- 1Education and Training Evaluation Commission, Riyadh, Saudi Arabia
- 2Department of Educational Psychology, College of Education, The University of Melbourne, Parkville, VIC, Australia
- 3Department of Psychology, College of Arts, Taif University, Taif, Saudi Arabia
This study investigated the relationship between three pre-university measures (high school grade point average, the General Aptitude Test, and the scholastic achievement admission test) and university performance as indicted by (preparatory year and cumulative university grade point average, and graduation). Data from 330,684 undergraduate students from 23 universities across the Kingdom of Saudi Arabia were analyzed using a multilevel structural equation modeling approach. Results indicated that all pre-university measures were consistent in predicting university performance. These findings supported previous results that pre-university measures performance predict eventual performance at the university, including performance at the preparatory year, and whether the student stays regular and eventually completes university studies. These results have implications for the importance of the pre university measures in predicting university eventual success and graduation and this study adds to the body of work that can inform further cost-benefits of the pre university measures. For policymakers, these findings suggest that strengthening support during secondary school levels can have positive effects on eventual performance at university.
Introduction
In many countries, university admission decisions depend on students’ performance on three measures: high school grade point average (HSGPA), standardized aptitude, and standardized achievement tests (Atkinson, 2001; Geiser and Studley, 2002; Zwick, 2012, 2017). According to Koljatic et al. (2013), aptitude tests focus on measuring verbal and mathematical abilities not directly tied to the curriculum, whereas achievement tests are based on clear curricular guidelines and measure accomplishment. Universities require these two admission standardized tests, as research has shown that students with high scores are most likely to succeed academically (Evans, 2015). This is based on the thought pattern that learning is an accumulative process, and students admitted with higher entry qualifications are expected to be better prepared for university study than those with lower qualifications. In addition to the predictive validity, the two admission standardized tests have other benefits (Beatty et al., 1999): (1) standardization: admission standardized tests are standardized; hence, they can eliminate the difference in high school curricula and grading; (2) efficiency: they can be conducted at a relatively low cost to students and are economical for the universities as they can compare a vast number of applicants’ profiles within a short time; and (3) opportunity: students can showcase their talents, even though their HSGPA may not be strong enough to attend prestigious universities.
In the Kingdom of Saudi Arabia, the number of universities and colleges has expanded primarily to satisfy the overflow in demand for post-secondary education (Alghamdi and Al-Hattami, 2014; Hamdan, 2017). However, the expansion involved finding a strategy that would restrict students’ admission to achieve certain standards (Hamdan, 2017). The Saudi government explicitly stated in the Ninth National Development Plan that the higher education system must focus on preparing professional graduates who can support the government’s ambitious multi-billion-dollar Saudi royal development projects that have been launched with the goal of transforming Saudi Arabia into a knowledge-based society (Ministry of Economy and Planning., 2010). Thus, the higher learning system became more challenging and demanding for students who were preparing for university studies that focused on promoting 21st-century skills. To assess students’ readiness for university studies, students are required, in addition to their HSGPA, to take the General Aptitude Test (GAT) and Scholastic Achievement Admission Test (SAAT)—developed by the National Center for Assessment. The GAT measures Arabic language proficiency, critical thinking, and mathematical reasoning ability; whereas the SAAT measures knowledge of biology, chemistry, physics, math, linguistics, and social studies (Education and Training Evaluation Commission, 2021). The weight allocated to the GAT and SAAT varies as admission requirements differ from one university to another. The GAT typically comprises 30%, while the remaining percentage is distributed between the SAAT (40%) and HSGPA (30%). Students can repeat the admission tests three times in a single year, and the best score is considered for admissions.
The combination of standardized test scores and high school GPA consistently predicts university success and retention (Burton and Ramist, 2001; Noble, 2003; Julian, 2005; Kobrin et al., 2008; Wiley, 2014). Kobrin et al. (2008) work showed an incremental increase in predictive validity by 0.08 when the standardized tests and HSGPS were combined. In an intensive review by Burton and Ramist (2001), the results indicated that the combination of standardized tests and HSGPA can make key and accurate contributions to predict first-year GPA, cumulative university GPA, and graduation. Mattern and Patterson’s (2012) research examined high school GPA and standardized tests to predict retention in the second and third years of college. The findings showed that students who, in addition to having standardized test scores, had high school GPA reported correspondingly higher retention rates in the second and third years of their universities than students having only HSGPA.
In the Kingdom of Saudi Arabia, many studies have indicated a positive relationship between pre-university measures and university performance (Alghamdi and Al-Hattami, 2014; Alnahdi, 2015). Al-Alwan (2009) and Al Alwan et al. (2013) work indicated a significant correlation between the three measures and the progress and future academic performance among students from health colleges. In addition, AlQataee (2014) conducted an analysis of data from a sample of over 5,000 students on the predictive validity of the three measures. The findings showed that the three measures significantly predicted first-year GPA. Alshumrani’s (2007) finding indicates that the three measures can explain 11% of first-semester college GPA.
Over the past 10 years, Saudi educators and policymakers have agreed that high school graduates lack essential education and skills—mathematics, reading comprehension, writing, English language, personality and communication, and information and technology skills—that would enable them to succeed in higher education as independent learners (Khoshaim et al., 2018). Atkinson (2007) argued that standardized tests do not reflect students’ readiness for university studies. These standardized tests are limited to assessing specific cognitive skills at the time of testing, but do not determine social and emotional adjustment. Many undergraduate students face challenges in their first year of university for different reasons. The sudden shift from the controlled environment of school and family to an environment in which students are expected to accept personal responsibility for both academic and social aspects of their lives may create distress, making them adopt maladaptive coping mechanisms manifested in dropping-out, underachievement, lack of motivation and interest, and disengagement from academic and social life (Lowe and Cook, 2003). Another reason is that schools lack preparation for high education as there is little or no emphasis on teaching independence (Mutambara and Bhebe, 2012). In addition, teaching and assessment styles in many secondary schools lend themselves to developing a particular set of study skills and learning strategies that are no longer entirely relevant to the more independent styles of learning expected in higher education (Giuliano and Sullivan, 2007).
One approach to prepare students for university life is through the preparatory year. Saudi universities have implemented a preparatory program to prepare students academically, socially, psychologically, and culturally. It aims to provide them with multiple skills to help them communicate well within their community and to construct successful study habits to become independent learners with capabilities and skills that enable them to move forward in the fields they want to study (Kamel, 2015). The preparatory program is a full academic year beyond high school, with a set of courses focusing on mathematics, English language, and personality and communication skills that must be completed successfully before joining the academic program (Khalil, 2010; Kamel, 2015; Khoshaim, 2017). The GPA of the preparatory year is added to overall university performance GPA. The specific structure of the preparatory year program varies across universities and depends on the demands of the academic program (Khoshaim et al., 2018). For example, in some preparatory year programs, potential medical and engineering majors require potential students to take at least one level of calculus. In contrast, it is sufficient for business or humanities majors to complete advanced algebra courses. However, some preparatory year programs do not require mathematics if the intended majors are humanities or arts, but instead focus on English language, research, and communication and technology skills. Many educators (e.g., Keup and Kilgo, 2014) advocate for the effectiveness of the preparatory year program in increasing students’ retention and graduation and promoting academic, personal, and communication skills.
The Current Study
Several factors affect student performance during their university years; but prior performance before entering university is predictive of how they will perform (McKenzie and Schweitzer, 2001; Hein et al., 2013; McManus et al., 2013) and even whether they withdraw from university. These “pre-university” factors that can impact university performance are important to investigate because early interventions can target them if they do exert an effect or are predictive of university performance. It is also of interest to investigate variations of this effect by university, as it could differ; therefore, a more customized or targeted approach might be needed for specific interventions. For example, if the predictive ability of certain measures of pre-university performance only applies to a subset of universities, the implications for interventions and even policy changes can only be valid for the subset.
In a related aspect, it is also of interest to investigate whether universities are attracting students based on their pre-university performance in a consistent manner. This is because some universities are more prestigious or appealing to future students for various reasons (e.g., reputation, quality, etc.). It is worth investigating whether the pre-university measures are consistent in how universities rank regarding the students who choose them. For example, if the best universities attract the best students based on their standardized entrance exams but not on their high school GPAs, it is important to know why this occurs.
The main research questions for this study are:
1. Do universities attract the best students uniformly across the three pre-university measures?
2. Do pre-university measures predict university performance?
These research questions are investigated using a different approach, and the results are reported separately in this article. Although these are discussed jointly, their implications are considered holistically.
Materials and Methods
Sample
Data were obtained from 330,684 students who graduated from high school with a science track and were enrolled in 23 universities across the Kingdom of Saudi Arabia as first-time students entering in the fall-term from 2013 to 2016. The universities were located across the five regions (north, south, west, east, or center) of the Kingdom. Each university reported cumulative GPA at the end of each academic term; academic status (dropped out or graduated); and composite scores of SAAT, GAT verbal, and GAT Quantitative. Information on the cumulative high school GPA, the type of university (private vs. public), and preparatory year program type (science, health, or humanities) was also provided by each university. Table 1 provides descriptive information of the universities.
Measures
Independent Variables
Pre-university performance is a latent independent variable that uses the following three measures as indicators:
1. HSGPA is the average score of subjects taken in grades 11 and 12. HSGPA is expressed on a scale of 100 points; however, it was standardized in this study to account for school variation.
2. The SAAT (Education and Training Evaluation Commission, 2021) assesses four major subject matter areas: biology, chemistry, physics, and mathematics. The SAAT focuses on the content of the official 3-year curriculum of Saudi Arabian high schools with a science track. The 110 items of the SAAT are distributed as follows: 20% of each subject for the first year of the high school syllabus and 40% of each subject for the second and third years of the high school syllabus. Each item contained only one correct response and three distractors. The SAAT exhibits acceptable psychometric properties (Tsaousis et al., 2018), with alpha reliabilities ranging between 0.62 and 0.74. In this study, the total SAAT score was calculated.
3. The GAT consists of two sections: verbal (GAT-V) and quantitative (GAT-Q). The GAT-Q consists of 55 multiple-choice items covering five content areas: arithmetic (20 items), geometry (13 items), algebra statistical and analytical (15 items), and comparison questions (7 items). Cronbach’s alphas (α) ranged from 0.73 (Arithmetic) to 0.82 (comparison) (Dimitrov, 2013). The GATV consists of 65 multiple-choice items covering three content-specific domains: analogy (21 items), sentence completion (17 items), and reading comprehension (27 items). Cronbach’s alphas were 0.821 for analogy, 0.748 for sentence completion, and 0.730 for reading comprehension (Dimitrov and Shamrani, 2015). In this study, total GATQ and GAT-V scores were used separately.
Dependent Variables
This represents university performance as a latent variable, and it is measured by,
1. The GPA of the preparatory academic year.
2. The cumulative GPA of academic terms after completing the preparatory year and specializing in different academic majors.
3. Completion is a dichotomous score assessing whether students graduated (0 = dropped out, 1 = graduated).
Procedure
Twenty-three university datasets were provided by the Research and Data Department at the Evaluation of Education and Training Commission in Saudi Arabia. These datasets were merged using R package (name of the package). The data from the 23 universities were analyzed to answer the first research question. However, given that some universities (12 universities) do not follow the preparatory year policy, the analysis of answering the second research was limited to 225,385 students from 11 university datasets.
Analytical Approach
For the first research question, the analytical approach used a ranking system in each of the pre-university measures (HSGPA, SAAT, GAT-V, and GAT-Q) to test if the ranks were consistent or uniform across all 23 universities. The approach looks at whether the universities that attract the best students based on one measure (and thus rank highly on this measure regarding other universities) are also attracting the best based on the other measures. To statistically test the hypothesis that the rankings are uniform across the four measures, the Friedman test (Friedman, 1940; Hollander and Wolfe, 1973) was used:
in which k represents the metrics (HSGPA, SAAT, and GAT) and n is the number of universities. The Q statistic was then tested using the chi-square test.
For the second research question, a multilevel structural equation model (Preacher et al., 2010) was used to investigate the relationship between pre-university performance and university performance. Pre-university performance is modeled as a latent variable (pre-university perf) measured by HSGPA, SAAT, GAT-V, and GAT-Q. University performance (university perf) is modeled as another latent variable, which is measured by a combination of the preparatory year GPA, GPA averages over 2 years, and completion (or currently not withdrawn). Because completion is a variable in the model, missing data that resulted in zero variance (e.g., no student has withdrawn for a particular university) for this variable necessitated excluding some universities from the analysis. The data for this analysis consisted of 225,385 students across 11 universities.
To consider university differences, the model is clustered by university with this second level being specified as a saturated level only (i.e., all variables were assessed at the individual level).
The model in Figure 1 is specified by extending the general model proposed by Muthén and Asparouhov (2008) as follows:
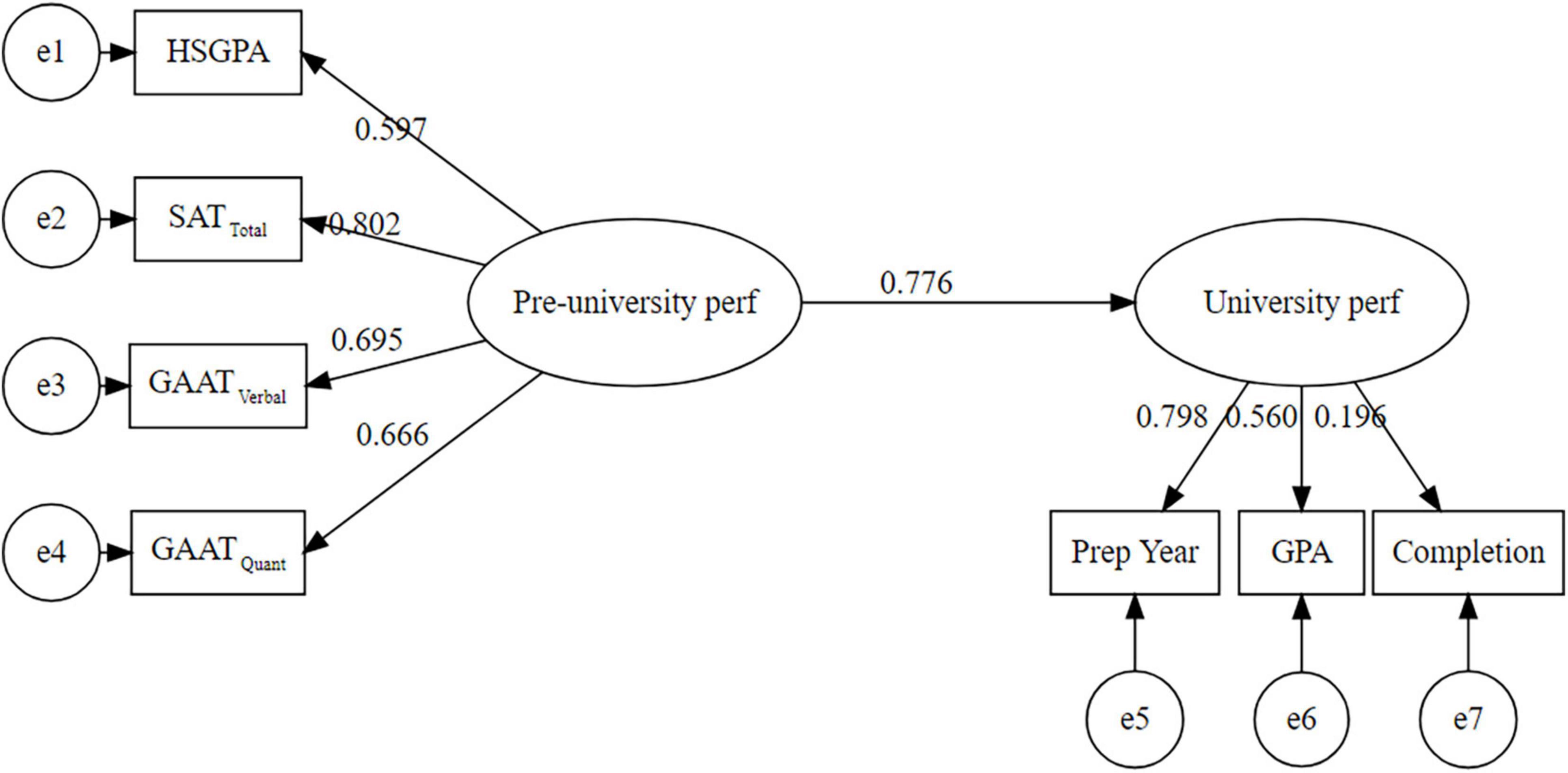
Figure 1. Path coefficients of the relationship between pre-university measures and university performance.
where η is a k × 1 vector of latent factors, α is a k × 1 vector of latent variable means, Ã is a k × p matrix of fixed regression parameters, and p is the number of predictors in X. The parameter matrices vj, Λj, K j, αj, βj, and Γj are allowed to vary at the cluster-level, where j indicates the cluster. The structural model can be extended to have a between component if there are cluster-level predictors (Preacher et al., 2010):
For the purposes of this study, all predictors are at the individual level; thus, there is no relationship between the structural model and the saturated model. All analyses were conducted in the R environment (R Core Team, 2019), and latent growth modeling was performed using the lavaan package (Rosseel, 2012).
Results
The First Research Question
The ranking based on the means of the pre-university measures are reported in Table 2, with rank 1 being the best regarding each measure mean (e.g., G04 students have the highest average high school GPA and GAT scores, while G15 students have the lowest average scores in the standardized exams).
The results of the Friedman test showed no significant differences: Δχ2(df = 3) = 0.353, p = 0.9498. This suggests that the rankings are not different from each other, and universities that rank high in their students’ high school GPA also tend to rank high in other pre-university measures. This is not surprising given the broad consistency in rankings shown in Table 2 and the moderate correlations across all four metrics.
This overall result looked at rankings across all pre-university measures for the 23 universities. To check whether the same holds for the measures based only on standardized tests (i.e., GAT-Q, GAT-V, and SAAT), a follow-up analysis was performed focusing on the rankings for these three measures only. The results were again non-significant, Δχ2(df = 2) = 0.609, p = 0.7376. This shows that university rankings are consistent, based on how they attract students based on their standardized results.
Given that the overall result and the results based on standardized measures are all non-significant, further post hoc multiple comparison tests were not performed. This evidence that universities tend to be consistent in attracting students based on their pre-university performance supports the use of a multilevel approach to investigate the second research question.
The Second Research Question
Structural equation modeling showed that the pre-university performance had significant effects on university performance, as indicted by the acceptable fit χ2(df = 13) = 7211.96, p < 0.01; comparative fit index (CFI) = 0.95, standardized root mean residual (SRMR = 0.044), root mean square error of approximation (RMSEA = 0.080), normative fit index (NFI = 0.95) based on the following thresholds: SRMR < 0.08 (Hu and Bentler, 1999), CFI and NFI > 0.90 (Bentler, 1990), and RMSEA < 0.09 would be considered acceptable fit (Steiger, 2007).
A heteroscedasticity-consistent Huber-White robust standard error estimator was used (White, 1980). As all variables were assessed at the individual level (Table 3), the main interest regarding the regression coefficients was at level 1. The unstandardized parameter estimates are reported and all parameters are significant (Table 4).
The model and resulting standardized loadings are shown in Figure 1. Among the four indicators, SAAT had the strongest effect on pre-university performance in this model. Further, results indicate that the pre-university measures have the strongest effect on the preparatory year, and eventually, this effect gets weaker, but still significant, throughout the university journey until the student graduates.
The main interest in the cluster-level (i.e., university level) model is the random variation and intraclass correlation coefficient of the predictors, which represent the variability attributed to the cluster. The results show that while preparatory year performance shows the largest relative variation regarding the cluster, all random effects at the cluster-level do not significantly vary between universities (Table 5).
Discussion
The ranking analysis showed that the measures of pre-university performance are consistent across universities and indicative of “student quality” in the sense that the best universities tend to attract the best students as measured by these pre-university metrics. In addition, this study investigated the relationship between pre-university measures and their effects on university performance. The results show that pre-university measures significantly predict university performance. Multilevel structural equation analysis has also shown that random effects do not vary significantly across universities.
The results support previous findings—that pre-university performance predicts university performance (e.g., McKenzie and Schweitzer, 2001; Hein et al., 2013). There have been multiple studies specific to the Kingdom of Saudi Arabia that also align with this finding (Yushau and Omar, 2007; Center for Excellence in Learning and Teaching., 2013; Alghamdi and Al-Hattami, 2014; McMullen, 2014; Alnahdi, 2015; Ahmad et al., 2017), although to varying degrees in scope and magnitude. It is important to note that the pre-university metrics were broadly consistent in their loadings on the latent factor of pre-university performance. This is not surprising given that the pre-university metrics are moderately correlated with each other, especially among the standardized exams. The impact of pre-university performance toward the latent factor of university performance loads strongest on the university preparatory year performance, although this is not surprising given the time proximity to the pre-university factors. But loadings show that pre-university performance significantly impacts even the university completion metric even if those two events are separated by a considerable amount of time. This highlights the importance of how a student’s performance before entering university has far-reaching effects. This has implications for the basic education sector and educational policy more broadly.
This study had some limitations. First, the examined relationships are broad and at the national level; however, it is likely that these may differ when examined at more local levels. To do so would require additional specific data, such as more detailed characteristics of the universities as well as additional demographic data for the students. This is beyond the scope of this study and the required data are not available; however, this is an interesting avenue to expand this research in the future. Second, the measure for university success is narrow given that we only have data for grades and completion; thus, it is possible that these metrics miss the career skills that may be more important for real-life success. Finally, a universally comparable metric for academic performance across all universities is lacking, as grades and completion are inextricably dependent on each university. Although we considered university differences by specifying the model to be clustered by university, this only mitigates this limitation.
Despite these limitations, given the large-scale nature of this study and the finding that university-level variation is not significant, the relationships among the variables are generalizable across the participating universities, which collectively represent the country. The findings imply that both high school grades and standardized exams predict university performance. Both factors significantly predict not only university grades but also whether students complete university studies or at least stay regular and do not withdraw. For policymakers, these findings suggest that strengthening support during secondary years can have positive effects on eventual performance at university.
Data Availability Statement
The raw data supporting the conclusions of this article will be made available by the authors, without undue reservation.
Ethics Statement
The studies involving human participants were reviewed and approved by the Education and Training Evaluation Commission. The patients/participants provided their written informed consent to participate in this study.
Author Contributions
AV designed the study, analyzed the data, and reported and discussed the results. GA developed the literature review, provided detailed description of the variables and procedure, and drafted the manuscript in accordance with the Frontiers in Psychology guidelines. Both authors read and approved the final manuscript.
Conflict of Interest
The authors declare that the research was conducted in the absence of any commercial or financial relationships that could be construed as a potential conflict of interest.
Publisher’s Note
All claims expressed in this article are solely those of the authors and do not necessarily represent those of their affiliated organizations, or those of the publisher, the editors and the reviewers. Any product that may be evaluated in this article, or claim that may be made by its manufacturer, is not guaranteed or endorsed by the publisher.
Acknowledgments
We would like to thank our colleague Fahad Al-Hozimi for his effort into collecting the data from the National Center for Assessment at the Education and Training Evaluation Commission.
References
Ahmad, W., Ali, Z., Aslam Sipra, M., and Hassan Taj, I. (2017). The impact of smartboard on preparatory year EFL learners’ motivation at a Saudi university. Adv. Lang. Lit. Stud. 8, 172–180. doi: 10.7575/aiac.alls.v.8n.3p.172
Al Alwan, I., Al Kushi, M., Tamim, H., Magzoub, M., and Elzubeir, M. (2013). Health sciences and medical college preadmission criteria and prediction of in-course academic performance: a longitudinal cohort study. Adv. Health Sci. Educ. Theory Pract. 18, 427–438. doi: 10.1007/s10459-012-9380-1
Al-Alwan, I. A. (2009). Association between scores in high school, aptitude and achievement exams and early performance in health science college. Saudi J. Kidney Dis. Transpl. 20, 448–453.
Alghamdi, A. K. H., and Al-Hattami, A. A. (2014). The accuracy of predicting university students’ academic success. J. Saudi Educ. Psychol. Assoc. 1, 1–8.
Alnahdi, G. H. (2015). Aptitude tests and successful college students: the predictive validity of the General Aptitude Test (GAT) in Saudi Arabia. Int. Educ. Stud. 8:4. doi: 10.5539/ies.v8n4p1
AlQataee, A. A. (2014). “The effect of the distributions of college course grades on the NCA admission test predictive validity,” in Proceedings of the First International Conference on Assessment & Evaluation. New York: Association for Computing Machinery.
Alshumrani, S. A. (2007). Predictive Validity of the General Aptitude Test and High School Percentage for Saudi Undergraduate Students. [PhD thesis]. Kansas: University of Kansas.
Atkinson, R. C. (2001). Achievement versus aptitude in college admissions. Issues Sci. Technol. 18, 31–36. doi: 10.4324/9780203463932_Achievement_versus_Aptitude_in_College_Admissions
Atkinson, R. C. (2007). Standardized Tests and Access to American Universities. California: University of California Press, 137–148.
Beatty, A., Greenwood, R., and Linn, R. L. (1999). Myths and Tradeoffs: The Role of Tests in Undergraduate Admissions. Washington, DC: National Academy Press.
Bentler, P. M. (1990). Comparative fit indexes in structural models. Psychol. Bull. 107, 238–246. doi: 10.1037/0033-2909.107.2.238
Burton, N. W., and Ramist, L. (2001). Predicting Success in College: SAT® Studies of Classes Graduating Since 1980. Research Report No. 2001–2. New York: College Entrance Examination Board.
Center for Excellence in Learning and Teaching. (2013). An Evaluative Study of the University Preparatory Year Program (PYP). Available online at: https://celt.ksu.edu.sa/en. (accessed on May 28, 2021).
R Core Team (2019). R: A Language and Environment for Statistical Computing. Vienna: R Foundation for Statistical Computing.
Dimitrov, D. M. (2013). General Aptitude Test-Quantitative: IRT and Trues-Core Analysis. Riyadh, Saudi Arabia: National Center for Assessment in Higher Education.
Dimitrov, D. M., and Shamrani, A. R. (2015). Psychometric Features of the General Aptitude Test–Verbal Part (GAT-V): a Large-Scale Assessment of High School Graduates in Saudi Arabia. Meas. Eval. Couns. Dev. 48, 79–94. doi: 10.1177/0748175614563317
Education and Training Evaluation Commission (2021). University Admission Tests. Kingdom of Saudi Arabia: ETEC.
Evans, B. J. (2015). College Admission Testing in America. International Perspectives in Higher Education Admission Policy: A Reader. New York: Peter Lang.
Friedman, M. (1940). A Comparison of Alternative Tests of Significance for the Problem of $m$ Rankings. Ann. Math. Statist. 11, 86–92. doi: 10.1214/aoms/1177731944
Geiser, S., and Studley, W. R. (2002). UC and the SAT: predictive validity and differential impact of the SAT I and SAT II at the University of California. Educ. Assess. 8, 1–26. doi: 10.1207/S15326977EA0801_01
Giuliano, B., and Sullivan, J. (2007). Academic wholism: bridging the gap between high school and college. Am. Second. Educ. 35, 7–18.
Hamdan, A. (2017). “Saudi Arabia: higher education reform since 2005 and the implications for women,” in Education in the Arab World, 1 Edn, ed. S. Kirdar (London: Bloomsbury Academic), 197–216. doi: 10.5040/9781474271035.ch-011
Hein, V., Smerdon, B., and Sambolt, M. (2013). Predictors of Postsecondary Success. Washington, DC: American Institutes for Research.
Hollander, M., and Wolfe, D. (1973). Nonparametric Statistical Methods. New York: John Wiley & Sons, 139–146.
Hu, L. T., and Bentler, P. M. (1999). Cutoff criteria for fit indexes in covariance structure analysis: conventional criteria versus new alternatives. Struct. Equ. Modeling 6, 1–55. doi: 10.1080/10705519909540118
Julian, E. R. (2005). Validity of the Medical College Admission Test for predicting medical school performance. Acad. Med. 80, 910–917. doi: 10.1097/00001888-200510000-00010
Kamel, J. (2015). “Preparatory year programs at the Saudi universities “a reading in the paradox between philosophy and structure,”,” in First National Conference of Preparatory Year in Saudi Universities, (Dammam, Saudi Arabia: Imam AbdulRahman Bin Faisal University).
Keup, J. R., and Kilgo, C. A. (2014). Conceptual considerations for first-year assessment. New Dir. Inst. Res. 2013, 5–18. doi: 10.1002/ir.20058
Khalil, R. (2010). Learning reconsidered: supporting the preparatory year student experience. Procedia Soc. Behav. Sci. 9, 1747–1756. doi: 10.1016/j.sbspro.2010.12.394
Khoshaim, H. B. (2017). High school graduates’ readiness for tertiary education in Saudi Arabia. Int. J. Instr. 10, 179–194. doi: 10.12973/iji.2017.10312a
Khoshaim, H. B., Khoshaim, A. B., and Ali, T. (2018). Preparatory year: the filter for mathematically intensive programs. Int. Interdiscip. J. Educ. 7, 127–136. doi: 10.36752/1764-007-009-009
Kobrin, J. L., Patterson, B. F., Shaw, E. J., Mattern, K. D., and Barbuti, S. M. (2008). Validity of the SAT® for Predicting First-Year College Grade Point Average. New York: College Board, 2005–2008.
Koljatic, M., Silva, M., and Cofré, R. (2013). Achievement versus aptitude in college admissions: a cautionary note based on evidence from Chile. Int. J. Educ. Dev. 33, 106–115. doi: 10.1016/j.ijedudev.2012.03.001
Lowe, H., and Cook, A. (2003). Mind the gap: are students prepared for higher education? J. Further Higher Educ. 27, 53–76. doi: 10.1080/03098770305629
Mattern, K. D., and Patterson, B. F. (2012). The Relationship Between SAT Scores and Retention to the Second Year: Replication with 2009 SAT Validity Sample. New York: College Board, 2011–2013.
McKenzie, K., and Schweitzer, R. (2001). Who succeeds at university? Factors predicting academic performance in first year Australian university students. Higher Educ. Res. Dev. 20, 21–33. doi: 10.1080/07924360120043621
McManus, I. C., Woolf, K., Dacre, J., Paice, E., and Dewberry, C. (2013). The Academic Backbone: longitudinal continuities in educational achievement from secondary school and medical school to MRCP (UK) and the specialist register in UK medical students and doctors. BMC Med. 11:242. doi: 10.1186/1741-7015-11-242
McMullen, M. G. (2014). The value and attributes of an effective preparatory English program: perceptions of Saudi university students. Engl. Lang. Teach. 7, 131–140. doi: 10.5539/elt.v7n7p131
Ministry of Economy and Planning. (2010). Ninth National Development Plan Saudi Arabia. Riyadh: Ministry of Economy and Planning.
Mutambara, J., and Bhebe, V. (2012). An analysis of the factors affecting students’ adjustment at a university in Zimbabwe. Int. Educ. Stud. 5, 244–260. doi: 10.5539/ies.v5n6p244
Muthén, B. O., and Asparouhov, T. (2008). “Growth mixture modeling: Analysis with non-Gaussian random effects,” in Longitudinal Data Analysis, eds G. Fitzmaurice, M. Davidian, G. Verbeke, and G. Molenberghs (Boca Raton, FL: Chapman & Hall/CRC), 143–165. doi: 10.1201/9781420011579.ch6
Noble, J. (2003). The Effects of Using ACT Composite Score and High School Average on College Admission Decisions For Racial/Ethical Groups. ACT Research Report Series. Iowa City: American Coll. Testing Program doi: 10.1037/e427972008-001
Preacher, K. J., Zyphur, M. J., and Zhang, Z. (2010). A general multilevel SEM framework for assessing multilevel mediation. Psychol. Meth. 15, 209–233. doi: 10.1037/a0020141
Rosseel, Y. (2012). Lavaan: an R package for structural equation modeling. J. Stat. Softw. 48, 1–36. doi: 10.3389/fpsyg.2014.01521
Steiger, J. H. (2007). Understanding the limitations of global fit assessment in structural equation modeling. Pers. Individ. Diff. 42, 893–898. doi: 10.1016/j.paid.2006.09.017
Tsaousis, I., Sideridis, G., and Al-Saawi, F. (2018). Differential Distractor Functioning as a Method for Explaining DIF: the Case of a National Admissions Test in Saudi Arabia. Int. J. Test. 18, 1–26. doi: 10.1080/15305058.2017.1345914
White, H. (1980). A heteroskedasticity-consistent covariance matrix estimator and a direct test for heteroskedasticity. Econometrica 48, 817–838. doi: 10.2307/1912934
Wiley, A. (2014). “Student success in college: How criteria should drive predictor development,” in Proceedings of the First International Conference on Assessment & Evaluation, (Riyadh: The National Center for Assessment in Higher Education).
Yushau, B., and Omar, M. H. (2007). Preparatory year program courses as predictors of first calculus course grade. Math. Comput. Educ. 41, 92–108.
Zwick, R. (2012). The role of admissions test scores, socioeconomic status, and high school grades in predicting college achievement. Pensa. Educ. 49:8.
Keywords: pre-university measures, preparatory year, Saudi Arabia, structural equation modeling, university performance
Citation: Vista A and Alkhadim GS (2022) Pre-university Measures and University Performance: A Multilevel Structural Equation Modeling Approach. Front. Educ. 7:723054. doi: 10.3389/feduc.2022.723054
Received: 23 August 2021; Accepted: 07 February 2022;
Published: 25 February 2022.
Edited by:
Feroze Kaliyadan, Sree Narayana Institute of Medical Sciences, IndiaReviewed by:
Ghaleb Hamad Alnahdi, Prince Sattam Bin Abdulaziz University, Saudi ArabiaShital Bhandary, Patan Academy of Health Sciences, Nepal
Copyright © 2022 Vista and Alkhadim. This is an open-access article distributed under the terms of the Creative Commons Attribution License (CC BY). The use, distribution or reproduction in other forums is permitted, provided the original author(s) and the copyright owner(s) are credited and that the original publication in this journal is cited, in accordance with accepted academic practice. No use, distribution or reproduction is permitted which does not comply with these terms.
*Correspondence: Ghadah S. Alkhadim, Z2hhZGFoLnNAdHUuZWR1LnNh