number of DSS-eligible Utterance (DSS_Utts). Recall that DSS is a score based on a developmental scale of syntax acquisition (Lee and Canter, 1971), with more advanced constructions receiving higher scores. A sentence like “Do you like mud?” (from the book “When Spring Comes”) gets six points for the interrogative “Do,” one for the personal pronoun “you,” one for the uninflected verb “like” and one for the whole sentence, which adds up to nine points. The Number of DSS-eligible Utterance in K3 picture books was remarkably higher than that of K1, indicating that texts in K3 are grammatically more complex than that of K1. Therefore, DSS_Utts is seemed to be a useful indicator in grading young children’s picture books as well.
The last index among the indices of syntactic complexity that differed significantly between grade levels is Total_non_zero_mors. Total_non_zero_mors counts how many of Brown (1973) 14 grammatical morphemes are present in a text. Total_non_zero_mors successfully differentiates not only between K1 and K3, but also between K1 and K2. This suggests that this index can be considered a useful reference for determining the difficulty levels of children’s picture books. Even though Brown’s 14 grammatical morphemes have been widely used and validated by previous research (Pinker, 1981; Bland-Stewart and Fitzgerald, 2001), this is the first time these have been used to measure text difficulty. In sum, syntactic complexity in the 29 picture books increases across grade K1 to K3, as demonstrated by Number of DSS-eligible Utterances, Mean Length of Utterance in morphemes, Number of Verbs per Utterance, and Total Number of Non-Zero Morphemes.
Vocabulary complexity has traditionally been measured looking both at the token frequency (i.e., the total number of words in a text), and the type frequency (i.e., the total number of different words that occur in texts). For example, in the sentence “Brown Bear, Brown Bear, What Do You See?,” there are six types and eight tokens, as the words “brown” and “bear” occur twice. In the current study, both token frequency and type frequency increased significantly from K1 to K2 and from K1 to K3. Token frequency had the largest effect size among the 11 indices, suggesting that the sheer number of words is a strong indicator of the difficulty level of a picture book. Therefore, it can be roughly said that the more words a text contains, the more complex the text is. The number of words (or new words) can thus be used as a proxy for text difficulty. Our study suggests that token frequency can be used to grade early reading texts.
However, we did not find significant differences in the Type/Token Ratio of 29 picture books among the three grade levels. TTRs were on average about 0.40, regardless of grade level. This ratio is similar to what Klee (1992) reported in his study of 2–4-year-old native English speakers (approximately 42 in each age group). TTR has also been widely used as a measure of vocabulary diversity and applied to examine the richness of vocabulary use. In our study, the TTR ranged from 0.21 to 0.62, suggesting that almost every word occurs twice or three times in texts. Nouns and verbs that occurred more than ten times in the 29 books can be found in Appendix 2. Words like “say” and “see” occur more than 60 times across the 29 books examined. This is not surprising, as it is a characteristic of children’s literature that words occur repetitively to create rhythm and predictability (Rog and Burton, 2001) and to reduce children’s cognitive load in processing and comprehending language. Furthermore, because the TTR is sensitive to sample size differences and because token frequency (i.e., number of words) increases along with the grade level, TTR might be a less suitable as an indicator of text complexity of children’s picture book.
Another vocabulary diversity index provided in CLAN is vocabulary diversity (VocD), which is also called D. It is a new method proposed later than the Type/Token Ratio by McKee et al. (2000) and it is assumed more reliable and more informative compared with the Type/Token Ratio as it is independent of sample size effects (MacWhinney, 2000). A higher vocabulary diversity value suggests greater vocabulary diversity. We found significant differences of vocabulary diversity between K1 and K3, indicating that vocabulary in the K3 picture books was more diverse than in K1 picture books. Vocabulary diversity was also numerically higher from K1 to K2 and from K2 to K3, but not significantly so. Our results support the argument that vocabulary diversity is indeed a more informative indicator than Type/Token Ratio (Richards, 1987).
Overall, vocabulary complexity in the 29 books increases across grade K1–K3, as reflected by frequency of types, frequency of tokens, and vocabulary diversity. These results provide compelling evidence about the usefulness of these three linguistic characteristics in investigating vocabulary complexity in children’s picture books.
However, although most indices – both syntactic and vocabulary indices – increase significantly across the three grade levels, only Vocabulary Diversity predicted experts’ grading of picture books. This suggests that other factors are at play when experts grade books. As mentioned above, experts’ judgment is holistic, and includes the consideration of aspects such as the quality of illustrations or the themes covered in a book. Vocabulary diversity may account for a large portion of what experts consider text difficulty (which is in turn one important aspect of overall difficulty/appropriateness of a book), and may therefore be a predictor of grade level. Among all linguistic measures, vocabulary diversity thus seems to be particularly well-suited for evaluating children’s picture books.
We used the software CLAN to automatically extract several linguistic indices that are typically used to measure child language development. We found that these indices can detect significant differences in text complexity between grade levels of picture books. Thus, automatically obtained, quantitative measures align with experts’ judgments of what constitutes a more challenging book text.
The indices capture both syntactic and vocabulary complexity. Among the syntactic measures, mean length of utterance (MLU) in morphemes turned out to be a better indicator than MLU in words, supporting the notion that MLUm is better suited as a morphosyntactic measure. In addition, we found that indices using grammatical morphemes (Brown’s 14 grammatical morphemes, DSS) were indicative of between-grade differences and can thus be used as indices for determining text difficulty levels. Finally, the more verbs a picture book contains, the more difficult it is judged by experts. Among the vocabulary measures, token frequency had the largest effect size. Thus, the more words a book contains, the more difficult it is judged by experts. However, among all indices, only D (vocabulary diversity) could be used to predict experts’ leveling. Our study thus shows that experts base their judgments on quantifiable differences in linguistic complexity, and that they also use other factors to determine which books are suitable for which age group.
We examined the usefulness of eleven linguistic characteristics in explaining which linguistic features determine text complexity in young children’s picture books. Our implementation differs from previous works in that we used CLAN, a software used to analyze child-directed and children’s speech, to automatically analyze texts. Our analysis narrowed these complexity indices down to those that are most aligned with experts’ overall leveling of picture books. Our findings allow teachers, curriculum designers, and early childhood educators to make use of open source and free online tools such as CLAN to help determine picture books levels for young EFL learners in kindergarten.
The current study investigated only 11 indices, focusing on two aspects of texts, vocabulary and syntax, while giving little concern to other features such as cohesion and other subsystems (e.g., phonology), which are also important in children’s language learning. In addition, it should be noted that although fully automatic and computerized analysis of text complexity can yield objective and quantitative data, it cannot be used as the sole method of grading books, especially children’s picture books. A quantitative approach leaves many components other than the text out of consideration. Elements such as illustrations of books, font size and layout of print, and rhymes also play important roles in children’s comprehension of picture books (Rog and Burton, 2001). In addition to factors such as readers’ prior knowledge and reading experience, the interaction between the text and the reader should also be considered. In conclusion, picture book difficulty level can and should be assessed through a combination of different approaches, including holistic human rating, automated text analysis, artificial intelligence assisted evaluation of text and illustrations, and ideally interactivity between the picture book and its readers.
The original contributions presented in the study are included in the article/Supplementary Material, further inquiries can be directed to the corresponding author.
JZ: conceptualization, methodology, formal analysis, writing – original draft, visualization, and revision. MZ: data curation, methodology, formal analysis, writing – original draft, and visualization. LR: methodology, formal analysis, writing – original draft, and visualization. SC: methodology, formal analysis, supervision, writing – review and editing, project administration, and funding acquisition. All authors contributed to the article and approved the submitted version.
This work was supported by the Fundamental Research Funds for the Central Universities under Grant (18wkz12) and Shenzhen iRead Non-profit Foundation (Shenzhen, China, grant number 7108762-01).
The authors declare that the research was conducted in the absence of any commercial or financial relationships that could be construed as a potential conflict of interest.
All claims expressed in this article are solely those of the authors and do not necessarily represent those of their affiliated organizations, or those of the publisher, the editors and the reviewers. Any product that may be evaluated in this article, or claim that may be made by its manufacturer, is not guaranteed or endorsed by the publisher.
We thank all research assistants for preparing the data. We also thank the editor and reviewers for their valuable comments on the earlier version of this manuscript.
The Supplementary Material for this article can be found online at: https://www.frontiersin.org/articles/10.3389/feduc.2022.758736/full#supplementary-material
Al Khaiyali, A. T. S. (2014). ESL elementary teachers’ use of children’s picture books to initiate explicit instruction of reading comprehension strategies. English Lang. Teach. 7, 90–102. doi: 10.5539/elt.v7n2p90
Beauchat, K. A., Blamey, K. L., and Walpole, S. (2009). Building preschool children’s language and literacy one storybook at a time. Read. Teacher 63, 26–39. doi: 10.1598/rt.63.1.3
Berendes, K., Vajjala, S., Meurers, D., Bryant, D., Wagner, W., Chinkina, M., et al. (2017). Reading demands in secondary school: does the linguistic complexity of textbooks increase with grade level and the academic orientation of the school track? J. Educ. Psychol. 110, 518–543. doi: 10.1037/edu0000225
Biemiller, A., and Boote, C. (2006). An effective method for building meaning vocabulary in primary grades. J. Educ. Psychol. 98, 44–62. doi: 10.1037/0022-0663.98.1.44
Bland-Stewart, L. M., and Fitzgerald, S. M. (2001). Use of Brown’s 14 grammatical morphemes by bilingual hispanic preschoolers: a pilot study. Commun. Disord. Quar. 22, 171–186. doi: 10.1177/152574010102200403
Crossley, S. A., Allen, D. B., and McNamara, D. S. (2011). Text readability and intuitive simplification: a comparison of readability formulas. Read. Foreign Lang. 23, 84–101.
Cutler, L., and Slicker, G. (2020). Picture book portrayals of the transition to kindergarten: who is responsible? Early Childhood Educ. J. 19, 671–701. doi: 10.1007/s10643-020-01040-w
Chen, S., Zhao, J., de Ruiter, L., Zhou, J., and Huang, J. H. (2020). A burden or a boost: the impact of early childhood English learning experience on lower elementary English and Chinese achievement. Int. J. Bilingual Educ. Bilingualism 25, 121–1229. doi: 10.1080/13670050.2020.1749230
Denning, J., Pera, M. S., and Ng, Y. K. (2016). A readability level prediction tool for K-12 books. J. Assoc. Inform. Sci. Technol. 67, 550–565. doi: 10.1002/asi.23417
Elmonayer, R. (2013). Promoting phonological awareness skills of Egyptian kindergarteners through dialogic reading. Early Child Dev. Care 183, 1229–1241. doi: 10.1080/03004430.2012.703183
Ellis, N., and Beaton, A. (1993). Psycholinguistic determinants of foreign language vocabulary learning. Lang. Learn. 43, 559–617. doi: 10.1111/j.1467-1770.1993.tb00627.x
Fitzgerald, J., Elmore, J., Koons, H., Hiebert, E. H., Bowen, K., Sanford-Moore, E. E., et al. (2015). Important text characteristics for early-grades text complexity. Am. Psychol. Assoc. 107, 4–29. doi: 10.1037/A0037289
Fountas, I. C., and Pinnell, G. S. (2009). The Fountas & Pinnell Leveled Book List, K-8, Print Version. Portsmouth, NH: Heinemann.
Fountas, I. C., and Pinnell, G. S. (1996). Guided Reading: Good First Teaching for all Children. Portsmouth, NH: Heinemann.
Frantz, R., Starr, L., and Bailey, A. (2015). Syntactic complexity as an aspect of text complexity. Educ. Research. 44, 387–393. doi: 10.3102/0013189X15603980
Graesser, A. C., McNamara, D. S., Louwerse, M. M., and Cai, Z. (2004). Coh-Metrix: analysis of text on cohesion and language. Behav. Res. Methods Instruments Computers 36, 193–202. doi: 10.3758/BF03195564
Hickey, T. (1991). Mean length of utterance and the acquisition of Irish. Linguist. Inst. Irel. 18, 553–569. doi: 10.1017/S0305000900011247
Hiebert, E. H. (2002). “Standards, assessment, and text difficulty,” in What Research has to say About Reading Instruction, 3rd Edn, eds A. E. Farstrup and S. J. Samuels (Newark, DE: International Reading Association), 337–369. doi: 10.1598/0872071774.15
Hiebert, E. H., and Pearson, P. D. (2014). Understanding text complexity: introduction to the special issue. Elementary School J. 115, 153–160. doi: 10.1086/678446
Hindman, A., Wasik, B., and Erhart, A. (2012). Shared book reading and head start preschoolers’ vocabulary learning: the role of book-related discussion and curricular connections. Early Educ. Dev. 23, 451–474. doi: 10.1080/10409289.2010.537250
Hoff, E., Welsh, S., Place, S., and Ribot, K. (2014). “Properties of dual language input that shape bilingual development and properties of environments that shape dual language input,” in Input and Experience in Bilingual Development, eds T. Grüter and J. Paradis (Amsterdam, PA: John Benjamins).
Holster, T., Lake, J., and Pellowe, W. (2017). Measuring and predicting graded reader difficulty. Read. Foreign Lang. 29, 218–244.
Hui, A. N. N., Chow, B. W.-Y., Chan, E. S. M., and Leung, M.-T. (2020). Reading picture books with elements of positive psychology for enhancing the learning of English as a second language in young children. Front. Psychol. 10:2899. doi: 10.3389/fpsyg.2019.02899
Huttenlocher, J., Waterfall, H., Vasilyeva, M., Vevea, J., and Hedges, L. (2010). Sources of variability in children’s language growth. Cogn. Psychol. 61, 343–365. doi: 10.1016/j.cogpsych.2010.08.002
Jin, T., Lu, X., and Ni, J. (2020). Syntactic complexity in adapted teaching materials: differences among grade levels and implications for benchmarking. Modern Lang. J. 104, 192–208. doi: 10.1111/modl.12622
Klee, T. (1992). Developmental and diagnostic characteristics of quantitative measures of children’s language production. Top. Lang. Disord. 12, 28–40. doi: 10.1097/00011363-199202000-00005
Lee, L. L., and Canter, S. M. (1971). Developmental sentence scoring: a clinical procedure for estimating syntactic development in children’s spontaneous speech. J. Speech Hear. Disord. 36, 315–340. doi: 10.1044/jshd.3603.315
Lu, X. (2010). Automatic analysis of syntactic complexity in second language writing. Int. J. Corpus Linguistics 15, 474–496. doi: 10.1075/ijcl.15.4.02lu
MacWhinney, B. (2000). The CHILDES Project: Tools for Analyzing Talk, 3rd Edn. Mahwah, NJ: Lawrence Erlbaum Associates.
Malvern, D. D., and Richards, B. J. (1997). “A new measure of lexical diversity,” in Evolving Models of Language, eds A. Ryan and A. Wray (Clevedon: Multilingual Matters).
Malvern, D., Richards, B., Chipere, N., and Durán, P. (2004). Lexical Diversity and Language Development. Basingstoke: Palgrave Macmillan.
Marchman, V., Martínez-Sussmann, C., and Dale, P. (2004). The language-specific nature of grammatical development: evidence from bilingual language learners. Dev. Sci. 7, 212–224. doi: 10.1111/j.1467-7687.2004.00340.x
Massey, S. L. (2004). Teacher–child conversation in the preschool classroom. Early Childhood Educ. J. 31, 227–231. doi: 10.1017/S0305000919000199
McKee, G., Malvern, D., and Richards, B. (2000). Measuring vocabulary diversity using dedicated software. Literary Linguistic Comput. 15, 323–338. doi: 10.1080/02687038.2011.606974
Meisel, J. M. (2011). Bilingual language acquisition and theories of diachronic change: bilingualism as cause and effect of grammatical change. Bilingualism: Lang. Cogn. 14, 121–145. doi: 10.1017/s1366728910000143
Mesmer, H. A., Cunningham, J. W., and Hiebert, E. H. (2012). Toward a theoretical model of text complexity for the early grades: learning from the past, anticipating the future. Read. Res. Quar. 47, 235–258. doi: 10.1002/RRQ.019
Miller, J. F. (1991). “Quantifying productive language disorders,” in Research on Child Language Disorders: A Decade of Progress, ed. J. F. Miller (Austin, TX: Pro-Ed), 211–220.
Montag, J. L. (2019). Differences in sentence complexity in the text of children’s picture books and child-directed speech. First Lang. 39, 527–546. doi: 10.1177/0142723719849996
Nice, M. M. (1925). Length of sentences as a criterion of a child’s progress in speech. J. Educ. Psychol. 16, 370–379. doi: 10.1037/h0073259
Parker, M. D., and Brorson, K. (2005). A comparative study between mean length of utterance in morphemes (MLUm) and mean length of utterance in words (MLUw). First Lang. 25, 365–376. doi: 10.1177/0142723705059114
Pearson, P. D., and Hiebert, E. H. (2014). The state of the field: qualitative analyses of text complexity. Elem. Sch. J. 115, 161–183. doi: 10.1086/678297
Pinker, S. (1981). On the acquisition of grammatical morphemes. J. Child Lang. 8, 477–484. doi: 10.1017/S0305000900003317
Rice, M. L., Smolik, F., Perpich, D., Thompson, T., Rytting, N., and Blossom, M. (2010). Mean length of utterance levels in 6-month intervals for children 3 to 9 years with and without language impairments. J. Speech Lang. Hear. Res. 53, 333–349. doi: 10.1044/1092-4388(2009/08-0183)
Richards, B. (1987). Type/Token ratios: what do they really tell us? J. Child Lang. 14, 201–209. doi: 10.1017/S0305000900012885
Rog, L. J., and Burton, W. (2001). Matching texts and readers: leveling early reading materials for assessment and instruction. Read. Teacher 55, 348–356.
School Renaissance Institute (2000). The ATOS Readability Formula for Books and how it Compares to Other Formulas. Madison, WI: School Renaissance Institute.
Sheehan, K. M., Kostin, I., Napolitano, D., and Flor, M. (2014). The TextEvaluator tool: helping teachers and test developers select texts for use in instruction and assessment. Elementary School J. 115, 184–209. doi: 10.1086/678294
Sierschynski, J., Louie, B., and Pughe, B. (2014). Complexity in picture books. Read. Teacher 68, 287–295. doi: 10.1002/trtr.1293
Smith, D. R. (1989). The Lexile Scale in Theory and Practice (Final Report). Washington, DC: MetaMetrics.
Snow, C. (2002). Reading for Understanding: Toward an R&D Program in Reading Comprehension. Santa Monica, CA: Rand Corporation.
Snow, C. E., Burns, M. S., and Griffin, P. (eds) (1998). Preventing Reading Difficulties in Young Children. Washington, DC: National Academy Press.
Sun, H., de Bot, K., and Steinkrauss, R. (2015). A multiple case study on the effects of temperamental traits in Chinese preschoolers learning English. Int. J. Bilingualism 19, 703–725. doi: 10.1177/1367006914534332
Sun, H., Steinkrauss, R., Tendeiro, J., and De Bot, K. (2016). Individual differences in very young children’s English acquisition in China: internal and external factors. Bilingualism-Lang. Cogn. 19, 550–566. doi: 10.1017/s1366728915000243
Thordardottir, E. (2011). The relationship between bilingual exposure and vocabulary development. Int. J. Bilingualism 15, 426–445. doi: 10.1177/1367006911403202
Torgesen, J. K. (2004). Preventing early reading failure and its devastating downward spiral. Am. Educ. 28, 6–19.
Wasik, B. A., Bond, M. A., and Hindman, A. (2006). The effects of a language and literacy intervention on Head Start children and teachers. J. Educ. Psychol. 98, 63–74. doi: 10.1037/0022-0663.98.1.63
Wieczorek, R. (2010). Using MLU to study early language development in English. Psychol. Lang. Commun. 14, 59–69. doi: 10.2478/v10057-010-0010-9
Zevenbergen, A. A., and Whitehurst, G. J. (2003). “Dialogic reading: a shared picture book reading intervention for preschoolers,” in On Reading Books to Children: Parents and Teachers, eds A. V. Kleeck and S. A. Stahl (Malwah, NJ: Lawrence Erlbaum Associates).
Keywords: linguistic features, children’s picture book, text complexity, English as a foreign language, leveled reading
Citation: Zhao J, Zhu M, de Ruiter L and Chen S (2022) Linguistic Indicators for Text Complexity in Picture Books for Young Chinese Children Learning English as a Foreign Language. Front. Educ. 7:758736. doi: 10.3389/feduc.2022.758736
Received: 14 August 2021; Accepted: 08 April 2022;
Published: 17 May 2022.
Edited by:
L. Quentin Dixon, Texas A&M University, United StatesReviewed by:
Yuuko Uchikoshi, University of California, Davis, United StatesCopyright © 2022 Zhao, Zhu, de Ruiter and Chen. This is an open-access article distributed under the terms of the Creative Commons Attribution License (CC BY). The use, distribution or reproduction in other forums is permitted, provided the original author(s) and the copyright owner(s) are credited and that the original publication in this journal is cited, in accordance with accepted academic practice. No use, distribution or reproduction is permitted which does not comply with these terms.
*Correspondence: Si Chen, c2ljNzczQG1haWwuaGFydmFyZC5lZHU=
Disclaimer: All claims expressed in this article are solely those of the authors and do not necessarily represent those of their affiliated organizations, or those of the publisher, the editors and the reviewers. Any product that may be evaluated in this article or claim that may be made by its manufacturer is not guaranteed or endorsed by the publisher.
Research integrity at Frontiers
Learn more about the work of our research integrity team to safeguard the quality of each article we publish.