- Department of Educational and Counseling Psychology, University at Albany, Albany, NY, United States
Certain quantification techniques may be more appropriate than others for single-case design analysis depending on the research questions, the data or graph characteristics, and other desired features. The purpose of this study was to introduce a newly developed and empirically validated user-friendly tool to assist researchers in choosing and justifying single-case design quantification techniques. A total of sixteen different quantification techniques and nine facets (research questions, data or graph characteristics, or desired features) that may affect the appropriateness of a single-case experimental design quantification technique were identified to be included in the tool. The resulting tool provides a ranking of recommended quantification techniques, from most to least appropriate, depending on the user input. A pretest and posttest design was utilized to test the effectiveness of the tool amongst 25 participants. The findings revealed that those who use single-case designs may need support in choosing and justifying their use of quantification techniques. Those who utilize single-case designs should use the developed tool (and other tools) to assist with their analyses. The open-source tool can be downloaded from osf.io/7usbj/.
Introduction
A single-case experimental design1 (SCED) is a research design that allows repeated measures of the dependent variable within one case (What Works Clearinghouse, 2020). The dependent variable is measured at different sequential moments in time and across different levels of the independent variable (i.e., treatment indicator), which are also known as “phases” (e.g., baseline and treatment phase). The case serves as its own control rather than having multiple participants in an experimental or control group, as is typical for group comparison designs. The goal of using SCEDs is to find evidence for a functional relation (experimental control) between the researcher-manipulated independent variable and the dependent variable of interest. Two key features of SCEDs are replication and randomization, as these help establish internal and external validity (Horner et al., 2005). Replication occurs when there are multiple opportunities to measure the effect of the independent variable on the dependent variable; this can occur within participants, between participants, or both. Randomization can enhance the internal validity of SCEDs by ensuring that any results are caused by the intervention and not caused by confounding or underlying variables. Randomization prevents experimenter bias because the introduction of the intervention is pre-determined.
Single-case experimental designs (SCEDs) have several benefits over group comparison designs, where inferences are made at the group level rather than at the individual level. SCEDs allow detailed information to emerge about a single case, which can be useful in fields such as behavior modification (Shadish and Sullivan, 2011) when the problem of interest (e.g., the behavior) occurs at an infrequent rate and/or it is difficult to find a large number of participants (Shadish, 2014). SCEDs can have a high degree of internal validity when they are well-designed (What Works Clearinghouse, 2020). Although SCEDs can be time consuming, as repeated data points need to be taken over time, they can be more cost effective than group designs, which require a larger sample size (Alnahdi, 2013). Similarly, they can be used for pilot studies before attempting a larger scale experiment using a group comparison design (Shadish, 2014).
Due to the unique benefits of SCEDs, researchers have used this research design for over 60 years (Horner et al., 2005). Especially since the turn of the century in 2000, there has been an increase in utilization of SCEDs for research in the field of psychology, as shown in Figure 1.
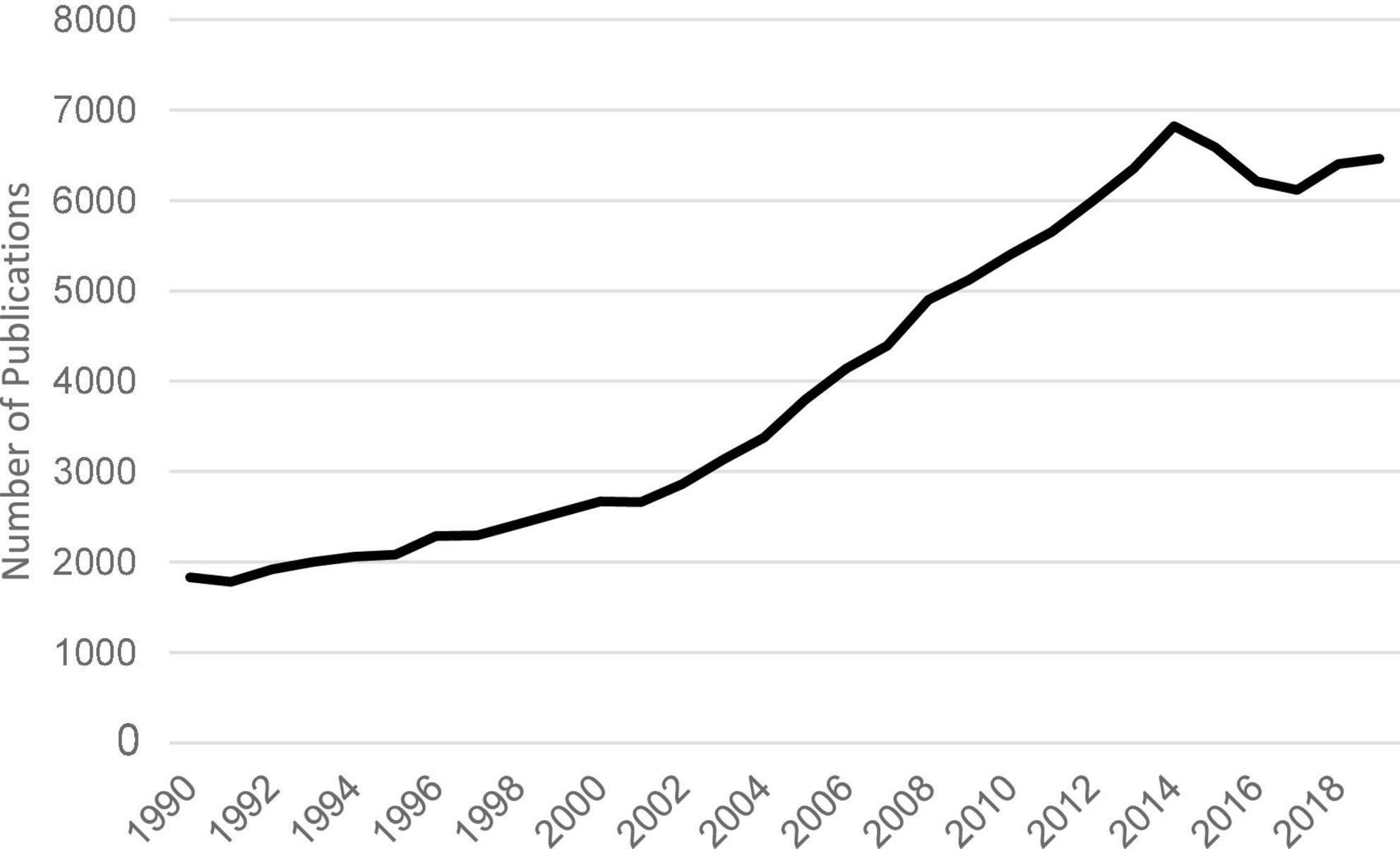
Figure 1. Number of SCED related publications between 1990 and 2019. Increase in the number of publications between 1990 and 2019 when searching terms “single subject” OR “single-subject” OR “single case” OR “single-case” OR “multiple baseline” OR “multiple-baseline” OR “reversal design” OR “ABAB design” OR “withdrawal design” OR “alternating treatment design” on PsychINFO database.
As SCEDs have become more popular, there has been an increased interest in the use of SCED quantitative analysis techniques to use complementary with visual analysis. Certain U.S. federal government laws [e.g., Individuals with Disabilities Education Act [IDEA], 2004] were enacted that called for the use of evidence-based practices, and so researchers who used SCEDs needed to ensure that they could quantitatively analyze the outcomes from the data (Solomon et al., 2015). Furthermore, there has been an increase in outside pressure from evidence-based practice communities to use statistics that can also be understood by group comparison design researchers (Shadish, 2014; What Works Clearinghouse, 2020). Shadish (2014) also acknowledged pressure from journals and publishing groups to quantify SCED results, and that editors from journals such as the Journal of School Psychology and School Psychology Quarterly have expressed the desire for more researchers to use quantitative analyses with SCEDs.
As a result of this increase in pressure to quantify SCED outcomes, many different statistical analysis techniques for SCEDs have been developed, including non-overlap indices (e.g., percent of non-overlapping data, Scruggs et al., 1987; improvement rate difference, Parker et al., 2009; non-overlap of all pairs, Parker and Vannest, 2009; Tau-U, Parker et al., 2011; baseline corrected Tau-U; Tarlow, 2017), regression-based effect sizes (e.g., Center et al., 1985-1986), the standardized mean difference (Hedges et al., 2012), the log-response ratio and related statistics (e.g., percent of goal obtained, Ferron et al., 2020).2 However, there remains a gap in knowledge as to how to select an appropriate quantification technique to reflect intervention effectiveness, as well as how to report these quantification techniques appropriately (Solomon et al., 2015). Furthermore, researchers rarely report their justification for the quantification technique that they use (Solomon et al., 2015), making it difficult to evaluate the appropriateness of the chosen technique. This can contribute to the improper use of these quantification techniques.
Many online calculators and other applications have been developed to assist in SCED calculations and selection (e.g., Baseline Corrected Tau Calculator, Tarlow, 2016; Single Case Research Calculator, Vannest et al., 2016; Tau Decision Chart, Fingerhut et al., 2021a). Other researchers have focused on improving the way that the quality and outcomes of SCEDs are assessed (e.g., Single-Case Analysis and Review Framework; Ledford et al., 2016) and reported (Single-Case Reporting Guideline in Behavioral Interventions; Tate et al., 2016). However, researchers may still benefit from using a tool that helps them systematically determine the best quantification technique to use depending on the research questions, data characteristics, and desired features.
The first purpose of this study is to introduce an empirically validated and user-friendly tool to help applied SCED researchers choose and justify their use of appropriate SCED quantification techniques. The second purpose of this study is to test the effectiveness of the tool in assisting users to choose an appropriate SCED quantification technique and provide a justification for the quantification technique. A third aim of the study is to determine the social validity of the tool (i.e., acceptance amongst applied researchers). Thus, the research questions and hypotheses for this study are as follows:
1. Given an AB graph/data set and research question, is a tool for single-case quantification effective in assisting single-case researchers to choose an appropriate quantification technique? It was hypothesized that a tool for single-case quantification causes a statistically significant change in single-case researcher’s ability to select an appropriate quantification technique.
2. Given an AB graph/data set and research question, is a newly developed tool for single-case quantification effective in assisting single-case researchers to provide appropriate justifications for using a quantification technique? It was hypothesized that a tool for single-case quantification causes a statistically significant change in single-case researcher’s ability to provide appropriate justifications for using a quantification technique.
3. Will single-case researchers find a newly developed tool for single-case quantification technique selection to be socially valid? It was hypothesized that the tool for single-case quantification has high social validity (i.e., most single-case researchers report the tool to be at least “somewhat useful”).
Materials and methods
Instrument
Sixteen different quantification techniques and nine different facets were identified to be included in a tool to help researchers choose and justify their use of SCED quantification techniques. These selected quantification techniques and components are based upon previous research about SCED quantification techniques (e.g., Manolov and Moeyaert, 2017; Manolov et al., 2022). Manolov and Moeyaert (2017) provided a comprehensive overview of the SCED quantification techniques available for use, along with various criterion that must be met for the technique to be considered both appropriate and useful. Manolov et al. (2022) built upon this review, while including more newly developed quantification techniques (e.g., log-response ratio; Pustejovsky, 2018). Manolov et al. (2022) proposed different dimensions and facets that can be used for justifying and selecting a proper quantification technique. Readers are advised to reference Table 1 of their article for details of these dimensions and facets.
The sixteen different quantification techniques that were identified for the tool based upon the previous research were: baseline-corrected Tau-U (Tarlow, 2017), generalized least squares regression (e.g., Center et al., 1985-1986), Hedge’s g for single-case (Hedges et al., 2012), improvement rate difference (Parker et al., 2009), log-response ratio (Pustejovsky, 2018), mean baseline reduction (e.g., Hershberger et al., 1999), mean phase difference (Manolov and Solanas, 2013), non-overlap of all pairs (Parker and Vannest, 2009), percent of goal obtained (Ferron et al., 2020), percentage of all non-overlapping data (Parker et al., 2007), percentage of data exceeding the median of the baseline phase (Ma, 2006), percentage of non-overlapping data points (Scruggs et al., 1987), percentage of data points exceeding the median trend (Wolery et al., 2010), percentage of zero data (Scotti et al., 1991), Tau-U (Parker et al., 2011), and Tau-U Trend A (Parker et al., 2011). The focus of the current study is to evaluate researchers’ ability to choose the most appropriate quantification technique for one case rather than across-cases, so between-case methods such as hierarchical linear modeling (Van den Noortgate and Onghena, 2008; Moeyaert et al., 2014) and between-case standardized mean difference (Pustejovsky et al., 2014) are not included.
Nine facets were identified for inclusion in the tool, based upon the previous research. These nine facets were identified in Manolov et al. (2022) as having an influence on the suitability of an analysis technique to quantify intervention effectiveness. These facets can be categorized within three broader categories, namely “research questions,” “data or graph characteristics,” and “desired features.” Although other facets exist, for the purpose of creating the tool the number of facets was limited to these nine. The four “research questions” included in the tool are: (a) What is the magnitude of change in level?, (b) What is the magnitude of change in linear trend?, (c) What is the magnitude of change in variability?, and (d) What is the amount of non-overlapping data points? The second category “data or graph characteristics” includes: (a) small number of data points in the baseline or treatment phase, (b) trend in baseline, (c) outliers, and (d) within-case variability. The third category, “desired features,” includes only one facet: a p-value to test for statistical significance. Although there are other research questions, data characteristics, and desired features that may affect appropriateness of quantification technique (e.g., autocorrelation, if the quantification technique produces a standardized or unstandardized outcome, etc.), other facets were not included because they are beyond the skill set of an applied researcher. Other facets may be added into a later version of the tool pending results from this current study (i.e., positive feedback indicating that all included facets were understood).
The tool was created in an Excel document. The user can input “yes” or “no” to each of these nine facets, and based on the user input, the tool determines the most appropriate quantification technique for use. For example, if the facet is “trend” (i.e., “Is there baseline trend?”), the user inputs “yes” if the user wants to find a quantification technique that can account for baseline trend/is robust against baseline trend. For example, referring to Figure 2, the two facets are (a) research question: What is the amount of non-overlapping data points? and (b) data characteristic: trend in baseline. Thus, using the input (outlined in red in Figure 3), all inputs would be set to “no” except for “overlap/data separation between phases” and “baseline trend.” The input would be set to “yes” for these two facets. The output then automatically updates and displays the ranked quantification techniques in order of appropriateness. Referring to Figure 3, baseline-corrected Tau-U is ranked first and thus most recommended, followed second by percentage of data exceeding the median trend and Tau-U Trend A, and the third most appropriate quantification techniques are found next (i.e., improvement rate difference, non-overlap of all pairs, and percentage of non-overlapping data).
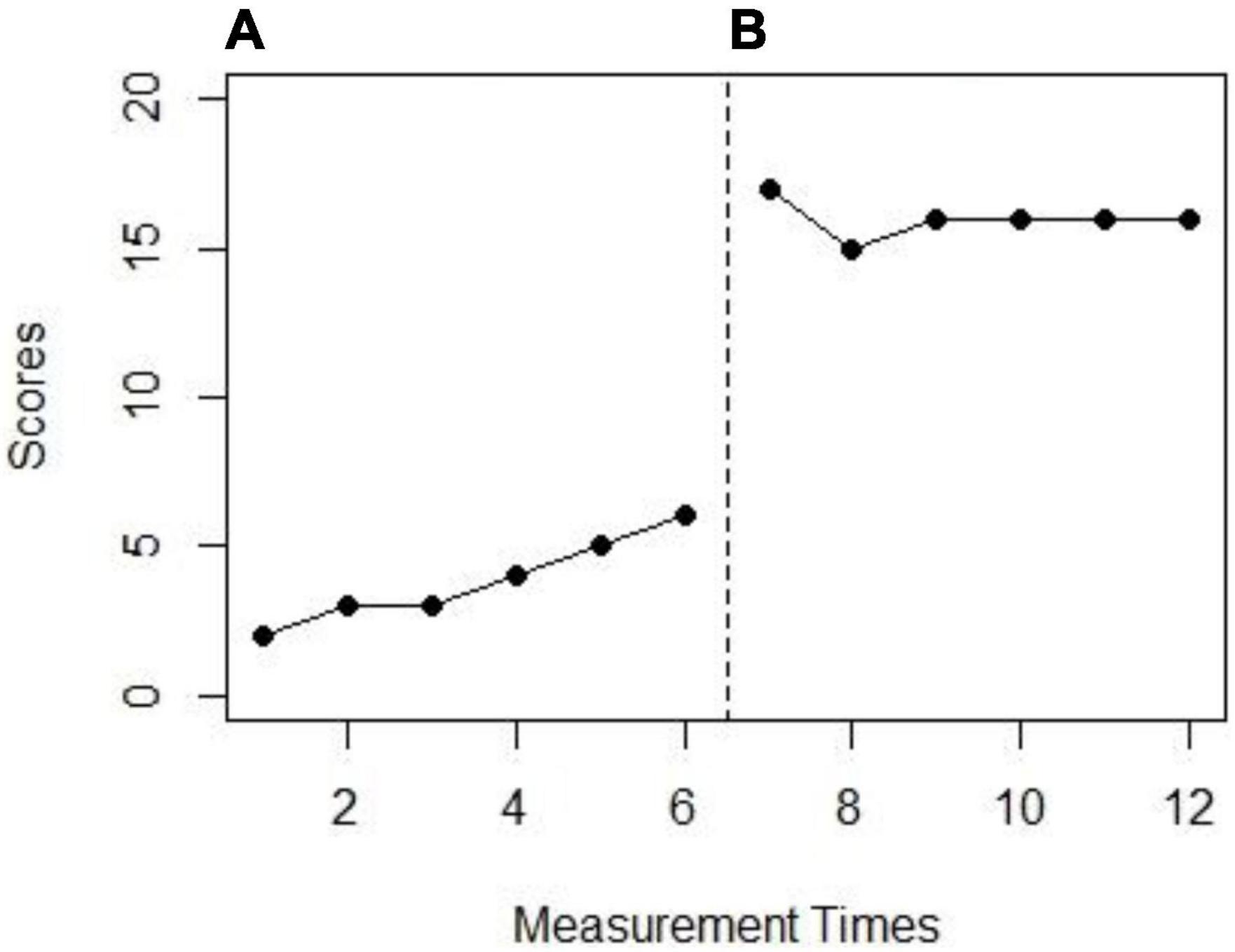
Figure 2. Example (A,B) graph with two facets (Data characteristic: baseline trend and research question: Non-overlapping data). Research question: What is the amount of non-overlapping data between phases (A,B)?
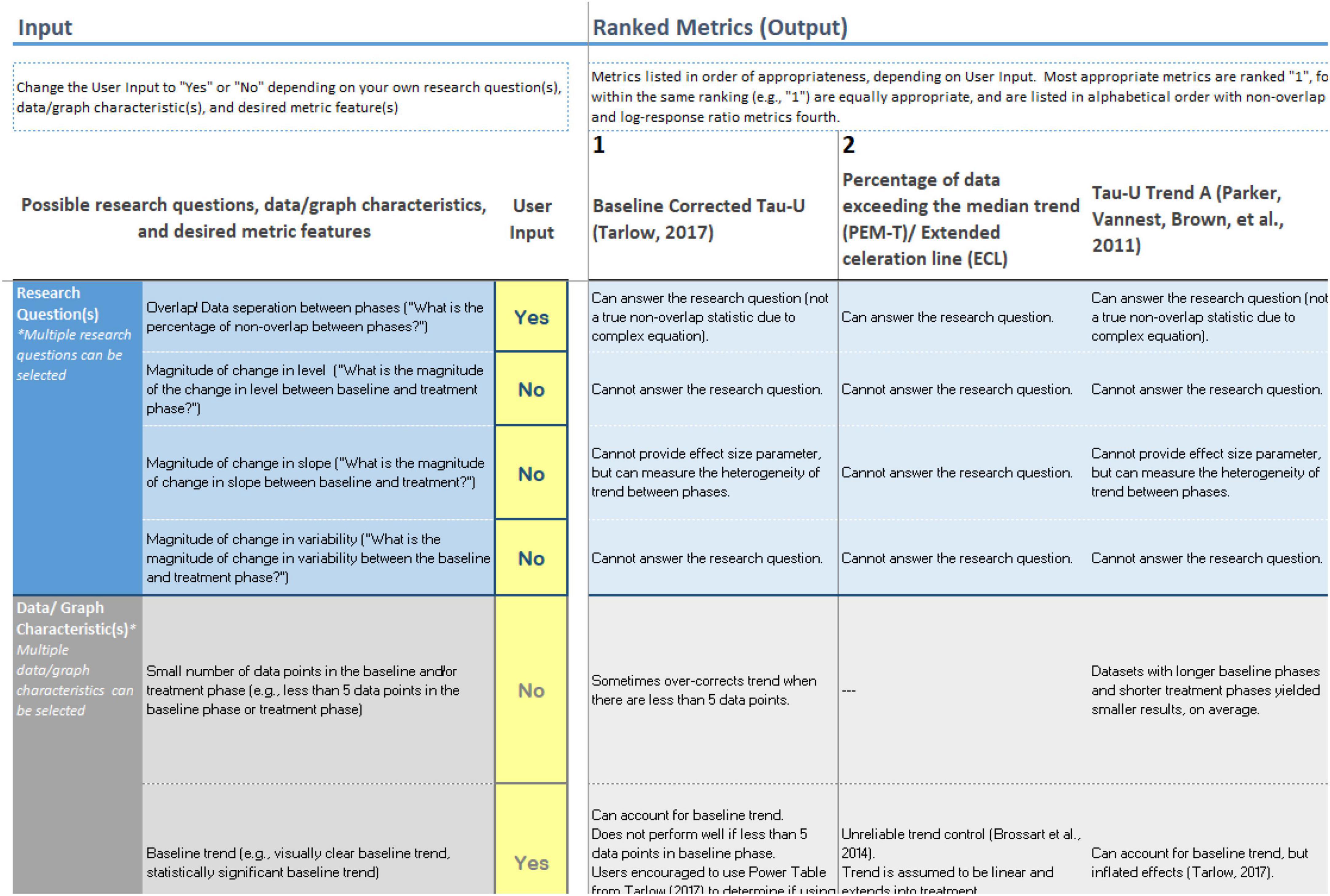
Figure 3. Tool input and output with ranked quantification techniques. As this is only a screenshot of the tool, readers are encouraged to download the tool themselves to read more details at: osf.io/7usbj/.
Instructions (in both written and video form) for how to use the tool were added to the first sheet of the Excel document. The tool, as described in the previous section and displayed in Figure 3, was added to the second sheet of the Excel document. On a third sheet, detailed information about each of the quantification techniques was added. On a fourth sheet, notes, references, and information about tool development were added. The complete tool with all four sheets can be downloaded from: osf.io/7usbj/
Five experts in the field of SCED validated the tool. These experts were systematically selected by searching “single-case” OR “single case” OR “single-subject” OR “single subject” on Web of Science starting from the year 2019, and sorting by Author/Creator. This ensured that the experts selected were familiar with the most recently developed SCED quantification techniques. First, the top five authors were contacted. After agreeing to review the tool, the experts were sent the tool and were asked to provide feedback regarding the accuracy of the information in the tool, the usability of the tool, and any other comments about the tool. Experts were also provided with a separate document that included five example graphs/data sets and research questions and were asked to evaluate the recommendations from the tool for each of the graphs/data sets and research questions. Next, the experts tested the tool and recorded their feedback. Three of the selected experts delivered feedback for the tool via writing (i.e., Word document) and two delivered feedback orally (i.e., Zoom meeting). There were several recommendations to adjust the tool that were made by multiple reviewers. Two reviewers recommended that the tool be explained carefully on the Instructions tab of the tool. Three reviewers recommended explaining in greater detail the definitions of the data characteristics. Two reviewers recommended clarifying that the tool is meant to be used for within-case estimates. All feedback was addressed, and the changes were made to the tool accordingly; the feedback along with the changes made were compiled into one document, which is found in Appendix A.
Participants
Institutional Review Board approval for the study was received from State University of New York at Albany. Anyone who conducted SCED research was eligible to participate. This was defined as someone who had interpreted the results of a SCED graph either with support (e.g., with help from an instructor or colleague) or independently within the last year. The participant needed to have conducted SCED analysis within the last year, and the participant also needed to be interested in quantifying the effect from a SCED graph. Snowball sampling was used to recruit participants. Participants were recruited from the Association for Behavior Analysis International list of colleges that offered applied behavior analysis courses; the professors who were part of the programs were contacted and asked to share information about the study with their students. Thus, potential participants were provided information about the study and then asked to provide other potential participants with information about the study.
A total of 25 participants participated in the study from a range of locations across several countries, with the majority of participants residing within the US. Two participants indicated Bachelor’s as their highest degree obtained, twelve participants indicated having a Master’s degree, and eleven participants indicated having a Ph.D. Seven participants identified with educational psychology department, six identified with school psychology, six identified with special education and/or applied behavior analysis, and six identified with a different department, such as psychology or prevention science. A total of two participants reported that they were very confident in their ability to choose appropriate quantification techniques, sixteen participants reported being somewhat confident, six participants were somewhat unconfident, and one participant reported being not at all confident. Similarly, one participant was very confident in their ability to justify using a quantification technique at the beginning of the study, sixteen participants were somewhat confident, seven participants were somewhat unconfident, and one participant was not at all confident. A total of ten participants had previously published SCED research in the past, thirteen participants had not yet published SCED research, but would like to in the future, and two participants had not and did not intend to publish SCED research.
Materials
Instrument
The Excel tool included the four sheets (Instructions, Tool, Metric Details, and Notes and References).
Pre/posttest AB graphs/data sets and research questions
Five pre/posttest AB graphs/data sets and research questions were created to help determine the effectiveness of the tool. AB graphs were chosen for this study as these are the “building blocks” of more complex SCEDs (e.g., multiple-baseline designs, withdrawal designs, etc.). SCED quantification techniques that are used with AB designs can be extended for use with these more complex designs. The raw data for the graphs/data sets were simulated so that each of the five pre/posttest graphs/data sets clearly demonstrated two facets: one facet related to data characteristic or desired quantification technique feature and one related to the research question. These graphs are displayed in Appendix B and an example is displayed in Figure 2.
Measures
Surveys
A demographic survey and social validity survey were developed to help evaluate the effectiveness of the tool. The demographic survey includes questions regarding the participant’s academic department, education level, and confidence in choosing and justifying the use of quantification techniques. The social validity survey asks participants on a 4-point Likert scale how likely they would be to use the tool in the future and how helpful they find the tool to be for choosing and justifying the use of quantification techniques.
Rubric with indicators
A rubric with three indicators was developed to measure the appropriateness of the quantification technique chosen and the appropriateness of the justification provided by the participant. This rubric is displayed in Table 1.
The elements used to evaluate the justification of the quantification technique are: “research question,” “quantification,” “number of observations,” “autocorrelation,” “outliers,” “missing data,” “baseline trend,” “variability,” “statistical properties,” and “sampling distribution.” These elements are founded upon the categories and facets identified by Manolov et al. (2022).
Applied example
Referring to Table 1, below is an example response and how it would be graded with the rubric.
Mean phase difference can be used for this graph and data set. MPD is a quantification technique that can estimate the magnitude of change in level between the baseline and treatment phase. The MPD estimate is 30.12.
This response is given a score of 5 for the indicator “quantification technique picked for use,” as MPD partially accounts for one of the essential elements and fully accounts for the other essential element. MPD can answer research questions related to the magnitude of change in level. MPD can be used when there are less than five data points in the baseline phase, but results are dependent on phase length, especially when the treatment phase is longer than the baseline phase (Manolov and Solanas, 2013). For “justification of the quantification technique,” this response would be given a score of 2 since it includes only one essential element in the justification (related to “research question”).
Procedure
After the tool was adjusted according to the expert feedback, participants were invited to participate in the study. Participants were given access to a Qualtrics link where they were able to read the consent form, located on the first page. After digitally signing the consent form, participants answered the screening questions. Next, the participants were shown the demographic survey and asked to complete the survey. After completing the demographic survey, participants were shown five pre/posttest AB graphs/data sets paired with the research questions. Participants were asked “Report the metric you would use to answer the research question(s). Provide a rationale for the metric(s) you would use. Optional: Complete the calculation.”
Next, the participants moved onto the next page where a link to the tool was provided. The participants read the instructions/watched the instructional video and could then access the tool. The participants were also provided with example graphs/data sets and research questions, and were allowed to practice using the tool for an unlimited amount of time. When participants were ready, they returned to the Qualtrics link. Participants were shown the same five pre/posttest AB graphs/data sets with research questions and given the same instructions as during pretest, but were instructed to use the tool to answer the questions. Once this was completed, participants moved onto the next page of Qualtrics and were asked to complete the demographic survey. The study completion time was recorded, and this marked the end of the study.
Statistical analysis
The participant received a score for each of the five pre/posttest AB graphs/data sets and research questions, as well as an accumulated score (i.e., across the five pre/posttest AB graphs/data sets and research questions) using the rubric in Table 1, both before using the tool (pretest) and after accessing the tool (posttest). An alpha level of.05 was used, and Bonferroni corrections were applied for multiple testing to avoid inflated family-wise type I error. All analyses were conducted with IBM SPSS Statistics (Version 27.0.1.0; IBM Corp, 2017).
Two-tailed paired t-tests were conducted to compare the pretest and posttest scores in choice and justification of quantification technique for each of the five questions, as well as the accumulated score. These results were also supplemented with the Wilcoxon signed-rank test, which is the non-parametric version of the parametric paired t-test. A total of 30 participants are typically needed to make the assumption that the sampling distribution is normally distributed (Brosamler, 1988; Fischer, 2010). If there are less than 30 participants and the pretest scores and change in scores are non-normal, then the sampling distribution will be non-normal. For this reason, all inferential results were supplemented with non-parametric tests, which do not make asymptotical assumptions. One-way ANOVAs were also conducted to determine if there were statistically significant differences in total (i.e., across the five questions) change in scores for choice and justification of quantification technique for participants depending on their academic background. The non-parametric equivalent of the ANOVA, Kruskal-Wallis test, was also conducted. Two-tailed independent paired t-tests and the non-parametric equivalent Mann-Whitney test were conducted to determine if there were statistically significant differences in total pretest scores and change in scores for choice and justification of quantification technique for participants depending on their education level. Descriptive analyses (i.e., averages and frequency) from the social validity survey were reported to determine the acceptability of the tool amongst the participants.
Results
Descriptive statistics
Upon completing the study, seventeen of the twenty-five participants reported that they would be very likely to use the tool in the future, six participants reported that they would be somewhat likely, and two participants reported that they would be somewhat unlikely to use it in the future. A total of twenty-one participants rated the tool as being very helpful for choosing an appropriate quantification technique, three participants rated the tool as being somewhat helpful, and one participant rated the tool as somewhat unhelpful. A total of nineteen participants rated the tool as being very helpful for justifying the use of a quantification technique, four participants rated the tool as somewhat helpful, and two participants rated the tool as somewhat unhelpful.3
Table 2 displays the pretest scores, posttest scores, and change in scores for choice and justification of quantification technique. There was a positive change in scores for each of the five questions for both choice and justification of quantification technique.
Inferential analyses
Change in scores
Six two-tailed paired t-tests were conducted to determine if the change in scores for (a) choosing and (b) justifying the quantification technique before and after using the tool was statistically significant for total (i.e., accumulated) change in scores and for each of the five individual questions.
The total average change in scores for choosing an appropriate quantification technique before (M = 18.16, SE = 1.17) and after (M = 27.08, SE = 0.33) using the tool was 8.92, d = 1.50, SE = 1.19, 95% CI (6.47, 11.37), t(24) = 7.52, p < 0.0083. This indicates a statistically and clinically significant change in total scores (i.e., across the five questions) after accessing the tool. The results of the non-parametric Wilcoxon signed-rank test were in line with the results of the parametric test (Z = 4.28, p < 0.0083). The total change in scores for justifying the use of a quantification technique before (M = 9.16, SE = 0.50) and after (M = 13.12, SE = 0.70) using the tool was 3.96, d = 1.08, SE = 0.73, 95% CI [2.45, 5.47], t(24) = 5.40, p < 0.0083, indicating a statistically and clinically significant change in scores between the pretest and posttest. Similar results were obtained from the Wilcoxon signed-rank test (Z = 3.69, p < 0.0083). There was a statistically significant change in scores for both choice and justification of quantification technique for each of the individual questions. These results are displayed in Table 3 and visually displayed in Figure 4.
Academic department differences
Four one-way ANOVAs were conducted to determine if there was a statistically significant difference in total change in scores for choice and justification of quantification technique, depending on the participant’s academic department (special education, educational psychology, school psychology, and others). For the purpose of inferential analyses, participants who reported being a part of both special education and applied behavior analysis were coded as “special education” (n = 2). Results of the ANOVAs are displayed in Table 4 and are visually displayed in Figure 5.
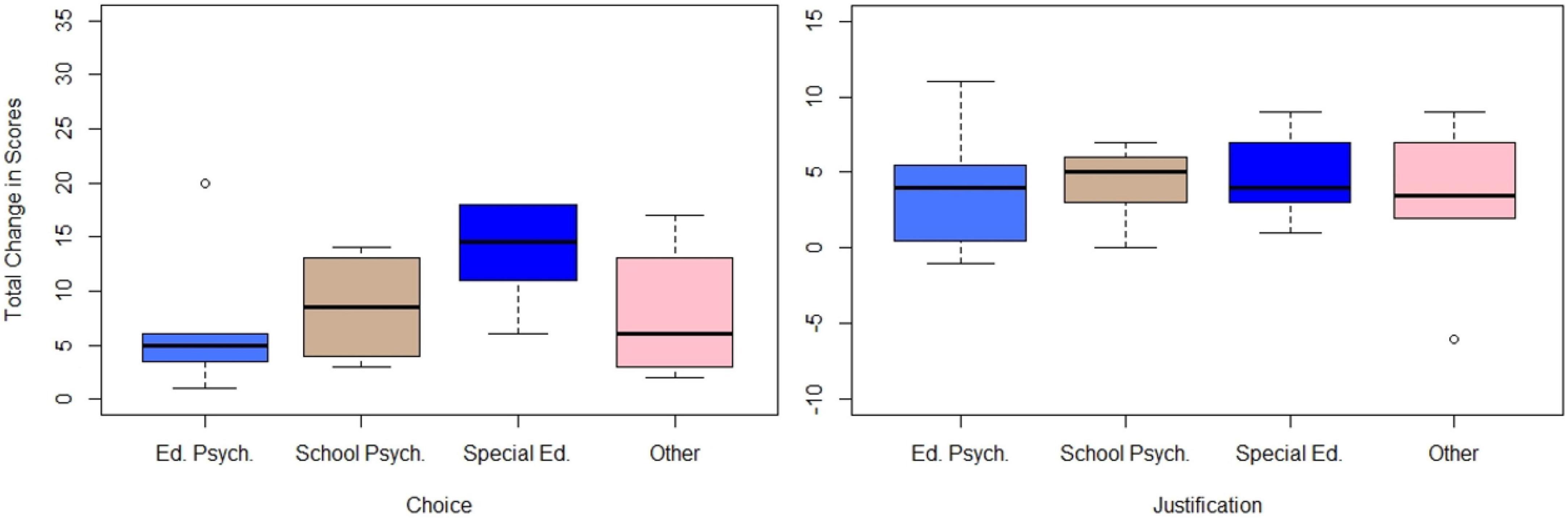
Figure 5. Total change in scores for choice and justification of quantification technique across academic departments.
The ANOVAs showed no statistically significant effect of academic department on total change in scores for choice of quantification technique [η2 = 0.23, F(3, 21) = 2.14, p = 0.13,], nor for justification of quantification technique [η2 = 0.02, F(3, 21) = 0.18, p = 0.91,]. A total of 23 and 2% of the variance in change in scores for choice and justification of quantification technique can be attributed to academic department, respectively. Although not statistically significant, 23% is a moderately large effect. The non-parametric Kruskal-Wallis test was also conducted and showed no effect of academic department on change in scores for choice of quantification technique [H(3) = 5.84, p = 0.12], nor for justification of quantification technique [H(3) = 0.44, p = 0.93].
Education level differences
Four independent two sample t-tests were conducted to determine if there was a difference in total change in scores for choice and justification of quantification technique depending on education level (Master’s Degree vs. Ph.D). Results of the independent t-tests are displayed in Table 5 and are visually displayed in Figure 6.
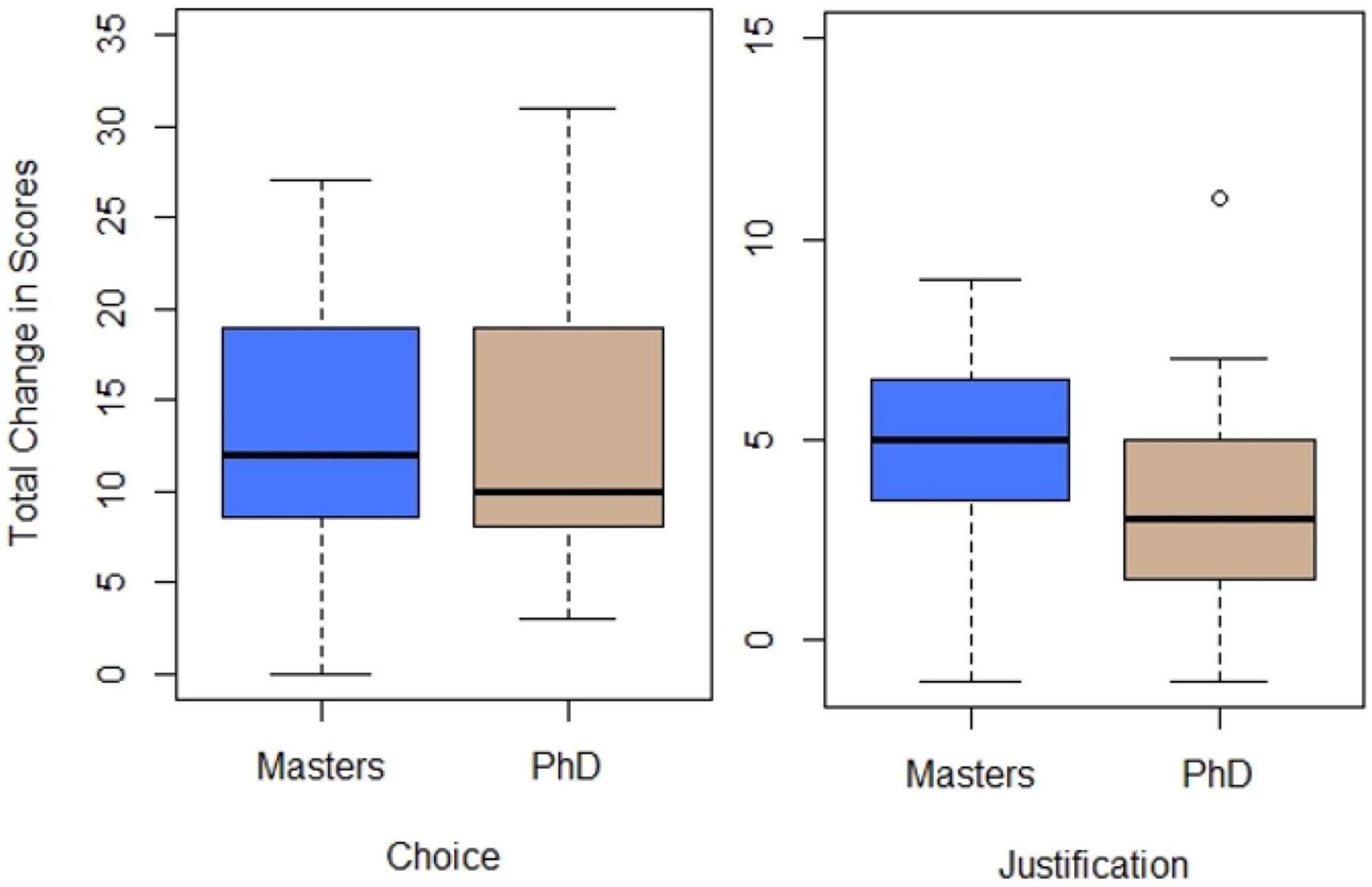
Figure 6. Total change in scores for choice and justification of quantification technique across education level.
The independent two sample t-test showed total change in score for quantification technique choice were not statistically significantly different for those with a Master’s Degree (M = 8.42, SE = 1.69), d = –0.26, t(21) = –0.61, p = 0.54, than for those with a Ph.D (M = 10.00, SE = 2.02). A Mann-Whitney test also indicated no difference in total change in scores for quantification technique choice between those with a Master’s degree than for those with a Ph.D (U = 57.50, p = 0.60). The independent two sample t-test showed total change in scores for quantification technique justification were not statistically significantly different for those with a Master’s Degree (M = 4.83, SE = 0.85), d = 0.38, t(21) = 0.92, p = 0.39 than for those with a Ph.D (M = 3.64 SE = 1.03). A Mann-Whitney test also indicated no difference in total change in scores for quantification technique justification between those with a Master’s degree than for those with a Ph.D (U = 47.00, p = 0.24).
Discussion
This study had several purposes. First, a user-friendly tool was developed to help researchers and practitioners choose and justify their use of appropriate SCED quantification techniques. Secondly, the tool was formally tested with a pretest and posttest design. Furthermore, participant data was collected to determine the social validity of the tool (i.e., acceptance amongst users). Follow up analyses were also conducted to determine differences between academic departments and education levels.
The tool is highly effective in assisting participants to choose appropriate quantification techniques for various research questions, data characteristics, and desired quantification technique features. The effectiveness of the tool is demonstrated through the statistically significant positive change in scores between pretest and posttest for all five questions, as well as the change in variability of scores between pretest and posttest. The tool is also effective in improving the justifications provided for using quantification techniques. The mean change in score for justification of the quantification technique across the five questions was 0.79, indicating that the average participant is able to provide one additional justification after accessing the tool. The results from the social validity questions also demonstrate the effectiveness of the tool. The majority of participants (n = 21, 84%) rated the tool as highly helpful for choosing an appropriate quantification technique to use. The majority of participants (n = 19, 76%) also rated the tool as highly helpful for justifying use of the quantification technique. Seventeen (68%) participants reported that they would be very likely to use the tool in the future; most participants reported that they would be at least somewhat likely to use the tool in the future, demonstrating that the majority of participants found the tool to be practical.
The results of the study show that the tool is effective across different levels of education. It is unsurprising that education level was not found to be related to the effectiveness of the tool; those with various education levels could have various SCED experience. Results of this study showed no statistically significant difference in change in scores for choice or justification of the quantification technique across academic departments. However, it is worth noting that the effect size for the difference in change in scores for choice of quantification technique between academic departments is moderate (η2 = 0.23), indicating that the lack of statistical significance could possibly be attributed to insufficient power as a result of a small sample size. Thus, it is possible that there is a true difference between the different academic departments. Although sampling across different departments to determine differences was beyond the scope of this study, future research could control for differences between academic departments, for example, to determine if there are true differences.
Implications for the field of single-case experimental design
The findings from this study have implications for the field of SCED. First, the low pretest scores demonstrate that the average SCED researcher, with varying education levels and from various academic departments, needs support in choosing and justifying SCED quantification techniques. For example, during pretest for Questions 14 and 3,5 sixteen (64%) participants chose non-overlap techniques for use, although non-overlap techniques are unable to provide a quantification of the intervention effect that reflects the magnitude of the change in level, nor the magnitude of change in variability. Thus, such quantification techniques cannot provide a quantification of the intervention effect that answers either research question. These results reflect the misuse of non-overlap quantification techniques in particular, as similar pattern of mistakes are not evident for Question 4, for which the research question was about the amount of overlap/data separation between phases. SCED users may not realize that non-overlap quantification techniques cannot represent the magnitude of change/size of the effect (Fingerhut et al., 2021a,b). No longer using terms such as “metric” or “effect size” to refer to non-overlap measures may better help SCED researchers carefully consider the quantification techniques they use, and better understand what exactly the quantification of the intervention effects represent.
Similarly, participants of various education levels and academic departments chose quantification techniques that are inappropriate for use with the present graph/data characteristics. During pretest for Question 2,6 four (16%) participants chose quantification techniques that are highly affected by baseline trend, even though trend was present in the baseline phase. Similarly, some participants chose quantification techniques that are not robust against outliers for Question 47 (n = 14, 56%). Taken together, this inability to choose an appropriate quantification technique is especially concerning because certain quantification techniques may not yield reliable results if the quantification techniques are inappropriate for use with the data. For example, Fingerhut et al. (2021b) found that Tau estimates depend upon data characteristics such as baseline trend, within-case variability, and number of data points in the baseline phase. Pustejovsky (2019) found that procedural features such as the length of the observation sessions and the recording system used to collect measurements of behavior can influence the quantification of the intervention effect of certain SCED techniques. Vannest et al. (2018) and Manolov et al. (2022) explicitly call for researchers to choose SCED quantification techniques carefully in the context of their own research questions and data characteristics. Thus, it is concerning that pretest results highlight the use of inappropriate SCED quantification techniques.
Results of this study also show that SCED researchers with various education levels and from different academic departments need more support in justifying their use of quantification techniques. This is a logical finding, as people cannot justify their use of quantification techniques if they are unable to choose quantification techniques that are wholly appropriate for use in the first place. While it is encouraging that the average participant could provide one justification during pretest, the results of this study show that SCED users need more support in justifying their use of quantification techniques. The results of this study show that a user-friendly tool can help improve justifications provided.
Failure to take the research questions and data characteristics into account when choosing a quantification technique can ultimately result in the dissemination of findings that do not accurately represent actual intervention effects. Since the enactment of laws such as Every Student Succeeds Act in 2015, there has been a greater push to use evidence-based practices in the field of special education. Therefore, it has become more imperative that quantifications of the intervention effects from studies accurately reflect the effectiveness of interventions. If teachers, behaviorists, clinicians, school psychologists, and other practitioners rely on SCED research to determine which interventions to use with students, they may be using interventions that are not actually effective if the results are unreliable. Meta-analyses use and rely on primary level summary findings; if inappropriate quantification techniques are used in these primary studies, then biased or misleading results may be obtained at the meta-analytic level as well. Thus, it is imperative that appropriate SCED quantification techniques are used, and this study highlights that those conducting SCED research may currently be using inappropriate quantification techniques to analyze their data. However, the results of this study are encouraging in that this study provides evidence that teachers, practitioners, and researchers are able to improve the way that they understand and analyze SCED data with a self-paced and user-friendly tool.
This tool has several practical uses. It would be useful if those who review SCED studies, such as journal editors, could reference the authors of studies undergoing peer review to the SCED tool so that they can critically think about quantifying intervention effectiveness and provide a strong justification for the analysis used. This tool could easily be incorporated into a 1- or 2-h college course. This tool can be introduced and disseminated at special education, applied behavior analysis, or school psychology conferences. The Institute of Education Sciences hosts an advanced training session each summer for single-case research methods; this is another environment in which the tool can be introduced and disseminated.
Limitations of the study and tool
A total of 25 people participated in this study, and so generalizations beyond this sample are limited. It is possible that only those who were previously interested in learning about quantification techniques participated in the study, and so the results may be biased. The tool was not designed to aid in interpretations of the underlying scales of the different quantification techniques. Any calculations conducted and the resulting quantification of the intervention effect must be interpreted carefully, and assistance in understanding the clinical significance of the quantification of the intervention effect is not something that is provided by using this tool. It is possible that the tool is missing some quantification techniques, especially as the field of SCED continues to grow. However, the tool is posted through OSF and can be downloaded from osf.io/7usbj/, and on the Notes and References sheet an email address is provided for users to send feedback (SCEDmetrictool@gmail.com). Thus, input of the broader research community is invited and can be used to further improve the tool. This tool is meant to be used for within-case estimates only. While some of the quantification techniques listed in the tool can be used for across-case estimates, there may be better options when conducting across-case estimates (see Notes and References sheet of the tool). Furthermore, as several of the experts who validated the tool pointed out, the tool is only appropriate for AB-based research designs, as the information listed in the tool assumes that the user is applying the quantification techniques to an AB-based design. Although the tool was tested with AB designs for the purpose of this study, researchers are encouraged to use the tool with stronger designs, such as withdrawal and reversal designs and multiple-baseline designs.
Future research
This study could be replicated with a larger sample. Prior SCED experience of participants could be a demographic question presented to participants, so that inferential results can be conducted to determine if there is a statistically significant relationship between length of SCED experience in years and the effectiveness of the tool. Future studies can focus on the effect of the tool on participants’ ability to calculate the quantification of the intervention effect correctly and interpret the quantification of the intervention effect as well. It would be helpful to conduct a formal study to determine if the tool is still helpful after it is made more complex. For example, more research questions (e.g., research questions about gradual effects or delayed effects) and data characteristics (e.g., autocorrelation) can be added into the tool. The tool can also be expanded so that it can be used for between-case estimates and with various research designs. It also can be converted into an R code or website.
Data availability statement
The original contributions presented in this study are included in the article/Supplementary material, further inquiries can be directed to the corresponding author.
Ethics statement
The studies involving human participants were reviewed and approved by Institutional Review Board at University at Albany. The patients/participants provided their written informed consent to participate in this study.
Author contributions
Both authors listed have made a substantial, direct, and intellectual contribution to the work, and approved it for publication.
Conflict of interest
The authors declare that the research was conducted in the absence of any commercial or financial relationships that could be construed as a potential conflict of interest.
Publisher’s note
All claims expressed in this article are solely those of the authors and do not necessarily represent those of their affiliated organizations, or those of the publisher, the editors and the reviewers. Any product that may be evaluated in this article, or claim that may be made by its manufacturer, is not guaranteed or endorsed by the publisher.
Supplementary material
The Supplementary Material for this article can be found online at: https://www.frontiersin.org/articles/10.3389/feduc.2022.1064807/full#supplementary-material
Footnotes
- ^ Also known as a single case, single subject, single-subject, interrupted time series, small n, n of 1 trial, and n = 1.
- ^ Other popular analysis techniques include masked visual analysis (Ferron and Jones, 2006), randomization tests (Edgington, 1996), and the reliable change index (Jacobson and Truax, 1991). While useful, these are not included in the present study because these methods primarily focus on p-values and statistical significance rather than quantifying the effect (i.e., producing an “effect size”).
- ^ Detailed participant data is available upon request from the first author.
- ^ Research question: What is the magnitude of change in level? Unique data characteristic: Small number of data points in baseline or treatment phase.
- ^ Research question: What is the magnitude of change in variability? Unique data characteristic: Variability.
- ^ Research question: What is the magnitude of change in trend? Unique data characteristic: Baseline trend.
- ^ Research question: What is the amount of non-overlapping data? Unique data characteristic: Outliers.
References
Alnahdi, G. H. (2013). Single-subject designs in special education: Advantages and limitations. J. Res. Spec. Educ. Needs 15, 257–265. doi: 10.1111/1471-3802.12039
Brosamler, G. A. (1988). An almost everywhere central limit theorem. Math. Proc. Camb. Philos. Soc. 104, 561–574. doi: 10.1017/S0305004100065750
Center, B., Skiba, R., and Casey, A. (1985-1986). A methodology for the quantitative synthesis of intra-subject design research. J. Spec. Educ. 19, 387–400. doi: 10.1177/002246698501900404
Edgington, E. S. (1996). Randomized single-subject experimental designs. Behav. Res. Ther. 34, 567–574.
Ferron, J., and Jones, P. K. (2006). Tests for the visual analysis of response-guided multiple-baseline design. J. Exp. Educ. 75, 66–81. doi: 10.3200/JEXE.75.1.66-81
Ferron, J., Goldstein, H., Olszewski, A., and Rohrer, L. (2020). Indexing effects in single-case experimental designs by estimating the percent of goal obtained. Evid. Based Commun. Assess. Interv. 14, 6–27. doi: 10.1080/17489539.2020.1732024
Fingerhut, J., Xu, X., and Moeyaert, M. (2021a). Selecting the proper Tau-U measure for single-case experimental designs: Development and application of a decision flowchart. Evid. Based Commun. Interv. 15, 99–114. doi: 10.1080/17489539.2021.1937851
Fingerhut, J., Xu, X., and Moeyaert, M. (2021b). Impact of within-case variability on Tau-U and regression-based effect size measures for single-case experimental data. Evid. Based Commun. Assess. Interv. 15, 115–141. doi: 10.3102/1583005
Fischer, H. (2010). A History of the Central Limit Theorem: From Classical to Modern Probability Theory. New York, NY: Springer. doi: 10.1007/978-0-387-87857-7_5
Hedges, L., Pustejovksy, J., and Shadish, W. (2012). A standardized mean difference effect size for single case designs. Res. Synth. Methods 3, 224–239. doi: 10.1002/jrsm.1052
Hershberger, S. L., Wallace, D. D., Green, S. B., and Marquis, J. G. (1999). “Meta-analysis of single-case designs,” in Statistical Strategies for Small Sample Research, ed. R. H. Hoyle (Thousand Oaks, CA: Sage), 109–132.
Horner, R. H., Carr, E. G., Halle, J., McGee, G., Odom, S., and Wolery, M. (2005). The use of single-subject research to identify evidence-based practice in special education. Except. Child. 71, 165–179. doi: 10.1177/001440290507100203
Jacobson, N. S., and Truax, P. (1991). Clinical significance: A statistical approach to defining meaningful change in psychotherapy research. J. Consult. Clin. Psychol. 59, 12–19. doi: 10.1037/0022-006X.59.1.12
Ledford, J. R., Lane, J. D., Zimmerman, K. N., Chazin, K. T., and Ayres, K. A. (2016). Single Case Analysis And Review Framework (SCARF). Available online at: http://ebip.vkcsites.org/scarf/ (accessed November 18, 2022).
Ma, H. (2006). An alternative method for quantitative synthesis of single-subject research: Percentage of data points exceeding the median. Behav. Modif. 30, 598–617. doi: 10.1177/0145445504272974
Manolov, R., and Moeyaert, M. (2017). Recommendations for choosing single-case data analytical techniques. Behav. Ther. 48, 97–114. doi: 10.1016/j.beth.2016.04.008
Manolov, R., and Solanas, A. (2013). A comparison of mean phase difference and generalized least squares for analyzing single-case data. J. Sch. Psychol. 51, 201–215. doi: 10.1016/j.jsp.2012.12.005
Manolov, R., Moeyaert, M., and Fingerhut, J. (2022). A priori metric justification for the quantitative analysis of single-case experimental data. Perspect. Behav. Sci. 45, 153–186. doi: 10.1007/s40614-021-00282-2
Moeyaert, M., Ferron, J. M., Beretvas, S. N., and Van den Noortgate, W. (2014). From a single-level analysis to a multilevel analysis of single-case experimental designs. J. Sch. Psychol. 52, 191–211. doi: 10.1016/j.jsp.2013.11.003
Parker, R. I., and Vannest, K. J. (2009). An improved effect size for single case research: Nonoverlap of all pairs (NAP). Behav. Ther. 40, 357–367. doi: 10.1016/j.beth.2008.10.006
Parker, R. I., Vannest, K. J., Davis, J. L., and Sauber, S. B. (2011). Combining nonoverlap and trend for single-case research: Tau-U. Behav. Ther. 42, 284–299. doi: 10.1016/j.beth.2010.08.006
Parker, R., Hagan-Burke, S., and Vannest, K. (2007). Percentage of all non-overlapping data (PAND): An alternative to PND. J. Spec. Educ. 40, 194–204. doi: 10.1177/00224669070400040101
Parker, R., Vannest, K., and Brown, L. (2009). The improvement rate difference for single case research. Except. Child. 75, 135–150. doi: 10.1177/001440290907500201
Pustejovsky, J. E. (2018). Using response ratios for meta-analyzing single-case designs with behavioral outcomes. J. Sch. Psychol. 68, 99–112. doi: 10.1016/j.jsp.2018.02.003
Pustejovsky, J. E. (2019). Procedural sensitivities of effect sizes for single-case designs with directly observed behavioral outcome measures. Psychol. Methods 24, 217–235. doi: 10.1037/met0000179
Pustejovsky, J. E., Hedges, L. V., and Shadish, W. R. (2014). Design-comparable effect sizes in multiple baseline designs: A general modeling framework. J. Educ. Behav. Stat. 39, 368–393. doi: 10.3102/1076998614547577
Scotti, J. R., Evans, I. M., Meyer, L. H., and Walker, P. (1991). A meta-analysis of intervention research with problem behavior: Treatment validity and standards of practice. Am. J. Ment. Retard. 96, 233–256.
Scruggs, T. E., Mastropieri, M. A., and Casto, G. (1987). The quantitative synthesis of single-subject research: Methodology and validation. Remedial Spec. Educ. 8, 24–33. doi: 10.1177/074193258700800206
Shadish, W. R. (2014). Statistical analyses of single-case designs: The shape of things to come. Curr. Direct. Psychol. Sci. 23, 139–146.
Shadish, W. R., and Sullivan, K. J. (2011). Characteristics of single-case designs used to assess intervention effects in 2008. Behav. Res. Methods 43, 971–980. doi: 10.3758/s13428-011-0111-y
Solomon, B. G., Howard, T. K., and Stein, B. L. (2015). Critical assumptions and distribution features pertaining to contemporary single-case effect sizes. J. Behav. Educ. 24, 438–458. doi: 10.1007/s10864-015-9221-4
Tarlow, K. R. (2016). Baseline Corrected Tau Calculator. Available online at: http://www.ktarlow.com/stats/tau
Tarlow, K. R. (2017). An improved rank correlation effect size statistic for single-case designs: Baseline corrected Tau. Behav. Modif. 41, 427–467. doi: 10.1177/0145445516676750
Tate, R. L., Perdices, M., Rosenkoetter, U., McDonald, S., Togher, L., Shadish, W., et al. (2016). The single-case reporting guideline in behavioural interventions (SCRIBE) 2016: Explanation and elaboration. Arch. Sci. Psychol. 4, 10–31. doi: 10.1037/arc0000027
Van den Noortgate, W., and Onghena, P. (2008). A multilevel meta-analysis of single-subject experimental design studies. Evid. Based Commun. Assess. Interv. 2, 142–158. doi: 10.1080/17489530802505362
Vannest, K. J., Parker, R. I., Gonen, O., and Adiguzel, T. (2016). Single Case Research: Web Based Calculators for SCR Analysis. (Version 2.0) [Web-based application]. College Station, TX: Texas A&M University.
Vannest, K., Peltier, C., and Haas, A. (2018). Results reporting in single case experiments and single case meta-analysis. Res. Dev. Disabil. 79, 10–18. doi: 10.1016/j.ridd.2018.04.029
What Works Clearinghouse (2020). What Works Clearinghouse Standards Handbook, Version 4.1. Washington, DC: U.S. Department of Education.
Keywords: single-case design, quantification techniques, evidence-based, open-source tool, decision-making, applied behavior analysis, practitioners, special education
Citation: Fingerhut J and Moeyaert M (2022) Selecting and justifying quantitative analysis techniques in single-case research through a user-friendly open-source tool. Front. Educ. 7:1064807. doi: 10.3389/feduc.2022.1064807
Received: 08 October 2022; Accepted: 11 November 2022;
Published: 29 November 2022.
Edited by:
James Martin Boyle, University of Strathclyde, United KingdomReviewed by:
Jeremy R. Sullivan, University of Texas at San Antonio, United StatesJairo Rodríguez-Medina, University of Valladolid, Spain
Copyright © 2022 Fingerhut and Moeyaert. This is an open-access article distributed under the terms of the Creative Commons Attribution License (CC BY). The use, distribution or reproduction in other forums is permitted, provided the original author(s) and the copyright owner(s) are credited and that the original publication in this journal is cited, in accordance with accepted academic practice. No use, distribution or reproduction is permitted which does not comply with these terms.
*Correspondence: Joelle Fingerhut, joelle.fingerhut@marist.edu