- Faculty of Educational Sciences and Law, Universidad del Pacífico, Guayaquil, Ecuador
Measurement of self-regulated learning through self-report questionnaires can help improve educational efforts. The Deep Learning Strategies Questionnaire has been recently validated, which consists of 30 items and four scales: basic self-regulated learning strategies, visual elaboration and summary strategies, deep information processing strategies, and self-regulated social learning strategies. We examined the characteristics of the questionnaire with 694 Ecuadorian students. The exploratory factor analysis resulted in four factors, like the original model. However, the factors identified as basic and social learning strategies included items of visual elaboration and summary and deep processing strategies. Further group comparisons showed that participants with high school finished used fewer visual and verbal elaboration strategies than those with higher education levels and that males use more deep information processing strategies than females. We discuss the difficulty of separating self-regulated learning strategies and conclude with suggestions for future research and recommendations for educational practice.
Introduction
One of the crucial aspects of school learning and lifelong learning is self-regulation, which means that students who use appropriate self-regulation strategies may learn more (Núñez Perez et al., 2006; Badali et al., 2022; Biwer et al., 2022; Jansen et al., 2022; Shin and Song, 2022). A basic premise of self-regulated learning is that there are regulatory processes (i.e., monitoring and control) that are performed on aspects associated with school learning, and can be understood, fostered, and even trained for being transferred to other learning tasks (Bell and Kozlowski, 2008; Usher and Schunk, 2018). The effort to understand self-regulative learning and its effect on academic performance has given rise to various evaluation methods that derive from theoretical models. One of these is the self-report questionnaire, which assumes that the learners can assess self-regulatory learning strategies separately and can be identified (Wolters and Won, 2017). The results of these questionnaires can be associated with the learning outcomes to estimate the advantages and limitations of a learning condition and student characteristics.
Measuring self-regulative learning strategies is still challenging for researchers (Veenman, 2007; Fan et al., 2022). One way to assess self-regulation may be to identify students’ strategies in realistic situations. Panadero et al. (2021) have developed the Deep Learning Strategies Questionnaire to recognize university students’ strategies during learning. The items are grouped into four factors representing different self-regulation strategies that mediate the effect of other factors (e.g., performance or self-efficacy). Although the questionnaire attained a satisfactory level of fitting, its application in other populations requires further examination. This exploratory study aimed to contribute to measuring self-regulation learning strategies by examining the validity and reliability of the Deep Learning Strategies Questionnaire in a population of university students from Ecuador. This article introduces the self-regulated learning strategies and the Panadero et al. (2021) questionnaire and finishes with a study of psychometric characteristics.
Self-regulated learning strategies
Self-regulated learning is a growing research interest that has been conceived from a variety of models (Montalvo and Torres, 2004; Panadero, 2017; Schunk and Greene, 2018). Most of them conceive self-regulated learning as recursive phases, processes, and strategies. For example, Zimmerman (2002) and Zimmerman and Moylan (2009) understand self-regulation in education from a sociocognitive perspective (Bandura, 1986) with three cyclical phases. The forethought phase concerns the processes and beliefs that happen before efforts to learn. The performance phase refers to the processes that occur during the implementation, and the self-reflection phase denotes the judgments and reactions that occur after learning effort (Zimmerman, 2002). For example, a group of three students (i.e., learning condition) formulate the goal (i.e., planning phase) of solving ten word problems of finding the perimeter of a circle (i.e., domain-specific knowledge). During solving each task (i.e., performing phase), a member asks to avoid non-task comments (i.e., group control process), and another member draws a circle with the measures (i.e., generating activity and load reduction). At the same time, another checks the results of manual calculations with the results of the cellphone (i.e., cognitive monitoring process). After finishing the learning session (i.e., self-reflection phase), the group solved four of ten problems during the time allotted. A member attributed this result to misunderstanding the difference between radius and diameter (i.e., causal attribution), and another member asked to set up more achievable goals for the next learning session (i.e., planning phase).
Self-regulated learning involves strategies students proactively perform for themselves (Greene, 2018). Self-regulated learners carry out mental processes of monitoring and controlling their prior knowledge, goals, strategies, motivations, affects, behaviors, and external environment during learning (Usher and Schunk, 2018). From this perspective, the student is assumed to be a proactive agent capable of supervising and controlling the learning processes (Zimmerman, 2002).
Examining self-regulated learning strategies is challenging because learning processes include cognitive-generic and knowledge-specific skills that are often difficult to separate (Dumas, 2020). Self-regulated learning strategies may encompass planning, monitoring progress, evoking prior knowledge, help-seeking, integrating sources of information, creating visual or verbal representations, predicting future performance, and so forth (Zimmerman and Pons, 2016; Fong et al., 2021). In general, these skills are domain-general, impose low mental load (if any), and might be flexibly applied to a variety of tasks (e.g., math and biology) already known (Tricot and Sweller, 2014). However, applying these strategies may be very demanding for novice students, especially when the tasks are complex, the strategy requires other specific knowledge (e.g., learning software to create maps or organize domain concepts), there is little time for study, or it is not clear which strategy is more effective for the task (e.g., narrated or written self-explanations for learning The independence of Latin America) (Van Gog et al., 2011; Baars et al., 2017; Dong et al., 2020). Consequently, the study of self-regulated learning strategies and their effectiveness may not be generalized to all learning conditions and groups of students (Hirt et al., 2021).
Basic self-regulated learning strategies
The basic strategies of self-regulation of learning are made up of phases of a cyclical nature. For example, the Zimmerman and Moylan (2009) model suggests the preparation phases, made up of the analysis and planning of the task; execution, when the task is performed, and progress control or monitoring is carried out; and assessment, where the student evaluates the achievements or results of it. In each of these processes, generic sub-processes are activated, such as metacognitive monitoring and control (Nelson and Narens, 1990), which may be difficult for the student to self-perceive unless they are instructed to make them explicit when the information from the learning materials is complex (Sweller and Paas, 2017; Mirza et al., 2019).
Visual elaboration strategies and summaries
Visual elaboration and summary strategies (i.e., generative strategies) identify how a student processes, understands, and stores information in her memory (García-Pérez et al., 2021). Generative elaborations can be used to learn self-regulation strategies during autonomous tasks (Fiorella and Mayer, 2015; Pilegard and Fiorella, 2016). These elaborations involve the conscious selection of information elements that help organize a temporary mental model that can be integrated into long-term memory (Mayer, 2012; Dunlosky et al., 2013). In addition, these activities could be more used by students with high self-regulation skills (Lim et al., 2009).
Deep information processing strategies
From a cognitive perspective, deep information processing strategies involve understanding and storing information in long-term memory (Ausubel, 1960; Mayer, 2012). These involve metacognitive processes associated with a network of preexisting elements or schemes (Bartsch and Oberauer, 2021). Metacognitive monitoring involves assessing ongoing progress or the current state of a particular learning activity (Son and Schwartz, 2002; Schraw, 2008), while metacognitive monitoring involves stopping during the task and making a decision to continue or change the strategy to learn better (Dunlosky and Metcalfe, 2009). The literature presents an important number of strategies that promote long-term learning, such as retrieval and interleaving practice (Dunlosky et al., 2013; Fiorella and Mayer, 2015; Zambrano and Yaguarema, 2021; Carpenter et al., 2022; Ekuni et al., 2022). These help monitor actions of significant association of prior knowledge with new information. In turn, the new schemes acquired help to more efficiently monitor learning new information (Witherby and Carpenter, 2021).
Social strategies for the regulation of learning
Social learning regulation strategies greatly influence task performance (Montalvo and Torres, 2004; Panadero and Järvelä, 2015). It is evidenced in the interactions that take place in classrooms or individual and group computer-supported activities (Lee et al., 2015). In this context, self-regulation of learning is a fact when individual, pair, or group feedback techniques are used. Social regulation can occur between teacher and student, student and student, and student-group (Allal, 2020).
Research on social self-regulation situations suggests that students can regulate themselves in a classroom or in small groups (Järvelä, 2015). This regulation can be at the individual level (i.e., self-regulation) when a student regulates himself during group work. It can be co-regulation when two students regulate each other during a task of mutual responsibility or socially shared regulation when different members of a group act as learning regulators of a larger group (Panadero and Järvelä, 2015; Hadwin et al., 2017).
Self-report of self-regulated learning strategies
There are questionnaires previously developed to that of Panadero et al. (2021) that categorize self-regulated learning strategies. For example, the Learning and Study Strategies Inventory (LASSI) comprises the subscales of information processing, selecting main ideas, self-testing, and so forth (Fong et al., 2021). Similarly, Zimmerman and Pons (2016) suggest other strategies such as self-evaluation, goal-setting and planning, seeking, and social assistance. However, measuring these constructs is complex (Rubio and García, 2018). The self-report method through questionnaires is more feasible to apply due to its ease of administration in large samples, interpretation, and scoring (Núñez Perez et al., 2006; Roth et al., 2015). The term self-report applies to any assessment in which people answer items to elicit information about the respondents’ attitudes, beliefs, perceptions, behaviors, skills, or knowledge (Wolters and Won, 2017). This broad category includes methods as varied as verbal interviews, surveys, questionnaires, diaries, think-aloud, and stimulated recall.
Self-report methods vary with respect to the type of item used to obtain a response and the structure imposed on the information solicited from participants (Wolters and Won, 2017). Response formats may be more open, allowing more flexibility in the nature and amount of information students can provide, or they may require selection from a short list of alternatives. Some self-report methods, for example, rely on more elaborate or authentic prompts (e.g., stimulated recall) that ground student responses in a specific context, while other prompts may consist of a single word or phrase (Roth et al., 2015; Wolters and Won, 2017).
This form of measurement is considered a first wave because it characterizes self-regulation as a static phenomenon (Panadero et al., 2015). Furthermore, these instruments can be retrospective, prospective, or a combination of both. One problem with these measurements may be internal and external validity because students may interpret the items without considering the mental processes that really happened (Karabenick et al., 2007) or are specific contexts or cultures that mediate the interpretation of the items (Hadwin et al., 2001; Huang and Prochner, 2003; Shi et al., 2013). Accordingly, self-regulated learning questionnaires should be examined before being administered to a different student population.
While there may instruments that measure self-regulated learning (see a review by Roth et al., 2015), we are specifically interested in self-regulated learning strategies. The LASSI (Weinstein et al., 1988; Fong et al., 2021) has a similar name, but it measures constructs the student may not be able to recognize in a learning session (e.g., information processing, attitude, etc.). Unlike the LASSI, the Panadero questionnaire asks for factors the student can recognize in realistic situations, such as preparing for doing the task and monitoring understanding (i.e., basic processes), drawing visualization or summaries, ask to others about the task, or connecting new information to what the one knows.
Deep strategies questionnaire
Panadero et al. (2021) developed the Deep Strategies Questionnaire with four factors and 30 items1. These factors were: basic learning self-regulation strategies, visual elaboration and summary strategies, deep information processing strategies, and social learning self-regulation strategies. Several confirmatory factor analyzes were performed in the original study to examine validity and reliability. Panadero et al. (2021) used a baseline to see how each of the factors is related to each other. They also used a confirmatory analysis using two sub-samples. The single-factor model resulted in significant estimates. However, the indices were not satisfactory (χ2/df = 5.44, RMSEA = 0.121, TLI = 0.71, CFI = 0.73), so the model was rejected, and the cross-validation analysis was not performed. They tested a correlated factor model (χ2/df = 2.42, RMSEA = 0.069, TLI = 0.91, CFI = 0.92) and a hierarchical model (χ2/df = 2.36, RMSEA = 0.067, TLI = 0.91, CFI = 0.92) with the first subsample, and found that all the estimated weights were significant, and the indices showed a good fit. In the hierarchical model, the four first-order factors (i.e., learning strategies) were considered indicators of the second-order construct named deep learning strategies (Panadero et al., 2021). Path-analysis and cross-validation with the second subsample were performed to evaluate the external validity. The cross-validation revealed good fit indexes, although it was concluded that the hierarchical had a better fit because of its lower ratio (χ2/df = 2.16, RMSEA = 0.062, TLI = 0.92, CFI = 0.91) than the correlated model (χ2/df = 2.20, RMSEA = 0.063, TLI = 0.91, CFI = 0.91). However, while the fit indices are very similar, it is not clear why the hierarchical model is better considering that a ratio ≤2 indicates a superior fit (Alavi et al., 2020). The non-significant negative relationship between deep strategies and the average self-estimated grade (−0.18) stands out among the results. The authors did not hypothesize the relationship between self-estimated academic performance with deep strategies. However, they managed to determine that these mediate the effects of other variables (e.g., orientation toward learning, self-efficacy, regulation style, avoidance, and effort).
Present study
Panadero et al. (2021) study revealed that the questionnaire helps identify four self-regulated strategies that students carry out during university learning. However, more studies need to be done to explore validity and reliability in different student populations. Consequently, this work aimed to examine the validity and reliability of the Deep Learning Strategies Questionnaire with Ecuadorian university students. Complementary, it explored potential differences between educational levels and gender.
Materials and methods
Participants
The study was authorized by the postgraduate committee of Universidad Del Pacífico. Participants were 718 Ecuadorian online undergraduate and graduate students, representing 95% of the total number of students enrolled in a faculty of education and law. Twenty-four cases were removed for not having completed the questionnaire correctly. Of these students, 481 were female (69.30%) and 213 male (30.70%), with a mean age of 34.04 (SD = 10.09). Concerning previous educational levels, 164 had finished high school (23.63%), 228 were technical degrees (32.85%), 216 had bachelor’s degrees (31.12%), and 86 had master’s degrees (12.39%).
Instruments and procedure
The Spanish version of the Deep Learning Strategies Questionnaire (Panadero et al., 2021) was used. It contains 30 items that are answered on a five-point Likert-type scale (1 = totally disagree; 5 = totally agree). The instrument has four factors: basic learning self-regulation strategies (eight items), visual and summary elaboration strategies (eight items), deep information processing strategies (eight items), and social elaboration study strategies (six items). The variable of gender and educational level was included. The latter was coded with 1 = high school, 2 = technical; 3 = bachelor, 4 = master.
Data collection was done through an electronic survey. Participants were contacted through the electronic channels of the university and during their online classes. They received a Google form via email that included the purpose of the study, that they should spend 10 min filling out the form, and that their participation was voluntary. The questionnaire was configured to be answered only once.
Results
A replication (i.e., confirmation) analysis of the models with structural equation modeling was performed. The following cutoff levels were considered: RMSEA < 0.06 to 0.08, CFI and TLI ≥ 0.95 (Schreiber et al., 2010). If models did not fit well, exploratory factor analysis was carried out to explore the psychometric structure of the questionnaire with our sample. Fit indexes of the three models developed by Panadero et al. (2021) were examined using the IBM AMOS package: single factor, correlated factors, and hierarchical. The data from the analysis with structural equations suggest that the models (Table 1) do not fit our sample well.
Since the models did not fit the data, exploratory factor analysis was conducted to explore if the items’ differential factorial loading explains the model’s lack of fit. Descriptive statistics indicate (Table 2) that values are concentrated on the right side of the distribution. The Kaiser–Meyer–Olkin measure of sampling adequacy was 0.940, and the Bartlett sphericity test was 9,076.12, df = 435, p < 0.001.
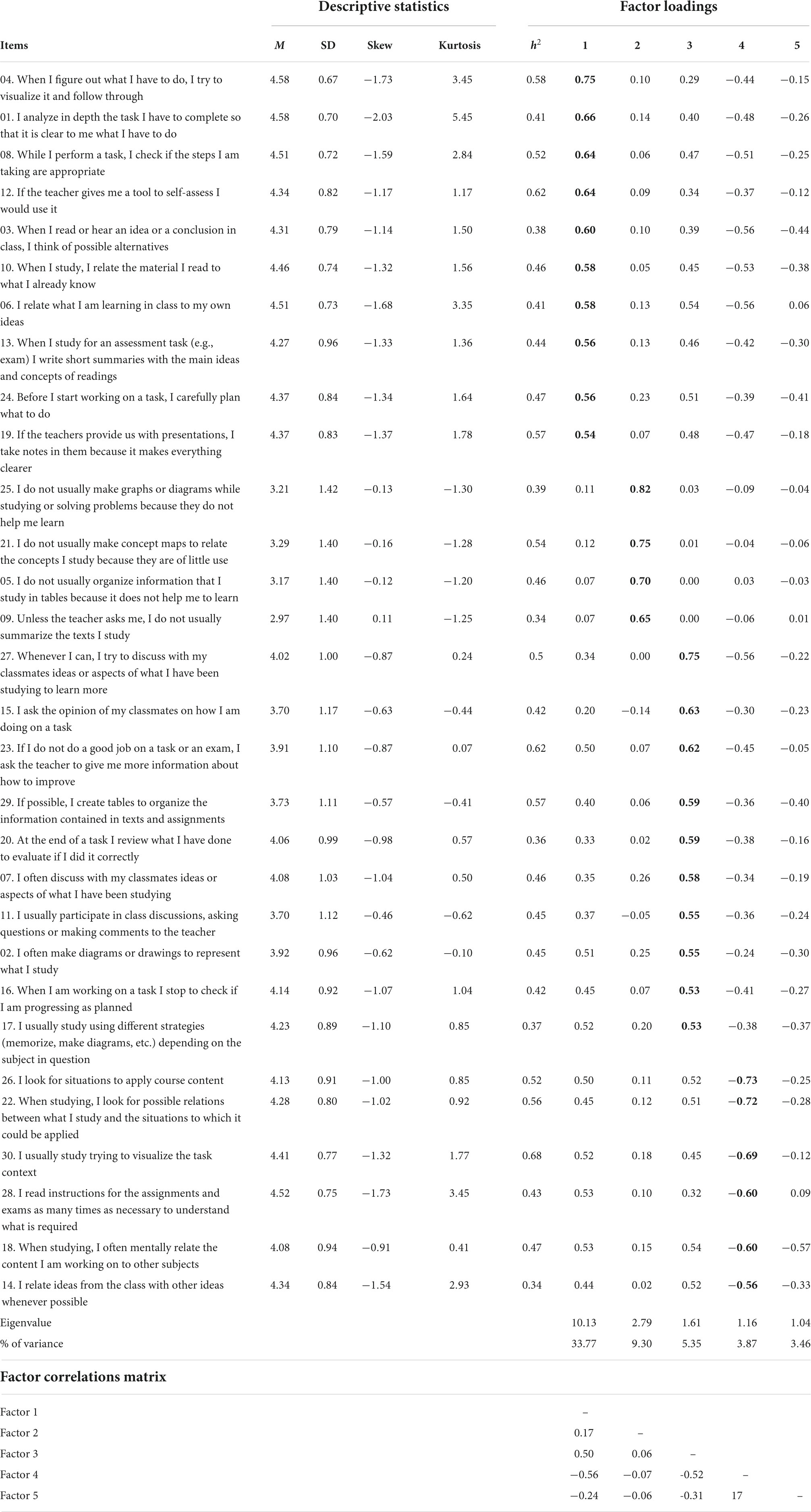
Table 2. Descriptive statistics of the items and structure matrix from unweighted least squares factor analysis: Communalities, eigenvalues, and percentages of variance.
Due to normal distribution may be improbable, unweighted least squares extraction method was used (Lloret-Segura et al., 2014). As the factors are theoretically related, an oblique rotation method (i.e., oblimin; Lackey and Sullivan, 2003) was conducted (Table 2). Five factors were found that explained 55.74% of the variance. The fifth factor can be discarded because it only had one item (Watkins, 2018), so the internal structure is assumed to be composed of four factors. A parallel analysis (Horn, 1965) was performed with the same dataset characteristics and suggested that only three factors (i.e., eigenvalues are bigger than randomly generated factors) should be retained (Watkins, 2018). These factors seem to correspond with basic learning strategies, visual and summary elaboration strategies, and social self-regulated learning strategies. Furthermore, all items (Table 2) met the practical significance minimal level (i.e., ±0.50 or greater) for interpretation of structure (Hair et al., 2019).
Although the questionnaire measured four factors, such as the original (Panadero et al., 2021), no consistency was observed in the items loading for each factor (Table 3). Sixty percent of the items (i.e., 18 of 30) were loaded in the original factors.
The internal consistency of the resulting scales (Table 4). Cronbach’s Alpha for all scales were good (i.e., >0.7; Kline, 1999). Kolmogorov–Smirnov tests indicate that the scales are not normally distributed: basic learning self-regulation strategies, KS = 14, p < 0.01; visual elaboration and summary strategies, KS = 0.07, p < 0.01; social strategies for self-regulation of learning, KS = 0.06, p < 0.01; deep information processing strategies, KS = 0.13, p < 0.01. Spearman’s correlation analyses between factors found that all factors are positively related. However, social strategies and visual and summary strategies are not significantly associated (Table 4).
Kruskal–Wallis tests were performed to explore if the factors for previous educational levels differ. The independent variable educational level was operationalized with high school, technologists, graduates, and masters. Statistically significant differences were found only for the visual elaboration and summaries strategies (Table 5). Pairwise Bonferroni comparisons indicated that those with a high school education level (average rank = 249.94) had a statistically significantly lower score than technologists (average rank = 384.01, p < 0.01), graduates (average rank = 372.64, p < 0.01), and masters (average rank = 373.62, p < 0.01). No differences were found between other educational level groups.
Finally, Mann–Whitney U tests were conducted to explore the difference between female and male students concerning each factor (Table 6). The tests revealed differences only in deep information processing strategies, favoring males.
Discussion
This study aimed to examine the validity and reliability of the Deep Learning Strategies Questionnaire (Panadero et al., 2021) with Ecuadorian university students and to explore potential differences in education level and gender. Our results suggest that the questionnaire measures four factors: basic learning self-regulation, visual elaboration and summary, social self-regulation, and deep information processing strategies. However, the parallel analysis indicated that the last factor (i.e., deep information processing strategy) may be removed. In addition, all the scales have a positive relationship, excepting social regulation and basic strategies, and an acceptable level of internal consistency (i.e., reliability).
An exploration of the items reveals a potential problem concerning construct validity in three of the four factors. Items 1, 4, 8, 12, and 24 variables were labeled as basic self-regulation strategies. Basic strategies refer to the cyclical phases of self-regulated learning, such as planning, execution, and evaluation (Zimmerman and Moylan, 2009). These items also include visualizations of the actions to be carried out, that is, in-depth analyses of the tasks and the verification of the steps taken, as well as using evaluation tools of the given actions. The excluded items have planning, performance, and evaluation content, such as checking the progress of the task, rehearsing for comprehension, planning the task, and previous reading of the instructions. These results are consistent with previous studies and theoretical elaborations (Schunk, 1986; Zimmerman, 2002; Carpenter et al., 2020) that indicate that visualization of the steps to be taken and monitoring are central elements when basic self-regulation strategies are executed. However, this factor involves measures that may also be interpreted as deep information processing or elaboration strategies, such as I relate what I am learning in class to my own ideas (i.e., 6) or when I study for an assessment task (e.g., exam) I write short summaries with the main ideas and concepts of readings (i.e., 13).
The second factor, seemingly referring to visual and summary elaboration strategies, also has evidence that suggests that self-regulated learning could be more productive when generative strategies are used (Fiorella and Mayer, 2015; Pilegard and Fiorella, 2016). In this factor, all loaded items (5, 9, 21, and 25) were intrinsically related to visual and verbal elaborations such as making graphs or diagrams, concept maps, charts, tables, and summaries. Two of the excluded items refer to visual and verbal elaborations, such as summaries (i.e., 13) and concept maps (i.e., 2), and the remaining refers to the use of different strategies depending on the subject, such as memorizing or drawing diagrams.
The third factor seems to refer to the social strategies of self-regulation of learning. The items (i.e., 7, 11, 15, 19, 23, and 27) refer to interactions with students and teachers associated with learning. These measures may refer to the social conditions of self-regulated learning, where students can ask for support (external regulation), regulate themselves, regulate others, and regulate themselves as a group during tasks (Järvelä, 2015; Panadero and Järvelä, 2015; Hadwin et al., 2017) and integrate multiple resources and generative processing strategies (Näykki and Järvelä, 2008). The excluded item (i.e., 19) refers to taking notes from professors’ presentations. Other measures also loaded in this factor, which include elaboration of diagrams or drawings (i.e., 2), task/goal monitoring (i.e., 16), construction of idea organization tables (i.e., 29), and rehearsing to understand (i.e., 20).
The fourth factor seems to coincide with the deep information processing strategies. The items involve applying relationships between the study material and the situations (i.e., 18, 22, and 26) and imagining or visualizing the situations to which the material refers (item 30). The excluded items (i.e., 3, 6, 10, and 14) also refer to cognitive processes of associating the information being learned with the structure of prior knowledge (Kalyuga, 2009; Mayer, 2012). This factor also included a new item that considers reviewing in-depth the instructions to understand (i.e., 28) that is consistent with the factor. The parallel analysis suggested that this factor may be excluded. However, this analysis should be considered cautiously when components are oblique (Beauducel, 2001; Green et al., 2011).
Concerning differences in educational level, our exploration suggests that students with high school use fewer visual elaboration and summary strategies. The little evidence on this subject suggests that high school students rely more on guided instruction and may therefore be less self-regulated than university students (Lawanto et al., 2013; Baldan Babayigit and Guven, 2020; Theobald, 2021). However, that difference is not clear concerning our students with high school levels studying at the university. A potential explanation for our finding may be that students who have finished a high school level need to develop independent learning skills (Vosniadou, 2020), while students with university degrees may use more visual and verbal elaboration strategies due to the need to autonomously learn a large amount of information that imposes a high cognitive load (Chi et al., 1989; Mirza et al., 2019; Shin and Song, 2022).
Regarding gender differences, our results indicate that males use more deep processing strategies. This result is consistent with previous studies showing gender differences in school learning, for example, that females frequently use more learning strategies (Salahshour et al., 2013) or that males use more retrieval and cognitive strategies (Carr et al., 2016). Our study did not include performance as a dependent variable to examine the effects of gender and the factors measured by the questionnaire. However, this result may serve to delve into theoretical gender-related achievement gaps (Halpern et al., 2007).
In general, our results suggest that the Deep Learning Strategies Questionnaire, while it seems to measure four factors, only the scales for visual elaboration and summary and deep information processing comprised consistent items. The items loaded in the basic and social regulation strategies are nuanced with visual and verbal elaboration and deep information processing measures. This lack of consistency also appears in the original study. For example, If the teacher gives me a tool to self-assess I would use it (item 4 that measures basic strategy) may also be considered social regulation. Similarly, If the teachers provide us with presentations, I take notes in them because it makes everything clearer (item 19, which measures social self-regulation) may also be considered as a visual elaboration and summary strategy.
This lack of consistency may be explained by the characteristics of both self-regulated learning and learning tasks. Mental activities such as planning, monitoring, or relating sources of information seem to be generic skills that do not impose a high mental load when the content or information being processed is already known (Geary, 2008; Tricot and Sweller, 2014). Nevertheless, learning new information or solving problems, even mentally undemanding, requires basic strategies, and some social regulation that includes verbal explanations, reviews to understand, and visual and verbal elaborations to reduce working memory load (Nückles et al., 2020; Roelle et al., 2022). These mental operations become more necessary when the information is more complex, and there is little knowledge about the task (Fiorella and Mayer, 2015; Zambrano et al., 2019).
In summary, this study shows that the Deep Learning Strategies Questionnaire applied to a population of Ecuadorian higher education students has four factors. The internal consistency of each scale was satisfactory, so the results derived from the analysis are reliable. However, the factors of basic self-regulation and social self-regulation strategies involved items that measure other factors (i.e., visual/verbal elaboration and deep processing). The correlation analysis showed that the factors have a positive and significant relationship, except for the relation between social regulation and basic strategies. It also found potential differences in educational level (i.e., participants with finished high school level use fewer visual/verbal elaborations) and gender (i.e., females use fewer deep processing strategies).
These results have educational implications, especially for students with a secondary education level and female students. Teachers should promote generative and deep learning strategies such as concept mapping, verbal explanations, or retrieval practice (Dunlosky et al., 2013; Fiorella and Mayer, 2015). This support should be given with a scaffolding approach (Kirschner et al., 2006; Van Merrieënboer and Kirschner, 2018): the first learning tasks should have a high level of support or external regulation (e.g., worksheets that guide the solution of domain-specific problems that incorporate metacognitive prompts) and then increase autonomy as students become knowledgeable and apply task-based metacognitive monitoring and control automatically.
Our study was not without limitations. Although our study had a lower number of male participants and masters, this may reflect the characteristics of the general teacher population. However, future studies must balance the samples to attribute differences to such groups. Another limitation was that academic performance was not included, nor was the questionnaire applied after a learning session. Future studies should replicate this study by controlling performance on learning tasks (i.e., with pre and post-test) and including mediating factors (e.g., self-efficacy and effort; Panadero et al., 2021). Further, as the parallel analysis suggested three factors and the exploratory analysis found four, more studies should confirm the internal structure of the questionnaire and consider whether deep information processing strategies can be better identified when associated with strategies that explicitly evoke prior knowledge and foster long retention (e.g., spacing and retrieval practice; Carpenter et al., 2022; Lyle et al., 2022).
Data availability statement
The raw data supporting the conclusions of this article will be made available by the authors, without undue reservation.
Ethics statement
The studies involving human participants were reviewed and approved by Postgraduate Committee of the Universidad del Pacífico. The patients/participants provided their written informed consent to participate in this study.
Author contributions
MY and JZ contributed to the conception and design of the study and wrote the first draft of the manuscript. MS organized the database. JZ performed the statistical analysis. All authors contributed to manuscript revision, read, and approved the submitted version.
Conflict of interest
The authors declare that the research was conducted in the absence of any commercial or financial relationships that could be construed as a potential conflict of interest.
Publisher’s note
All claims expressed in this article are solely those of the authors and do not necessarily represent those of their affiliated organizations, or those of the publisher, the editors and the reviewers. Any product that may be evaluated in this article, or claim that may be made by its manufacturer, is not guaranteed or endorsed by the publisher.
Footnotes
- ^ See the complete questionnaire in English and Spanish at: http://www.ernestopanadero.es/Publications/Manuals/DLS_Q_Manual_Deep_Learning_Strategies_questionnaire.pdf.
References
Alavi, M., Visentin, D. C., Thapa, D. K., Hunt, G. E., Watson, R., and Cleary, M. (2020). Chi-square for model fit in confirmatory factor analysis. J. Adv. Nurs. 76, 2209–2211. doi: 10.1111/jan.14399
Allal, L. (2020). Assessment and the co-regulation of learning in the classroom. Assess. Educ. 27, 332–349. doi: 10.1080/0969594X.2019.1609411
Ausubel, D. P. (1960). The use of advance organizers in the learning and retention of meaningful verbal material. J. Educ. Psychol. 51, 267–272. doi: 10.1037/h0046669
Baars, M., Wijnia, L., and Paas, F. (2017). The association between motivation, affect, and self-regulated learning when solving problems. Front. Psychol. 8:1346. doi: 10.3389/fpsyg.2017.01346
Badali, S., Rawson, K. A., and Dunlosky, D. (2022). Do students effectively regulate their use of self-testing as a function of item difficulty? Educ. Psychol. Rev. 34, 1651–1677. doi: 10.1007/s10648-022-09665-6
Baldan Babayigit, B., and Guven, M. (2020). Self-regulated learning skills of undergraduate students and the role of higher education in promoting self-regulation. Eurasian J. Educ. Res. 89, 47–70. doi: 10.20344/amp.8350
Bandura, A. (1986). Social foundations of thought and action: A social cognitive theory. Hoboken, NJ: Prentice-Hall.
Bartsch, L. M., and Oberauer, K. (2021). The effects of elaboration on working memory and long-term memory across age. J. Mem. Lang. 118:104215. doi: 10.1016/j.jml.2020.104215
Beauducel, A. (2001). Problems with parallel analysis in data sets with oblique simple structure. Methods Psychol. Res. Online 6, 141–157.
Bell, B. S., and Kozlowski, S. W. J. (2008). Active learning: Effects of core training design elements on self-regulatory processes, learning, and adaptability. J. Appl. Psychol. 93, 296–316. doi: 10.1037/0021-9010.93.2.296
Biwer, F., De Bruin, A., and Persky, A. (2022). Study smart-impact of a learning strategy training on students’ study behavior and academic performance. Adv. Health Sci. Educ. 1–21. doi: 10.1007/s10459-022-10149-z
Carpenter, S. K., Endres, T., and Hui, L. (2020). Students’ use of retrieval in self-regulated learning: Implications for monitoring and regulating effortful learning experiences. Educ. Psychol. Rev. 32, 1029–1054. doi: 10.1007/s10648-020-09562-w
Carpenter, S. K., Pan, S. C., and Butler, A. C. (2022). The science of effective learning with spacing and retrieval practice. Nat. Rev. Psychol. 1, 496–511. doi: 10.1038/s44159-022-00089-1
Carr, M., Barned, N., and Otumfuor, B. (2016). Peers influence mathematics strategy use in early elementary school. Int. J. Educ. Psychol. 5:27. doi: 10.17583/ijep.2016.1861
Chi, M. T. H., Bassok, M., Lewis, M. W., Reimann, P., and Glaser, R. (1989). Self-explanations: How students study and use examples in learning to solve problems. Cogn. Sci. 13, 145–182. doi: 10.1016/0364-0213(89)90002-5
Dong, A., Jong, M. S.-Y., and King, R. B. (2020). How does prior knowledge influence learning engagement? The mediating roles of cognitive load and help-seeking. Front. Psychol. 11:591203. doi: 10.3389/fpsyg.2020.591203
Dumas, D. (2020). “Strategic processing within and across domains of learning,” in Handbook of strategies and strategic processing, eds D. L. Dinsmore, L. K. Fryer, and M. M. Parkinson (London: Routledge), 11–28.
Dunlosky, J., Rawson, K. A., Marsh, E. J., Nathan, M. J., and Willingham, D. T. (2013). Improving students’ learning with effective learning techniques: Promising directions from cognitive and educational psychology. Psychol. Sci. Public Int. 14, 4–58. doi: 10.1177/1529100612453266
Ekuni, R., de Souza, B. M. N., Agarwal, P. K., and Pompeia, S. (2022). A conceptual replication of survey research on study strategies in a diverse, non-WEIRD student population. Scholarsh. Teach. Learn. Psychol. 8, 1–14. doi: 10.1037/stl0000191
Fan, Y., Van Der Graaf, J., Lim, L., Raković, M., Singh, S., Kilgour, J., et al. (2022). Towards investigating the validity of measurement of self-regulated learning based on trace data. Metacogn. Learn. doi: 10.1007/s11409-022-09291-1
Fiorella, L., and Mayer, R. E. (2015). Eight ways to promote generative learning. Educ. Psychol. Rev. 28, 717–741. doi: 10.1007/s10648-015-9348-9
Fong, C. J., Krou, M. R., Johnston-Ashton, K., Hoff, M. A., Lin, S., and Gonzales, C. (2021). LASSI’s great adventure: A meta-analysis of the Learning and Study Strategies Inventory and academic outcomes. Educ. Res. Rev. 34:100407. doi: 10.1016/j.edurev.2021.100407
García-Pérez, D., Fraile, J., and Panadero, E. (2021). Learning strategies and self-regulation in context: How higher education students approach different courses, assessments, and challenges. Eur. J. Psychol. Educ. 36, 533–550. doi: 10.1007/s10212-020-00488-z
Geary, D. C. (2008). An evolutionarily informed education science. Educ. Psychol. 43, 179–195. doi: 10.1080/00461520802392133
Green, S. B., Levy, R., Thompson, M. S., Lu, M., and Lo, W.-J. (2011). A proposed solution to the problem with using completely random data to assess the number of factors with parallel analysis. Educ. Psychol. Meas. 72, 357–374. doi: 10.1177/0013164411422252
Hadwin, A. F., Järvelä, S., and Miller, M. (2017). “Self-regulated, co-regulated, and socially shared regulation of learning,” in Handbook of self-regulation of learning and performance, 2nd Edn, eds D. H. Schunk and J. A. Greene (London: Routledge), 83–105. doi: 10.1097/ACM.0000000000001583
Hadwin, A., Winne, P., Stockley, D., Nesbit, J., and Woszczyna, C. (2001). Context moderates students’ self-reports about how they study. J. Educ. Psychol. 93, 477–487. doi: 10.1037/0022-0663.93.3.477
Hair, J. F. Jr., Black, W. C., Babin, B. J., and Anderson, R. E. (2019). Multivariate data analysis, 8th Edn. Boston, MA: Cengage.
Halpern, D. F., Benbow, C. P., Geary, D. C., Gur, R. C., Hyde, J. S., and Gernsbacher, M. A. (2007). The science of sex differences in science and mathematics. Psychol. Sci. Public Int. 8, 1–51. doi: 10.1111/j.1529-1006.2007.00032.x
Hirt, C. N., Karlen, Y., Merki, K. M., and Suter, F. (2021). What makes high achievers different from low achievers? Self-regulated learners in the context of a high-stakes academic long-term task. Learn. Individ. Differ. 92:102085. doi: 10.1016/j.lindif.2021.102085
Horn, J. L. (1965). A rationale and test for the number of factors in factor analysis. Psychometrika 30, 179–185. doi: 10.1007/BF02289447
Huang, J., and Prochner, L. (2003). Chinese parenting styles and children’s self-regulated learning. J. Res. Child. Educ. 18, 227–238. doi: 10.1080/02568540409595037
Jansen, R. S., Leeuwen, A., Janssen, J., and Kester, L. (2022). Exploring the link between self-regulated learning and learner behaviour in a massive open online course. J. Comput. Assist. Learn. 38, 993–1004. doi: 10.1111/jcal.12675
Järvelä, S. (2015). How research on self-regulated learning can advance computer supported collaborative learning/El papel de la investigación sobre aprendizaje autorregulado en el desarrollo del aprendizaje colaborativo asistido por ordenador. J. Study Educ. Dev. 38, 279–294. doi: 10.1080/02103702.2015.1016747
Kalyuga, S. (2009). Knowledge elaboration: A cognitive load perspective. Learn. Instr. 19, 402–410. doi: 10.1016/j.learninstruc.2009.02.003
Karabenick, S. A., Woolley, M. E., Friedel, J. M., Ammon, B. V., Blazevski, J., Bonney, C. R., et al. (2007). Cognitive processing of self-report items in educational research: Do they think what we mean? Educ. Psychol. 42, 139–151. doi: 10.1080/00461520701416231
Kirschner, P. A., Sweller, J., and Clark, R. E. (2006). Why minimal guidance during instruction does not work: An analysis of the failure of constructivist, discovery, problem-based, experiential, and inquiry-based teaching. Educ. Psychol. 41, 75–86. doi: 10.1207/s15326985ep4102_1
Lackey, N. R., and Sullivan, J. J. (2003). Making sense of factor analysis: The use of factor analysis for instrument development in health care research. Thousand Oaks, CA: SAGE.
Lawanto, O., Butler, D., Cartier, S., Santoso, H., Goodridge, W., Lawanto, K., et al. (2013). Pattern of task interpretation and self-regulated learning strategies of high school students and college freshmen during an engineering design project. J. Stem Educ. 14, 34–46.
Lee, A., O’Donnell, A. M., and Rogat, T. K. (2015). Exploration of the cognitive regulatory sub-processes employed by groups characterized by socially shared and other-regulation in a CSCL context. Comput. Hum. Behav. 52, 617–627. doi: 10.1016/j.chb.2014.11.072
Lim, K. Y., Lee, H. W., and Grabowski, B. (2009). Does concept-mapping strategy work for everyone? The levels of generativity and learners’ self-regulated learning skills. Br. J. Educ. Technol. 40, 606–618. doi: 10.1111/j.1467-8535.2008.00872.x
Lloret-Segura, S., Ferreres-Traver, A., Hernández-Baeza, A., and Tomás-Marco, I. (2014). El análisis factorial exploratorio de los ítems: Una guía práctica, revisada y actualizada. Anales de Psicología 30, 1151–1169. doi: 10.6018/analesps.30.3.199361
Lyle, K. B., Bego, C. R., Ralston, P. A. S., and Immekus, J. C. (2022). Spaced retrieval practice imposes desirable difficulty in calculus learning. Educ. Psychol. Rev. 34, 1799–1812. doi: 10.1007/s10648-022-09677-2
Mayer, R. E. (2012). “Information processing,” in APA educational psychology handbook: Theories, constructs, and critical issues, Vol. 1, eds K. R. Harris, S. Graham, T. Urdan, C. B. McCormick, G. M. Sinatra, and J. Sweller (Washington, D.C: American Psychological Association), 85–99. doi: 10.1037/13273-004
Mirza, F., Agostinho, S., Tindall-Ford, S., Paas, F., and Chandler, P. (2019). “Self-management of cognitive load. Potencial and challenges,” in Advances in cognitive load theory: Rethinking teaching, (London: Routledge), 157–167. doi: 10.4324/9780429283895-13
Montalvo, F. T., and Torres, M. C. G. (2004). El aprendizaje autorregulado: Presente y futuro de la investigación. Electron. J. Res. Educ. Psychol. 2, 1–33.
Näykki, P., and Järvelä, S. (2008). How pictorial knowledge representations mediate collaborative knowledge construction in groups. J. Res. Technol. Educ. 40, 359–387. doi: 10.1080/15391523.2008.10782512
Nelson, T. O., and Narens, L. (1990). “Metamemory: A theoretical framework and new findings,” in Psychology of learning and motivation, Vol. 26, ed. G. H. Bower (Cambridge, MA: Academic Press), 125–173. doi: 10.1016/S0079-7421(08)60053-5
Nückles, M., Roelle, J., Glogger-Frey, I., Waldeyer, J., and Renkl, A. (2020). The self-regulation-view in writing-to-learn: Using journal writing to optimize cognitive load in self-regulated learning. Educ. Psychol. Rev. 32, 1089–1126. doi: 10.1007/s10648-020-09541-1
Núñez Perez, J. C., Solano Pizarro, P., González-Pienda, J. A., and Rosário, P. (2006). El aprendizaje autorregulado como medio y meta de la educación. Papeles del Psicoìlogo 27, 139–146.
Panadero, E. (2017). A review of Self-regulated Learning models: Zimmerman, Boekaerts, Winne & Hadwin, Pintrich, Efklides, and Hadwin, Järvelä & Miller. Front. Psychol. 8:422. doi: 10.3389/fpsyg.2017.00422
Panadero, E., Alonso-Tapia, J., García-Pérez, D., Fraile, J., Sánchez Galán, J. M., and Pardo, R. (2021). Estrategias de aprendizaje profundas: Validación de un modelo situacional y su cuestionario/Deep learning self-regulation strategies: Validation of a situational model and its questionnaire. Revista de Psicodidáctica 26, 10–19. doi: 10.1016/j.psicod.2020.11.003
Panadero, E., and Järvelä, S. (2015). Socially shared regulation of learning: A review. Eur. Psychol. 20, 190–203. doi: 10.1027/1016-9040/a000226
Panadero, E., Klug, J., and Järvelä, S. (2015). Third wave of measurement in the self-regulated learning field: When measurement and intervention come hand in hand. Scand. J. Educ. Res. 60, 723–735. doi: 10.1080/00313831.2015.1066436
Pilegard, C., and Fiorella, L. (2016). Helping students help themselves: Generative learning strategies improve middle school students’ self-regulation in a cognitive tutor. Comput. Hum. Behav. 65, 121–126. doi: 10.1016/j.chb.2016.08.020
Roelle, J., Froese, L., Krebs, R., Obergassel, N., and Waldeyer, J. (2022). Sequence matters! Retrieval practice before generative learning is more effective than the reverse order. Learn. Instr. 80:101634. doi: 10.1016/j.learninstruc.2022.101634
Roth, A., Ogrin, S., and Schmitz, B. (2015). Assessing self-regulated learning in higher education: A systematic literature review of self-report instruments. Educ. Assess. Eval. Account. 28, 225–250. doi: 10.1007/s11092-015-9229-2
Rubio, J. R., and García, ÁP. (2018). Estrategias de aprendizaje significativo en estudiantes de Educación Superior y su asociación con logros académicos. Revista electrónica de investigación y docencia 19, 145–166. doi: 10.17561/reid.v0i19.3570
Salahshour, F., Sharifi, M., and Salahshour, N. (2013). The relationship between language learning strategy use, language proficiency level and learner gender. Procedia-Social Behav. Sci. 70, 634–643. doi: 10.1016/j.sbspro.2013.01.103
Schraw, G. (2008). A conceptual analysis of five measures of metacognitive monitoring. Metacogn. Learn. 4, 33–45. doi: 10.1007/s11409-008-9031-3
Schreiber, J. B., Nora, A., Stage, F. K., Barlow, E. A., and King, J. (2010). Reporting Structural Equation Modeling and Confirmatory Factor Analysis Results: A Review. J. Educ. Res. 99, 323–338. doi: 10.3200/joer.99.6.323-338
Schunk, D. H. (1986). Verbalization and children’s self-regulated learning. Contemp. Educ. Psychol. 11, 347–369. doi: 10.1016/0361-476X(86)90030-5
Schunk, D. H., and Greene, J. A. (2018). “Historical, contemporary, and future perspectives on self-regulated learning and performance,” in Handbook of self-regulation of learning and performance, eds D. H. Schunk and J. A. Greene (London: Routledge), 1–16. doi: 10.4324/9781315697048
Shi, Y., Frederiksen, C. H., and Muis, K. R. (2013). A cross-cultural study of self-regulated learning in a computer-supported collaborative learning environment. Learn. Instr. 23, 52–59. doi: 10.1016/j.learninstruc.2012.05.007
Shin, Y., and Song, D. (2022). The effects of self-regulated learning support on learners’ task performance and cognitive load in computer programing. J. Educ. Comput. Res. 60, 1490–1513. doi: 10.1177/07356331211052632
Son, L. K., and Schwartz, B. L. (2002). “The relation between metacognitive monitoring and control,” in Applied metacognition, eds T. J. Perfect and B. L. Schwartz (Cambridge: Cambridge University Press), 15–38. doi: 10.1017/CBO9780511489976.003
Sweller, J., and Paas, F. (2017). Should self-regulated learning be integrated with cognitive load theory? A commentary. Learn. Instr. 51, 85–89. doi: 10.1016/j.learninstruc.2017.05.005
Theobald, M. (2021). Self-regulated learning training programs enhance university students’ academic performance, self-regulated learning strategies, and motivation: A meta-analysis. Contemp. Educ. Psychol. 66:101976. doi: 10.1016/j.cedpsych.2021.101976
Tricot, A., and Sweller, J. (2014). Domain-specific knowledge and why teaching generic skills does not work. Educ. Psychol. Rev. 26, 265–283. doi: 10.1007/s10648-013-9243-1
Usher, E. L., and Schunk, D. H. (2018). “Social cognitive theoretical perspective of self-regulation,” in Handbook of self-regulation of learning and performance, 2nd Edn, (London: Routledge), 19–35.
Van Gog, T., Kester, L., and Paas, F. (2011). Effects of concurrent monitoring on cognitive load and performance as a function of task complexity. Appl. Cogn. Psychol. 25, 584–587. doi: 10.1002/acp.1726
Van Merrieënboer, J. J. G., and Kirschner, P. A. (2018). Ten steps to complex learning: A systematic approach to four-component instructional design, 3th Edn. London: Routledge.
Veenman, M. V. J. (2007). The assessment and instruction of self-regulation in computer-based environments: A discussion. Metacogn. Learn. 2, 177–183. doi: 10.1007/s11409-007-9017-6
Vosniadou, S. (2020). Bridging secondary and higher education. The importance of self-regulated learning. Eur. Rev. 28, S94–S103. doi: 10.1017/s1062798720000939
Watkins, M. W. (2018). Exploratory factor analysis: A guide to best practice. J. Black Psychol. 44, 219–246. doi: 10.1177/0095798418771807
Weinstein, C. E., Zimmermann, S. A., and Palmer, D. R. (1988). “Assessing learning strategies: The design and development of the LASSI,” in Learning and study strategies: Issues in assessment, instruction, and evaluation, eds C. E. Weinstein, E. T. Goetz, and P. A. Alexander (Cambridge, MA: Academic Press), 25–40. doi: 10.1016/B978-0-12-742460-6.50009-8
Witherby, A. E., and Carpenter, S. K. (2021). The rich-get-richer effect: Prior knowledge predicts new learning of domain-relevant information. J. Exp. Psychol. 45, 37–55. doi: 10.1037/xlm0000996
Wolters, C. A., and Won, S. (2017). “Validity and the use of self-report questionnaires to assess self-regulated learning,” in Handbook of self-regulation of learning and performance, eds D. H. Schunk and J. A. Greene (London: Routledge), 307–322.
Zambrano, R. J., and Yaguarema, M. (2021). Estrategias de enseñanza efectivas para los tiempos de y pospandemia. Yachana Revista Científica 10, 40–45.
Zambrano, R. J., Kirschner, F., Sweller, J., and Kirschner, P. A. (2019). Effects of prior knowledge on collaborative and individual learning. Learn. Instruct. 63:101214. doi: 10.1016/j.learninstruc.2019.05.011
Zimmerman, B. J. (2002). Becoming a self-regulated learner: An overview. Theory Into Practice 41, 64–70. doi: 10.1207/s15430421tip4102_2
Zimmerman, B. J., and Moylan, A. R. (2009). “Self-regulation: Where metacognition and motivation intersect,” in Handbook of metacognition in education, eds D. J. Hacker, D. John, and A. C. Graesser (London: Routledge), 299–315.
Keywords: self-regulated learning, measurement of self-regulated learning, gender differences, university students, generative activity, learning strategies
Citation: Yaguarema M, Zambrano R J and Salavarría M (2022) Analysis of the Deep Learning Strategies Questionnaire with Ecuadorian students. Front. Educ. 7:1004874. doi: 10.3389/feduc.2022.1004874
Received: 27 July 2022; Accepted: 13 October 2022;
Published: 02 November 2022.
Edited by:
Evely Boruchovitch, State University of Campinas, BrazilReviewed by:
Mario Mäeots, University of Tartu, EstoniaZuleima Santalla-Banderali, Espiritu Santo University, Ecuador
Copyright © 2022 Yaguarema, Zambrano R and Salavarría. This is an open-access article distributed under the terms of the Creative Commons Attribution License (CC BY). The use, distribution or reproduction in other forums is permitted, provided the original author(s) and the copyright owner(s) are credited and that the original publication in this journal is cited, in accordance with accepted academic practice. No use, distribution or reproduction is permitted which does not comply with these terms.
*Correspondence: Jimmy Zambrano R., amltbXkuemFtYnJhbm9AdXBhY2lmaWNvLmVkdS5lYw==
†These authors have contributed equally to this work and share first authorship