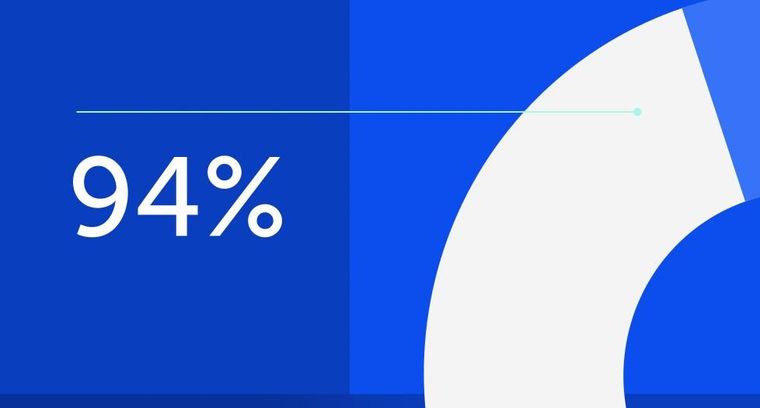
94% of researchers rate our articles as excellent or good
Learn more about the work of our research integrity team to safeguard the quality of each article we publish.
Find out more
ORIGINAL RESEARCH article
Front. Educ., 18 November 2022
Sec. Assessment, Testing and Applied Measurement
Volume 7 - 2022 | https://doi.org/10.3389/feduc.2022.1043471
Use of quantile regression, analysis at different levels of aggregation, and inclusion of covariates were used to assess how different methodologies produced varying results with contradictory implications for policy interventions regarding the relation between school funding and achievement. Results indicated that significantly different relations existed at various quantiles of the distribution of achievement, both for student and school-level outcomes. Further, significant differences were found when outcomes at the school-level were compared to outcomes for individual students. Finally, the inclusion of SES drastically altered the results of every analysis in this study, indicating the importance of controlling for confounds. Taking all of these findings into account, we find that higher levels of funding at the student-level were associated with smaller gaps in growth in achievement by SES for lower achieving students. The opposite finding was found at the school level, with higher levels of funding at the school level being associated with larger gaps in growth in achievement based on SES. We compare our findings to results of existing studies, framing our results and interpretations thereof in weighted-student funding literature. Discussion on how these findings can be translated into quasi-experimental research designs are also included.
The relation between school-funding and academic achievement has been studied for decades. Key early studies such as the Coleman et al. (1966) and Jencks’s (1972) reports, as well as most literature prior to 1995 (Jackson, 2020), showed very weak relations between student academic achievement. However, this relation, present or not, is one that appears to be more complex and differential dependent on several factors. Confounding variables, different relations based on a school’s prior achievement or level of funding, and level of aggregation can all potentially play significant roles in altering the observed relation between funding and achievement. Recent research in the area has aimed to take these factors and other such into account, ushering in an era of more advanced research designs and methodological approaches. Recent work has moved away from the more correlational body of work towards more direct quasi-experimental research (Jackson et al., 2016; Abott et al., 2020), finding clearer positive relations between funding achievement. Despite this, quasi-experimental designs are often more cumbersome and time intensive to conduct then cross-sectional correlational studies. Correlational studies can still be useful when conducted correctly as an inductive body of research, pointing towards areas where future research needs to be conducted. This paper aims to serve as a study in how choices in methodological design for cross-sectional studies can lead to drastically different results, as well as to provide cross-sectional evidence on how relations between funding and achievement may differ based on level of aggregation, prior student achievement, and the inclusion of SES as a covariate.
Supporting the idea that different relations between funding and achievement may exist dependent upon methodological choices made, some work has suggested that the impact funding has on student performance may vary largely for different groups or due to multiple moderators (Hanushek, 1989; Hanushek, 1997). One such variable that appears to moderate the association of funding to student achievement is socioeconomic status (SES), which has been shown to directly moderate the association in two distinct ways. First, the SES composition of a school often plays a role in determining the amount of funding a school receives and is subsequently able to be put towards instructional or other costs. Second, the overall SES of a school or the individual SES of a student are both consistently found to be moderate correlates of student and school performance (White,1982; Sirin, 2005). In addition to these two direct ways SES moderates the relation between funding and student achievement, SES also likely has a role in more complicated ways. For example, the SES of an individual student or of the students comprising a school could moderate the possible level of impact that funding increases has on said student or school. It is also possible that the relations between funding, SES, and student performance may differ based on whether or not the analysis is done on the student- or aggregated school-level (Fertig and Wright, 2005), or on how low or high achieving a student or school is (Costanzo and Desimoni, 2017). As a result, the model or analysis chosen to examine these relations, and the confounding variables included, may greatly affect the way in which results are interpreted, and in turn what policies are implemented. Seeing if funding is related to achievement in specific contexts can help infer research using more rigorous designs and can point towards potential areas in which differential impacts of funding on achievement may exist.
Though a significant body of research has been conducted on funding’s relation to student achievement, the lone consistent finding appears to be that this relation is inconsistent and highly differential. Two seminal studies in this field, the Coleman report (1966) and the Jenck’s report (1972), found limited evidence overall for an impact of school funding on student achievement. Subsequent work, including empirical analysis by Sebold and Dato (1981), as well as meta-analyses by Hedges et al. (1994), Borman et al. (2003), and Jeynes (2015), have similarly shown the relation between funding and achievement to be inconsistent and potentially very weak. Consistent across all this work is that the analytical techniques used examined the mean effect of school funding on average achievement, whereas it is widely accepted that this relation is extremely complex (Lockwood and McLean, 1997). Recent advances in analytical tools allows for a more nuanced examination of the relation. This work has shown that school-level at-risk factors including prior levels of student achievement could be linked to how funding impacts achievement within a school. One such article found that the impact of a funding-based educational intervention was significantly higher for at-risk and prior low-performing students than it was for the average or higher performing student (Henry et al., 2010). To continue this newer trend of using advanced analytical techniques to gain deeper understandings of the role of school funding on student achievement, here we introduce a quantile regression approach. Quantile regression is ideal because it will allow us to examine the relation of school funding on student achievement across the distribution of student achievement (Petscher and Logan, 2014).
Compared to the vast majority of papers that have looked at mean estimations of school quality, including school funding, on student performance, far fewer have done so using conditional quantile estimates to gain a deeper understanding of this complex relation. Costanzo and Desimoni (2017) examined several factors related to school inequity as measured by immigrant status, geographical region, and socio-economic status using a quantile regression framework. They found statistically significant differential relations throughout the achievement distribution between academic achievement and the variables related to inequity. Work by Eide and Showalter (1998) directly explored the impact of school quality on student math performance using a smaller cohort and again found that differential effects existed between various school quality variables and achievement throughout the distribution of student academic performance. The use of quantile regression in both studies allowed researchers to estimate impacts of these school quality related variables on achievement separately based on the quantile of achievement or performance a school fell in. This helped to give deeper insight into these complex relations, by examining how these relations differ when a school is low, high, or average achieving. Work by Shero and Hart (2020) used a different method to examine the efficiency of individual schools in spending to promote student growth in the Florida public school system, and found that varying degrees of efficiency existed with implications for the relation between funding and achievement. These varying efficiency scores show that while some schools are getting the most possible out of their current levels of funding and can only increase performance by first increasing funds, others are performing well below the efficient level and for them increased funding may not be necessary for growth. Further, this difference in necessity in increasing funding shows the potential for significantly different impacts of funding overall across these schools.
The significantly strong relation between student, school, and community SES and student achievement has been studied for decades. Meta-analyses by White (1982) and the update by Sirin (2005) have found that on average, the correlation between SES and student achievement is 0.299. More recent studies have shown the same finding, with lower-SES students performing significantly worse than their higher-SES peers on measures of academic achievement in all subjects including math, reading, and science (Lacour and Tissington, 2011; Perera, 2014; Galindo and Sonnenschein, 2015).
Beyond the relation between SES and achievement directly, other research has found SES to be significantly associated with other factors related to student achievement, specifically school funding. It was once the case that schools in higher poverty areas had lower levels of funding, compounding the inequalities that lower-SES students experience compared to higher-SES students (Augenblick et al., 1997). However, due to social changes in school funding decisions, schools with high populations of lower-SES students now typically have higher levels of funding than those with fewer lower-SES students (Urban Institute, 2017). Despite this change, research has shown that the gap between high- and lower-SES students still persists, with some studies having shown the gap to actually be increasing (Lee and Burkham, 2002; Reardon, 2011). The combination of lower-SES being negatively related to achievement but positively related to funding levels, leads to a scenario in which funding appears to be negatively related to student achievement. This scenario not only leads to puzzling relations, but further emphasizes the complex nature of the relation between funding and achievement.
Overall, although the most commonly cited works in the funding literature have shown limited and weak relations between funding and student achievement, recent studies have shown that using more complex models and taking other confounds into account could actually lead to different results. Here we expand this research by using quantile regression to study this relation. Through using quantile regression, we examine the relations of both funding and SES to academic achievement at different levels of achievement for schools and students. Doing so will illustrate how analyses conducted at the mean may differ from those in the lowest areas of the distribution in which we are most interested, and will describe these varying relations on the basis of achievement levels.
The bulk of research conducted on funding has been at the school-level rather than the student-level. This is likely due to funding, and funding reports, being based at the school-level, and individual spending per specific students being typically unavailable. However, only conducting analyses at this level is missing a large part of the picture. Aggregation bias exists when there is a significant difference between the effects found, whether in size or direction, depending on the level of aggregation analyzed (Greenwood and Luloff, 1979; Moorman, 1979). Fertig and Wright (2005) analyzed the relations between school quality variables and achievement at various levels of aggregation, finding significant differences in the results based on each level of analysis. The idea of aggregation bias is nothing new and tends to result in what is known as the ecological fallacy, or the incorrect assumption that findings seen at a broader level will generalize to what is seen at an individual level (Robinson, 1950). As a result, there is little reason to believe research on funding and achievement conducted at the school-level will generalize to the student-level.
This now begs the question of what is the end goal of school-funding policies. If the goal is simply to raise aggregate school-level achievement, then conducting school-level analyses is appropriate. However, if the goal is to target individual students or subgroups of student then only student-level analyses will suffice. Given that a large number of policies are aimed at directly impacting these specific groups of students (i.e., policies related to at-risk students, demographic achievement gaps, or any equity based policy), examining these impacts at the student-level should be more common than it currently is. Further, the policy decisions and evaluations we make should better align with the specific goals of said policies. The extent to which research has been conducted looking at funding directly on student-level achievement is virtually non-existent, however some policies, and studies of said policies, have focused on student-specific funding as an option to most efficiently improve student outcomes. These studies focus largely on a system of funding known as weighted-student-funding (WSF), and the potential it holds for decreasing achievement gaps and boosting student performance. Empirical work by Tuchman et al. (2022) and Baker (2013) supported the idea of WSF, finding that districts employing WSF outperformed control schools in growth and gap-closing rates. Additionally, work by Jarmalowski et al. (2022) found that not only did differing the funding have positive outcomes, but that WSF was effective at ensuring more funds actually reached the most at-risk students. Based on these studies, there is some evidence that funding may impact students differently than schools and that targeting these differences can be effective at improving performance, however the lack of work exploring how funding in general relates to said individual students greatly limits our ability to take such an approach. As such, here we conduct and present alongside each other analyses at both the school- and student-level, aiming to show what differences arise based on this level of aggregation and to provide one of the first studies on the individual differences that exist in student achievement relations to funding levels.
The prior research presented, or lack of prior research in some areas, combined with the focus of this paper, has led us to develop two main research questions. First, does the relation between funding and achievement, while accounting for SES, differ by where in the distribution of achievement a school or student lies? Second, does the relation between funding and achievement at these different quantiles differ based on the SES of the school or student? In addition to these research questions, particular attention was paid to the differences that arise when answering each based on the level of aggregation analyzed.
This study used data from on all public elementary students and schools in Florida for the 2009–2010 school year. This study combined data from two sources, the Florida Progress Monitoring and Reporting Network (PMRN), and the Florida Department of Education’s Program Cost Analysis Series Reports.1 The 2009–2010 school year is the most recent year for which funding data and data on all public elementary students was made available to us, and as such was the year chosen for analysis. Analyses were conducted at both the school and student-level by aggregating student data on achievement and SES for each school. Having access to such a large and robust dataset makes it possible to conduct our analysis at both the school- and student-level.
Participants for this study were all public elementary schools and students in the state of Florida for which funding and achievement data were available. A total of 1,446 schools and 573,869 students were used for analysis, ranging from grades 1 through 6. Students in kindergarten were initially considered for inclusion in the study, however no relevant measure of reading achievement was collected that could have been used for analysis in this study. By grade, students were proportionally represented with 19.9% from grades 1 and 2, 22.1% from grade 3, 19.1% from grade 4, and 18.9% from grade 5, and were almost evenly broken down by sex with students being 48.5 and 51.5% female and male, respectively.
School funding data was obtained via a Florida Department of Education website at see Footnote 1. This source provided per pupil expenditures for each individual school, broken down into various sub-categories: salaries, employee benefits, purchasing services, materials and supplies, capital outlay, and other direct and indirect costs. For this study, we used total program costs as the measure of school funding, which is reported as the sum of all subcategories of funding.
SES was operationalized as student’s free and reduced lunch status, which was available at the student-level in the Florida PMRN. Four possible categories were reported for a student’s free and reduced lunch status: (1) student did not apply, (2) student applied but was ineligible, (3) student is eligible, and 4) student is enrolled in a USDA-approved Provision Z school. We used these categories to create a new, dichotomized SES variable, so that options 1 and 2 were coded as higher-SES (coded as “1”), and options 3 and 4 were coded as lower-SES (coded as “0”). This new dichotomized variable was used at the student-level as a measure of a student’s familial SES. For school-level analysis, student-level SES values were aggregated across each student’s SES in the school, resulting in a measure of the proportion of students within each school on free and reduced lunch.
Achievement for this study was measured through the Florida Assessment for Instruction in Reading (FAIR) reading comprehension subtest, available in the Florida PMRN. For students in grades 1 and 2, students had to read short passages for 1 min, with their final score being number of words correctly read. For students in grades 3–5 students completed computer-adaptive testing consisting of answering questions based on passages of various difficulty, length, and genre. These grade level differences in the reading comprehension subtest were designed purposely, reflecting grade level differences in student reading comprehension learning. FAIR grade 1 and 2 was reported to have a test–retest reliability of Cronbach’s alpha equal to 0.66 and 0.80, respectively, between Fall and Spring measures (Florida Center for Reading Research, 2009). For the current sample specifically and to assess the reliability of the measure over a smaller timeframe than the full year represented in the past reported reliabilities, test–retest reliability between Fall and Winter measures was computed separately for students in grades 1–2 finding a reliability of 0.50, and again for grades 3–5 finding a test-reliability of 0.75. For grades 3–5, FAIR reading comprehension had a generic IRT estimate of reliability ranging from 0.90 to 0.9 (Florida Center for Reading Research, 2009). The reading comprehension test was administered at three separate times throughout the year (Fall, Winter, and Spring assessment) to provide several points of progress monitoring for students. To operationalize student achievement, we calculated student growth across the year by computing standardized residual change scores by residualizing the change from students Fall to Spring scores for each individual student. This residualizing and standardizing was done at each grade level independently to provide more accurate measures of expected versus realized scores. For student-level analysis, these standardized residual change scores were used as the measure of a student’s growth in reading comprehension which was used as a proxy for student growth in educational achievement. Following the computation of student-level residualized gain scores, the student-level results were aggregated at the school-level by calculating a mean score across all students in each school, to allow for analysis of each school’s reading comprehension growth. That is, the average student residualized change score at a given school was used as that school’s measure of average growth. For analysis at the school-level, the school-level mean standardized residual change scores in reading comprehension were used as a measure of school growth in educational achievement.
Quantile regression is a method that allows for estimation of relations at different conditional quantiles of the outcome variable (Koenker, 2005). Quantile regression recognizes that predictors for a given outcome may be more or less important depending on an individual’s given score in said outcome (Koenker and Bassett, 1978). In this case, the outcome variable is a school’s average student growth in achievement measured by a residual change score in reading comprehension, with the effects of interest being those due to funding, SES, and the interaction between funding and SES. Unlike OLS regression which estimates effects only at the mean, this method allows for a deeper analysis, looking more into how specifically these effects vary for different schools. This will be used to conduct the school-level analysis, however a more complex approach must be taken for analysis at the student-level. For this, three separate models will be computed, beginning with Model 1 estimating the impact of funding on student-achievement, Model 2 estimating this again but now including student-level SES as a predictor of student-achievement, and Model 3 building off Model 2 with the inclusion of an interaction term between student-level SES and funding.
Since students are nested within the schools, it is reasonable to assume that the school which they attend may explain a significant portion of their individual growth. For this reason, the same quantile regression approach that was used for school-level analysis will not work for the student analysis. Rather, to control for school-level effects when examining impacts on the student-level, a linear quantile mixed modeling approach was taken. This approach combines methods from hierarchical linear modeling and quantile regression to separate school and student-level effects (Geraci and Bottai, 2014). Through doing this, the impact of a student’s school is accounted for within the model, and the effects on an individual student are estimated. Similar to traditional hierarchical linear modeling, several models were tested until that which best matched the data and aligned with theory was found. The resulting model used was a random-intercept fixed-slope model with effects varying by school. This model indicated that a child’s expected gain may be smaller or larger depending on the school they attend, but that the effect of SES or funding will not differ across schools. More complex models were tested, however these resulted in issues with singularity, and as such this simpler model was used. These more complex models included testing models with both random intercepts and slopes, as well as including both student-level and school-level SES simultaneously.
Descriptive statistics and correlations for the school-level and student-level measures are presented alongside each other in Tables 1, 2. At the school-level, results show that the average school spent $7,811 per pupil (range: $5,303–$15,183) and had 34.6% students of higher-SES. As a reminder, the key outcome was residualized gain scores across a school year, meaning “achievement” was standardized change, so a mean of 0 and standard deviation of 1 was seen. Raw scores used to calculate the achievement variable are also included. All results were normally distributed, with slight positive skew seen for funding, but not to the extent that it offers any concern. All correlations were statistically significant at p < 0.001, with funding having a moderate negative correlation with both proportion of higher-SES students and achievement. Achievement and proportion of higher-SES students were seen to have a high positive correlation as was expected. At the student-level, results showed that higher SES had a weak to moderate positive relation with achievement as measured by residualized changed, as well as all raw scores, and a weak to moderate negative relation with funding. Funding on the other hand showed a weak negative relation with all achievement variables.
Results for the quantile regression model conducted at the school-level are seen in Table 3, and results for the linear quantile mixed model conducted at the student-level are presented in Table 4.
Table 3. Quantile regression results for predicting school-level residualized change from school funding and schoolwide socioeconomic status.
Table 4. Linear quantile mixed model fixed effects results for predicting student-level residualized change from school funding and student socioeconomic status.
At the school-level for model 1, which measured the association of funding to school achievement, all effects of funding on growth were negative. There was however a clear increasing trend towards becoming less negative when moving from the lower quantiles of achievement upwards. This indicates that the negative impact of funding observed decreases when moving from lower to higher achieving schools. Additionally, this is without controlling for SES and its relation with funding, and thus higher funding in this situation also means higher proportions of lower-SES students. Given the relation between SES and achievement, schools with higher levels of funding therefor are expected to have the lower levels of achievement linked to their higher proportion of lower-SES students. Results from the linear quantile mixed models conducted at the student-level showed that on average, 96.95% of the proportion of variance in achievement was attributable to the student-level, with 3.05% of variance being attributable to the schools. The fixed effects are reported in Table 4. For Model 1, moving from low-achievers to high-achievers, the impact that funding has on the individual student gradually increases from the more negative in the lower quantiles to slightly positive in the highest quantiles, mirroring what was seen in the school-level model. There does appear to be a slight increase in impact of funding at the lowest quantiles in this model (15th and below), however the same general trend is still seen. Effect sizes ranged from −0.11 SD’s to just over 0 SD’s per 1,000 dollars spent per student at the 10th and 95th quantiles, respectively.
For the school-level analyses, in model 2 when SES was added, the same upward trend of funding’s impact on achievement was observed. In fact, in this model, once crossing over the lowest quartile of achieving schools the impact of funding actually shifted from negative to positive, and further increased for the highest achieving schools. This change from the first model is likely due to the inclusion of SES, which was positively associated with achievement yet negatively associated with funding. Here, the positive relation between SES and funding, and the negative relation between SES and achievement are being controlled for. Controlling for these resulted in the negative impact of funding disappearing in most quantiles. For SES, results from model 2 show that having a higher-SES had a positive impact on schools throughout the entirety of the distribution. Moving upwards through the quantiles however, this impact decreased moving from 3.43 SD’s in the 5th quantile down to 2.01 SD’s in the 95th quantile. This indicates that SES had a larger impact on lower achieving schools, meaning that having more lower-SES students had an added negative impact for already lower achieving schools.
At the student-level, when including SES into Model 2 the effect of funding remains nearly the same throughout the distribution, dipping slightly below 0 in the lower quantiles and slightly above 0 in the upper quantiles, but now halved in size from the effects seen in Model 1. For SES, a trend is observed with a higher-SES having a larger positive impact at the lower ends of achievement (0.35 SD’s) and gradually decreasing when moving upward in the achievement distribution (0.06 SD’s). This effect never crosses zero however, and a positive effect of higher-SES is seen throughout the entire distribution.
At the school-level, model 3, which now added an interaction term between funding and SES, again shows an upward trend for the impact of funding on achievement when moving upwards through the quantiles, switching from negative to positive only in the highest quantiles however. The impact of SES was nearly constant throughout the first 3 quartiles, but then jumped for the highest quantiles of achievement (see Table 3). This change in relation from model 2 is due to the addition of the interaction between funding and SES on achievement. When plotting this interaction (Figure 1 below), one can see that the gradually decreasing impact of SES when moving upwards towards the quantiles of achievement. However, it can now be seen that the magnitude of the decrease in SES’s impact is directly related to the level of funding a school receives. This interaction shows that as funding levels get higher per student, the gap between lower-SES and higher-SES schools increases, and does so at faster rates in lower achieving schools. This indicates that school SES may be moderating the impact that funding has on achievement at the school-level. Increases to funding at the school-level appear to be affecting schools in such a way that the impact of SES appears to rise as funding rises for lower achieving schools. As an example, holding all else constant, the gap between a school with 0% low-SES students in the 5th quantile of achievement and a school with 100% low-SES students in the 5th quantile of achievement is expected to rise from around 2.7 SD’s when schools receive $5,000 per student, to approximately 4.2 SD’s when funding is raised to $10,000 per student. For schools in the 50th quantile of achievement and above, the effect of SES is unimpacted by greater funding, and in the highest quantiles the impact of funding is seen to be positive regardless of SES.
Figure 1. Expected impact of SES on achievement, varied by achievement quantile and funding levels. Expected impact presented between schools at the extremes of SES, i.e., schools with 0% of students on free and reduced lunch versus schools with 100% of students on free and reduced lunch.
At the student-level for model 3, funding again remained relatively constant throughout the distribution, centering right around 0 with slightly more negative effects in the lower ends and slightly higher in the upper quantiles. For SES, the effects followed the same trend, but with an extreme drop in effect size beginning at the 25th quantile and having largest impact at the lowest quantiles of achievement, with an expected effect of 1.33 SD’s at the 10th quantile. A significant interaction was additionally found between funding and SES at the lowest quantiles. The direction of this interaction is negative, meaning that at this level additional funding decreases the expected difference between high and lower-SES students. These results together show that SES has a greater impact at the lowest levels of achievement, but that this impact can be offset by higher levels of funding for these students with every 1,000 dollars spent decreasing that gap by 0.05–0.12 SD’s. Given that a $10,000 per pupil spending range is seen in this dataset, this equates to roughly cutting that effect in half between the lowest and highest funded schools. As an example, holding all else constant the expected difference between high and low-SES students in the 5th and 10th quantiles is expected to decrease from 0.5 to 0.7 SD’s, respectively, to 0.2 and 0.1. SD’s, respectively, when funding is increased from $5,000 to $10,000 per student. Figure 2 illustrates this interaction by showing expected impacts of SES on achievement at given levels of spending representing over 90% of the entire funding range.
The goal of this paper was to answer two research questions related to how differences in analyses and models chosen can have significant impacts on results and in turn interpretations for future policies, through deeper examinations of the complex relations between funding, SES, and achievement. For the first research question looking at how the relation of both funding and SES with academic achievement may differ based on the quantile of achievement examined, both the school and student-level, use of quantile regression proved to be effective in providing deeper analysis of these relations. At both levels, a significant relation between funding and achievement was found, and the size of the effect positively increased when moving upwards in the achievement distribution. This indicates that estimates conducted at the mean for the relation between funding and achievement are missing out on key parts of the relation, and that the potential effect of funding may be higher than previously thought. That is, for schools and students that are highly achieving the relation between additional funding and achievement may be quite meaningful. Mean analyses would not uncover this trend, and rather would simply see a general negative relation between funding on achievement. Rather, this quantile analysis reveals that differential relations between funding and achievement exist, and that some groups have more positive relations between funding and achievement than others. Further research should be conducted to uncover why exactly this is, to help uncover what methods lead to the best use of funding, and what variables may be limiting the relation seen in schools with the lowest relation between funding and achievement. Quasi-experimental designs could replicate this type of research using a similar approach. In doing so, we would be able to better examine how funding impacts achievement dependent on how a school or student is performing. Alternatively, analyses conducted only in sub-populations (e.g., schools with low achievement) could aim to study these relations separately for these most at-risk school, but would sacrifice the statistical power that comes with using a quantile regression based technique. Regardless, these results point towards differing cross-sectional relations between academic achievement and funding, pointing for the need for quasi-experimental work to replicate this quantile specific work. This would help in identifying first if the trends seen here replicate in more causal research designs, but also would help identify the schools that are putting funding to the best use to produce gains in achievement.
For SES and achievement, the opposite relation was found, with this relation gradually decreasing as achievement levels got higher. Mean analyses here would lead to generalizations about the relation between SES and achievement that would not be accurate for all schools and students in the distribution, overestimating this relation for high achieving schools and students, and underestimating this relation for those in the low ends of the distributions. Interventions based on the mean analyses thus would treat SES the same in all these schools and students, leading to inefficient and inequitable allocation of resources and interventions by giving the same to schools and students who have different needs. Overall however, what is clear from these results is that they align with past work in showing that a gap in achievement on the basis of SES still exists, and further that said persisted throughout the distribution of achievement scores and both at the aggregated school- and individual student-levels.
Looking at research question 1 examining how different levels of achievement impact the observed relation between funding and achievement, the level of aggregation appeared to not play a significant role in the results. Rather agreement was found between the school and student level analyses, both showing positively increasing relations between funding and achievement when moving upwards through the distributions of achievement. This provides support for the idea that these relations may not be susceptible to aggregation bias, and instead provides two levels of support for the idea that funding differentially impacts achievement conditional on the level of achievement of the student or school in question. Further, this consistency between both levels was found for relations between funding and achievement as well as for relations between funding and SES. Tying this back into existing literature, the results up until this point appear to contradict the findings from Henry et al. (2010) finding that higher impacts of funding on achievement may exist for students and schools at the most risk for low achievement. Though this work is correlational and cannot speak to impacts, the weakened relations we observe in the lower ends of the distribution compared to the higher end suggest a potential weakened impact of funding on achievement at this point of the distribution. Despite this however, thus far this discussion has focused only on the direct relations between these variables, with no moderation or mediation effects taken into account as may be the case with these complexly related variables.
For the second research question on whether the relation between funding and achievement differs based on SES, results from the tested interactions between funding and SES showed that a complex relation exists between the two in both the school-level and student-level models. For the school-level model, SES moderates the effects of funding in such a way that higher levels of funding lead to larger gaps in achievement between higher and lower-SES schools. It cannot be determined whether or not this widening of the gap is due to higher-SES schools improving more quickly than lower-SES schools as funding increases, or if lower-SES schools are actually getting worse as funding increases. However, prior research on the Matthew effect (Stanovich, 1986; Schatschneider et al., 2016) would suggest that it is due to a fan-spread growth pattern with the higher SES schools growing faster than lower-SES schools as more funding is introduced. If this is the case, this points to the idea that these more at-risk schools with higher proportions of lower-SES students may require larger levels of funding compared to schools with lower proportions of these students. That is, higher-SES schools may be able to make more growth than lower-SES schools with the same increase to funding, and thus in order to encourage the same level of growth in high and low-SES schools, equitable funding would be that which differentially provides extra resources to low-SES schools in a way that growth is equal between high and low-SES schools. Results of this analysis would suggest that policy interventions give additional funding more to lower-SES schools, to ensure that growth is equal for all schools.
At the student-level, SES moderates the relation between funding and achievement in such a way that higher levels of funding were related to smaller gaps between high and low-SES students. That is, in schools with lower levels of funding, the achievement of high-SES students is considerably larger than low-SES students, but that for schools with larger levels of funding, the gap between the same high and low-SES students is expected to be considerably smaller. This is particularly true for students in the lower ends of the achievement distribution, but seems to disappear in the 50th quantile and above. Though quasi-experimental research designs help to provide a way to control for SES, directly measuring and including SES into the models has clear impacts on the observed results. Further, aiming to remove the effect of SES entirely does provide the clearest picture of understanding how funding relates to achievement in general, but removes an important part of the equation. Funding levels can be significantly influenced by many of the achievement covariates we are interested in, such as SES, and as such directly modelling these relations can provide policy makers with information on which schools and students will respond the best to increases in funding. Policy implications for this finding could suggest that additional funding would be most beneficial for these lowest achieving low-SES students, to decrease the gap most efficiently between high and low-SES students. Ignoring this information could lead to funding being equally increased rather than equitably increased. On the surface, equal funding for high- and low-SES students and high and low achieving students sounds like a positive thing. However, the results of this suggests that equitable funding would be that in which lower achieving and lower-SES students receive more funding than their higher achieving and higher-SES counterparts. These results at this level align with the findings of Henry et al. (2010) that funding may have greater impact for the most at-risk students. Here we find that the relations among funding and achievement were highest for these groups, and with the interaction included found that this finding of higher relations among the variables existed for risk based both on past performance and low-SES.
Comparing the school- and student-level results it appears that the level of aggregation chosen for analysis also has significant impacts on the findings, and in turn downstream policy recommendations. The results within align with the past work by Fertig and Wright (2005) suggesting potential of differing effects of funding at the school- versus student-levels.
For the second research question examining the extent to which SES moderates the relation between funding and achievement, the ecological fallacy appears to be at play when trying to generalize school-level results to student outcomes. At the school-level, higher levels of funding appear to exacerbate the gaps in achievement between low and high-SES students, whereas the opposite is found at the student-level. Student-level results indicate that higher levels of funding may be associated with decrease these gaps, however only when the funding is directed to the low achieving low-SES students who need it the most. A combination of analyses at the two different levels would provide the most comprehensive results and findings. This would indicate that funding could be an effective intervention for individual populations of students, and that in turn this could help lead to overall better gains in achievement when aggregated to the school-level. Simulation based work in this area by Grosskopf et al. (2017) directly explored if using a weighted-student-funding approach could be used in this way to increase the efficiency of spending and better target school funding to more rapidly improve student outcomes. Here, they found that such a method was effective in doing exactly this, and further additionally reduced inequities in achievement at a greater rate than the status quo funding. This also relates back to the aforementioned work by Tuchman et al. (2022) which found a weighted-student-funding approach was more effective at increasing achievement and decreasing gaps at the district-level than the status quo funding methods. Overall, the results of these studies combined with the current study point to the idea that funding impacts individual students differently, and that if we can understand these differences we can allocate funds accordingly and improve student outcomes at a faster rate.
The level of aggregation of analysis thus appears to play a significant role in the interpretation of results, and in turn downstream policy implementations and evaluations. It is important now to step back and ask the question again of what the goals are for the policies implemented, and whether the existing research and evaluations reflect those goals. If goals are simply related to aggregate school-level results, then aggregate analyses are appropriate. If the goal is to improve the achievement of as many individual students as possible, with particular interest to at-risk students, then school-level analysis is not appropriate and appears not to generalize to this individual level. Rather, student-level analysis would be more accurate for evaluating policies. Further, student-level policies would likely be more aligned with achieving these goals. The level of aggregation of analyses does not have to be all-or-nothing however, but rather different information is gained from each analysis. Combining the two levels of analysis in this study could lead to interpretations that are more holistic than each on its own. For example, school-level analysis suggests that equitable funding is necessary to lead to equal growth between high and low-SES schools, and student-level analysis provides a path for directing said increased funds in such a way as to maximize growth. Quasi-experimental research designs should be utilized to examine the more causal link between funding and achievement at the varied levels of aggregation. Using those designs, researchers can observe not only the direct impact of school expenditures on individual students, but additionally can analyze how individual differences in student and school characteristics impact responsiveness to school expenditures. Examining research at this level can help to identify the best path for directing funding, beyond the school-level. Whereas school-level analysis can help identify trends in which schools will benefit most from increased funding, student-level analysis takes into consideration that schools are comprised of individual students, all with the potential to differentially respond to increases in funding. The combination of the two would suggest a student-centered approach to funding, giving the most funding to low-SES and low achieving students, and in turn giving more funding to the schools with the greatest proportion of these students. This would both satisfy equitable school-level funding, while also ensuring the funds were directed to the individual students who need and would benefit from them the most. Combining the results of both research questions, keeping level of aggregation in mind, we suggest that funding should be directed so that the most at-risk students receive the most funding towards their education, and in turn the schools with the highest proportions of these students would receive the largest levels of funding. This would mean that lower achieving and lower-SES students receive additional funding, leading to the maximal student growth that in turn leads to schools serving the highest proportions of these students receiving more funds and directing these funds in a more efficient way leading to both school- and student-level growth.
A limitation to this study is that it was only able to examine one of the complex relations that funding and achievement share with many student risk variables. However, if further research was done examining similar relations between funding and other at-risk variables such as by race, English language proficiency, region, and others throughout achievement distributions, specific areas for effective intervention through funding could be found. The sum of these studies could help guide funding towards an empirically based individual student-need based system, with significant potential to decrease various gaps in achievement. Additionally, although it was not the aim of the present study, more in depth analyses exploring the characteristics of the current sample, or of samples from future studies conducting similar analyses, could be effective in identifying what aspects outside of those already assessed are potentially limiting the observed relations within said schools. Another limitation of the current study is the low reliability and lack of validity reported for the FAIR reading measure in grades 1–2. Given the low reliability and lack of validity, we must use caution when interpreting the results. We also want to use this opportunity to emphasize the importance of using reliable measures both in research and when evaluating data for policymaking decisions. An additional and obvious limitation of the study lies in the inability to directly measure student funding/expenditures at the student-level, and instead having to rely on school-level data for these analyses. We also note this as a limitation and issue not only for our current study, but for the field, leading to virtually no literature on how funding relates to individual students. From the results, it is clear that funding relates differently to different individual students, and that funding relates differently to achievement at the student-level as it does at the school-level. As such, improving measurement and reporting of how funding is allocated in greater detail than at the average school-level would greatly improve future research on how funding impacts school achievement, as well as the best path forward in using funding as a mechanism to improve student performance. In the meantime, applying school-level funding data to student-level data in the way presented here can still help to build up a body of evidence on the importance of such measurement and future studies, and can provide meaningful literature to which the current results could be compared.
Overall, results show that simply abandoning funding as a potential intervention for students and schools would be unwise, but further that funding based interventions could be directed on individual needs in order to be most effective. More than anything, these results show that more rigorous models are needed for understanding the complex relations at play between variables such as those presented here. An additional limitation, and a point that we aimed not to overstep on, is that these data and analyses are purely cross-sectional and cannot be used to try any causal inferences. Rather, we aimed through this paper to demonstrate how the inclusion or exclusion of variables, as well as the research design and analytic choices used, can lead to drastically different results even when using an identical sample of data. We additionally aimed to have this cross-sectional research uncover trends and findings related to funding, achievement levels, and SES that may be interesting to researchers in the field, and that can be used to help develop models and future studies that are able to leverage more rigorous quasi-experimental designs. The move towards quasi-experimental designs allowing for more causal relations to be identified has been of the utmost importance in the field, but research methods still should be expanded within these approaches to better capture the complex relations. Further, this paper shows that the type of analyses and level of aggregation chosen have significant impacts on the results, and in turn interpretations of results, and as such aligning the goals of a policy with the type of analysis used is incredibly important and often overlooked.
The project data and code are publicly available via the LDBase repository. This data can be found at: https://ldbase.org/projects/80e05827-295d-4252-b05d-884fc39aaff1.
Ethical review and approval was not required for the study on human participants in accordance with the local legislation and institutional requirements. Written informed consent from the participants’ legal guardian/next of kin was not required to participate in this study in accordance with the national legislation and the institutional requirements.
JS and SH wrote the manuscript. JS conducted the data analysis. SH supervised the research and led project conceptualization. All authors contributed to the article and approved the submitted version.
This paper was supported by two grants through the Eunice Kennedy Shriver National Institute of Child Health and Development (HD052120 and HD095193). Funding provided general research support.
The authors declare that the research was conducted in the absence of any commercial or financial relationships that could be construed as a potential conflict of interest.
All claims expressed in this article are solely those of the authors and do not necessarily represent those of their affiliated organizations, or those of the publisher, the editors and the reviewers. Any product that may be evaluated in this article, or claim that may be made by its manufacturer, is not guaranteed or endorsed by the publisher.
Abott, C., Kogan, V., Lavertu, S., and Peskowitz, Z. (2020). School district operational spending and student outcomes; evidence from tax elections in seven states. J. Public Econ. 183, 104142–104124. doi: 10.1016/j.jpubeco.2020.104142
Augenblick, J. G., Myers, J. L., and Anderson, A. B. (1997). Equity and adequacy in school funding. The Future of Children 7, 63–78.
Baker, B. (2013). Review of “weighted student funding for California.” Boulder, CO: National Education Policy Center.
Borman, G. D., Hewes, G. M., Overman, L. T., and Brown, S. (2003). Comprehensive school reform and achievement: a meta-analysis. Rev. Educ. Res. 73, 125–230. doi: 10.3102/00346543073002125
Coleman, J. S., Campbell, E., Hobson, C., McPartland, J., Mood, A., and Weinfeld, F. et al. (1966). Equality of Educational Opportunity, Washington, DC: Government Printing Office.
Costanzo, A., and Desimoni, M. (2017). Beyond the mean estimate: a quantile regression analysis of inequalities in educational outcomes using INVALSI survey data. Large Assess Educ. 5:14. doi: 10.1186/s40536-017-0048-4
Eide, E., and Showalter, M. (1998). The effect of school quality on student performance: a quantile regression approach. Econ. Lett. 58, 345–350. doi: 10.1016/S0165-1765(97)00286-3
Fertig, M., and Wright, R. (2005). School quality, educational attainment and aggregation bias. Econ. Lett. 88, 109–114. doi: 10.1016/j.econlet.2004.12.028
Florida Center for Reading Research. (2009). Florida Assessments for Instruction in Reading: Technical Manual 2009–2010 Edition Kindergarten- Grade 2. Tallahassee: State of Florida, Department of Education.
Galindo, C., and Sonnenschein, S. (2015). Decreasing the SES math achievement gap: initial math proficiency and home learning environments. Contemp. Educ. Psychol. 43, 25–38. doi: 10.1016/j.cedpsych.2015.08.003
Geraci, M., and Bottai, M. (2014). Linear quantile mixed models. Stat. Comput. 24, 461–479. doi: 10.1007/s11222-013-9381-9
Greenwood, P. H., and Luloff, A. E. (1979). Inadvertent social theory: Aggregation and its effects on community research. Journal of the Northeastern Agricultural Economics Council. 8, 44–47.
Grosskopf, S., Hayes, K., Taylor, L., and Weber, W. L. (2017). Would weighted-student funding enhance intra-district equity in Texas? A simulation using DEA. J. Oper. Res. Soc. 68, 377–389. doi: 10.1057/jors.2015.93
Hanushek, E. (1989). The impact of differential expenditures on school performance. Educ. Res. 18, 45–62. doi: 10.3102/0013189X018004045
Hanushek, E. A. (1997). Assessing the effects of school resources on student performance: an update. Educ. Eval. Policy Anal. 19, 141–164. doi: 10.3102/01623737019002141
Hedges, L. V., Laine, R. D., and Greenwald, R. (1994). An exchange: part I: does money matter? A meta-analysis of studies of the effects of differential school inputs on student outcomes. Educ. Res. 23, 5–14. doi: 10.3102/0013189X023003005
Henry, G. T., Fortner, C. K., and Thompson, C. L. (2010). Targeted funding for educationally disadvantaged students: a regression discontinuity estimate of the impact on high school student achievement. Educ. Eval. Policy Anal. 32, 183–204. doi: 10.3102/0162373710370620
Jackson, C. K. (2020). “Does school spending matter? The new literature on an old question,” in APA Bronfenbrenner Series on the Ecology of Human Development. Confronting Inequality: How Policies and Practices Shape Children's Opportunities. eds. L. Tach, R. Dunifon, and D. L. Miller (Washington: American Psychological Association).
Jackson, C. K., Johnson, R. C., and Persico, C. (2016). The effects of school spending on educational and economic outcomes: evidence from school finance reforms. Q. J. Econ. 131, 157–218. doi: 10.1093/qje/qjv036
Jarmalowski, H., Alderman, C., and Roza, M. (2022). Do districts using weighted student funding formulas deliver more dollars to low-income students? Peabody J. Educ. 97, 427–438. doi: 10.1080/0161956X.2022.2109912
Jencks, C. (1972). Inequality: A Reassessment of the Effect of Family and Schooling in America. New York: Basic Books.
Jeynes, W. H. (2015). A meta-analysis on the factors that best reduce the achievement gap. Educ. Urban Soc. 47, 523–554. doi: 10.1177/0013124514529155
Koenker, R., and Bassett, G. (1978). Regression quantiles. Econometrica 46, 33–50. doi: 10.2307/1913643
Lacour, M., and Tissington, L. (2011). The effects of poverty on academic achievement. Educ. Res. Rev. 6, 522–527.
Lee, V. E., and Burkam, D. T. (2002). Inequality at the starting gate: Social background differences in achievement as children begin school. Economic Policy Institute.
Lockwood, R. E., and McLean, J. E. (1997). Twenty-five years of data on educational funding and student achievement: what does it mean? Educ. Res. Q. 2, 3–12.
Moorman, J. (1979). Aggregation bias: an empirical demonstration. Sociol. Methods Res. 8, 69–94. doi: 10.1177/004912417900800104
Perera, L. D. H. (2014). Parents’ attitudes towards science and their children’s science achievement. Int. J. Sci. Educ. 36, 3021–3041. doi: 10.1080/09500693.2014.949900
Petscher, Y., and Logan, J. A. R. (2014). Quantile regression in the study of developmental sciences. Child Development 85, 861–881.
Reardon, S. F. (2011). “The widening academic achievement gap between the rich and the poor: new evidence and possible explanations,” in Whither opportunity? Rising inequality and the uncertain life chances of low-income children. eds. R. Murnane and G. Duncan (New York: Russell Sage Foundation Press).
Robinson, W. (1950). Ecological correlations and the behavior of individuals. Am. Sociol. Rev. 15, 351–357. doi: 10.2307/2087176
Schatschneider, C., Wagner, R. K., Hart, S. A., and Tighe, E. L. (2016). Using simulations to investigate the longitudinal stability of alternative schemes for classifying and identifying children with reading disabilities. Sci. Stud. Read. 20, 34–48. doi: 10.1080/10888438.2015.1107072
Sebold, F. D., and Dato, W. (1981). School funding and student achievement: an empirical analysis. Public Finance Q. 9, 91–105. doi: 10.1177/109114218100900108
Shero, J., and Hart, S. A. (2020). The intersection between school efficiency and student individual differences. PsyArXiv: w7a8j [preprint].
Sirin, S. (2005). Socioeconomic status and academic achievement: a meta-analytic review of research. Rev. Educ. Res. 75, 417–453. doi: 10.3102/00346543075003417
Stanovich, K. E. (1986). Matthew effects in reading: some consequences of individual differences in the acquisition of literacy. Read. Res. Q. 22, 360–407.
Tuchman, S., Gross, B., and Chu, L. (2022). Weighted student funding and outcomes: implementation in 18 school districts. Peabody J. Educ. 97, 479–496. doi: 10.1080/0161956X.2022.2109917
Urban Institute. (2017). School funding: do poor kids get their fair share? Available at: http://apps.urban.org/features/school-funding-do-poor-kids-get-fair-share/ (Accessed October 20, 2022).
Keywords: reading, school funding, socioeconomic, SES, methodologies
Citation: Shero JA and Hart SA (2022) Methodological decisions and their impacts on the perceived relations between school funding and educational achievement. Front. Educ. 7:1043471. doi: 10.3389/feduc.2022.1043471
Received: 23 September 2022; Accepted: 20 October 2022;
Published: 18 November 2022.
Edited by:
Aldo Bazán-Ramírez, National University Federico Villareal, PeruReviewed by:
Maria Jesus Perez Curiel, University of Valladolid, SpainCopyright © 2022 Shero and Hart. This is an open-access article distributed under the terms of the Creative Commons Attribution License (CC BY). The use, distribution or reproduction in other forums is permitted, provided the original author(s) and the copyright owner(s) are credited and that the original publication in this journal is cited, in accordance with accepted academic practice. No use, distribution or reproduction is permitted which does not comply with these terms.
*Correspondence: Jeffrey A. Shero, amVmZnJleXNoZXJvQGdtYWlsLmNvbQ==
Disclaimer: All claims expressed in this article are solely those of the authors and do not necessarily represent those of their affiliated organizations, or those of the publisher, the editors and the reviewers. Any product that may be evaluated in this article or claim that may be made by its manufacturer is not guaranteed or endorsed by the publisher.
Research integrity at Frontiers
Learn more about the work of our research integrity team to safeguard the quality of each article we publish.