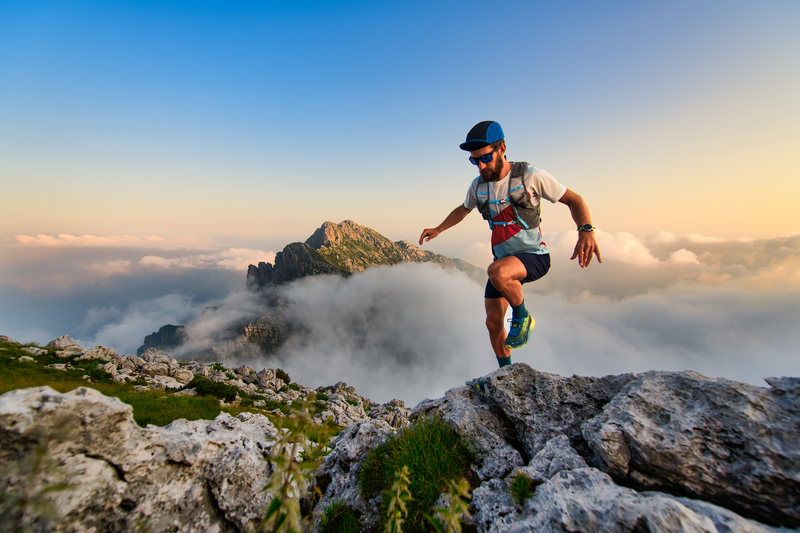
94% of researchers rate our articles as excellent or good
Learn more about the work of our research integrity team to safeguard the quality of each article we publish.
Find out more
ORIGINAL RESEARCH article
Front. Educ. , 15 December 2022
Sec. Teacher Education
Volume 7 - 2022 | https://doi.org/10.3389/feduc.2022.1077989
This article is part of the Research Topic Digitalization in Education: Developing Tools for Effective Learning and Personalisation of Education View all 12 articles
Mobile learning (ML) is extremely relevant to distance teaching. Although much is known about ML usage in teacher education, less is known about crucial points in teachers’ ML adoption process under constraints such as the COVID-19 pandemic. The aim of this exploratory case study was to gain insight into the ML adoption process, including its critical points, by examining teachers’ emotion-related language. This study investigated the emotional response of 32 inservice teachers to Mobile Learning (ML) adoption while attending ML training during the COVID-19 pandemic. The data were collected using semi-structured interviews (10), focus groups (3), and participants’ reflections (96) at five time points. The data underwent multilevel analysis (content and linguistic analyses), revealing two critical stages during the ML adoption process and indicating several factors that may affect the quality of emotional response, thereby promoting or impeding this process. The study highlights the critical sages and their related features that must be addressed to promote optimal ML adoption in teacher education in both routine and emergency conditions.
The importance of encouraging teachers to acquire technopedagogical skills was recognized before the outbreak of the COVID-19 pandemic (Voithofer and Nelson, 2021). Preparing teachers to use technology effectively (Carrillo and Flores, 2020; Lin and Johnson, 2021; Frei-Landau and Avidov-Ungar, 2022) was considered an aspect of teachers’ professional development in the digital age (Tsybulsky and Levin, 2019; Avidov-Ungar and Tsybulsky, 2021; Tsybulsky and Muchnik-Rozanov, 2021). The outbreak of the pandemic has made the transition to distance learning even more urgent, prompting scholars and practitioners to investigate and explicate the processes involved in the adoption and implementation of technology-based teaching, especially in the field of teacher education (Frei-Landau et al., 2022).
The use of digital mobile learning1 (ML), which involves the use of mobile devices, has become increasingly popular (Giannakas et al., 2018; Mahdi, 2018; Zilka, 2019; Özhan and Kocadere, 2020; Xodabande et al., 2022) because of its unique advantages in remote teaching, which include ease of access and affordability. This study aimed to expand our understanding of the ML-adoption process by inservice teachers during the COVID-19 pandemic, by analyzing their emotion-related language. In particular, we sought to explore inservice teachers’ emotional response to adopting ML and identify the factors that affect the quality of emotional responses and thereby either promote or impede this process.
Mobile learning involves the use of mobile computing devices, as an integral component of teaching and learning processes (Grant, 2019; Zilka, 2019; Mohammadi et al., 2020). Through the enrichment of learning environments with multimedia content (Becker et al., 2020), ML provides a varied learning experience (Park et al., 2018), which improves learners’ academic outcomes (Chen et al., 2020; Mitra and Gupta, 2020) and increases their motivation, learning efficacy (Blau, 2019), and learning involvement (Hatun Ataş and Delialioğlu, 2018). Several theoretical frameworks of ML usage have been proposed (Okai-Ugbaje, 2021), among them, “Mobagogy, “which emphasizes the pedagogical benefits of ML implementation in education (Schuck et al., 2013), as well as social collaborative learning (Danish and Hmelo-Silver, 2020), Self-regulated learning (Kramarski and Heaysman, 2021), and self-determination theory (Yang et al., 2019).
The advantages of ML adoption have been well-established, such as its reliance on the highly pervasive smartphone technology, which can help reduce social gaps and inequality in learning (Ilgaz, 2021; Frei-Landau and Avidov-Ungar, 2022), and the ability to adjust and monitor learning through digital applications, which creates a more personalized learning experience (Nedungadi and Raman, 2012); thus, suits different learning styles. These benefits were even more significant when distance learning became a necessity (Eutsler, 2021) during the COVID-19 crisis, resulting in the need to minimize the impact of socioeconomic status and guarantee equitable access to education (UNESCO, 2020; Grogan et al., 2022). However, ML offers a universal form of learning (Aljawarneh, 2020) that can be applied not only in future emergencies but also routinely, providing a solution for learners who are unable to attend face-to-face meetings, even when they cannot gain access to a computer.
Previous studies mostly examined the use of ML in routine times (Lai, 2020), and less than one-third of the existing studies focused on teachers (Baran, 2014). Furthermore, in the majority of studies, the focus was either on identifying aspects that promote or inhibit ML adoption (Moya and Camacho, 2021), participants’ perceptions and attitudes toward ML (Gikas and Grant, 2013), or their intention to adopt ML (Buabeng-Andoh, 2021). However, we have yet to fully understand the critical stages within the process of ML adoption and implementation in teaching (Lai, 2020). This study aims to fill this gap by exploring teachers’ emotions to identify such critical stages.
Awareness of teachers’ emotions is crucial for understanding teaching and teachers (Zembylas, 2003; Korthagen and Vasalos, 2005). In particular, understanding teachers’ emotions associated with the processes of adopting and implementing technology-based teaching is vital given that emotions tend to affect teachers’ beliefs regarding acquiring certain technopedagogical skills (Bandura, 1986). Moreover, it is often teachers’ emotional response to technology that defines their ability to design creative, technology-rich practices (Azzaro and Martínez Agudo, 2018). Few recent studies showed how examining the emotional response evoked along the process of technology adoption can contribute to understanding technology acceptance (Meishar-Tal and Levenberg, 2021). For instance, Holzmann et al. (2020) suggested that high levels of nervousness or discomfort related to using a technology negatively affected teachers’ intention to implement it. According to Straub (2009), since a technology change tends to evoke a particular emotional response, encouraging technology adoption means accepting the possibility of a negative emotional response. Overall, the existing research on emotional aspects of technology adoption and the way in which certain teachers’ emotional responses may affect the acceptance of technology is relatively limited and deals mainly with the feelings of either satisfaction or tediousness experienced when learning in an online setting (Stein and Wanstreet, 2015; Meishar-Tal and Levenberg, 2021; Togaibayeva et al., 2022).
Studies conducted in the higher education context have suggested that teachers’ negative emotional response to the adoption of the latest technology tends to lead to technology’s anxiety, thereby affecting teachers’ adoption of mobile learning (Mac Callum et al., 2014; Dolawattha et al., 2019; Torres, 2022). Bennett (2014) studied lecturers’ emotions associated with integrating new technology into their teaching practices. Although a range of positive emotions was documented, these did not outnumber the negative emotions, such as anxiety, fear, humiliation, and anger. However, to the best of our knowledge, no study has explored the emotional response of teachers to the process of ML adoption during the COVID-19 pandemic, so this work seeks to expand our understanding of this process by analyzing the participants’ emotion-related language to gain an understanding of the critical stages in teachers’ ML adoption.
This study employs the broaden–and–build theory of positive emotions proposed by Fredrickson (2004) to conceptualize the role of positive emotional experiences in the ML-adoption process during the COVID-19 pandemic. According to Fredrickson’s theory, positive emotions can broaden one’s awareness and encourage novelty in thinking and actions even in the face of challenges, thus advancing the building one’s skills and personal resources (Fredrickson, 2001). Moreover, according to the theory, positive emotional experiences create “efficient antidotes for the lingering effects of negative emotions” (Fredrickson, 2000, p. 8).
In the realm of education, the broaden–and–build theory has been employed to explore the role of positive emotions in second language learning, demonstrating the profound effects that positive emotions have on language learners’ motivation, learning, and achievements (Rahimi and Bigdeli, 2014). Another study employing the broaden–and–build theory postulated the impact of positive emotions on teenagers’ engagement in learning at school (Reschly et al., 2008). In higher education context, the broaden–and–build theory has been employed to explore the effects of gratitude intervention on students’ problem solving, anxiety, and psychological well-being (Lee and Chen, 2009), as well as on teachers’ teaching satisfaction (Nalipay et al., 2019). In a more recent study, Sriwidharmanely et al. (2021) suggested that the broaden–and–build theory helps alleviate the negative effects of technostress on technology users’ performance. However, to the best of our knowledge, the broaden–and–build theory has not been employed to study emotional aspects of the ML-adoption process, especially during the COVID-19 pandemic. The current study applies the broaden–and–build theory to understand the critical learning stages in the ML-adoption process during the COVID-19 pandemic, by exploring teachers’ emotional response to it and using two complementary analytic methodologies: linguistic and content analysis. The linguistic analysis helped examine language choices manifested throughout the reflective reports, focus group, and interview data (Trent, 2013; Schieble et al., 2015) made it possible to identify implicitly conveyed messages, while the content analysis served to organize and understand the explicitly conveyed perceptions (Muchnik-Rozanov and Tsybulsky, 2019). Thus, the combination of these two methods provided comprehensive answers to our research questions.
Following Venkatesh et al. (2003), we defined emotional response to the ML-adoption process as individuals’ overall affective reaction (represented in their emotion-related language) to their exposure to and hands-on practice and implementation of ML technology during the training period. Therefore, the research questions were composed as follows:
How did teachers’ emotional response to their experience of ML adoption during the COVID-19 pandemic manifest in their emotion-related language, throughout the different stages?
Based on the teachers’ emotion-related language, which factors are related to the quality of emotional response, and therefore may be perceived as either promoting or impeding the ML-adoption process?
The ML training workshop is a short-term five-stage program, designed in accordance with Rogers’ Diffusion of Innovation Theory (Rogers, 2003) that addresses knowledge, persuasion, decision, implementation, and confirmation. Figure 1 describes the training stages in light of this theory and shows the data collection points. The workshop was held at an academic teacher education college and was attended by experienced teachers. During this training program, the teachers were first introduced to five ML tools and used them as learners. Then they selected a specific tool from among the five and implemented it while teaching their peers. Finally, they used this tool as schoolteachers conducting an ML-based lesson plan. Each of these phases was accompanied by individual and group reflection processes.
Given that the goal of this study was to understand participants’ perceptions of the ML workshop experience, a qualitative approach was deemed most suitable. Employing an exploratory case study design (Yin, 2014) complemented by the use of multiple data sources throughout the five-stage ML-adoption process, afforded us an in-depth view of the explored phenomenon.
Of the 45 teachers enrolled in the ML workshop that was conducted as part of the Master of Education program, 32 gave their informed consent to participate in the study. We chose teachers, as opposed to preservice teachers, considering that the professional literature indicates that at this stage of their career, teachers are more likely to be ready to incorporate technology use into their routine teaching (Russell et al., 2003). The participants taught in kindergarten (28%), elementary school (56%), middle school (6%), and high school (10%) (Participants demographics are presented in Table 1).
The reliance on multiple data sources and different modalities for eliciting the data not only ensured our ability to attain an in-depth understanding of the phenomenon (Bogdan and Biklen, 1998) but also enhanced the study’s trustworthiness, and facilitated cross-validity checks:
We collected written reflections from the participants at three time-points throughout the training. The instructions for preparing the reflections were to freely elaborate about their experiences, feelings, and thoughts, and to describe the aspects that they found most relevant. Overall, 96 teachers’ reflections were reviewed.
The three focus groups were held at Stage 3, after the participants had selected one of the ML tools and had implemented it in a lesson taught to their peers. Each group (consisting of 8–10 participants and lasting approximately 45 min) was told to share experiences and respond to each other’s comments. The group sessions were recorded using the ZOOM platform, and then transcribed and coded.
Using the ZOOM platform, semistructured interviews of approximately 25–50 min were conducted individually with 10 of the participants. Each interviewee was asked to first summarize the learning experience using a metaphor and then describe the overall process. The interviews were videotaped, transcribed, and coded. A summary of data collection modalities and the collection times during the training are shown in Figure 1.
The Ethics Committee of the higher-education institute approved the study plan. After the participants were informed about the study and were told that they could leave at will, they signed informed consent forms. Their personal information was concealed, and the files were kept in a password-protected folder to ensure participants’ anonymity. The focus groups and interviews were conducted by a research assistant, so that the workshop participants would not feel pressured to participate.
The data were analyzed through two sequentially conducted complementary methods: linguistic and qualitative content analyses (Muchnik-Rozanov and Tsybulsky, 2019). The linguistic analysis was conducted by the first author, with a Ph.D. in Linguistics, (using AntConc software). The qualitative content analysis was conducted in two phases using Atlas.ti. Firstly, the analysis was conducted by the first and second author, with a Ph.D. in Psychology. Secondly, the third author, with a Ph.D. in Education, analyzed about 20% of the collected data. The percent agreement between the third author and authors 1 and 2 ranged from 90 to 100%. Trustworthiness was ensured by triangulating the research instruments through which the data were retrieved (i.e., interviews, focus groups, and reflections) and conducting a multilevel analysis. Furthermore, member checks were conducted to further enhance trustworthiness (Kornbluh, 2015).
The theoretical frameworks and approaches underlying the linguistic analysis used in the present study are as follows: In accordance with Holmes et al. (2007) and Tausczik and Pennebaker (2010), speakers’ or writers’ “emotion words” are analyzed, to determine the extent of their emotional involvement when discussing a specific topic. Next, the valence of the emotion words is determined; to further understand participants’ perceptions of various phenomena. Thus, for example, words such as “glad,” “happy,” and “moving” indicate participants’ positive perceptions of phenomena, whereas “cruel,” “hard,” and “neglected” indicate a negative perception of phenomena. Thus, the study of emotion words and the valence attributed to these words provides a profile not only of participants’ involvement in the educational-technological discourse but also of the quality of their emotional experiences, as these developed and shifted throughout the ML-adoption process.
We also used the Systemic Functional Linguistics (SFL) theory (Halliday, 1978) to analyze the participants’ emotionally colored experiences. According to the SFL framework, certain lexical choices and patterns are linguistic indications of emotional experiences (Halliday and Matthiessen, 2004). More specifically, the use of linguistic means of intensification when reflecting on or describing emotionally colored experiences indicates a higher or lower intensity of the emotional response. Thus, intensifications may be “interpersonally neutral, “using words, such as very, much, really, completely, utterly, almost, etc., or may “derive from some interpersonally significant scale,” e.g., amazingly, awfully, unbelievably, perfectly, etc. (Halliday and Matthiessen, 2004, p. 356). Intensification suggests that the experience described was particularly meaningful for the participant (Halliday, 1978; Halliday and Matthiessen, 2004; Kupferberg et al., 2013). Based on this approach, the analysis of intensification was employed to explore the aspects of an ML training workshop that were experienced as meaningful by the participating.
To summarize, the following three categories of linguistic markers were examined:
• The frequency of emotion words (indicating participants’ degree of emotional immersion when discussing or reporting on their ML-adoption experience). Thus, using software AntConc of Anthony (2013) (as per Muchnik-Rozanov and Tsybulsky, 2022), we identified the emotion words. Then, to determine the participants’ emotional involvement in each stage of the ML-adoption workshop, we calculated the number of emotion words in each stage of the process as a percentage of the total amount of words in that stage, and then compared the percentages per stage.
• The valence of emotion words (indicating participants’ attribution of quality—positive or negative—to their emotional response regarding the ML-adoption experience). We identified the valence attributed by the participants to the emotion words they employed and calculated the percentage of positive and negative emotion words relative to the total number of emotion words used in each stage, and then compared the findings per stage.
• The presence of adverbial intensifiers (whereby, the intensity of the participants’ emotional response to an experience indicates that they view that experience as particularly meaningful). To this end, we identified the experiences that were described with the use of intensifiers and interpreted these as developments that were meaningful to the participants’ ML-adoption process.
Overall, 62,455 words were analyzed. The data sources (i.e., participants’ reflective reports, collected at three time-points throughout the training, focus group data, and interview data) were analyzed separately. Table 2 summarizes the distinctive linguistic markers, subcategories, coding methods, and examples.
As a result of the previous, linguistic stage of the data analysis, we identified a change in the teachers’ emotional response in Stages 2 (hand-on experience as learners) and stage 4 (implementing ML as schoolteacher). To understand why emotional changes occurred specifically in these two stages, we used a qualitative content analysis to deepen our understanding of the experiences that were indicated as meaningful during these two critical stages. These meaningful experiences were grouped into two major categories “Experiences positively affecting the quality of emotional response and thereby promoting the adoption of ML” vs. “Experiences negatively affecting the quality of emotional response and thereby impeding the adoption of ML.” The qualitative content analysis was conducted in two phases, using the Atlas.ti. Firstly, the analysis was conducted by the first author, with a Ph.D. in linguistics and experience in conducting content analyses of this kind and second author, with a Ph.D. in Psychology. Reliability and validity were achieved by conducting a careful review of the stages by the two researchers. Each researcher analyzed the data separately, to the best of their knowledge, and then reported the findings in a brainstorming session, which included a discussion that focused on the research questions. In cases of disagreement, the issue was further discussed until full agreement was reached, which included all team members. Secondly, the third author, with a Ph.D. in Education, analyzed about 20% of the collected data. Percent agreement between the third author and authors 1 and 2 ranged from 90 to 100%. Regarding the qualitative content analysis.
This study examined the teachers’ emotional responses during their experience of the ML-adoption process in the context of the COVID-19 pandemic. In the following section, the findings are presented in accordance with the research questions. All names used in the findings section are pseudonyms.
The linguistic analysis of the reflective reports and the focus group data revealed certain changes in the teachers’ degree of emotional immersion. Reflective reports submitted after the ISTs’ familiarization with the tools and their hands-on experience of ML as learners (Stage 2) and after teaching an ML-based lesson in a school classroom as teachers (Stage 4) were characterized by a certain increase in the degree of emotional immersion (compared to the preceding stages, 1 and 3, respectively), as manifested in the greater frequency of emotion words used. By contrast, the analysis of the focus group discussions regarding the peer-teaching experience (Stage 3) revealed a decrease in the degree of emotional immersion, as manifested in the less frequent use of emotion words. Table 3 summarizes the findings regarding the degree of emotional immersion.
The analysis of the data collected at the first four stages revealed a predominantly positive valence of emotion words although both positive and negative emotion words were used. This finding pertains to our understanding of the ML-adoption process overall, as it suggests that the teachers portrayed their emotional perception of the experiences along the process as mostly positive. Additionally, two stages of the ML-adoption process were marked by a change in the ratio between positive and negative emotion words. In Stage 2, as the teachers were getting exposure to the tools and were learning to use them, there was a change in the ratio between positive and negative words (85 and 15, respectively), compared to the ratio in Stage 1 (75 and 25 respectively). Hence, Stage 2 was marked by a surge in positive emotions. The following example illustrates a teacher’s positive emotional response to her experience during the exposure to ML tools, portrayed using positive emotion words and adverbial intensifiers: “Finally, my experience using the tools was positive and extremely interesting.” (Keren). In the example below, Lana portrays a negative emotional response to her experience during the same stage of the ML training by employing a negative emotion word and an adverbial intensifier: “I found using that tool quite difficult” (Lana).
In Stage 3, the ratio of positive to negative emotions was the same as that found in Stage 2 (85 and 15). However, when applying the acquired knowledge to designing and teaching an ML-based lesson to school children (Stage 4), there was a relative surge in negative emotions, as the ratio of positive to negative emotion words nearly coincided with the ratio found at Stage 1 (76 and24). The following sentence, written by Hodaya, illustrates a negative emotional experience related to the stage of implementing ML technology in a classroom, which was depicted using two negative emotion words and an adverbial intensifier: “The Internet connection was extremely slow, which made the students impatient” (Hodaya). It should be noted that despite the surge in negative emotional experiences depicted at this stage of designing and teaching an ML-based lesson to school children, there were nevertheless some positive emotional responses, as the following example demonstrates (note the employment of positive emotion words and an adverbial intensifier).
I was very glad that I used the iPad in the lesson. The children cooperated well, focused on the task, and progressed at their own pace. The lesson was good in terms of the experience, the learning, and the enjoyment it afforded (Dana).
Table 4 summarizes the findings regarding the valence ratios.
Based on the obtained findings, we wanted to understand which factors had influenced the changes in the quality of teachers’ emotional response. To this end, we analyzed the use of intensification in Stages 2 (when the participants were first introduced to the tools and used them as learners) and 4 (when the participants designed and implemented an ML-based lesson plan as schoolteachers). In the analysis of Stage 2, we sought to detect factors that had possibly contributed to the increase in the number of positive emotional responses and found 106 instances of portrayals using intensification which we regarded as marking teachers’ meaningful experiences. In the analysis of Stage 4, we sought to detect factors that had possibly contributed to the increase in the negative (and hence decrease in positive) emotional responses and found 130 instances of intensification. We also analyzed the data from ISTs’ personal interviews (Stage 5), in which participants summarized their experiences of the ML-adoption process, and found 771 instances of portrayals using intensification, which we regarded as marking teachers’ meaningful experiences.
Based on the linguistic analysis, which indicated the participants’ attribution of significance to their descriptions of Stage 2 and Stage 4 experiences, we conducted a content analysis of the verbal data adjacent to emotion-related language found throughout the reflective reports collected during these stages and in the post-program interview data (Stage 5), to identify which experiences the teachers considered meaningful. Five meaningful experiences were revealed. Four of the five experiences were categorized as positively affecting the quality of emotional response and thereby promoting the adoption of ML technology. The teachers described extensive practice using the ML tools during the workshop as a catalyst for implementing ML technology into their teaching practices. In the following quote, the teachers’ experience was perceived as meaningful because of the participant’s use of an adverbial intensifier to describe it: “Thanks to the exposure and experience using digital tools, I began to actually use them in my work with students” (Adva). These findings were further substantiated in the analysis of the personal interviews. In the following quote, Haggit’s use of intensification emphasizes the importance of hands-on practice in the training process: “But when I actually started implementing the newly acquired skills in the classroom, well… then, there is no doubt that it coincides with---with my—apparently suppressed—worldview” (Haggit).
Similarly, the teachers’ use of adverbial intensification portrayed the exposure to innovative teaching approaches as a meaningful experience, which contributed to their positive emotional response. This positive and meaningful experience increased their motivation to implement the ML technology, as the following examples demonstrate: “It’s always fun to learn new tools and teaching methods that integrate well with existing materials and lesson preparations” (Dana); “Overall, I really like the tools because they arouse students’ interest and add color to the lessons. They are pedagogically useful and bring a novel freshness into the teaching” (Etti).
Another aspect of the teachers’ positive emotional response to this stage of the ML-adoption process was associated with feeling curious, interested, and happy as learners during the training. The following quotes illustrate the use of adverbial intensifiers to emphasize the positive quality of the emotional response: “In summary, I’d like to note that, as a learner, these tools really aroused my curiosity” (Etti); “I am very pleased and satisfied with the process I experienced in this course” (Hodaya).
Finally, adverbial intensification was used to also highlight the positive response to experiencing the COVID-19 crisis as a catalyst for digitalization and implementation of the ML technology: “Before the first year of COVID, we had heard about the use of technology, but we did not quite understand what it could do or its many facilitative aspects” (Sarah).
Although Stage 2 was marked by a surge in teachers’ positive emotional responses, experiencing difficulties and fears can be seen as a negative emotional experience that the subjects found meaningful based on its portrayal using adverbial intensification. “Nevertheless, I should mention that for me personally, any new experience makes me really anxious” (Lana). “I am petrified indeed when I have to learn to use an unfamiliar tool” (Adva). These findings were further substantiated by the analysis of the personal interviews, as the following quote demonstrates.
To tell the truth [giggles], I had a kind of phobia…, I was really afraid of technology, and the COVID development threw me into deep waters. I would ask the other teachers to help me set up the ZOOM lesson, to upload, download…I just avoided it all, as much as possible (Sarah).
It appears that experiencing difficulties and fears during Stage 2, when the participants were first introduced to the tools and used them as learners, can be seen as the only factor that negatively affected the quality of emotional response, thereby impeding the ML-adoption process. This factor, however, was outweighed by four types of the teachers’ meaningful experiences that contributed to strengthening the positive emotional response to the ML-adoption process throughout Stage 2.
The stage of applying the acquired knowledge to designing and teaching an ML-based lesson to school children (Stage 4) was marked with a certain surge in negative emotional response and a corresponding decrease in the positive emotional response. Therefore, in reviewing the data collected at Stage 4, we examined all the instances where participants employed linguistic intensification (using adverbial expressions and in one instance a superlative adjective) indicating significant experiences, to identify which factors had contributed to the change in the valence of their emotional responses. Five meaningful experiences were identified. Of these, four were described while indicating a negative emotional response, thereby impeding the adoption of ML technology, and the remaining one was described while conveying a positive effect. As noted, the positive experience associated with implementing ML technology in the classroom was outweighed by four instances of negative experiences that the teachers portrayed as meaningful while reflecting on Stage 4. The documented preponderance of the negative experiences that accounts for the surge in the participants’ negative quality of emotional responses was related to the integration of ML tools into their teaching routine at school. The teachers described the perceived challenge of leaving their comfort zone as a meaningful—albeit negative--emotional experience.
I watched the guidance videos and I tried to complete the tasks during the lesson, but I found them very difficult. I cannot tell if this was because I was afraid, or lacked sufficient technological knowledge, but I could not even seem to connect to these topics or to the idea of creating a game and thinking in a nontraditional way (Keren).
Another meaningful but negative experience that the teachers found challenging and even frustrating and which they described using adverbial intensifiers was related to the transition from theory to practice: “I found it extremely difficult to take it beyond the course and into the classroom where I teach” (Lana); “The initial experience was unnerving and even embarrassing, being in front of the parents and the kids who were looking forward to it, and I could not deliver (Shira).
Another meaningful experience that was described as evoking a negative emotional response to the ML adoption was related to the lack of resources, such as time, equipment, and a stable Internet connection while trying to implement ML technology in the classroom: “The Internet connection was extremely slow, which made the students impatient” (Hodaya); “There was an insufficient number of PCs, tablets, and mobile phones, especially among those from the socioeconomically lower class, and it became extremely difficult to include them in the lesson” (Haggit).
Finally, some teachers’ meaningful experiences while implementing ML technology were related to having a conflict with the institutional policy. One of the teachers described such an experience in the interview as discouraging.
But it also creates some upheaval in the classroom, and the Ministry of Education and the school would prefer to keep things peaceful and quiet, so the upheaval makes a bad impression. Especially, if the lesson is in a classroom located within hearing range of the principal’s office and he comes in to see what the noise is all about. Well, that’s already discouraging enough” (Keren).
These negative but meaningful teaching experiences, i.e., fear of leaving one’s comfort zone, the difficulty in transitioning from theory to practice, the lack of resources, and the clashes with the institutional policy did more than merely evoke a negative emotional response in the teachers: the relative surge in negative emotions during this stage of ML implementation might have impeded the completion of the ML-adoption process.
However, the teachers’ emotional response to teaching an ML-based lesson in class was not entirely negative. Some teachers pointed out a positive experience; specifically, implementing ML in the classroom helped them understand the importance of digitalizing teaching practices: “I want to clarify that I enjoyed every single moment and, based on the feedback from the children and their enthusiastic reactions; I understood that they too enjoyed the outcome very much” (Etti). This positive experience was further substantiated by the interview data:
Their [the students’] desire to participate is truly the best indication. Those who were absent during the lesson …went in on their own to complete the task during their free time after class–well that’s the best feedback; they had fun (Sarah).
Similarly, in the following quote, one of the teachers explains how the experience clarified the importance of the digitalization of teaching practices.
You’re simply speaking their language and so they want to participate…how can I explain it? When students are given a worksheet, they get bored right away. But it’s different when you assign questions to be answered using a colorful and attractive app (Shira).
Figure 2 summarizes the goals, process, and findings of the linguistic and content analyses.
This study examined the teachers’ emotional response throughout their experience of ML-adoption process in the context of the COVID-19 pandemic, in an attempt to identify the critical stages in ML adoption process and its related factors that facilitate or impede this process. The study highlights two critical stages in the ML adoption process in which the participants demonstrated the deepest emotional immersion: (1) after they had been introduced to and had gained extensive practice using the ML tools; and (2) after implementing the use of a particular tool to teach an ML-based lesson plan as schoolteachers. It appears that these two stages are crucial to teachers’ experience of ML adoption; thus, extra attention should be given to these stages when training teachers to implement ML. Respectively, the study has both theoretical and practical contributions. Specifically, the stage of being exposed to ML as a learner, which evokes positive emotions turns out to have a crucial role in establishing teachers’ adoption of ML. Additionally, the stage within which the teacher implements ML as a classroom teacher is also a sensitive stage that may affect teachers’ ML adoption; thus, also requires extra attention to ensure optimal training.
Long before the COVID-19 outburst, teachers had often mentioned technology use as one of their objectives for professional development, and despite an explicit desire to acquire and master new technopedagogical skills, many found implementing these tools into their everyday teaching practices quite challenging (Louws et al., 2017). The COVID-19 pandemic magnified the need to enable teachers to use technology efficiently and confidently (Carrillo and Flores, 2020; Lin and Johnson, 2021). Recent studies on remote teaching during the pandemic crisis have indicated the need for extensive training to implement technology in teaching (Amhag et al., 2019; van der Spoel et al., 2020) and the necessity of adequate teacher training aimed at incorporating technology-based teaching practices for a successful technology adoption process (Baran, 2014; Hadar et al., 2020). Thus, detecting critical stages in this process is essential to designing such adequate training and facilitates best practice, especially in the context of distant learning under crisis as well as in routine conditions.
Deeper emotional immersion documented after implementing a particular tool to teach an ML-based lesson may indicate the magnitude of this newly acquired ability, perceived as a watershed event. On the other hand, implementing an ML tool when teaching their peers (stage 3) was accompanied by a decrease in the degree of emotional immersion. This finding can be attributed to the teachers’ perception of this experience as less crucial and demanding compared to the real-time teaching challenges, which included clashes with institutional policy. Furthermore, our findings regarding the quality of teachers’ emotional response expressed via the valence of emotion words indicated that the participants used positive and negative emotion words to reflect on the ML-adoption process. The predominance of emotion words with the positive valence was documented as reaching its peak during the phase of exposure to the tools as learners. This mainly positive valence indicates that the Teachers viewed these experiences as primarily positive, which, in turn, encourages an open and receptive attitude toward the ML training, in particular, and toward new learning and teaching experiences, in general (Fredrickson, 2001). Positive emotional perception is known to contribute to the development of new skills and personal resources, by being “an efficient antidote” (Fredrickson, 2004, p. 9) to the difficulties and challenges inherent to the process. This finding underscores the importance of teachers being exposed to ML first and foremost as learners to ensure they have experienced positive emotions and gained confidence before attempting to implement ML independently in the classroom.
Based on the broaden–and–build theory, which underscores the quality of emotional response induced by the learning process, the analysis highlighted two critical shifts in the ML-adoption process that were characterized by a change in the emotional response: the stage of gaining hands-on experience as ML learners (Stage 2) and the stage of implementing the acquired knowledge in an ML-based lesson plan as schoolteachers (Stage 4). To clarify, given that the ratio of positive to negative emotion words did not change between Stage 2 and Stage 3, the focus of our analysis was on the positive surge in Stage 2 and the negative surge in Stage 4. A graphic summary of the positive and negative experiences in each of the two meaningful Stages is provided in Figure 3.
Thus, when first exposed to innovative teaching approaches and practicing them as learners, the teachers emphasized that they felt curious and happy while learning. According to Fredrickson’s theory (2001), such a positive emotional response promoted the learning process and thus guided the teachers toward the goal of ML adoption. Furthermore, as the teachers reflected on the stage of familiarizing and acquiring the ML tools, they referred back to their teaching experience prior to the COVID-19 pandemic and before participating in this course. Those experiences had demonstrated the need for and motivated the participants to acquire new technopedagogical skills and overcome difficulties and fears when adopting and implementing the ML technology. The emphasized positive experiences during the initial stage of the ML training were rewarding, eye-opening learning experiences that led the teachers to comprehend the importance of school digitalization. In this regard, our findings corroborate those of Saikat et al. (2021), who suggested that using a variety of online tools and apps can encourage learners to pay attention during the lessons, thereby making teachers’ experiences more positive and rewarding. These meaningful experiences can, in turn, enhance teachers’ motivation to continue implementing ML-based lesson plans, given that teachers’ motivation plays a crucial role in effective technology use in schools (Backfisch et al., 2020).
However, the analysis of teachers’ reflections on their implementation of ML-based lesson plans as schoolteachers revealed a decrease in the positive emotional response at that stage. Understanding the nature of this change is crucial, as it may increase the negative emotional response, which could potentially impede the process of ML adoption overall. This observation is in line with Dolawattha et al. (2019) who found negative attitudes to be among major obstacles for teachers when using technology. An observed decrease in positive emotional response and an increase in the negative indicates that the negative experiences emphasized by the teachers at that stage had a strong influence, outweighing the effect of the positive experiences. These findings corroborate Bennett (2014) who demonstrated that lecturers’ positive emotions reported while integrating new technology into their teaching practices, were insufficient to outnumber a range of their negative emotions. Such negative emotional response to technology adoption can result in technology anxiety and may have a strong negative impact on the future use of technology (Mac Callum et al., 2014). The content analysis revealed that, during this phase (implementation of ML-based lesson plan), extra attention should be given to the following issues: leaving one’s comfort zone, difficulties in transition from theory to practice, and the lack of resources and potential conflicts with the institutional policy. For example, participants mentioned the lack of a stable Internet connection and insufficient resources and/or preparedness, which are already known to constitute major challenges in implementing ML and other distance-learning technologies (Al-Emran, 2020). However, teacher educators involved in the process of the ML training should be aware that implementing new technologies entails extending one’s efforts beyond the habitual comfort zone, an endeavor that calls for emotional exertion. Hence, attending to potential objective obstacles, such as resource availability, may be the factor that determines whether this effort becomes a positive or a negative experience.
The self-reported nature of the data can be seen as a limitation of the present study since the findings may have been affected by social desirability bias. Nevertheless, we believe that both the triangulation of data sources and the fact that the data were collected by an external research assistant helped minimize the chance of bias. Furthermore, the convenience (i.e., availability) rather than random sampling for this study can be seen as a limitation since the sample cannot be seen as representative, which calls for extreme caution while generalizing our findings. Given the increased use of ML and other distance-learning technologies, an examination of the ML-adoption process under routine conditions may further highlight the factors that tend to promote or impede ML adoption for both educators and learners in the post-COVID-19 settings. In addition, certain decontextualization of emotion words could be observed in the linguistic analysis, partially as a result of applying AntConc software. Such decontextualization should be viewed as a limitation of the present study. We recommend conducting linguistic analysis by more than one rater and achieve high interrater reliability to minimize the instances of decontextualization and, thereby, enhance the study reliability. We further recommend comparing teachers’ and students’ portrayal of the ML-adoption process by comparing their descriptions in terms of language behavior. Such a comparison could identify additional aspects that may accompany the process of ML adoption in education and suggest possible challenges and coping strategies. We also recommend comparing the portrayal of the ML-adoption process by teachers of different grade levels to understand whether and in what way the process of ML adoption can be affected by this factor.
The current study contributes to the field in two ways. First, it contributes to the ongoing discourse regarding the ways to promote optimal ML adoption. By examining teachers’ emotional response to the ML training, not only did this study point at t the significance of learners’ emotions to their learning process, but it also identified two critical stages in the process of ML adoption, marked by a shift in the emotional response, thereby highlighting the factors that may promote or impede the learning process. Taking these findings into account can increase the effectiveness of future training programs dedicated to ML adoption. Second, the current work demonstrates the value of employing two complementary methodological approaches, linguistic and content analyses, in educational research. Specifically, examining the emotion-related language may serve as an indicator of critical learning phases that can ultimately guide the sequential content analysis. Given the significant findings, we may conclude that using more than one approach for the data analysis is highly promising in terms of providing a comprehensive picture of the explored educational phenomena.
The datasets presented in this article are not readily available because due to the nature of this research, participants of this study did not agree for their data to be shared publicly, so supporting data is unavailable. Requests to access the datasets should be directed to Uml2aXBzeUBnbWFpbC5jb20=.
The studies involving human participants were reviewed and approved by Ethics Committee of Achva Academic College. The patients/participants provided their written informed consent to participate in this study.
YM-R, RF-L, and OA-U contributed to the design and implementation of the research, the analysis of the results, and writing of the manuscript. All authors contributed to the article and approved the submitted version.
This study was supported by the Chief Scientist, The Ministry of Education, Israel (No. 20.11/23).
The authors declare that the research was conducted in the absence of any commercial or financial relationships that could be construed as a potential conflict of interest.
All claims expressed in this article are solely those of the authors and do not necessarily represent those of their affiliated organizations, or those of the publisher, the editors and the reviewers. Any product that may be evaluated in this article, or claim that may be made by its manufacturer, is not guaranteed or endorsed by the publisher.
1. ^Mobile learning, hereafter: ML;INTW- Interviews.FG- Focus Group.
Al-Emran, M. (2020). Mobile learning during the era of COVID-19. Rev. Virt. Univ. Catól. Norte 61, 1–2. doi: 10.35575/rvucn.n61a1
Aljawarneh, S. A. (2020). Reviewing and exploring innovative ubiquitous learning tools in higher education. J. Comput. High. Educ. 32, 57–73. doi: 10.1007/s12528-019-09207-0
Amhag, L., Hellström, L., and Stigmar, M. (2019). Teacher educators’ use of digital tools and needs for digital competence in higher education. J. Digit. Learn. Teach. Educ. 35, 203–220. doi: 10.1080/21532974.2019.1646169
Anthony, L. (2013). A critical look at software tools in corpus linguistics. Linguistic Res 30, 141–161. doi: 10.17250/khisli.30.2.201308.001
Avidov-Ungar, O., and Tsybulsky, D. (2021). Shaping Teachers' perceptions of their role in the digital age through participation in an online PBL-based course. Electron. J. e-Learn. 19, pp186–pp198. doi: 10.34190/ejel.19.3.2300
Azzaro, G., and Martínez Agudo, J. D. D. (2018). “The emotions involved in the integration of ICT into L2 teaching: emotional challenges faced by L2 teachers and implications for teacher education,” in Emotions in Second Language Teaching (Cham: Springer), 183–203.
Backfisch, I., Lachner, A., Hische, C., Loose, F., and Scheiter, K. (2020). ‘Professional knowledge or motivation? Investigating the role of teachers’ expertise on the quality of technology-enhanced lesson plans. Learn. Instr. 66:101300. doi: 10.1016/j.learninstruc.2019.101300
Baran, E. (2014). A review of research on mobile learning in teacher education. J. Educ. Technol. Soc. 17, 17–32.
Becker, S., Klein, P., Gößling, A., and Kuhn, J. (2020). Using mobile devices to enhance inquiry-based learning processes. Learn. Instr. 69:101350. doi: 10.1016/j.learninstruc.2020.101350
Bennett, L. (2014). Putting in more: emotional work in adopting online tools in teaching and learning practices. Teach. High. Educ. 19, 919–930. doi: 10.1080/13562517.2014.934343
Blau, I. (2019). “Real-time mobile assessment of learning: insights from an experiment with middle school students from remedial, mainstream, and excellence tracks,” in Mobile Technologies in Educational Organizations (Hershey: IGI Global), 283–301.
Bogdan, R. C., and Biklen, S. K. (1998). Qualitative Research in Education: An Introduction to Theory and Methods. Boston, MA: Pearson.
Buabeng-Andoh, C. (2021). Exploring university students’ intention to use mobile learning: a research model approach. Educ. Inf. Technol. 26, 241–256. doi: 10.1007/s10639-020-10267-4
Carrillo, C., and Flores, M. A. (2020). COVID-19 and teacher education: a literature review of online teaching and learning practices. Eur. J. Teach. Educ. 43, 466–487. doi: 10.1080/02619768.2020.1821184
Chen, Z., Chen, W., Jia, J., and An, H. (2020). The effects of using mobile devices on language learning: a meta-analysis. Educ. Technol. Res. Dev. 68, 1769–1789. doi: 10.1007/s11423-020-09801-5
Danish, J., and Hmelo-Silver, C. E. (2020). On activities and affordances for mobile learning. Contemp. Educ. Psychol. 60:101829. doi: 10.1016/j.cedpsych.2019.101829
Dolawattha, D. D. M., Pramadasa, H. K., and Jayaweera, P. M. (2019). The impact model: Teachers' Mobile learning adoption in higher education. Int. J. Educ. Dev. Using Inf. Commun. Technol. 15, 71–88.
Eutsler, L. (2021). Pandemic-induced remote learning increases need for mobile game-based learning to engage learners. Educ. Technol. Res. Dev. 69, 185–188. doi: 10.1007/s11423-020-09861-7
Fredrickson, B. L. (2000). Cultivating positive emotions to optimize health and well-being. Prev. Treat. 3:1a. doi: 10.1037/1522-3736.3.1.31a
Fredrickson, B. L. (2001). The role of positive emotions in positive psychology: the broaden–and–build theory of positive emotions. Am. Psychol. 56, 218–226. doi: 10.1037/0003-066X.56.3.218
Fredrickson, B. L. (2004). ‘The broaden–and–build theory of positive emotions’, philosophical transactions of the Royal Society of London. Philos. Trans. R. Soc. Lond. B Biol. Sci. 359, 1367–1377. doi: 10.1098/rstb.2004.1512
Frei-Landau, R., and Avidov-Ungar, O. (2022). Educational equity amidst COVID-19: exploring the online learning challenges of Bedouin and Jewish female preservice teachers in Israel. Teach. Teach. Educ. 111:103623. doi: 10.1016/j.tate.2021.103623
Frei-Landau, R., Muchnik-Rozanov, Y., and Avidov-Ungar, O. (2022). Using Rogers' diffusion of innovation theory to conceptualize the mobile-learning adoption process in teacher education in the COVID-19 era. Educ. Inf. Technol. doi: 10.1007/s10639-022-11148-8
Giannakas, F., Kambourakis, G., Papasalouros, A., and Gritzalis, S. (2018). A critical review of 13 years of mobile game-based learning. Educ. Technol. Res. Dev. 66, 341–384. doi: 10.1007/s11423-017-9552-z
Gikas, J., and Grant, M. M. (2013). Mobile computing devices in higher education: student perspectives on learning with cellphones, smartphones & social media. Internet High. Educ. 19, 18–26. doi: 10.1016/j.iheduc.2013.06.002
Grant, M. M. (2019). Difficulties in defining mobile learning: analysis, design characteristics, and implications. Educ. Technol. Res. Dev. 67, 361–388. doi: 10.1007/s11423-018-09641-4
Grogan, M., Young, M. D., and Byrne-Jiménez, M. (2022). Education leadership and the COVID-19 crisis. Front. Educ. 7:838313. doi: 10.3389/feduc.2022.838313
Hadar, L. L., Ergas, O., Alpert, B., and Ariav, T. (2020). Rethinking teacher education in a VUCA world: student teachers’ social-emotional competencies during the Covid-19 crisis. Eur. J. Teach. Educ. 43, 573–586. doi: 10.1080/02619768.2020.1807513
Halliday, M. A. K. (1978). Language as Social Semiotic: The Social Interpretation of Language Meaning. London: Edward Arnold.
Halliday, M. A., and Matthiessen, C. M. I. M. (2004). An Introduction to Functional Grammar. 3rd Edn. London: Edward Arnold.
Hatun Ataş, A., and Delialioğlu, Ö. (2018). A question-answer system for mobile devices in lecture-based instruction: a qualitative analysis of student engagement and learning. Interact. Learn. Environ. 26, 75–90. doi: 10.1080/10494820.2017.1283331
Holmes, D., Alpers, G. W., Ismailji, T., Classen, C., Wales, T., Cheasty, V., et al. (2007). Cognitive and emotional processing in narratives of women abused by intimate partners. Viol. Against Women 13, 1192–1205. doi: 10.1177/1077801207307801
Holzmann, P., Schwarz, E. J., and Audretsch, D. B. (2020). Understanding the determinants of novel technology adoption among teachers: the case of 3D printing. J. Technol. Transf. 45, 259–275. doi: 10.1007/s10961-018-9693-1
Ilgaz, H. (2021). The role of mobile technologies in shifting to digital. Educ. Technol. Res. Dev. 69, 189–190. doi: 10.1007/s11423-021-09945-y
Kornbluh, M. (2015). Combatting challenges to establishing trustworthiness in qualitative research. Qual. Res. Psychol. 12, 397–414. doi: 10.1080/14780887.2015.1021941
Korthagen, F., and Vasalos, A. (2005). Levels in reflection: core reflection as a means to enhance professional growth. Teach. Teach. 11, 47–71. doi: 10.1080/1354060042000337093
Kramarski, B., and Heaysman, O. (2021). A conceptual framework and a professional development model for supporting teachers’“triple SRL–SRT processes” and promoting students’ academic outcomes. Educ. Psychol. 56, 298–311. doi: 10.1080/00461520.2021.1985502
Kupferberg, I., Gilat, I., Dahan, E., and Doron, A. (2013). Exploring the discursive positioning of a schizophrenic inpatient via method triangulation. Int J Qual Methods 12, 20–38. doi: 10.1177/160940691301200108
Lai, C. L. (2020). Trends of mobile learning: a review of the top 100 highly cited papers. Br. J. Educ. Technol. 51, 721–742. doi: 10.1111/bjet.12884
Lee, H. M., and Chen, M. T. (2009). The effects of grateful learning intervention program on college students: test of broaden–and–build theory hypothesis. Curric. Instruct. Q. 12, 107–134.
Lin, L., and Johnson, T. (2021). Shifting to digital: informing the rapid development, deployment, and future of teaching and learning. Educ. Technol. Res. Dev. 69, 1–5. doi: 10.1007/s11423-021-09960-z
Louws, M. L., Meirink, J. A., van Veen, K., and van Driel, J. H. (2017). Teachers' self-directed learning and teaching experience: what, how, and why teachers want to learn. Teach. Teach. Educ. 66, 171–183. doi: 10.1016/j.tate.2017.04.004
Mac Callum, K., Jeffrey, L., and Kinshuk, (2014). Factors impacting teachers adoption of mobile learning. J. Info. Technol. Educ. Res. 13, 142–162. doi: 10.28945/1970
Mahdi, H. S. (2018). Effectiveness of mobile devices on vocabulary learning: a meta-analysis. J. Educ. Comput. Res. 56, 134–154. doi: 10.1177/0735633117698826
Meishar-Tal, H., and Levenberg, A. (2021). In times of trouble: higher education lecturers' emotional reaction to online instruction during COVID-19 outbreak. Educ. Inf. Technol. 26, 7145–7161. doi: 10.1007/s10639-021-10569-1
Mitra, S., and Gupta, S. (2020). Mobile learning under personal cloud with a virtualization framework for outcome-based education. Educ. Inf. Technol. 25, 2129–2156. doi: 10.1007/s10639-019-10043-z
Mohammadi, M., Sarvestani, M. S., and Nouroozi, S. (2020). Mobile phone use in education and learning by faculty members of technical-engineering groups: concurrent mixed methods design. Front. Educ. 5:16. doi: 10.3389/feduc.2020.00016
Moya, S., and Camacho, M. (2021). Identifying the key success factors for the adoption of mobile learning. Educ. Inf. Technol. 26, 3917–3945. doi: 10.1007/s10639-021-10447-w
Muchnik-Rozanov, Y., and Tsybulsky, D. (2019). Toward understanding the language of student teachers reflections in the context of professional identity development. Reflective Pract. 20, 520–532. doi: 10.1080/14623943.2019.1642188
Muchnik-Rozanov, Y., and Tsybulsky, D. (2022). “Linguistic analysis of science Teachers’ narratives using ant Conc software,” in Research Anthology on Applied Linguistics and Language Practices (Hershey: IGI Global), 665–684.
Nalipay, M. J. N., Mordeno, I. G., and Frondozo, C. E. (2019). Implicit beliefs about teaching ability, teacher emotions, and teaching satisfaction. Asia Pac. Educ. Res. 28, 313–325. doi: 10.1007/s40299-019-00467-z
Nedungadi, P., and Raman, R. (2012). A new approach to personalization: integrating e-learning and m-learning. Educ. Technol. Res. Dev. 60, 659–678. doi: 10.1007/s11423-012-9250-9
Okai-Ugbaje, S. (2021). Toward a pedagogical and sociotechnical framework for the strategic integration of mobile learning in higher education in low and middle-income countries. High. Educ. Res. Dev. 40, 581–598. doi: 10.1080/07294360.2020.1773768
Özhan, Ş. Ç., and Kocadere, S. A. (2020). The effects of flow, emotional engagement, and motivation on success in a gamified online learning environment. J. Educ. Comput. Res. 57, 2006–2031. doi: 10.1177/0735633118823159
Park, S. Y., Lee, H. D., and Kim, S. Y. (2018). South Korean university students’ mobile learning acceptance and experience based on the perceived attributes, system quality and resistance. Innov. Educ. Teach. Int. 55, 450–458. doi: 10.1080/14703297.2016.1261041
Rahimi, A., and Bigdeli, R. A. (2014). The broaden–and–build theory of positive emotions in second language learning. Procedia Soc. Behav. Sci. 159, 795–801. doi: 10.1016/j.sbspro.2014.12.451
Reschly, A. L., Huebner, E. S., Appleton, J. J., and Antaramian, S. (2008). Engagement as flourishing: the contribution of positive emotions and coping to adolescents' engagement at school and with learning. Psychol. Sch. 45, 419–431. doi: 10.1002/pits.20306
Russell, M., Bebell, D., O'Dwyer, L., and O'Connor, K. (2003). Examining teacher technology use: implications for preservice and inservice teacher preparation. J. Teach. Educ. 54, 297–310. doi: 10.1177/0022487103255985
Saikat, S., Dhillon, J. S., Wan Ahmad, W. F., and Jamaluddin, R. A. (2021). A systematic review of the benefits and challenges of mobile learning during the COVID-19 pandemic. Educ. Sci. 11:459. doi: 10.3390/educsci11090459
Schieble, M., Vetter, A., and Meacham, M. (2015). A discourse analytic approach to video analysis of teaching: Aligning desired identities with practice. J. Teach. Educ. 66, 245–260.
Schuck, S., Aubusson, P., Kearney, M., and Burden, K. (2013). Mobilising teacher education: a study of a professional learning community. Teach. Dev. 17, 1–18. doi: 10.1080/13664530.2012.752671
Sriwidharmanely, S., Sumiyana, S., Mustakini, J. H., and Nahartyo, E. (2021). Encouraging positive emotions to cope with technostress’s adverse effects: insights into the broaden–and–build theory. Behav. Inform. Technol. 41, 2201–2214. doi: 10.1080/0144929X.2021.1955008
Stein, D. S., and Wanstreet, C. E. (2015). Discussions in online and blended learning. Assess. Online Blend. Learn. Environ. 253–267.
Straub, E. T. (2009). Understanding technology adoption: theory and future directions for informal learning. Rev. Educ. Res. 79, 625–649. doi: 10.3102/0034654308325896
Tausczik, Y. R., and Pennebaker, J. W. (2010). The psychological meaning of words: LIWC and computerized text analysis methods. J. Lang. Soc. Psychol. 29, 24–54. doi: 10.1177/0261927X09351676
Togaibayeva, A., Ramazanova, D., Yessengulova, M., Yergazina, A., Nurlin, A., and Shokanov, R. (2022). Effect of Mobile learning on students’ satisfaction, perceived usefulness, and academic performance when learning a foreign language. Front. Educ. 7:946102. doi: 10.3389/feduc.2022.946102
Torres, K. M. (2022). “Disrupting the college classroom experience: avoiding technology pitfalls,” in Digital Distractions in the College Classroom (Hershey: IGI Global), 223–242.
Trent, J. (2013). From learner to teacher: Practice, language, and identity in a teaching practicum. Asia-Pac. J. Teach. Educ. 41, 426–440.
Tsybulsky, D., and Levin, I. (2019). Science teachers worldviews in the age of the digital revolution: structural and content analysis. Teach. Teach. Educ. 86:102921. doi: 10.1016/j.tate.2019.102921
Tsybulsky, D., and Muchnik-Rozanov, Y. (2021). Worldviews of science teachers in educational-technological context as a key factor in digitalization of teaching practices. Israel Sci. Found. Gateway 10:71. doi: 10.12688/f1000research.28074.3
UNESCO (2020). COVID-19--educational disruption and response, 29.3.20. Available at: https://en.unesco.org/news/covid-19-educational-disruption-and-response
van der Spoel, I., Noroozi, O., Schuurink, E., and van Ginkel, S. (2020). Teachers’ online teaching expectations and experiences during the COVID19-pandemic in the Netherlands. Eur. J. Teach. Educ. 43, 623–638. doi: 10.1080/02619768.2020.1821185
Venkatesh, V., Morris, M. G., Davis, G. B., and Davis, F. D. (2003). User acceptance of information technology: toward a unified view. MIS Q. 27, 425–478. doi: 10.2307/30036540
Voithofer, R., and Nelson, M. J. (2021). Teacher educator technology integration preparation practices around TPACK in the United States. J. Teach. Educ. 72, 314–328. doi: 10.1177/0022487120949842
Xodabande, I., Iravi, Y., Mansouri, B., and Matinparsa, H. (2022). Teaching academic words with digital flashcards: investigating the effectiveness of Mobile-assisted vocabulary learning for university students. Front. Psychol. 13:893821. doi: 10.3389/fpsyg.2022.893821
Yang, S., Zhou, S., and Cheng, X. (2019). Why do college students continue to use mobile learning? Learning involvement and self-determination theory. Br. J. Educ. Technol. 50, 626–637. doi: 10.1111/bjet.12634
Zembylas, M. (2003). Emotions and teacher identity: a poststructural perspective. Teach. Teach. 9, 213–238. doi: 10.1080/13540600309378
Keywords: mobile-learning, teacher education, COVID-19, emotional response, linguistic analysis
Citation: Muchnik-Rozanov Y, Frei-Landau R and Avidov-Ungar O (2022) Mobile-learning adoption in teacher education amidst COVID-19: Identifying two critical stages by exploring teachers’ emotions. Front. Educ. 7:1077989. doi: 10.3389/feduc.2022.1077989
Received: 23 October 2022; Accepted: 23 November 2022;
Published: 15 December 2022.
Edited by:
Manpreet Kaur Bagga, Partap College of Education, IndiaReviewed by:
Ana Remesal, University of Barcelona, SpainCopyright © 2022 Muchnik-Rozanov, Frei-Landau and Avidov-Ungar. This is an open-access article distributed under the terms of the Creative Commons Attribution License (CC BY). The use, distribution or reproduction in other forums is permitted, provided the original author(s) and the copyright owner(s) are credited and that the original publication in this journal is cited, in accordance with accepted academic practice. No use, distribution or reproduction is permitted which does not comply with these terms.
*Correspondence: Yulia Muchnik-Rozanov, eXVsaWFtdWNobmlrQGdtYWlsLmNvbQ==
Disclaimer: All claims expressed in this article are solely those of the authors and do not necessarily represent those of their affiliated organizations, or those of the publisher, the editors and the reviewers. Any product that may be evaluated in this article or claim that may be made by its manufacturer is not guaranteed or endorsed by the publisher.
Research integrity at Frontiers
Learn more about the work of our research integrity team to safeguard the quality of each article we publish.