- 1Instituto Universitario de Investigación en Tecnología Centrada en el Ser Humano, Universitat Politècnica de València, Valencia, Spain
- 2Escuela de Artes y Humanidades, EAFIT, Medellín, Colombia
- 3School of Engineering and Sciences, Tecnológico de Monterrey, Monterrey, Mexico
This study is a literature review with educational evaluation mediated by intelligent tutoring systems (ITS) as its central axis seeking to establish state of the art on implementations executed in the last 20 years and their impact on the evaluation process. The PRISMA methodology was applied for the literature review; the studies were included using the R software and bibliometric techniques with a general search equation that allowed access to all ITS production in Scopus. Subsequently, with the help of artificial intelligence, text mining was used to identify topics of interest in the scientific community, followed by further filtering. Finally, the selected full texts were analyzed using the NVivo software to extract emerging challenges in the field, obtaining 163 full texts for analysis. Among the main findings, the primary purpose of evaluation in ITS was summative, peer and self-evaluation did not have the same level of importance as hetero evaluation, and ITS focus was quantitative. All of this allowed us to conclude that the analyzed texts did not implement a holistic perspective and therefore evidenced the need to establish a framework for constructing an ITS using current technologies that integrate the mentioned variables.
Introduction
According to Álvarez de Zayas (2010), assessment is a systemic, holistic, and dialectical process, or, in other words, a complex process. However, this conception of evaluation does not always correspond to what those involved in educational processes put into practice. For example, in higher education, it is common that the preferred instrument for collecting information is the exam (Gibb et al., 2011). It is also common to confound evaluating with grading, measuring, correcting, classifying, or examining and focusing on the quantitative aspects (Álvarez, 2001). Although the grading process is related to evaluation and provides valuable data for decision-making (refer to Figure 1), it needs to be complemented with multiple instruments that integrate qualitative and continuous aspects that allow transforming classroom dynamics, not only at the end of the academic periods. In other words, they must be aligned with the true meaning of evaluation—a formative, regulatory, pedagogical, and communicative tool (Carless and Boud, 2018).
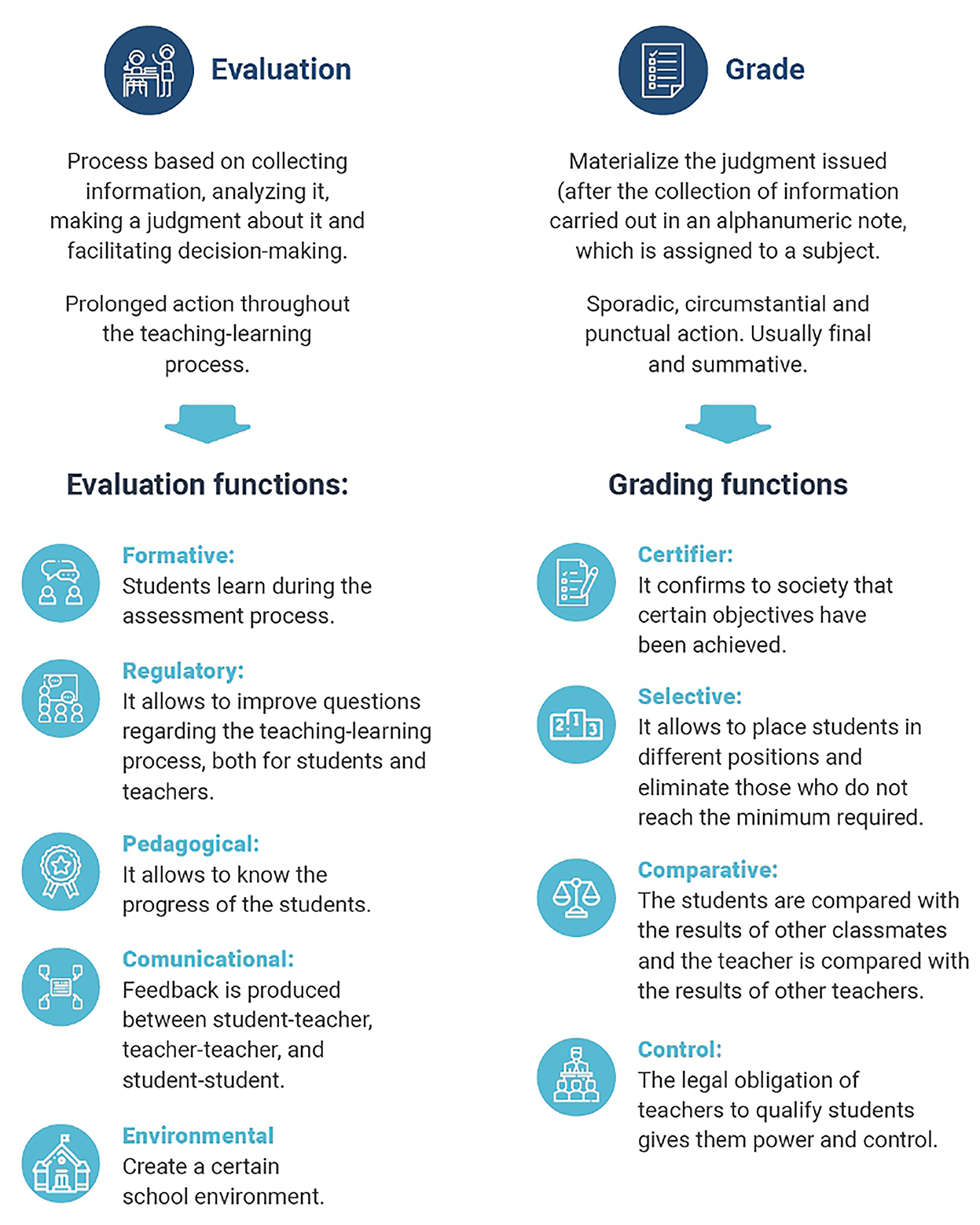
Figure 1. Evaluating and grading process. Adapted from Hamodi et al. (2014).
This situation can be better understood if we consider the different objectives of evaluation, i.e., it can be diagnostic, formative, or summative. In the diagnostic case, decisions can be made based on the student's starting level, adjusting methodologies, and monitoring strategies. In formative evaluation, the focus is on the learning process and, therefore, the acquisition of competencies; this implies, in most cases, the constant intervention of the teacher or, as will be explained in this study, the teacher supported by technology. Finally, the summative evaluation usually takes place at the end of the process and serves as a control. It is usually related to quantitative assessments that provide relevant information for decision-making for students and teachers. Furthermore, in relation to the holistic character of these evaluation objectives, the teacher must move among all three of them constantly (Chufama and Sithole, 2021; Rehhali et al., 2022; Sudakova et al., 2022).
In the case of basic sciences, the misinterpreted evaluation focused on results aggravates the problems of performance, grade repetition, and, in some cases, drop out. For example, according to Castillo-Sánchez et al. (2020), one of the leading causes of repetition in the first mathematics course is low academic performance in the first partial exam.
Introductory science courses are conventionally graded through exams, with the percentage distribution depending on the university. For example, in the Mathematics School at the National University of Colombia, there are three midterms of 25, 30, and 30%, respectively, and a short exam of 15% (Cuéllar Rojas, 2013). This implies that the student receives feedback on his learning process only in some specific moments and not in all classes.
However, given this approach, it is difficult to avoid the question: How can an evaluation process that overcomes these difficulties be implemented in courses with many students? This question has already been addressed, although not resolved. Digital technologies offer the educational community a wide range of ways to collect information, such as interactive videos, simulations, and surveys (Torres Mancera and Gago Saldaña, 2014)—all of which may be configured to be assessed automatically without requiring excessive teacher time. However, if these tools were implemented, the evaluation process would continue without solving the fundamental evaluative aspect. What decisions are to be made with the data? Or, even more complex, how to analyze these data?
One of the favorable environments for these implementations is the intelligent tutoring system (ITS). It is possible to transition from exam-centered grading to one that draws on multiple instruments. In this context, the student receives constant cognitive and metacognitive feedback. As mentioned earlier, formative assessment is a crucial element for learners' success. It involves three agents, namely, the teacher, the peers, and the learner himself/herself. Although formative assessment is not new, it has been limited in contexts where the number of students exceeds the teacher's physical capacity to accompany each of them. There are other tools that the teacher can use to compensate for this deficiency, such as self-assessment and peer assessment, which have a broader scope. This learning process involves students using the aforementioned metacognitive process to evaluate their learning outcomes (Schildkamp et al., 2020; Shemshack and Spector, 2020).
The main task of an ITS is to evaluate students' knowledge acquisition throughout the education process. In general, aAdaptive ITS provides learning environments in which all relevant information about students is kept and used to guide them (Lemke, 2013; Tan and Chen, 2022).
Intelligent tutoring system uses artificial intelligence principles and methods, for example, neural networks, to make inferences and learn autonomously. This characteristic enables ITS to be adaptive, since it alters its structure, functionality, or interface for the user and their needs (Anohina, 2007).
Intelligent tutoring system has different configurations according to the application context, but four modules stand out in educational courses, namely, (1) the pedagogical module, (2) the student module (diagnosis), (3) the expert module, and (4) the communications module. These modules are complemented by the models created from the data they provide, which are represented in blue (refer to Figure 2A).
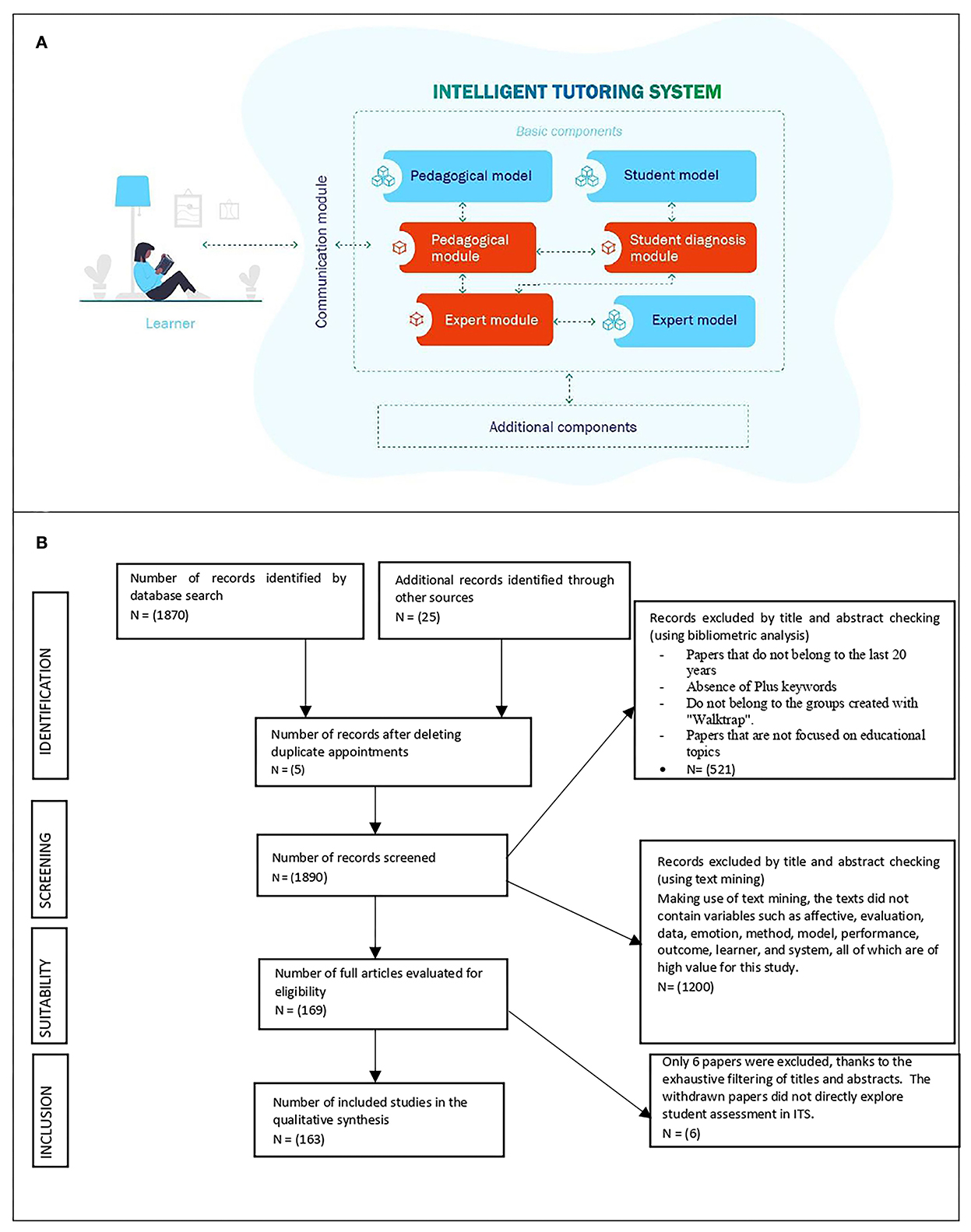
Figure 2. (A) ITS Basic components. Adapted from Anohina (2007) and Al-Hanjori et al. (2017) and (B) the process of PRISMA for data collection and analysis.
This structure integrates naturally with massive courses, favoring learning environments with lesser teacher interaction. Student and teacher interactions using these modules produce large volumes of mixed data. Unfortunately, this information is difficult to analyze on a massive scale. Considering that Massive Online Course has exceeded 180 million students (Shah, 2020) and that the number of participants per course easily exceeds 1,000 in some of them (Kaser and Gütl, 2016), these figures justify mass-grading strategies, with which it is possible to achieve constant and automatic feedback, minimizing the interaction with the tutor and turning the student into the protagonist of the learning process. However, the amount of data generated by this constant interaction grows exponentially and quickly, exceeding the human capacity to analyze them and make decisions that are not always quantitative.
This system responds to qualitative questions about each student, as specific as:
1. Which of the concepts covered in class require further study?
2. What are the performance levels in the fundamental competencies of the course from the first class?
3. What methodological adjustments are required in the course to favor the student process?
4. What curricular adjustments are necessary to favor the development of the competencies offered by the course?
5. What feedback do teachers and students require to make decisions that favor the acquisition of the competencies offered by the course?
Furthermore, all aspects related to the individual process of the subjects are complex even for a conventional number of students, since the evaluative processes of this level of personalization require an investment of time on behalf of the educational actors that do not correspond to the implementation model (maximizing the number of participants, minimizing tutors).
These tasks for massive groups require an intelligent data processing system that learns from the data and acts as a virtual master, performing accurate decision-making evaluations. However, the approaches to this problem are still under development. Fundamental variables have been considered (Rajendran et al., 2019; Torres-Madroñero et al., 2020). For example, students' self-regulation or motivation has been included in some ITS. However, aspects such as diagnostic, formative, and summative evaluation have not been considered together. Therefore, a systematic review was conducted to identify and evaluate articles that propose implementations of evaluation systems using machine learning techniques for massive volumes of data.
Methodology
Method
This systematic review was conducted based on the preferred reporting items for systematic reviews and meta-analyses (PRISMA) proposed by Moher et al. (2015). Figure 2B displays the process of PRISMA for data collection and analysis.
Research questions
This systematic review responds to the following research questions:
• RQ 1: What is the ITS primary evaluation purpose?
• RQ 2: What is the main evaluating agent (in evaluation processes)?
• RQ 3: What is the main approach used in the selected ITS?
• RQ 4: Is the ITS evaluation process implemented holistically?
Search strategy
With the search equation *intelligent tutoring*, the following results presented in Table 1 were obtained. However, it is crucial to remember that this general equation is only considered since it was expected to obtain new filtering criteria that will lead to a more refined equation.
A total of 1,890 results were found in Scopus, covering 42 years of academic production. The texts considered were articles published in specialized journals, although it is recognized that this field of knowledge has important dissemination through conferences. However, due to the objective of the study to identify structured knowledge with an important level of depth, conference papers were not included in this analysis. Thus, a total of 3,819 authors were considered in this initial search.
Academic production began in 1979; in 2014, it reached its maximum (105 papers), and since 2016, such production has slightly decreased (Figure 3A).
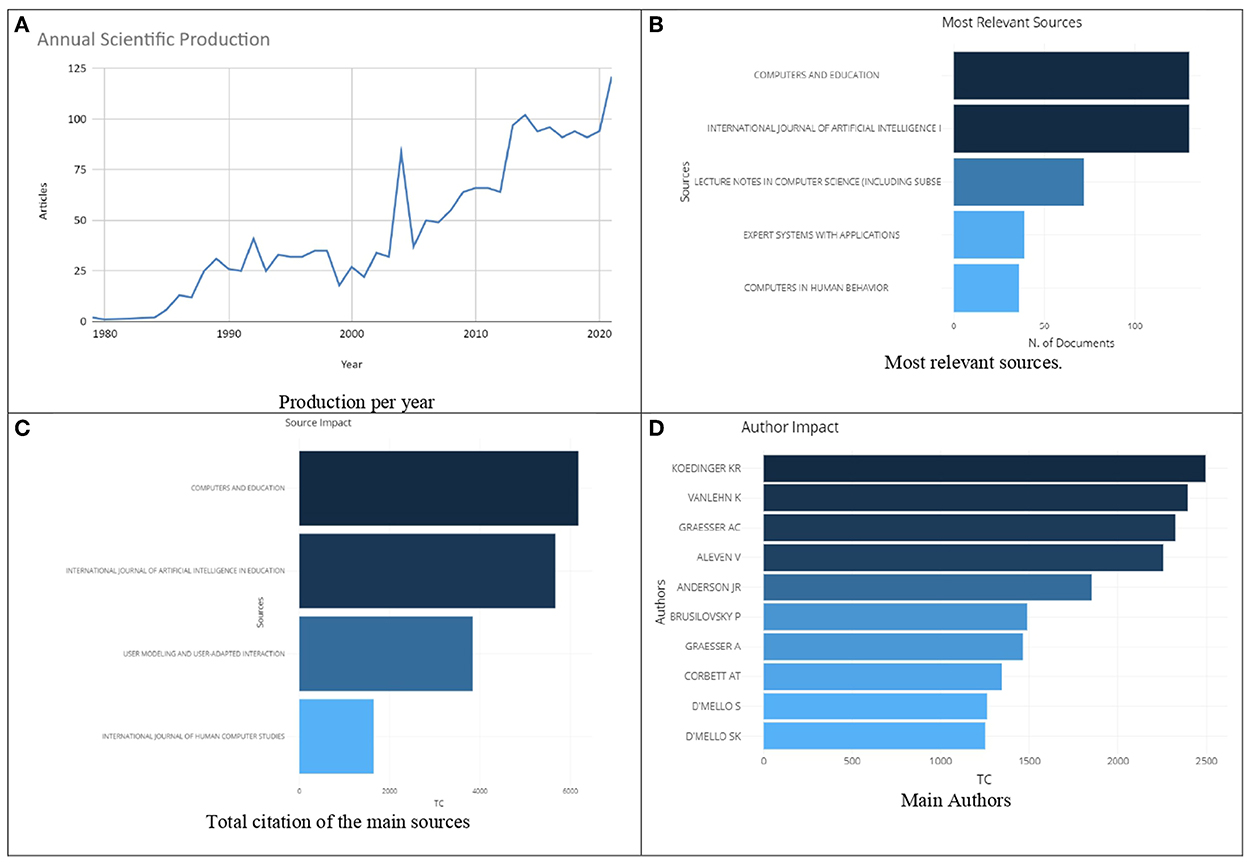
Figure 3. (A) Production per year, (B) Most relevant sources, (C) Total citation of the main sources, and (D) Main Authors.
Figure 3B shows that the largest source of texts was the International Journal of Artificial Intelligence in Education, classified in Q1. Figure 3C shows the top five most-cited journals in relation to ITS. The journal Computers and Education stands out with a total of 4,814 citations.
The main authors by total citations in the chosen period are presented in Figure 3D. For example, Kenneth R. Koedinger, professor of human-computer interaction and psychology at Carnegie Mellon University, is the founder and current director of the Pittsburgh Learning Science Center, with 2,112 citations.
The data represented in Figure 4 are the KeyWords Plus count. They were generated from words or phrases that frequently appear in the articles' references but do not appear in the article's title. Using R and the Bibliometrix plugin, it is possible to obtain them. KeyWords Plus enhances the power of cited reference searching by looking across disciplines for all articles with commonly cited references.
Garfield claimed that Keywords Plus terms could capture an article's content with greater depth and variety (Garfield and Sher, 1993). However, Keywords Plus is as effective as Author Keywords in the bibliometric analysis of the knowledge structure of scientific fields, but it is less comprehensive in representing an article's content (Zhang et al., 2016).
In Figure 4, computer-aided instruction is the main topic, representing 17% of the frequencies examined in the text references. Finally, for the elaboration of Figure 5, it was considered that co-occurrences could be normalized using similarity measures such as the Salton cosine, the Jaccard index, the equivalence index, and the strength of association (van Eck and Waltman, 2009).
The selected algorithm was the strength of the association since it is proportional to the relationship between the observed number of co-occurrences of objects i and j and the expected number of co-occurrences of objects i and j under the assumption that the occurrences of i and j are statistically independent.
For the grouping strategy, “Walktrap” was selected as one of the best alongside “Louvain” (Lancichinetti et al., 2010). The graph is interpreted by considering the following characteristics:
• Centrality/periphery (position).
• Dimension of the bubble (number of citations).
• Strength of relationships (links).
• Clusters (and density).
• Bridges.
The colors represent the groups to which each word belongs. In this case, there are three groups. In the first one, colored in red, the theme of computer-aided instruction is dominant in citations. Citation is not the central theme in the green one but relationships, that is, Expert Systems relating topics of interest such as artificial intelligence. Finally, the third group, colored blue, seems to be a subgroup of the first one focused on educational issues.
The search string was as follows: TITLE-ABS-KEY (*intelligent tutoring system*) AND [LIMIT-TO (DOCTYPE,“ar”)] AND [LIMIT-TO (PUBYEAR,2021) OR LIMIT-TO (PUBYEAR,2020) OR LIMIT-TO (PUBYEAR,2019) OR LIMIT-TO (PUBYEAR,2018) OR LIMIT-TO (PUBYEAR,2017) OR LIMIT-TO (PUBYEAR,2016) OR LIMIT-TO (PUBYEAR,2015) OR LIMIT-TO (PUBYEAR,2014) OR LIMIT-TO (PUBYEAR,2013) OR LIMIT-TO (PUBYEAR,2012) OR LIMIT-TO (PUBYEAR,2011) OR LIMIT-TO (PUBYEAR,2010) OR LIMIT-TO (PUBYEAR,2009) OR LIMIT-TO (PUBYEAR,2008) OR LIMIT-TO (PUBYEAR,2007) OR LIMIT-TO (PUBYEAR,2006) OR LIMIT-TO (PUBYEAR,2005) OR LIMIT-TO (PUBYEAR,2004) OR LIMIT-TO (PUBYEAR,2003)].
Text mining
Although the bibliometric analysis found the authors and journals with the most impact in the specific field and the possible thematic fields based on the analysis of the Keywords Plus and the classification of these in groups, it was necessary to perform additional analysis to identify more specific thematic groups, for which the Software KNIME (Berthold et al., 2009) was used.
Figure 6A shows the scheme under which the database downloaded from Scopus was processed. Data were previously filtered from 2003, when a production peak occurred, which is of interest. Finally, in this analysis, all the abstracts of the selected papers were considered.
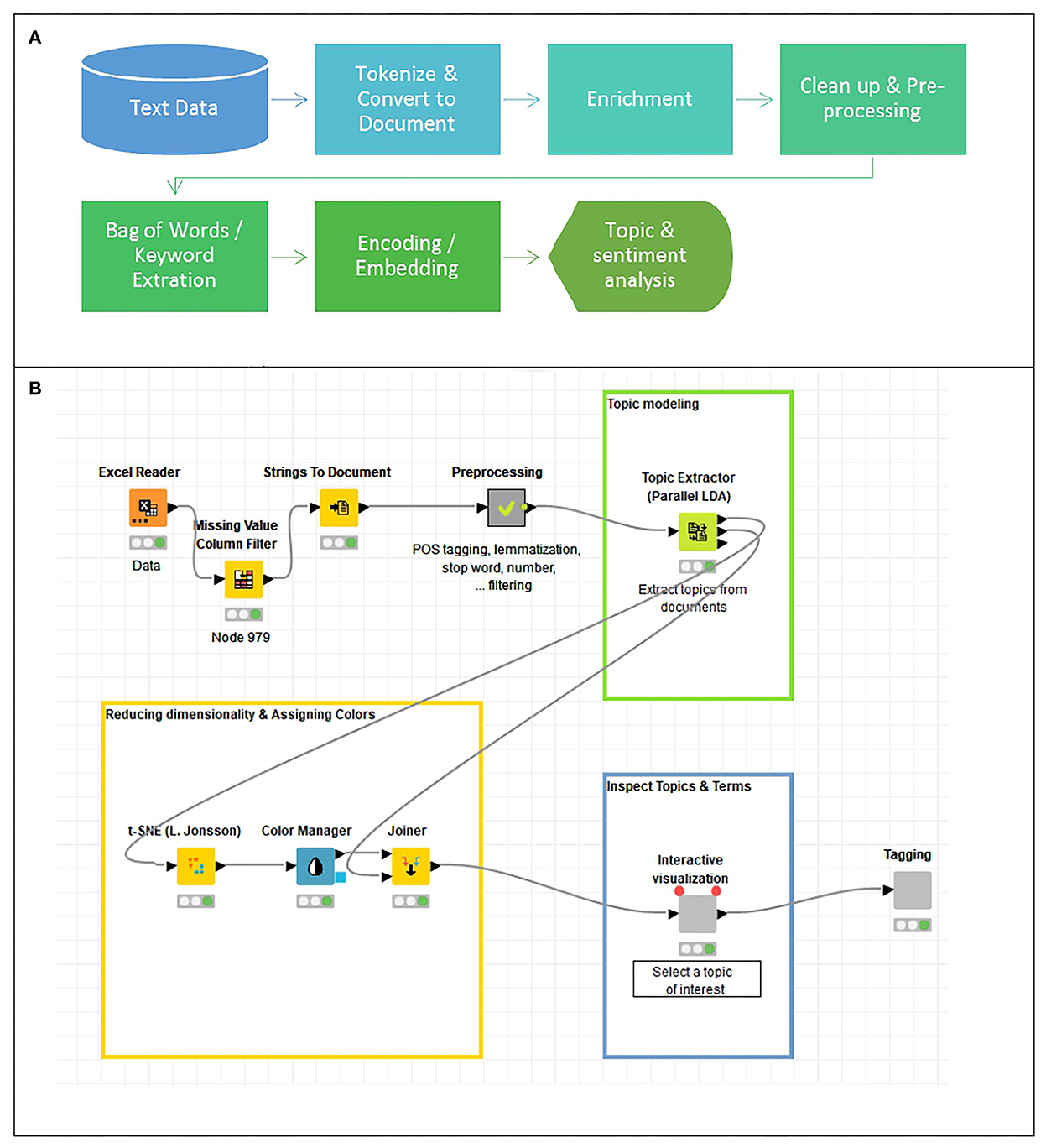
Figure 6. (A) Co-occurrence of words in a flow-diagram and (B) Co-occurrence of words in Keyword Plus from all sources.
Figure 6B shows the workflow developed in KNIME, with which it was possible to analyze 1,369 abstracts and extract the hidden thematic structure, identifying the topics that best describe a set of documents.
Table 2 describes each item presented in Figure 6B.
After going through this process, the algorithm returned all the selected terms, which were classified into five topics from the 1,369 abstracts; each topic required interpretation. However, the focus of the analysis was to determine if some of them were related to the category of interest: evaluation.
The program interface allowed the analyst to explore the five topics, as shown in Figure 7.
For example, topic_0 contains the terms game, instruction, intelligent, language, reading, skill, strategy, study, and system. In the “document” column, the text and the contribution weight for each of the terms were displayed.
The topic_3, represented in yellow in Figure 8, emerges naturally among the analyzed abstracts. The terms that compose it are affective, assessment, data, emotion, method, model, performance, result, student, and system, all of which have high values for this studio.
Data extraction
The results with high values were used as the selection criteria to link the full texts analyzed in NVivo in the next phase. From the text mining of the emerging group represented in Figure 9A, 163 papers were selected. It is essential to consider that the weight of the term assessment is not high compared to the other terms identified in topic_3 and even less compared to the total number of identified terms, as shown in Figure 9B.
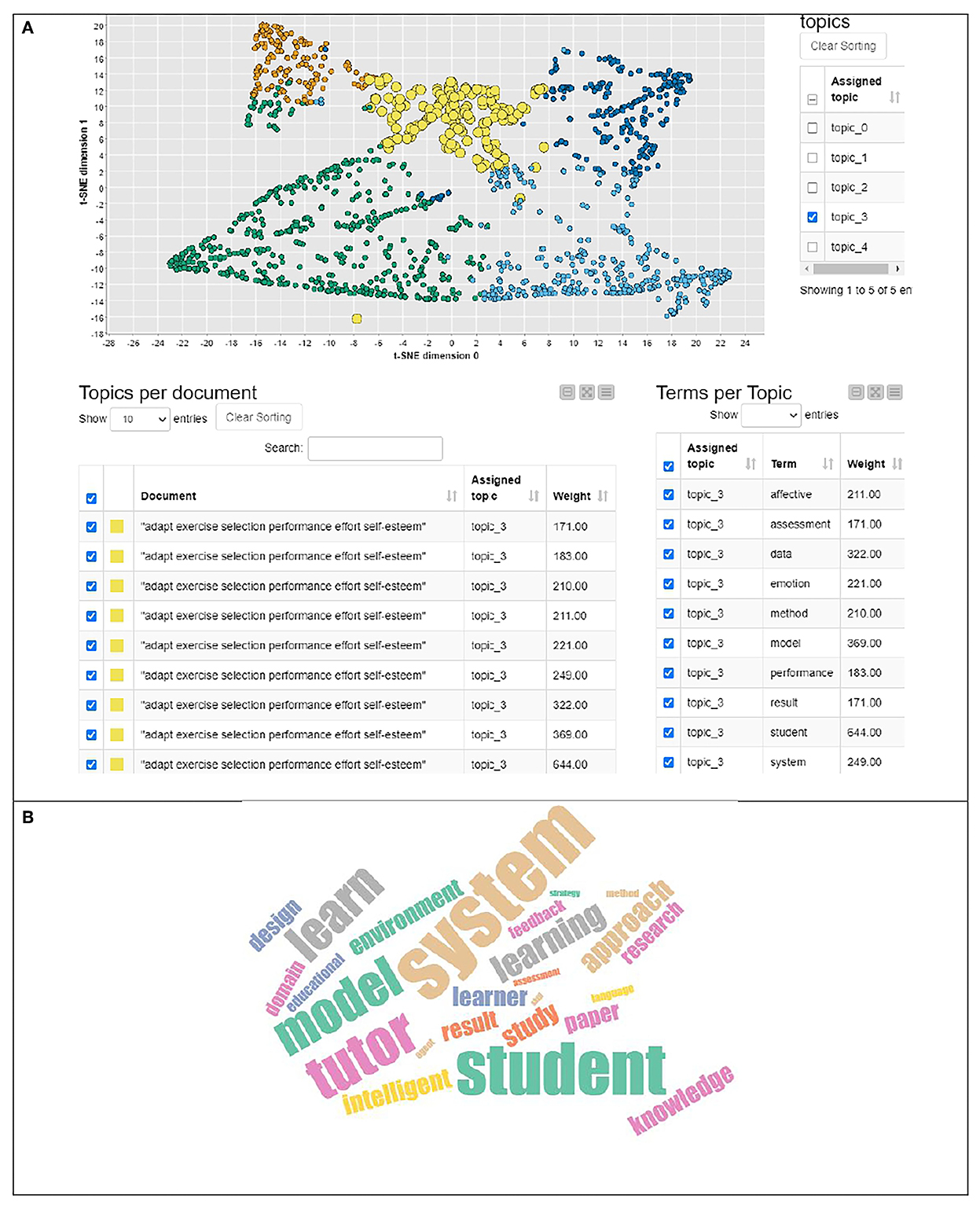
Figure 9. (A) Inspect topics and terms, highlighting topic 3, related to the assessment and (B) Inspect topics and terms as a word cloud.
Results
In this section, a year-wise representation is given in Figure 10.
These results were characterized by research questions posed earlier in this study. The variables of selected studies are presented in Table 3.
• Q1: What is the main purpose of the evaluation in these ITS?
• Q2: What is the main evaluating agent (in evaluation processes)?
• Q3: What is the main approach used in the selected ITS?
• Q4: Is the ITS evaluation process implemented holistically?
To answer these questions, three pillars were considered, namely, each selected paper's purpose, agent, and evaluation approach. Using the NVivo program (NVivo, 2020), a case has been created for each. Subsequently, the percentages of their presence in the selected complete papers have been identified with a search matrix. Finally, considering that a proper holistic evaluation uses all the pillars comprehensively, the holistic column has been completed, with the finding that none of the studies possess the simultaneous presence of all the sub-variables. Table 4 summarizes the results and identifies whether the study was holistic or not. The continuation of Table 4 is presented in Annex A.
Next, we present each research question and its results.
• Q1: What is the main purpose of the evaluation in these ITS?
According to the data found, the primary purpose of the evaluation is summative; that is, most of the evaluation sections in the ITS analysis tried to establish reliable balances of the results obtained, focusing on the collection of information and the elaboration of instruments that allow reliable measurements of the knowledge to be evaluated at the end of a teaching-learning process.
• Q2: What is the main evaluating agent (in evaluation processes)?
The main evaluating agents were those external to the student or their peers; that is, hetero evaluation was prioritized. This is consistent with the purpose found in question 1. Most ITS identify gaps or “weak spots” that need to be reinforced before moving forward with the program and design redress activities aimed at the group or individuals who require it.
• Q3: What is the main approach used in the selected ITS?
The main approach was quantitative, which makes sense since smart tutors use data to achieve process automation. However, qualitative approaches were evidenced to a lesser extent, and in some cases, both were used due to the technological development that allows emotional interpretation and the participants' language.
• Q4: Is the evaluation process implemented in ITS holistic?
To answer this question, the criterion was the following: in each of the selected papers, diagnostic, formative, and summative evaluation elements were sought. Whether the STI used hetero evaluation, peer review, or self-assessment was also tracked. Furthermore, it was determined whether it integrated qualitative and quantitative approaches. All this accounted for a holistic assessment that favors deep learning. Texts that met all these criteria would be classified as holistic.
Under the criteria applied, it is possible to affirm that holistic designs were not found in the analyzed texts. Mainly, special attention is required for the diagnostic and formative evaluations. Furthermore, it is also necessary to encourage the participation of other agents in the evaluation processes of ITS, specifically peer evaluation and the participation of other actors, such as the family. Finally, the mixed approach can enrich the reading of the process; the qualitative evaluative aspects in ITS are a technical challenge; however, these can be included through professionally trained bots.
Emerging challenges
Based on Table 4, it was possible to identify the analysis foci and propose the following challenges.
Demonstrate the pedagogical value of scaffolding by intelligent tutors
According to Arevalillo-Herráez et al. (2017), to facilitate problem-centered instructional models, scaffolding is necessary, that is, contingent support from another more capable person who helped others solve complex problems and acquire valuable skills in doing so; these include deep content learning, argumentation skills, and problem-solving skills. Providing this type of coaching traditionally requires small groups and personalized training processes.
Using intelligent tutoring systems, it is possible to provide this support in large groups; however, the expected learning outcomes of scaffolding respond to different variables, such as cognitive, motivational, or metacognitive aspects. In the cognitive aspects, it has been found that intelligent tutoring systems favor noteworthy progress. However, the motivational and metacognitive aspects require further research to demonstrate their pedagogical value. This can be evidenced by the priority given in the selected full texts to evaluating summative aspects.
Link an efficient evaluation mechanism
Current trends indicate that online learning has become a vital learning mode; however, the analyzed texts did not identify a holistic evaluation mechanism.
The learning performance assessment assess what students learn during the process. It is usually summative or formative; however, both have been confused with the rating in some ITS, focusing on materializing a numerical value. This is clearly due to the learning framework in which each research is inscribed. However, to mobilize higher thinking skills such as problem-solving, critical thinking, or creativity (typical of deep learning), and according to the results found in Table 4, it is necessary to complement this approach with qualitative approaches.
Use multiple data sources
The fundamental challenges to address when considering an intelligent tutor are usually the data sources to feed the predictive models, which come from the summative assessment, such as the result of exercise A or the performance in unit B (Anderson et al., 2011). However, it is crucial to determine the pedagogical value of the actions that led to these results and their implications in predicting the participants' performance (Penumatsa et al., 2006).
The need to link e-learning environments with intelligent tutoring systems
In large-scale courses, for example, accurate and meaningful evaluation is a demanding task for tutors. Assessment of students' performance on exercises could delay the tutor's feedback to students for days or even weeks. Then, sometimes, tutors may have to reduce the number of assignments given to their students due to time constraints. Moreover, achieving accuracy is often challenging for subjective and objective reasons.
Possible solutions to the emerging challenges
In the above discussion, several challenges were identified. To address them, the following research challenges are posed.
Understand and implement the difference between evaluating and grading
Intelligent tutoring systems require moving toward an interpretation of the numerical results, which allows for feedback as proposed by Daniel Wilson, director of the “Zero” project at Harvard University, who indicates that the feedback process consists of the following four ascending phases: clarify, value, express concerns, and make suggestions, which allows focusing on communication with the student in the construction of meaning toward the achievement of deep learning (Krechevsky et al., 2013). Currently, developments have focused on grading.
Designing a holistic framework
The theory of conscious processes, elaborated by Álvarez de Zayas (2010), is of a systemic, holistic, and dialectical nature, that is, complex. It presents a redefinition of the school as a space where teaching and systematization would eventually lead to the training process essentially. This is currently ratified by Schildkamp et al. and Shemchack and Spector, who agree that evaluation can be understood in a systemic, articulated, holistic, and dialectical manner (Schildkamp et al., 2020; Shemshack and Spector, 2020). Teachers need to move easily between diagnostic, formative, and summative approaches at the evaluation time. Focusing only on instruments that lead to a numerical assessment is not enough, since these results are important sources of information about teaching and learning processes, but they need to be complemented with peer or self-assessment tools that include aspects related to purpose, extension, evaluating agents, moments, approaches, and standards of comparison. These dialectically produced instruments favor cognitive and metacognitive processes.
Focus on the process, not just the outcome
To provide a solution to this aspect, ITS must move toward formative evaluation, which implies collecting, analyzing, and identifying student progress (learning monitoring) and reflecting, providing feedback, reorienting, and creating support strategies for students (pedagogical use of the results). The latter is a technological challenge, which implies training the ITS not only with quantitative data.
Implement learning analytics systems that impact the curriculum
When the evaluation process is done correctly, changes to the curriculum emerge naturally, enabling the student to access authentic deep learning. This line of research would imply establishing a framework that allows artificial intelligence to detect new learning goals for the students based on the analysis of mixed data.
Conclusion
The use of text mining was fundamental to extracting knowledge from a wide field of academic production. Other researchers in different fields can use the workflow adapted in KNIME to optimize reading time and focus attention only on the aspects of interest.
Based on intelligent tutors' research, it was possible to identify that progress has been made in detecting concepts that require further study in the constant feedback given to students and teachers in a personalized and automatic way. First, however, it is necessary to propose a framework that offers mixed feedback to students and teachers and facilitates decision-making based on implementing predictive methods, an evaluation that transcends the grading, which is possible due to the fusion between pedagogical and technological aspects.
Deep learning seeks to give meaning to new information; that is, it aims to incorporate critical perspectives on specific learning and, in doing so, favors its understanding to allow its long-term retention. Achieving it requires moving toward a complex evaluation that involves different evaluation forms, actors, moments, approaches, and analyses.
The ITS requires moving toward interpreting the numerical results, allowing communication with the student to focus on constructing meaning toward a holistic evaluation. This holistic evaluation includes the student's participation and peers' diagnostic, formative, and summative aspects. These changes will allow it to account for the depth of learning achieved.
Moving toward this type of evaluation involves analyzing quantitative and qualitative variables. Therefore, it is necessary to create a framework that allows artificial intelligence to integrate all these variables and effectively communicate its results. In other words, an ITS is required to assess and measure all variables related to deep learning and achieve a truly holistic assessment.
Data availability statement
Publicly available datasets were analyzed in this study. This data can be found at: https://docs.google.com/spreadsheets/d/1suQHtzhovtvXITpv-C_KCMSpoJbOudDz/edit?usp=sharing&ouid=10357276 3070360419912&rtpof=true&sd=true.
Author contributions
All authors listed have made a substantial, direct, and intellectual contribution to the work and approved it for publication.
Funding
Completion of the current review was funded by the School of Engineering and Sciences, Tecnológico de Monterrey.
Acknowledgments
The first version of this research was preprinted at Research Square [https://doi.org/10.21203/rs.3.rs-673038/v1]. The authors thank the reviewers for their valuable comments and suggestions that helped improve the paper's quality.
Conflict of interest
The authors declare that the research was conducted in the absence of any commercial or financial relationships that could be construed as a potential conflict of interest.
Publisher's note
All claims expressed in this article are solely those of the authors and do not necessarily represent those of their affiliated organizations, or those of the publisher, the editors and the reviewers. Any product that may be evaluated in this article, or claim that may be made by its manufacturer, is not guaranteed or endorsed by the publisher.
Supplementary material
The Supplementary Material for this article can be found online at: https://www.frontiersin.org/articles/10.3389/feduc.2022.1047853/full#supplementary-material
References
Abbasi, A. R., Dailey, M. N., Afzulpurkar, N., and Uno, T. (2010). Student mental state inference from unintentional body gestures using dynamic Bayesian networks. J. Multimodal User Interfaces 3, 21–31. doi: 10.1007/s12193-009-0023-7
Abdi, A., and Idris, N. (2014). “Automated summarization assessment system: quality assessment without a reference summary,” in The International Conference on Advances in Applied Science and Environmental Engineering—ASEE (Kuala Lumpur, Malaysia). doi: 10.15224/978-1-63248-004-0-89
Alepis, E., Virvou, M., and Kabassi, K. (2008). Requirements analysis and design of an affective bi-modal intelligent tutoring system: the case of keyboard and microphone. Stud. Comput. Intell. 104, 9–24. doi: 10.1007/978-3-540-77471-6_2
Al-Hanjori, M. M., Shaath, M. Z., and Abu-Naser, S. S. (2017). Learning Computer Networks using Intelligent Tutoring System. Gaza.
Alqahtani, F., Katsigiannis, S., and Ramzan, N. (2021). Using wearable physiological sensors for affect-aware intelligent tutoring systems. IEEE Sens. J. 21, 3366–3378. doi: 10.1109/JSEN.2020.3023886
Álvarez, J. M. (2001). “El campo semántico de la evaluación. Más allá de las definiciones,” Evaluar para conocer, examinar para excluir, (1985). Available online at: http://farq.edu.uy/estructura/unidades_de_gestion/uap/matevalaprend/JuanManuelAlvarez~Mendez.pdf (accessed May 5, 2021).
Anderson, J., Betts, S., Ferris, L. J., Fincham, J. M., and Yang, J. (2011). Using brain imaging to interpret student problem solving. IEEE Intell. Syst. 26, 22–29. doi: 10.1109/MIS.2011.57
Anderson, J. R. (2012). Tracking problem solving by multivariate pattern analysis and Hidden Markov Model algorithms. Neuropsychologia 50, 487–498. doi: 10.1016/j.neuropsychologia.2011.07.025
Anderson, J. R., Betts, S., Ferris, J. L., and Fincham, J. M. (2012). Tracking children's mental states while solving algebra equations. Hum. Brain Mapp. 33, 2650–2665. doi: 10.1002/hbm.21391
Anohina, A. (2007). Advances in intelligent tutoring systems: problem-solving modes and model of hints. Int. J. Comput. Commun. Control 2, 48. doi: 10.15837/ijccc.2007.1.2336
Arevalillo-Herráez, M., Marco-Giménez, L., Arnau, D., and González-Calero, J. A. (2017). Adding sensor-free intention-based affective support to an intelligent tutoring system. Knowl. Based Syst. 132, 85–93. doi: 10.1016/j.knosys.2017.06.024
Berthold, M. R., Cebron, N., Dill, F., Gabriel, T. R., Kötter, T., Meinl, T., et al. (2009). KNIME-the Konstanz information miner: version 2.0 and beyond. AcM SIGKDD Explor. Newsl. 11, 26–31. doi: 10.1145/1656274.1656280
Carless, D., and Boud, D. (2018). The development of student feedback literacy: enabling uptake of feedback. Assess. Eval. High. Educ. 43, 1315–1325. doi: 10.1080/02602938.2018.1463354
Castillo, O., Melin, P., Pedrycz, W., and Kacprzyk, J. (2014). Recent advances on hybrid approaches for designing intelligent systems. Stud. Comput. Intell. 551. doi: 10.1007/978-3-319-05170-3
Castillo-Sánchez, M., Gamboa-Araya, R., and Hidalgo-Mora, R. (2020). Factores que influyen en la deserción y reprobación de estudiantes de un curso universitario de matemáticas. Uniciencia 34, 219–245. doi: 10.15359/ru.34-1.13
Chen, W., Mostow, J., and Aist, G. (2013). Recognizing young readers' spoken questions. Int. J. Artif. Intell. Educ. 21, 255–269. doi: 10.3233/JAI-130031
Chufama, M., and Sithole, F. (2021). The pivotal role of diagnostic, formative and summative assessment in higher education institutions ' teaching and student learning. Int. J. Multidiscip. Res. Publ. 4, 5–15. Available online at: http://ijmrap.com/wp-content/uploads/2021/10/IJMRAP-V4N4P107Y21.pdf
Cuéllar Rojas, O. A. (2013). Validación de una Propuesta Evaluativa Integral Para el curso de Cálculo Diferencial de la Universidad Nacional sede Medellín, Basada en el uso de un LMS (Learning Mannager System), Moodle. Medellín: Facultad de Ciencias.
Garfield, E., and Sher, I. H. (1993). Key words plus [TM]-algorithmic derivative indexing. J. Am. Soc. Inf. Sci. 44, 298. doi: 10.1002/(SICI)1097-4571(199306)44:53.0.CO;2-A
Gibb, H., Haver, C., Gaylor, D., Ramasamy, S., Lee, J. S., Lobdell, D., et al. (2011). Utility of recent studies to assess the National Research Council 2001 estimates of cancer risk from ingested arsenic. Environ. Health Perspect. 119, 284–290. doi: 10.1289/ehp.1002427
Gobert, J. D., Kim, Y. J., Sao Pedro, M. A., Kennedy, M., and Betts, C. G. (2015). Using educational data mining to assess students' skills at designing and conducting experiments within a complex systems microworld. Think. Skills Creat. 18, 81–90. doi: 10.1016/j.tsc.2015.04.008
Guzmán, E., and Conejo, R. (2005). Self-assessment in a feasible, adaptive web-based testing system. IEEE Trans. Educ. 48, 688–695. doi: 10.1109/TE.2005.854571
Hamodi, C., López-Pastor, V. M., and Pastor, A. T. L. (2014). Medios, técnicas e instrumentos de evaluación formativa y compartida del aprendizaje en educación superior. Perfiles Educ. 37, 146–161. doi: 10.22201/iisue.24486167e.2015.147.47271
Jraidi, I., and Frasson, C. (2013). Student's uncertainty modeling through a multimodal sensor-based approach. Educ. Technol. Soc. 16, 219–230. Available online at: http://www.jstor.org/stable/jeductechsoci.16.1.219
Kabanza, F., and Rousseau, K. (2005). Teaching while selecting images for satellite-based forest mapping. Int. J. Knowl. Based Intell. Eng. Syst. 9, 183–189. doi: 10.3233/KES-2005-9302
Kaser, S., and Gütl, C. (2016). Informe Sobre Permanencia de Estudiantes en MOOCs (Attrition and Retention Aspects in MOOC Environments). Austria.
Kaya, H., Özkaptan, T., Ali Salah, A., and Gurgen, F. (2015). Random discriminative projection based feature selection with application to conflict recognition. IEEE Signal Process. Lett. 22, 671–675. doi: 10.1109/LSP.2014.2365393
Khalfallah, J., and Ben Hadj Slama, J. (2017). “Relevant metrics for facial expression recognition in intelligent tutoring system,” in Lecture Notes in Educational Technology (Singapore), 119–122. doi: 10.1007/978-981-10-2419-1_17
Krechevsky, M., Mardell, B., Rivard, M., and Wilson, D. (2013). Visible Learners: Promoting Reggio-inspired Approaches in All Schools. New York, NY: John Wiley and Sons.
Krivec, J., and Guid, M. (2020). The influence of context on information processing. Cogn. Process. 21, 167–184. doi: 10.1007/s10339-020-00958-8
Kuk, K. V., Milentijevic, I., Randelović, D. M., Popovic, B., and Císar, P. (2017). The design of the personal enemy - MIMLebot as an intelligent agent in a game-based learning environment. Acta Polytech. Hung. 14, 121–139. Available online at: https://epa.oszk.hu/02400/02461/00073/pdf/EPA02461_acta_polytechnica_hungarica_2017_04_121-139.pdf
Lancichinetti, A., Kivelä, M., Saramäki, J., and Fortunato, S. (2010). Characterizing the community structure of complex networks. PloS ONE 5, e11976. doi: 10.1371/journal.pone.0011976
Lemke, C. (2013). Intelligent Adaptive Learning. Vendor Supplied Whitepaper. Available online at: http://www-static.dreambox.com/wp-content/uploads/2013/03/white-paper-intelligent-adaptive-learning-21st-century-teaching-and-learning.pdf
Litman, D. J., and Forbes-Riley, K. (2006). Recognizing student emotions and attitudes on the basis of utterances in spoken tutoring dialogues with both human and computer tutors. Speech Commun. 48, 559–590. doi: 10.1016/j.specom.2005.09.008
Moher, D., Shamseer, L., Clarke, M., Ghersi, D., Liberati, A., Petticrew, M., et al. (2015). Preferred reporting items for systematic review and meta-analysis protocols (PRISMA-P) 2015 statement. Syst. Rev. 4, 1–9. doi: 10.1186/2046-4053-4-1
Moradi, H., Moradi, S., and Kashani-Vahid, L. (2014). Students' performance prediction using multi-channel decision fusion. Stud. Comput. Intell. 524, 151–174. doi: 10.1007/978-3-319-02738-8_6
Moridis, C. N., and Economides, A. A. (2009). Prediction of student's mood during an online test using formula-based and neural network-based method. Comput. Educ. 53, 644–652. doi: 10.1016/j.compedu.2009.04.002
Mostow, J., and Beck, J. (2006). Some useful tactics to modify, map and mine data from intelligent tutors. Nat. Lang. Eng. 12, 195–208. doi: 10.1017/S1351324906004153
Muldner, K., and Burleson, W. (2015). Utilizing sensor data to model students' creativity in a digital environment. Comput. Hum. Behav. 42, 127–137. doi: 10.1016/j.chb.2013.10.060
Nielsen, R. D., Ward, W., and Martin, J. H. (2009). Recognizing entailment in intelligent tutoring systems. Nat. Lang. Eng. 15, 479–501. doi: 10.1017/S135132490999012X
NVivo, Q. S. R. (2020). NVivo Qualitative Data Analysis Software. Melbourne, VIC: QSR International Pty Ltd.
Olsen, J. K., Sharma, K., Rummel, N., and Aleven, V. (2020). Temporal analysis of multimodal data to predict collaborative learning outcomes. Br. J. Educ. Technol. 51, 1527–1547. doi: 10.1111/bjet.12982
Paaßen, B., Göpfert, C., and Hammer, B. (2018). Time series prediction for graphs in Kernel and dissimilarity spaces. Neural Process. Lett. 48, 669–689. doi: 10.1007/s11063-017-9684-5
Penumatsa, P., Ventura, M., Graesser, A. C., Louwerse, M., Hu, X., Cai, Z., et al. (2006). The right threshold value: what is the right threshold of cosine measure when using latent semantic analysis for evaluating student answers? Int. J. Artif. Intell. Tools 15, 767–777. doi: 10.1142/S021821300600293X
Rajendran, R., Iyer, S., and Murthy, S. (2019). Personalized affective feedback to address students' frustration in ITS. IEEE Trans. Learn. Technol. 12, 87–97. doi: 10.1109/TLT.2018.2807447
Rehhali, M., Mazouak, A., and Belaaouad, S. (2022). The digital assessment of learning: current situation and perspectives: case of teachers of life and earth sciences. J. Inf. Technol. Manag. 14, 65–78. doi: 10.22059/jitm.2022.87534
Rus, V., and Stefanescu, D. (2016). “Toward non-intrusive assessment in dialogue-based intelligent tutoring systems,” in Lecture Notes in Educational Technology (Singapore), 231–241. doi: 10.1007/978-981-287-868-7_26
Sanz Garcia, M. T., González-Calero, J. A., and Arevalillo-Herráez, M. (2019). Using reading comprehension to build a predictive model for the fourth-grade grade students' achievement when solving word problems in an intelligent tutoring system. Rev. Educ. 2019, 41–69. doi: 10.4438/1988-592X-RE-2019-384-409
Šarić-Grgić, I., Grubišić, A., Šerić, L., and Robinson, T. R. (2020). Student clustering based on learning behavior data in the intelligent tutoring system. Int. J. Distance Educ. Technol. 18, 73–89. doi: 10.4018/IJDET.2020040105
Schildkamp, K., van der Kleij, F. M., Heitink, M. C., Kippers, W. B., and Veldkamp, B. P. (2020). Formative assessment: a systematic review of critical teacher prerequisites for classroom practice. Int. J. Educ. Res. 103, 101602. doi: 10.1016/j.ijer.2020.101602
Shah, D. (2020). Class Central, By the numbers: MOOCs in 2020. Available online at: https://www.classcentral.com/report/mooc-stats-2020/ (accessed June 06, 2021).
Shemshack, A., and Spector, J. M. (2020). A systematic literature review of personalized learning terms. Smart Learn. Environ. 7, 33. doi: 10.1186/s40561-020-00140-9
Snow, E. L., Likens, A. D., Allen, L. K., and McNamara, D. S. (2016). Taking control: stealth assessment of deterministic behaviors within a game-based system. Int. J. Artif. Intell. Educ. 26, 1011–1032. doi: 10.1007/s40593-015-0085-5
Sudakova, N. E., Savina, T. N., Masalimova, A. R., Mikhaylovsky, M. N., Karandeeva, L. G., Zhdanov, S. P., et al. (2022). Online formative assessment in higher education: bibliometric analysis. Educ. Sci. 12:607–616. doi: 10.3390/educsci12030209
Tan, J. S. H., and Chen, W. (2022). Peer feedback to support collaborative knowledge improvement: what kind of feedback feed-forward? Comput. Educ. 187, 104467. doi: 10.1016/j.compedu.2022.104467
Ting, C. Y., and Phon-Amnuaisuk, S. (2012). Properties of Bayesian student model for INQPRO. Appl. Intell. 36, 391–406. doi: 10.1007/s10489-010-0267-7
Torres Mancera, D., and Gago Saldaña, D. (2014). Los moocs y su papel en la creación de comunidades de aprendizaje Y participación. Rev. Iberoam. Educ. Distancia 17, 13–34. doi: 10.5944/ried.17.1.11570
Torres-Madroñero, E. M., Torres-Madroñero, M. C., and Botero, L. D. R. (2020). Challenges and possibilities of ICT-mediated assessment in virtual teaching and learning processes. Future Internet 12, 1–20. doi: 10.3390/fi12120232
Van Amelsvoort, M., Joosten, B., Krahmer, E., and Postma, E. (2013). Using non-verbal cues to (automatically) assess children's performance difficulties with arithmetic problems. Comput. Human Behav 29, 654–664. doi: 10.1016/j.chb.2012.10.016
van Eck, N. J., and Waltman, L. (2009). How to normalize cooccurrence data? An analysis of some well-known similarity measures. J. Am. Soc. Inf. Sci. Technol. 60, 1635–1651. doi: 10.1002/asi.21075
Whitehill, J., Serpell, Z., Lin, Y.-C., Foster, A., and Movellan, A. R. (2014). The faces of engagement: automatic recognition of student engagement from facial expressions. IEEE Trans. Affect. Comput. 5, 86–98. doi: 10.1109/TAFFC.2014.2316163
Yang, F., and Li, F. W. B. (2018). Study on student performance estimation, student progress analysis, and student potential prediction based on data mining. Comput. Educ. 123, 97–108. doi: 10.1016/j.compedu.2018.04.006
Yang, S., Ding, S., and Yao, Z. (2009). The theory about CD-CAT based on FCA and its application. Int. J. Distance Educ. Technol. 7, 61–78. doi: 10.4018/jdet.2009062404
Zhang, J., Zheng, F., Long, C., Lu, Z., and Duan, Z. (2016). Comparing keywords plus of WOS and author keywords: a case study of patient adherence research. J. Assoc. Inf. Sci. Technol. 67, 967–972. doi: 10.1002/asi.23437
Keywords: tutoring system, bibliometric analysis, literature review, text mining, educational innovation
Citation: Cuéllar-Rojas O-A, Hincapié-Montoya M, Contero M and Güemes-Castorena D (2022) Bibliometric analysis and systematic literature review of the intelligent tutoring systems. Front. Educ. 7:1047853. doi: 10.3389/feduc.2022.1047853
Received: 18 September 2022; Accepted: 02 November 2022;
Published: 21 December 2022.
Edited by:
Clifford A. Shaffer, Virginia Tech, United StatesReviewed by:
Cucuk W. Budiyanto, Sebelas Maret University, IndonesiaSyamsul Nor Azlan Mohamad, MARA University of Technology, Malaysia
Copyright © 2022 Cuéllar-Rojas, Hincapié-Montoya, Contero and Güemes-Castorena. This is an open-access article distributed under the terms of the Creative Commons Attribution License (CC BY). The use, distribution or reproduction in other forums is permitted, provided the original author(s) and the copyright owner(s) are credited and that the original publication in this journal is cited, in accordance with accepted academic practice. No use, distribution or reproduction is permitted which does not comply with these terms.
*Correspondence: Mauricio Hincapié-Montoya, maurhin@eafit.edu.co; David Güemes-Castorena, guemes@tec.mx
†These authors have contributed equally to this work and share first authorship
‡These authors share senior authorship