- 1School of Economic and Administrative Sciences, Corporación Universitaria de Asturias, Bogotá, Colombia
- 2Faculty of Natural Sciences and Engineering, Universidad de Bogotá Jorge Tadeo Lozano, Bogotá, Colombia
Higher education is one of the ways to overcome social inequalities in rural areas in developing countries. This has led states to develop public policies aimed at access, retention and timely graduation of students in those sectors, yet the high drop-out rates among the rural student population, which were catalysed by COVID-19, prevent the intrinsic and extrinsic benefits of obtaining a higher education degree from materialising. Thus, the study of the phenomenon of dropout before and after the pandemic has not sufficiently addressed the economic issues raised by this phenomenon for the different actors at the educational level. The purpose of this paper is to model the economic effects of rural student dropout at the higher education level for students and families, Higher Education Institutions (HEIs) and the State, based on public policies for access to higher education, in the pandemic and post-pandemic scenario. In order to delimit the operationalisation of the proposed model, a set of undergraduate training programmes in Colombia was taken as a reference. System dynamics was used as the main modelling technique. The model was based on data from the 20 training programmes with the highest number of students enrolled in rural areas for the year 2019, by running three computational simulations. The results showed the description of the dynamic model and the financial effects of dropout for the actors of the educational level with the current policies of access to higher education, the scenario in which COVID-19 would not have occurred and the consolidation of the public policy of tuition fee exemption in public HEIs as a result of the pandemic. It was concluded that the model developed is very useful for the valuation of these economic effects and for decision-making on policies to be implemented, given that the costs of dropout are characterised by high costs for students and their families as well as for HEIs, and where it was determined that current policies are inefficient in preventing and mitigating dropout.
Introduction
Higher education has been conceived as a way to overcome social inequalities in developing countries (Marginson, 2011; OECD 2015; Herbaut and Geven, 2020; Guzmán et al., 2021a), hence, the interest of the states to intervene through the development of public policies with the aim of facilitating access, permanence and timely graduation of students at this level of education (Herbaut and Geven, 2020). That said, it is recognised that there are a number of population groups where social inequalities are more pronounced, especially those located in rural areas. This was confirmed by the United Nations when it stated that by the year 2020, 80% of the world’s poor people would live in rural areas, and that in some countries the majority of the population would be concentrated in rural areas (United Nations, 2021).
In this context, public policies developed by states to facilitate access, retention and timely graduation of students in rural areas of developing countries, especially in developing countries, have been based on a paradigm in which the state assumes the role of funder of students (Marginson, 2016; McCowan, 2016). In this way, the State finances tuition under the form of educational credits or tuition fee exemptions, either totally or partially, where in the latter case, it relies on the Higher Education Institutions (HEI), the family or the student him/herself to cover the totality of the expenses.
Although there has been a generalised concern on the part of states regarding access, retention and timely graduation in higher education for rural populations, it is necessary to recognise that the strategies used for financing bring with them multiple effects for the actors at the educational level (student and family, HEI, State, among others), especially when there are high dropout rates in this student population, as exemplified in the Colombian case where the Ministry of National Education (Ministry of National Education, 2009) indicated that the dropout rate per cohort was close to 50%. Taking into consideration what was previously stated added to the context of the COVID-19 pandemic, it has been identified as a catalyst for problems at the educational level (e.g: Pokhrel and Chhetri, 2021; Dennis, 2020; Mailizar et al., 2020; Abbasi et al., 2020; Sobaih et al., 2020; Favale et al., 2020; Basilaia and Kvavadze, 2020; Kerres, 2020; Wang et al., 2020), including dropout levels among the most vulnerable populations (Guzmán et al., 2021a; United Nations, 2020), Hence, a critical analysis of the economic effects of this pandemic on education stakeholders related to the drop-out phenomenon is required.
In this sense, both public policy makers and researchers at the higher education level have not analysed in detail, either before or during the pandemic, the financial problems caused by drop-out in rural populations for students and their families, HEIs and the state, within the framework of public policies for financing higher education. This is largely due to the lack of robust models that allow the valuation of the economic effects of dropout, as well as the general interest of the academic community in analysing other aspects of the COVID-19 pandemic for this student population, such as the use of and access to technological resources (Cameron-Standerford et al., 2020).
In this context, the analysis of the economic effects of dropout in the framework of public higher education funding policies, prior to and during COVID-19, has been characterised by being clustered at the national or state level (e.g.: Sahoo et al., 2021; Dennis, 2020; World Bank, 2020; Denning, 2017; Richburg-Hayes et al., 2015; Bettinger, 2015), or, cases of individual studies in an HEI (e.g.: Bernal, 2018; Barragán and Rodríguez, 2015), for which there is not a panorama reflecting the realities of rural students. In addition, the improvements developed are not usually of a holistic nature and integrate the actors at the educational level, but are characterised by being individualised for each of the actors (student and family, HEI or State), especially in the field of modelling (e.g: Cristia and Pulido 2020; Moreno et al., 2019, Sosu and Pheunpha 2019; Hällsten 2017; Fack and Grenet 2015; Rubin 2011; Qu, 2009).
Considering the public policies of access to education, the phenomenon of dropout in rural populations, the effects that COVID-19 has had on the educational level, the fragmentary analysis of the economic effects of dropout and the lack of models that integrate the actors of the educational level, especially in rural populations, the aim of this article was to model the economic effects of rural student dropout at the higher education level for students and families, HEIs and the state, based on public policies for access to higher education, in the pandemic and post-pandemic scenario. For the operationalisation of the proposed model, a set of undergraduate training programmes in Colombia was taken as a reference.
The selection of Colombia for the operationalisation of the model is due to the various social disparities experienced in the country, which are directly or indirectly related to the level of education, and which have been catalysed by COVID-19 especially in rural areas, such as: high levels of poverty, low employability, poor accessibility to basic services such as electricity and internet, unequal access to information and communication technologies, among others. This was made evident in the Agronet Report (2020), where in rural areas 44,362 people became newly unemployed and 108,000 unemployed 2 weeks after the declaration of the national health emergency, and the contraction of the economy has affected the rural population, leading to a generalised decrease in food prices and, therefore, in the income of this population.
With the fulfilment of the objective, various contributions are made to the analysis of the problem of drop-out in the rural student population. Firstly, this article complements the advances in the understanding of both the economic effects of dropout in the framework of COVID-19 and its modelling, as it concerns all higher education actors; this complementation is based on the methodological contribution in terms of dynamic modelling, adding a holistic perspective to the analysis in relation to rural populations, as well as a more robust model for the understanding of the studied phenomenon. Secondly, this article provides feedback to the managers of public policies on the financing of higher education based on credit and tuition fee waivers with the aim of maintaining, modifying or eliminating them, in order to mitigate to some extent the financial consequences of dropout and overcome the social inequalities experienced in rural areas of developing countries. Thirdly, the model makes it easier for direct and indirect users of educational access policies to make informed decisions by recognising the economic effects of dropping out.
This article is divided into five sections. The first one was the introductory overview and justification; the second concerns the theoretical framework and proposed model, which presents the conceptualisation of dropout, the documented economic effects on education stakeholders and concludes with the proposed dynamic model; the third concerns the methodology used for the fulfilment of the objective and for the operationalisation of the model through the use of system dynamics; the fourth reports on the design of the model and the results of the computational simulations developed; and the fifth discusses the main findings and incorporates the conclusions.
Theoretical Framework and Proposed Model
Drop-Out
Student drop-out as an educational phenomenon does not have a single meaning, but rather there are multiple definitions in the literature and public policies. This is a result of the complexity of this phenomenon which involves several levels of analysis as stated by Guzmán et al. (2021a), Guzmán et al. (2021b) and Kehm et al. (2019). In this sense, this variety of conceptualisations allows for a broader understanding of dropout because it links different aspects, variables, representations, models and effects of dropping-out.
Taking into consideration what was previously stated, the meanings can be categorised as theoretical and operational. In the case of the theoretical ones, they obey those provided by the academic community in which the interaction of multiple explanatory variables of the drop-out phenomenon is contemplated, such as the one given in the framework of the ALFA GUIA project in which this phenomenon was defined as “the cessation of the relationship between the student and the training programme leading to the award of a Higher Education degree, before achieving the degree. It is also an event of a complex, multidimensional and systemic nature, which can be understood as cause or effect, failure or reorientation of a training process, choice or obligatory response, or as an indicator of the quality of the education system” (Proyecto ALFA GUIA DCI-ALA/2010/94, 2013, p. 6); or, as explained by Zuñiga (2006), the student’s decision to terminate his or her training process in advance of its completion.
The second category corresponds to the operational meanings developed by public policies, which facilitate the measurement of drop-out at the higher education level, as well as the evaluation and monitoring of some variables. In the Colombian case, this definition is given according to the time in which a student was not linked to the HEI, being considered a deserter if he/she has not legalised enrolment in two consecutive academic periods, and if he/she has not graduated or dropped out (Ministry of National Education, 2009). This article falls into the latter category, as it facilitates time-dependent counts, allowing the economic effects of the phenomenon to be modelled and assessed.
Recognising drop-out is a multifactorial circumstance and based on the nature of the objective of this article, its study is based on an economistic approach which seeks to understand which socio-economic variables influence students’ decision to end their education process early, as well as the effects of this decision on higher education stakeholders. In this respect, the preference of various authors for investigating the socio-economic context of the student, the identification and treatment of this type of variable as presented in the studies developed by Rodríguez-Hernández et al. (2020), Palacio Sprockel et al. (2020), Adrogue and García (2018), De Clercq et al. (2017), Erola et al. (2016), among others. This same situation is present in the study of dropout in rural higher education, as evidenced in the works of (Cook et al., 2021; Mncube et al., 2021; Guzmán et al., 2021b; Castleman and Meyer, 2020; Ramírez et al., 2020; Lewine et al., 2019; Muñoz, 2013; De Hart and Venter, 2013; Qu, 2009).
However, the literature concerning the economic effects of drop-out for higher education stakeholders has not been widely addressed, especially from the perspective of public policies on access, retention and timely graduation. Thus, Herbaut and Geven (2020) indicated that in recent years this type of financial consequences have received increasing, but still insufficient, attention. Studies have shown that this type of policy has the capacity to reduce the drop-out rate and increase the graduation rate in the medium term, representing a higher level of indebtedness for students and their families, as well as a lower stranded cost for HEIs. However, from the approach of these policies it has also been observed that in the long term they lose the capacity to reduce this rate once the expected result of the implementation of public policies of access to higher education based on credit has been achieved (Mayer et al., 2015). In the case of HEIs, research has quantified the stranded costs of drop-out and the effects they have on their substantive functions (e.g.: Barragán and Rodríguez, 2015). Finally, the economic effects of this phenomenon on states have been linked to its potential to mitigate the improvement in the income of the population (Cristia and Pulido 2020) and its inability to increase productivity (Atchoarena et al., 2005; McMahon, 2010), lacking an analysis of higher education access policies that are implemented for specific educational populations.
Based on this theoretical framework, the economic effects of drop-out on students and their families, HEIs and the state are presented below in the context of public policies on access to higher education.
Economic Effects of Drop-Out on the Student and Family
Human Capital Theory, consolidated by Becker (1962), suggests that the student and his family are rational actors who base their decision to invest in education on comparing the costs (e.g. tuition values, possible sunk costs, etc.) and the monetary benefits (e.g. wages, rental income, etc.) of higher educational achievement (Marginson, 2019). The student and his or her family will make the decision to continue their education according to the short, medium and long term economic benefits for them (Marginson, 2019; Didenko, 2015 cited by; Gruzina et al., 2021). Thus, they will make a joint decision to enter higher education, comparing the cost of tuition fees at HEIs, the possibilities of financing with their own resources (Qu, 2009) or the possibility of financing with public or private entities (Nizar and Nazir, 2020; Suhendra, 2020). In this way, of the total number of applicants (high school graduates), only those students who have the financial capacity or who perceive some benefit or a better opportunity cost of higher education in the terms expressed in the Human Capital Theory will be admitted (Chen and DesJardins, 2008; Chen and DesJardins, 2010; Özdoğan, 2021).
In other words, it is understood that the more academic periods a student spends in higher education, the greater the investment made, and consequently in the event of the student dropping out, the higher the investment will be if the student decides to interrupt the process due to the influence of individual variables, (Arias-Velandia et al., 2018; Behr et al., 2020), socioeconomics (Contreras, 2018; Palacio Sprockel et al., 2020; Schmitt et al., 2020), academics (Guzmán, et al., 2020; Heidrich et al., 2018) and institutional variables (Armstrong et al., 2018; Choi and Kim, 2018), as well as the higher the stranded cost will be for the student or the student’s family (Larroucau, 2016; Laaser, 2018). In addition, by resorting to credits originating in public policies, the dropout student and his or her family will incur interest payments, which represents an increase in these costs (Moreno et al., 2019). Due to the existence of drop-outs, it is expected that the number of students enrolled in HEIs in this cohort will decrease (Barragán and González, 2017). Finally, the lack of timely graduation of students represents a major stranded cost when students drop out of school (OECD, 2017).
However, the literature has shown the impact caused by the COVID-19 pandemic on the graduation rate of rural high school graduates, represented in a lower number of students with this academic level and triggering a social crisis (Chatterji and Li, 2021; United Nations, 2021) as a result of not being able to attend classes and not having access to adequate Information and Communication Technologies to ensure their training process (Expósito and Marsollier, 2020; Mncube et al., 2021). On the other hand, COVID-19 has decreased the rate of access to higher education, due to the high costs that the educational level represents for the student and his family, as a result of the economic crisis and the social emergency, since they cannot cover the tuition and other costs associated with the educational level either with their own resources or with credits (Potra et al., 2021; United Nations, 2021). In addition, COVID-19 has led to a decrease in student retention in higher education, which implies a higher drop-out rate (Cruz et al., 2020; Alyoussef, 2021; Guzmán et al., 2021b; Delnoij et al., 2021; López-Aguilar and Álvarez-Pérez, 2021; Teuber et al., 2021).
Economic Effects of Drop-Out in HEIs
As in the case of students and their families, drop-out at the higher education level has a direct impact on the finances of HEIs by generating economic instability, especially in those that depend on tuition fees (private and mixed economy HEIs), and in the case of public HEIs, dropping out is seen as a waste of public funds (Choudhary and Hammayun, 2015; Esteban et al., 2017; Becerra et al., 2020).
In this sense, HEIs with a high drop-out rate have high opportunity costs, as they lose out on tuition fees for a number of periods not taken (Barragán and Rodríguez, 2015; Améstica-Rivas et al., 2020). Thus, it is presumed that the more academic periods a student attends, the lower the opportunity cost for HEIs will be. The quantification of the opportunity cost to HEIs resulting from dropping out has been of great interest in developing countries in view of the difficulties faced by institutions in ensuring their sustainability, particularly in developing countries. An example of this is the work developed by Bernal (2018) in which this cost was estimated for a Colombian HEI for the period 2011 to 2014, which amounted to 9,430,866,735 pesos (equivalent to USD 2,468,177), or the work developed by Améstica-Rivas et al. (2020) who estimated that the opportunity cost for Chilean HEIs was USD 23,000,000 for those students who had scholarship credits.
In addition to calculating this opportunity cost, the literature recognises that the higher the drop-out rate, the more difficulties HEIs face in the development of their substantive functions (teaching, research and social outreach), since they have fewer resources available for hiring teachers, training them, developing research projects, disseminating their results and managing links with the external sector, among other things (Voelkle and Sander, 2008). Consequently, the opportunity costs of student drop-out have a direct impact on the quality conditions of the programmes, as well as on the reputation of HEIs, leading to a decrease in the access rate to HEIs (Ortiz and Dehon, 2013; Basilaia and Kvavadze, 2020).
In contrast to the stranded cost of untimely graduation for the student and his/her family, for HEIs this becomes an unconsidered income which, although not directly related in the literature, can to some extent reduce the opportunity cost and the difficulties of drop-out in the substantive functions.
Economic Effects of Drop-Out for the State
In the case of the state, the economic effects of drop-out have been addressed in two ways. The first concerns the consequences on the economy in the short, medium and long term, this being the main line, where studies have indicated the loss of income and purchasing power by the student population who drop-out, which results in lower productivity for the State represented in a stagnation or decrease in Gross Domestic Product (Chetty et al., 2020; Hanushek and Woessmann, 2020); and the second, which concerns the monetary quantification of the drop-out in which this article is focused.
Thus, in the case of this second stream, previous research recognises that states, when they assume student fees, especially in public HEIs, assume a stranded cost (Choudhary and Hammayun, 2015), as stated by the World Bank (2020) “ (…) students who do not graduate on time (or at all) when they receive public funding consume valuable fiscal resources, which in many cases are not recoverable” (p. 14). On the other hand, and based on the educational credit policy to facilitate access to higher education, the states that implement it accept the risk of non-payment by students and their families (Moreno et al., 2019), which leads them to accept such a loss of resources, implying a de-financing of state programmes to grant credits (Améstica-Rivas et al., 2020; López-Aguilar and Álvarez-Pérez, 2021).
Proposed Dynamic Model
Based on the economic effects of drop-out for the student and family, HEIs and the state, as described in the preceding sections, a Causal Loop Diagram or dynamic hypothesis was designed (see Figure 1), in which the existing relationships between the variables of the system are synthesised.
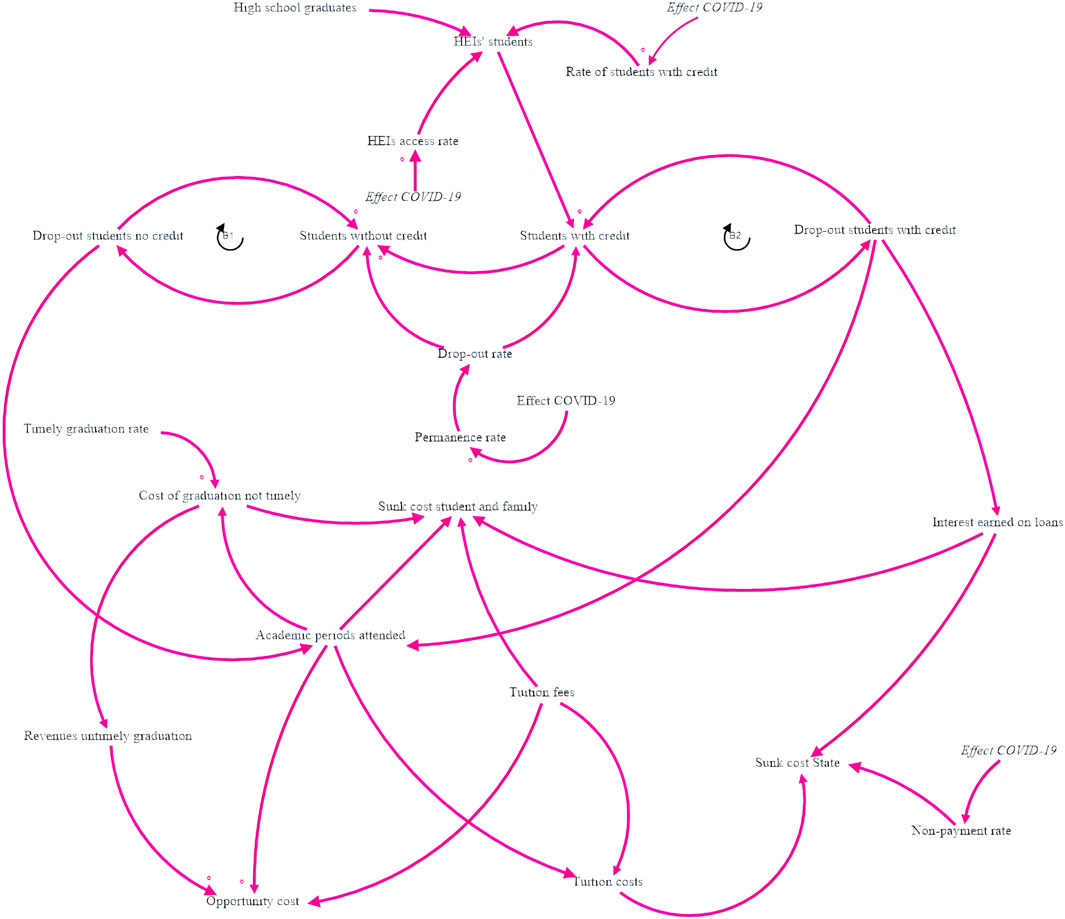
FIGURE 1. Causal Loop Diagram. Note: B indicates that it is a balance loop. For the purposes of this diagram, positive relationships have no loadings on the connectors.
High school graduates who are the potential population for admission to higher education undergo the admission process and, once accepted by a HEI, may choose to pay their tuition fees by means of a loan or use their own and their family’s resources. Hence, two causal loops are formed. The first one (B1), relates students who did not have access to credits, who are affected by a drop-out rate which, being high, will represent a higher number of non-credit drop-outs and consequently a lower number of students. The second (B2) represents credit drop-outs who, like non-credit students, are affected by a drop-out rate, resulting in an increase in credit drop-outs. The higher the drop-out rate in either case, the lower the retention rate and the higher the stranded cost for the student and his/her family. The higher the number of academic periods completed, the higher the stranded cost due to the impact of variables such as the cost of untimely graduation, tuition fees and interest on credits.
As far as the opportunity cost of HEIs is concerned, this is determined by the cost of untimely graduation, given that the higher the number of academic periods taken in addition to those established in the study plan, the lower the cost will be, generating unforeseen income for the HEI, provided that they are private. In the case of public HEIs, the cost of untimely graduation represents a detriment to the state. On the other hand, the earlier students drop-out, i.e. the earlier they have completed fewer academic terms, the higher the opportunity cost.
In the case of the stranded cost of the state, this will be the result of the value of the tuition fees of students who drop out of public HEIs and the rate of non-payment of credits granted in the framework of public policies for access to higher education. Finally, COVID-19 influences multiple variables that cause the behaviour of the system to vary, such as the number of new entrants, the survival rate and the drop-out rate, among others, thus intensifying the economic effects of drop-out on the actors in the higher education system.
Methodology
In order to fulfil the objective of this article, and to operationalise the proposed model (Figure 1), System Dynamics was used as the main modelling and simulation technique. This technique is borrowed from control theory, more specifically, from feedback systems and is distinguished by its ability to deal with nonlinearity, time delay, and multi-loop structures of complex and dynamic systems (Bala et al., 2017). Thus, System Dynamics allows us to analyse the structure of a system, the interactions between its elements and the behaviours derived from these interactions as a function of time (
That said, for the formal construction of the model, the procedure suggested by Bala et al. (2017) was followed, which consists of four stages. The first corresponded to the construction of the flows and levels diagram, understood as the physical structure of the system, in which the levels represent its condition for a defined
In the case of stage three, we used historical data from the National Higher Education Information System (SNIES), the System for the Prevention of Drop-out in Higher Education (SPADIES) and the MEN. In the case of SNIES, information related to undergraduate programmes (technical, technologist and professional) taught in rural areas was extracted, as well as the evolution of first semester enrolments in these programmes and the annual cost of enrolments. In total, the 20 training programmes with the highest number of enrolled students from rural areas for the year 2019 were included, being 25% technical training, 25% technological training and 50% vocational training (see Supplementary Material Table S1). From SPADIES, we extracted the number of students per training programme who accessed a credit with ICETEX (2021) in the medium and long-term modality and the survival rate of each of the training programmes; this rate represents the proportion of students in each semester who remain enrolled (Ministry of National Education, 2009). Finally, from the studies developed by the MEN, the impact of COVID-19 at the higher education level was determined. The period of observation of the data was from 2015 to 2020, whose history was crucial for the estimation of parameters and validation of the model proposed in this article in terms of structure and behaviour.
With the model and data, the model was run to assess the economic effects of rural student drop-out on higher education stakeholders. To this end, the simulations described in Table 1 were carried out.
Finally, the computational work on the model and the simulations were implemented in Stella Architect Software version 1.9.5. The following model settings were taken into account:
Results
In accordance with the methodology, the following presents firstly the formal construction of the model, and secondly the results of the simulation scenarios.
Forrester Diagram and Mathematical Model
Based on the dynamic hypotheses (see Figure 1), the Forrester diagram and the mathematical model were designed considering the flow, level and auxiliary variables necessary to replicate the drop-out phenomenon (see Supplementary Material S2). With the design of the diagram and the base model presented below, the corresponding adaptations were made to run the simulations, since the training programmes in the sample have different numbers of academic periods that students must complete to graduate (see Guzmán et al., 2021b; Guzmán et al., 2021c; Guzmán et al., 2021d). Thus, the base model was divided into five subsystems.
Subsystem One
The first subsystem corresponded to the behaviour of enrolment, retention, dropout and graduation of rural students at the higher education level. This starts with first semester enrolment, which is affected by the number of available bachelor graduates in the catchment area of the HEI and the first semester enrolment rate. Subsequently, enrolled students must make a decision at the end of each academic period whether to continue their education or drop out, and this is repeated until the group of students graduates, thus forming the group of graduates. The equations describing the behaviour of this subsystem are presented below. It should be noted that, for all subsystems,
The previous set of equations operates as long as the technical condition of non-negativity are found
On the other hand, by not considering academic periods prior to the observation period
Subsystem Two
The second sub-system represented the enrolment, retention, dropout and graduation of rural students in one of the state programmes with respect to the financing of enrolment with educational credits. Recognising that each country has particular policies, a synthesis of the mathematical model that can be adjusted to different varieties of educational credits is presented, which, in the case of Colombia, are categorised as long and medium term.
As long as the provided technical conditions of non-negativity are found,
Now, for the observation period
Subsystem Three
The third sub-system describes the economic effects of drop-out for HEIs. It is divided into three sectors. The first sector corresponds to the opportunity cost for HEIs, where the higher the number of dropouts in the first academic terms, whether credit or non-credit students, the higher the opportunity cost. For the quantification of this cost a time lag was used, recognising that once a student drops out, it is in the next academic term that the financial impact will materialise. This is represented in the following set of equations.
The second sector corresponds to the benefits or additional income resulting from untimely graduation, these are only quantified when part of the student population attends more academic periods than those established by the training programme; for the purposes of this study, only two additional academic periods were considered, although the model is adjustable to as many periods as required. The equations for this sector are described below.
The third sector of this subsystem quantifies the financial effects of drop-out for HEIs. In this case
4.1.4 Subsystem Four
The fourth sub-system refers to the financial effects of drop-out for the student and his/her family. This represents the stranded cost which is the result of the cumulative value of tuition fees paid by dropouts, the interest generated by educational credits and the cost of untimely graduation. This is shown in the following set of equations.
Subsystem Five
The fifth sub-system represents the financial effects for the state. In this case, a portion of dropouts will not be able to cover the debts of their educational credits, and this portion is likely to increase due to the effects of COVID-19. Furthermore, in the case of public HEIs where the state bears the cost of tuition fees, drop-out represents an additional cost. This is described by the following set of equations.
The above set of equations operates, as long as the provided technical conditions of non-negativity for
Operationalisation Results of the Model and Simulations
With regard to the simulations, in the case of SIM-1, which reflected the reference mode, as for example the behaviour of the system under the initial parameters, it was found that in the case of the technical training programmes from the second semester of 2019, 2019-2 (
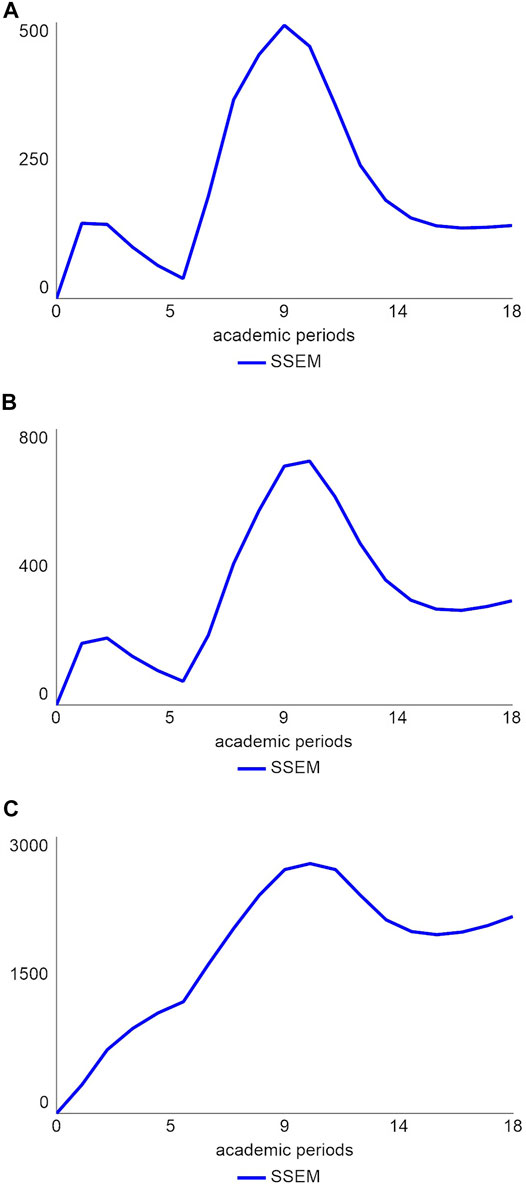
FIGURE 2. Drop-out behaviour by academic period for SIM-1. Note: (A) presents technical training programmes, (B) technology training and (C) professional training. SEM: Total number of dropouts per academic period.
In terms of student drop-out rates for the technical, technological and vocational training programmes in the sample, before the start of the pandemic there were 747, 330 and 4,250 drop-outs respectively (see Figure 3), as a result of the effect of COVID-19, it was estimated that for the period 2024-1 (
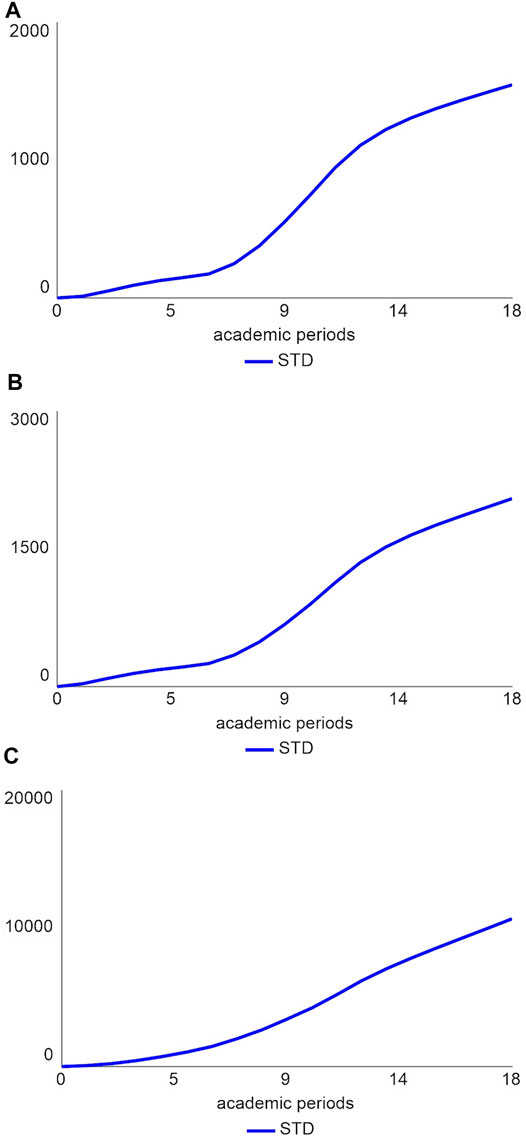
FIGURE 3. Drop-out behaviour for SIM-1. Note: (A) presents technical training programmes, (B) technological training and (C) professional training. STD: Total number of dropouts per
However, with regard to the number of students with academic credits who drop out, it is characterised by being low in comparison with the number of total dropouts from the training programmes under study, which can be explained to a large extent by the low rate of access to educational credits by the rural population. In this sense, the simulation for
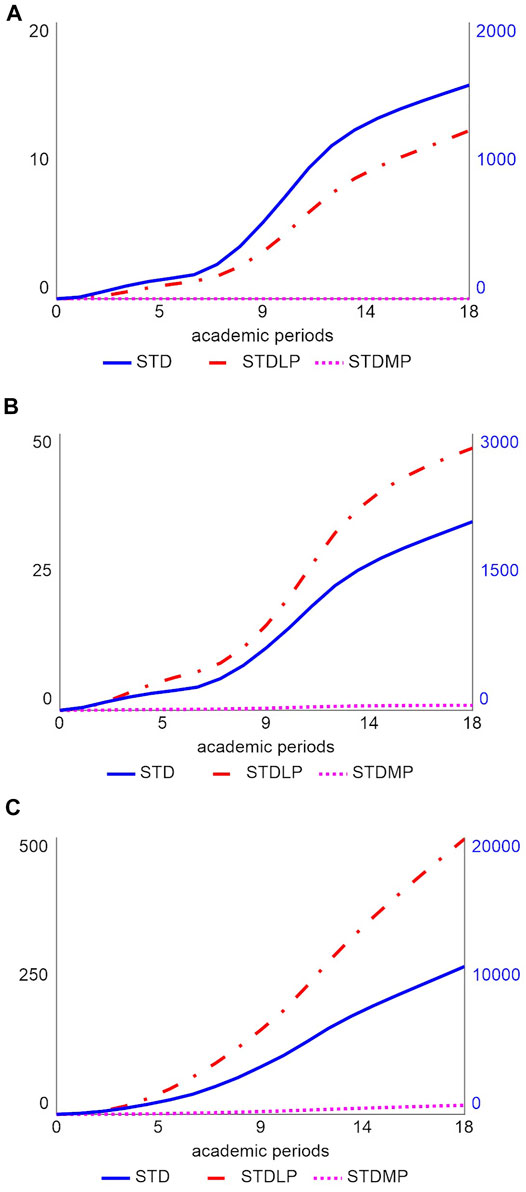
FIGURE 4. Drop-out behaviour by type of academic credit for SIM-1. Note: (A) presents technical training programmes, (B) technological training and (C) professional training. STD: Total number of dropouts per
Regarding the economic effects of drop-out for the actors in the education system, the simulation showed that for the five technical training programmes for the period 2019-2 (
In the case of the five technological training programmes, the drop-out costs for t = 9 were 1,280,000,000 Colombian pesos (USD 338,833.02), for the students and their families 1,600,000,000 pesos (USD 423,541.28), and for the state 128,000,000 Colombian pesos (USD 33,883.30). In turn, for this same type of programme for
For the ten professional training programmes, the drop-out costs for
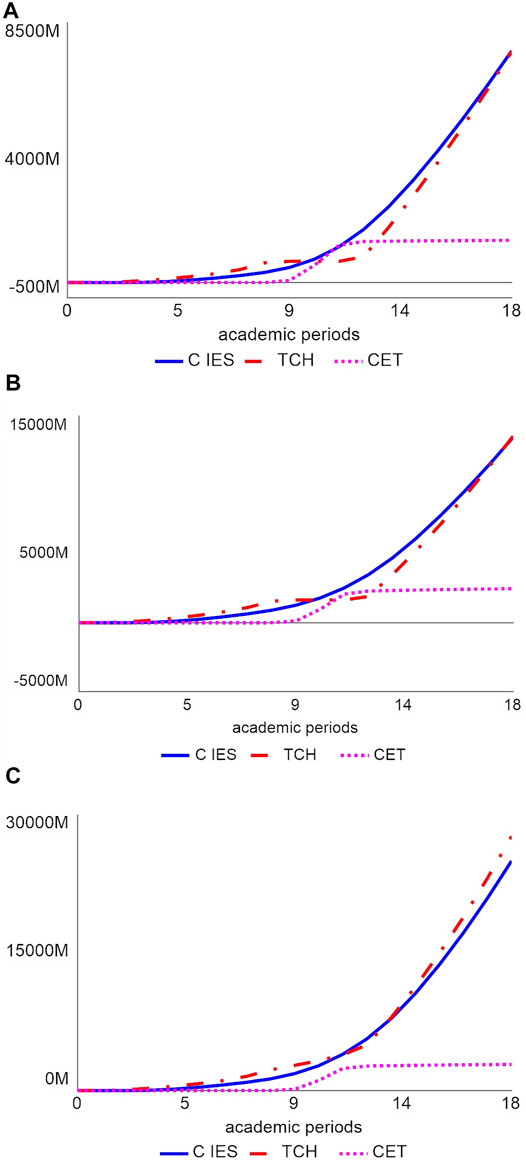
FIGURE 5. Behaviour of the economic effects of drop-out for SIM-1. Note: (A) presents technical training programmes, (B) technological training and (C) professional training. C IES: Total cost for the training programmes under study per
SIM-2 assessed the possible economic effects of drop-out in the absence of COVID-19 and changes in the state’s higher education access policies; firstly, it was determined that the number of drop-outs for the training programmes in the sample would increase, since in the absence of the pandemic there would not be a decrease in enrolments. Thus, for the five technical training programmes, the number of dropouts for
On the other hand, in the absence of tuition fee exemption policies in public HEIs, the cost of drop-out should be assumed by the student and his or her family. Given the above for
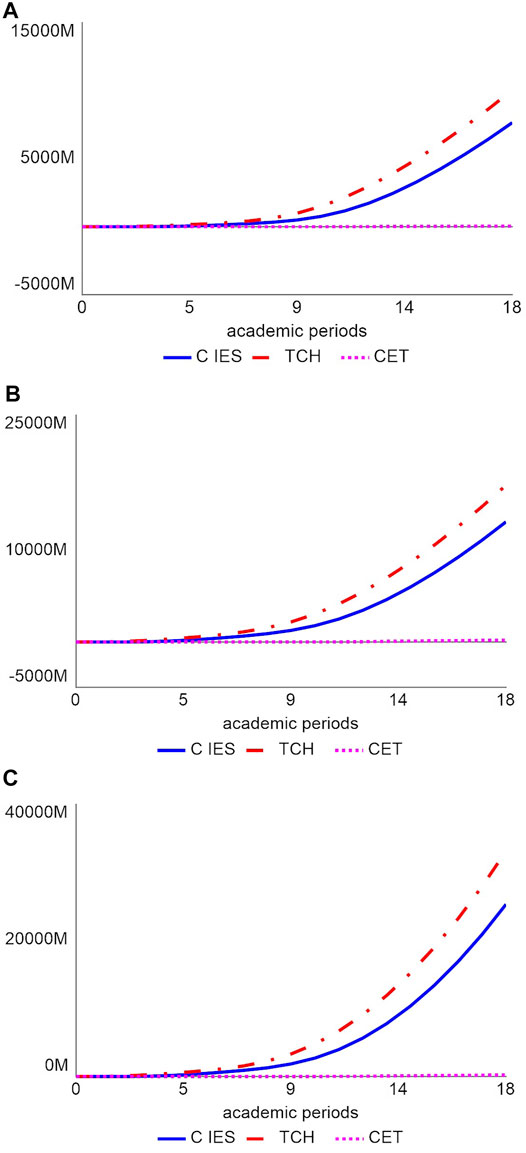
FIGURE 6. Behaviour of the economic effects of drop-out for SIM-2. Note: (A) presents technical training programmes, (B) technological training and (C) professional training. C IES: Total cost for the training programmes under study per
Finally, in relation to SIM-3, this sought to evaluate the economic effects of the new policies of access to higher education implemented in the framework of COVID-19, which for the Colombian case has included the financing of the academic periods of students in public universities by generating the exemption of tuition fees. Thus in this simulation for the periods from
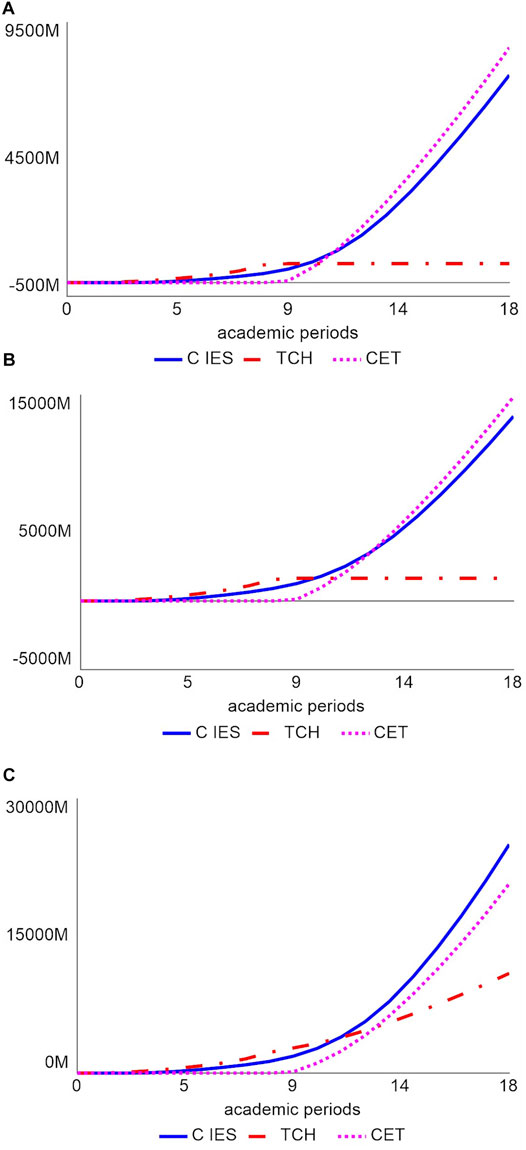
FIGURE 7. Behaviour of the economic effects of drop-out for SIM-3. Note: (A) presents technical training programmes, (B) technological training and (C) professional training. C IES: Total cost for the training programmes under study per
5 Discussion and Conclusion
As presented in the results section, the objective of this article was achieved, which was to model the economic effects of rural student drop-out at the higher education level for students and families, HEIs and the state, based on public policies for access to higher education, in the pandemic and post-pandemic scenario. However, it is necessary to recognise that the present study raises a new perspective on the phenomenon of drop-out and COVID-19, which had not been widely explored before this article, such as the economic effects for higher education actors, thus complementing previous studies that have analysed this relationship, such as those developed by Teuber et al. (2021), Cruz et al. (2020), Delnoij et al. (2021), Alyoussef (2021) and Cameron-Standerford et al. (2020).
The development of the model for the evaluation of the economic effects of drop-out was based on an integrative vision, distinguishing it from previous models that analysed these effects individually for each actor in the system, such as the works by World Bank, (2020), Bernal (2018), Barragán and Rodríguez (2015). On the other hand, this model makes it possible to link the consequences of COVID-19 on the rates of access to higher education, survival, drop-out and non-payment of educational credits. In addition to the above, the proposed model, based on System Dynamics, made it possible to understand and predict the economic effects of drop-out in terms of time t, brought about by the implementation, modification and elimination of public policies for access to higher education for the actors at this level of education. Although the article focused on rural areas, because of the intrinsic value of education for the development of these areas, especially in developing countries (Herbaut and Geven, 2020; Guzmán et al., 2021a; United Nations, 2021), the model is useful for any student population, HEI or state, as it seeks to represent the behaviour of the education level system.
With regard to the economic effects of drop-out in rural populations, it became evident that prior to the pandemic, in the case of Colombia, and more specifically of the training programmes in the sample, students and their families assumed a considerably high stranded cost, especially when, on average and according to UNDP statistics (2021), 50% of this population lived in monetary poverty and 27.9% in extreme poverty, with monthly incomes of less than 199,828 pesos (USD 53.17). However, during the pandemic and with the prospective scenarios after the pandemic, the financial relief achieved by public policies of temporary tuition fee waivers is insufficient, given that by 2024 the stranded cost for students and their families is estimated to be higher than the cost assumed by HEIs and the state. In the case of HEIs, COVID-19 has represented an exponential increase in opportunity cost in rural areas. Consequently, the pandemic has the singularity of directly affecting the development of the substantive functions of training programmes, due to the decrease in first-time enrolments and the increase in drop-out rates, which is in line with (Basilaia and Kvavadze, 2020). For the State, prior to the pandemic, the cost incurred was limited to non-payment of loans, however, the student population of the sampled programmes does not typically make use of educational loans so the non-payment rate may be high and not have a major impact on the credit-based student tuition funding programmes.
In the scenario in which COVID-19 had not occurred and affected the level of education in rural areas, the economic effects of drop-out would have intensified for students and their families, as well as for HEIs, because enrolment and its rates would have remained high for training programmes. Finally, for the simulation of the implementation of policies not based on educational credit but on tuition fees, the cost for the student and his or her family would be significantly reduced, as it would be assumed by the state.
Consequently, this article highlights the shortcomings of public policies on access to higher education for the rural student population, both in the pandemic and post-pandemic scenarios, as they do not have the capacity to mitigate the drop-out phenomenon, which contrasts with the results of (Mayer et al., 2015). For this reason, it is necessary to continue delving into the reasons why rural students drop-out, since socio-economic variables related to tuition fees do not fully explain the dropping out and high drop-out rates among this student population, and the policies developed by Western countries are insufficient to transmit the intrinsic and extrinsic benefits of the educational level, as stated by Guzmán et al (2021b).
Thus, the limitations of the study, such as the sample size of the training programmes and policies analysed, must be acknowledged. Finally, the academic community is invited to consider the model as a reference, to adjust and adapt it to learn about other economic effects on diverse student populations. On the other hand, decision-makers in the development of public policies for access, retention and timely graduation of higher education students in rural areas are urged to foresee the effects that the simulations presented with the proposed model will have on the different actors at the educational level.
Data Availability Statement
Publicly available datasets were analyzed in this study. This data can be found here: https://doi.org/10.6084/m9.figshare.16944235.v1.
Author Contributions
AGR and SBM contributed to conception and design of the study. AGR organized the database. AGR performed quantitative and qualitative analysis. AGR and SBM wrote the first draft of the manuscript. AGR, SBM, and FC-V reviewed and edited. SBM and FC-V supervised both the development of the research and the manuscript. All authors contributed to manuscript revision, read, and approved the submitted version.
Conflict of Interest
The authors declare that the research was conducted in the absence of any commercial or financial relationships that could be construed as a potential conflict of interest.
Publisher’s Note
All claims expressed in this article are solely those of the authors and do not necessarily represent those of their affiliated organizations, or those of the publisher, the editors and the reviewers. Any product that may be evaluated in this article, or claim that may be made by its manufacturer, is not guaranteed or endorsed by the publisher.
Acknowledgments
The University Corporation of Asturias, whose support covered the cost of the publication, and to Cecilia Carabajal who, with her unconditional support, made the style correction and translation of this article.
Supplementary Material
The Supplementary Material for this article can be found online at: https://www.frontiersin.org/articles/10.3389/feduc.2021.812114/full#supplementary-material
References
Abbasi, S., Ayoob, T., Malik, A., and Memon, S. I. (2020). Perceptions of Students Regarding E-Learning during Covid-19 at a Private Medical College. Pak J. Med. Sci. 36, S57. doi:10.12669/pjms.36.COVID19-S4.2766
Adrogue, C., and García de Fanelli, A. M. (2018). Gaps in Persistence under Open-Access and Tuition-free Public Higher Education Policies. epaa 26, 126. doi:10.14507/epaa.26.3497
Agronet Report (2020). Trabajadores rurales no han escapado de la crisis por la COVID-19. Available at: https://www.agronet.gov.co/Noticias/Paginas/Trabajadores-rurales-no-han-escapado-de-la-crisis-por-la-COVID-19.aspx (Accessed December 1, 2021).
Alyoussef, I. (2021). E-learning System Use during Emergency: An Empirical Study during the COVID-19 Pandemic. Front. Educ. 6, 677753. doi:10.3389/feduc.2021.677753
Améstica-Rivas, L., King-Domínguez, A., Sanhueza Gutiérrez, D. A., and Ramírez González, V. (2020). Efectos económicos de la deserción en la gestión universitaria: el caso de una universidad pública chilena. Hallazgos 18. doi:10.15332/2422409X.5772
Arias Ortiz, E., and Dehon, C. (2013). Roads to Success in the Belgian French Community's Higher Education System: Predictors of Dropout and Degree Completion at the Université Libre de Bruxelles. Res. High Educ. 54, 693–723. doi:10.1007/s11162-013-9290-y
Arias-Velandia, N., Rincón-Báez, W. U., and Cruz-Pulido, J. M. (2018). Desempeño De Mujeres Y Hombres En Educación Superior Presencial, Virtual Y A Distancia En Colombia - Women and Men Performance in Face-To-Face, Virtual and Distance Higher Education in Colombia. pnrm 12, 57–69. doi:10.15765/pnrm.v12i22.1142
Armstrong, S. N., Early, J. O., Burcin, M. M., Bolin, K., Holland, N., and No, S. (2018). New Media Tools Impact on Online, Health Science Students' Academic Persistence and Support: Lessons Learned from Two Pilot Studies. TechTrends 62, 266–275. doi:10.1007/s11528-018-0261-1
Atchoarena, D., Holmes, K., Atchoarena, D., and Holmes, K. (2005). The Role of Agricultural Colleges and Universities in Rural Development and Lifelong Learning in Asia. Asian J. Agric. Dev. 2, 15–24. doi:10.22004/AG.ECON.165777
Bala, B. K., Arshad, F. M., and Noh, K. M. (2017). System Dynamics. Singapore: Springer Singapore. doi:10.1007/978-981-10-2045-2
Barragán Moreno, S. P., and González Támara, L. (2017). Acercamiento a la deserción estudiantil desde la integración social y académica. Revista de la Educación Superior 46, 63–86. doi:10.1016/j.resu.2017.05.004
Barragán, S., and Rodríguez, R. (2015). Diagnóstico y seguimiento de la deserción en la Universidad de Bogotá Jorge Tadeo Lozano. 1st ed. Bogotá: Universidad Jorge Tadeo Lozano.
Basilaia, G., and Kvavadze, D. (2020). Transition to Online Education in Schools during a SARS-CoV-2 Coronavirus (COVID-19) Pandemic in Georgia. PEDAGOGICAL RES. 5. doi:10.29333/pr/7937
Becerra, M., Alonso, J. D., Frias, M., Angel-Urdinola, D., and Vergara, S. (2020). Latin America and the Caribbean: Tertiary Education. Available at: https://documents1.worldbank.org/curated/en/720271590700883381/COVID-19-Impact-on-Tertiary-Education-in-Latin-America-and-the-Caribbean.pdf.
Becker, G. S. (1962). Investment in Human Capital: A Theoretical Analysis. J. Polit. Economy 70, 9–49. Available at: https://www.jstor.org/stable/1829103 (Accessed August 29, 2021). doi:10.1086/258724
Behr, A., Giese, M., Teguim Kamdjou, H. D., and Theune, K. (2020). Dropping Out of university: a Literature Review. Rev. Educ. 8, 614–652. doi:10.1002/rev3.3202
Bernal, O. O. B. (2018). Evaluación financiera ocasionada por la deserción estudiantil en la Universidad Libre Seccional Cúcuta. interfaces 1. Available at: https://revistas.unilibre.edu.co/index.php/interfaces/article/view/3676 (Accessed August 27, 2021).
Bettinger, E. (2015). Need-Based Aid and College Persistence. Educ. Eval. Pol. Anal. 37, 102S–119S. doi:10.3102/0162373715576072
Cameron-Standerford, A., Menard, K., Edge, C., Bergh, B., Shayter, A., Smith, K., et al. (2020). The Phenomenon of Moving to Online/Distance Delivery as a Result of COVID-19: Exploring Initial Perceptions of Higher Education Faculty at a Rural Midwestern University. Front. Educ. 5, 583881. doi:10.3389/feduc.2020.583881
Castleman, B. L., and Meyer, K. E. (2020). Can Text Message Nudges Improve Academic Outcomes in College? Evidence from a West Virginia Initiative. Rev. Higher Educ. 43, 1125–1165. doi:10.1353/rhe.2020.0015
Chatterji, P., and Li, Y. (2021). Effects of COVID-19 on School Enrollment. Econ. Educ. Rev. 83, 102128. doi:10.1016/j.econedurev.2021.102128
Chen, R., and DesJardins, S. L. (2008). Exploring the Effects of Financial Aid on the Gap in Student Dropout Risks by Income Level. Res. High Educ. 49, 1–18. doi:10.1007/s11162-007-9060-9
Chetty, R., Friedman, J. N., Hendren, N., Stepner, M., and Team, T. O. I. (2020). The Economic Impacts of COVID-19: Evidence from a New Public Database Built Using Private Sector Data. Massachusetts: National Bureau of Economic Research. doi:10.3386/w27431
Choi, H. J., and Kim, B. U. (2018). Factors Affecting Adult Student Dropout Rates in the Korean Cyber-University Degree Programs. J. Contin. Higher Educ. 66, 1–12. doi:10.1080/07377363.2017.1400357
Choudhary, L. A., and Hammayun, A. (2015). Economic Effects of Student Dropouts: A Comparative Study. J. Glob. Econ. 3, 1–4. doi:10.4172/2375-4389.1000137
Contreras, C. (2018). Rendimiento académico de los alumnos de último año de Licenciaturas presenciales e Ingeniería de la Facultad Multidisciplinaria de Ilobasco durante el ciclo I - 2017. Anuario de Investigación 7, 125–139. Available at: http://localhost:80/xmlui/handle/123456789/217 (Accessed March 28, 2021).
Cook, J., Burke, P. J., Bunn, M., and Cuervo, H. (2021). Should I Stay or Should I Go? the Impact of the COVID-19 Pandemic on Regional, Rural and Remote Undergraduate Students at an Australian University. Educ. Rev., 1–15. doi:10.1080/00131911.2021.1958756
Cristia, J., and Pulido, J. (2020). “Education in Latin America and the Caribbean: Segregated and Unequal,” in The Inequality Crisis: Latin America and the Caribbean at the Crossroad. Editor M. Busso (Messina, Washington D.C.), 166–193.
Cruz, M. F., Rodríguez, J. Á., Ruiz, I. Á., López, M. C., Camargo, C. d. B., Rosas, F. D., et al. (2020). Evaluation of the Emotional and Cognitive Regulation of Young People in a Lockdown Situation Due to the Covid-19 Pandemic. Front. Psychol. 11, 565503. doi:10.3389/fpsyg.2020.565503
De Clercq, M., Galand, B., and Frenay, M. (2017). Transition from High School to university: a Person-Centered Approach to Academic Achievement. Eur. J. Psychol. Educ. 32, 39–59. doi:10.1007/s10212-016-0298-5
De Hart, K., and Venter, J. M. P. (2013). Comparison of Urban and Rural Dropout Rates of Distance Students. Perspect. Educ. 31, 66–76. Available at: https://www.ajol.info/index.php/pie/article/view/87989 (Accessed May 28, 2021).
Delnoij, L., Janssen, J., Dirkx, K., Gijselaers, H., de Groot, R. H., Neroni, J., et al. (2021). Predicting Completion: The Road to Informed Study Decisions in Higher Online Education. Front. Educ. 6, 668922. doi:10.3389/feduc.2021.668922
Denning, J. T. (2017). College on the Cheap: Consequences of Community College Tuition Reductions. Am. Econ. J. Econ. Pol. 9, 155–188. doi:10.1257/pol.20150374
Dennis, M. J. (2020). The Impact of COVID ‐19 on the World Economy and Higher Education. Enrollment Manage. Rep. 24, 3. doi:10.1002/emt.30720
Didenko, D. V. (2015). Intellektualoemkaya Ekonomika: Chelovecheskiy Kapital v Rossiyskom I Mirovom Socialno-Ekonomicheskom Razvitii. Saint Petersburg: Alateya.
Erola, J., Jalonen, S., and Lehti, H. (2016). Parental Education, Class and Income over Early Life Course and Children's Achievement. Res. Soc. Stratification Mobility 44, 33–43. doi:10.1016/j.rssm.2016.01.003
Esteban, M., Bernardo, A., Tuero, E., Cervero, A., and Casanova, J. (2017). Variables influyentes en progreso académico y permanencia en la universidad. Eur. J. Educ. Psychol. 10, 75–81. doi:10.1016/j.ejeps.2017.07.003
Expósito, C. D., and Marsollier, R. G. (2020). Virtualidad y educación en tiempos de COVID-19. Un estudio empírico en Argentina. educ.humanismo 22. doi:10.17081/eduhum.22.39.4214
Fack, G., and Grenet, J. (2015). Improving College Access and Success for Low-Income Students: Evidence from a Large Need-Based Grant Program. Am. Econ. J. Appl. Econ. 7, 1–34. doi:10.1257/app.20130423
Favale, T., Soro, F., Trevisan, M., Drago, I., and Mellia, M. (2020). Campus Traffic and E-Learning during COVID-19 Pandemic. Computer Networks 176, 107290. doi:10.1016/j.comnet.2020.107290
Gruzina, Y., Firsova, I., and Strielkowski, W. (2021). Dynamics of Human Capital Development in Economic Development Cycles. Economies 9, 67. doi:10.3390/economies9020067
Guzmán, A., Barragán, S., and Cala Vitery, F. (2021b). Dropout in Rural Higher Education: A Systematic Review. Front. Educ. 6, 727833. doi:10.3389/feduc.2021.727833
Guzmán, A., Barragán, S., and Cala-Vitery, F. (2021d). Forrester Diagram Level of Professional Education. doi:10.6084/m9.figshare.16944235.v1
Guzmán, A., Barragán, S., and Cala-Vitery, F. (2021c). Forrester Diagram Level of Technical Education. doi:10.6084/M9.FIGSHARE.16944244.V1
Guzmán, A., Valencia, L. I., Moreno, W., and Segovia, N. (2020). “Factores pre-matricula asociados a la deserción en educación superior en modalidad virtual: una revisión sistematizada,” in Inclusión, Tecnología y Sociedad: investigación e innovación en educación (Madrid: Dykinson), 784–794.
Guzmán Rincón, A., Barragán, S., and Cala Vitery, F. (2021a). Rurality and Dropout in Virtual Higher Education Programmes in Colombia. Sustainability 13, 4953. doi:10.3390/su13094953
Hällsten, M. (2017). Is Education a Risky Investment? the Scarring Effect of University Dropout in Sweden. Eur. Sociol. Rev. 33 (2), 169–181. doi:10.1093/esr/jcw053
Hanushek, E. A., and Woessmann, L. (2020). The Economic Impacts of Learning Losses. OECD Educ. Working Pap. 225, 1–24. doi:10.1787/19939019
Heidrich, L., Victória Barbosa, J. L., Cambruzzi, W., Rigo, S. J., Martins, M. G., and dos Santos, R. B. S. (2018). Diagnosis of Learner Dropout Based on Learning Styles for Online Distance Learning. Telematics Inform. 35, 1593–1606. doi:10.1016/j.tele.2018.04.007
Herbaut, E., and Geven, K. (2020). What Works to Reduce Inequalities in Higher Education? A Systematic Review of the (Quasi-)experimental Literature on Outreach and Financial Aid. Res. Soc. Stratification Mobility 65, 100442. doi:10.1016/j.rssm.2019.100442
ICETEX (2021). Crédito educativo más colombiano que nunca. Instituto Colombiano de Crédito Educativo y Estudios Técnicos en el Exterior. Available at: https://portal.icetex.gov.co/mascolombianoquenunca/index.html (Accessed February 1, 2021).
Kehm, B. M., Larsen, M. R., and Sommersel, H. B. (2019). Student Dropout from Universities in Europe: A Review of Empirical Literature. HERJ 9, 147–164. doi:10.1556/063.9.2019.1.18
Kerres, M. (2020). Against All Odds: Education in Germany Coping with Covid-19. Postdigit Sci. Educ. 2, 690–694. doi:10.1007/s42438-020-00130-7
Laaser, W. (2018). El impacto económico y las posturas de los actores principales en un ámbito universitario digitalizado. Red. Available at: https://revistas.um.es/red/article/view/327361 (Accessed August 29, 2021).
Larroucau, T. (2016). Estudio de los factores determinantes de la deserción en el sistema universitario chileno. Revista Estudios de Políticas Públicas 1, 1–23. doi:10.5354/0719-6296.2015.38351
Lewine, R., Manley, K., Bailey, G., Warnecke, A., Davis, D., and Sommers, A. (2019). College Success Among Students from Disadvantaged Backgrounds: “Poor” and “Rural” Do Not Spell Failure. J. Coll. Student Retention: Res. Theor. Pract. 23, 686–698. doi:10.1177/1521025119868438
López-Aguilar, D., and Álvarez-Pérez, P. R. (2021). Modelo predictivo PLS-SEM sobre intención de abandono académico universitario durante la COVID-19. Rev. Complut. Educ. 32, 451–461. doi:10.5209/rced.70507
Mailizar, M., Almanthari, A., Maulina, S., and Bruce, S. (2020). Secondary School Mathematics Teachers' Views on E-Learning Implementation Barriers during the COVID-19 Pandemic: The Case of Indonesia. EURASIA J. Math. Sci. Tech. Ed. 16, em1860. doi:10.29333/ejmste/8240
Marginson, S. (2011). Equity, Status and freedom: a Note on Higher Education. Cambridge J. Educ. 41, 23–36. doi:10.1080/0305764X.2010.549456
Marginson, S. (2019). Limitations of Human Capital Theory. Stud. Higher Educ. 44, 287–301. doi:10.1080/03075079.2017.1359823
Marginson, S. (2016). The Worldwide Trend to High Participation Higher Education: Dynamics of Social Stratification in Inclusive Systems. High Educ. 72, 413–434. doi:10.1007/s10734-016-0016-x
Mayer, A., Patel, R., and Gutierrez, M. (2015). Four-Year Effects on Degree Receipt and Employment Outcomes from a Performance-Based Scholarship Program in Ohio. SSRN J., 1–34. doi:10.2139/ssrn.2594482
McCowan, T. (2016). Three Dimensions of Equity of Access to Higher Education. Compare: A J. Comp. Int. Educ. 46, 645–665. doi:10.1080/03057925.2015.1043237
McMahon, W. W. (2010). “The External Benefits of Education,” in International Encyclopedia of Education (Elsevier), 260–271. doi:10.1016/B978-0-08-044894-7.01226-4
Ministry of National Education (2009). Deserción estudiantil en la educación superior colombiana: Metodología de seguimiento, diagnóstico y elementos para su prevención. 1st ed. Bogotá: Colombia: Ministry of National Education.
Mncube, V., Mutongoza, B. H., and Olawale, E. (2021). Managing Higher Education Institutions in the Context of Covid-19 Stringency: Experiences of Stakeholders at a Rural South African university. PiE 39. doi:10.18820/2519593X/pie.v39.i1.24
Moreno, W., Segovia, N., Grillo, C., Dworaczek, H. O., and Coy, H. V. (2019). “Naturaleza del endeudamiento como base de la propuesta de política pública para la educación superior en Colombia desde 2013,” in Innovación Docente e Investigación en Ciencias Sociales, Económicas y Jurídicas (Madrid: Dykinson), 25–36.
Muñoz, S. M. (2013). "I Just Can't Stand Being like This Anymore": Dilemmas, Stressors, and Motivators for Undocumented Mexican Women in Higher Education. J. Student Aff. Res. Pract. 50, 233–249. doi:10.1515/jsarp-2013-0018
Nizar, N. I., and Nazir, A. (2020). Faktor Human Capital Pada Pertumbuhan Ekonomi Kreatif. JM 4, 52–65. doi:10.33753/mandiri.v4i1.103
Özdoğan Özbal, E. (2021). Dynamic Effects of Higher Education Expenditures on Human Capital and Economic Growth: an Evaluation of OECD Countries. Pol. Rev. Higher Educ. 5, 174–196. doi:10.1080/23322969.2021.1893125
Palacio Sprockel, L. E., Vargas Babilonia, J. D., and Monroy Toro, S. L. (2020). Análisis bibliométrico de estudios sobre factores socioeconómicos en estudiantes universitarios. Educ. Educ. 23, 355–375. doi:10.5294/edu.2020.23.3.1
Pokhrel, S., and Chhetri, R. (2021). A Literature Review on Impact of COVID-19 Pandemic on Teaching and Learning. Higher Educ. Future 8, 133–141. doi:10.1177/2347631120983481
Potra, S., Pugna, A., Pop, M. D., Negrea, R., and Dungan, L. (2021). Facing COVID-19 Challenges: 1st-Year Students' Experience with the Romanian Hybrid Higher Educational System. Int. J. Environ. Res. Public Health 18, 3058. doi:10.3390/ijerph18063058
Proyecto ALFA GUIA DCI-ALA/2010/94 (2013). Estudio sobre Políticas Nacionales sobre el abandono en la Educación Superior en los países que participan en el Proyecto ALFA-GUIA. Madrid: Gestión Universitaria Integral del Abandono.
Richburg-Hayes, L., Patel, R., Brock, T., de la Campa, E., Rubb, T., and Valenzuela, I. (2015). Providing More Cash for College: Interim Findings from the Performance-Based Scholarship Demonstration in California. MDRC Available at: https://eric.ed.gov/?id=ED560254 (Accessed August 24, 2021).
Rodríguez-Hernández, C. F., Cascallar, E., and Kyndt, E. (2020). Socio-economic Status and Academic Performance in Higher Education: A Systematic Review. Educ. Res. Rev. 29, 100305. doi:10.1016/j.edurev.2019.100305
Rong Chen, R., and Stephen L. DesJardins, S. L. (2010). Investigating the Impact of Financial Aid on Student Dropout Risks: Racial and Ethnic Differences. J. Higher Educ. 81, 179–208. doi:10.1080/00221546.2010.1177904810.1353/jhe.0.0085
Rubin, R. B. (2011). The Pell and the Poor: A Regression-Discontinuity Analysis of On-Time College Enrollment. Res. High Educ. 52, 675–692. doi:10.1007/s11162-011-9215-6
Rueda Ramírez, S. M., Urrego Velásquez, D., Páez Zapata, E., Velásquez, C., and Hernández Ramírez, E. M. (2020). Perfiles de riesgo de deserción en estudiantes de las sedes de una universidad colombiana. Psico 38, 275–297. doi:10.18800/psico.202001.011
Sahoo, K. K., Muduli, K. K., Luhach, A. K., and Poonia, R. C. (2021). Pandemic COVID-19: An Empirical Analysis of Impact on Indian Higher Education System. J. Stat. Manage. Syst. 24, 341–355. doi:10.1080/09720510.2021.1875571
Schmitt, J., Fini, M. I., Bailer, C., Fritsch, R., and de Andrade, D. F. (2020). WWH-Dropout Scale: When, Why and How to Measure Propensity to Drop Out of Undergraduate Courses. J. Appl. Res. Higher Educ.. doi:10.1108/JARHE-01-2020-0019
Sobaih, A. E. E., Hasanein, A. M., and Abu Elnasr, A. E. (2020). Responses to COVID-19 in Higher Education: Social Media Usage for Sustaining Formal Academic Communication in Developing Countries. Sustainability 12, 6520. doi:10.3390/su12166520
Sosu, E. M., and Pheunpha, P. (2019). Trajectory of University Dropout: Investigating the Cumulative Effect of Academic Vulnerability and Proximity to Family Support. Front. Educ. 4, 6. doi:10.3389/feduc.2019.00006
Suhendra, I. (2020). Determinan Modal Manusia Di Indonesia: Menggunakan Estimasi Panel. Jurnal Riset Bisnis dan Manajemen Tirtayasa 4, 162–177. doi:10.48181/jrbmt.v4i2.1049010.48181/jrbmt.v4i1.9611
Teuber, Z., Jia, H., and Niewöhner, T. (2021). Satisfying Students' Psychological Needs during the COVID-19 Outbreak in German Higher Education Institutions. Front. Educ. 6, 679695. doi:10.3389/feduc.2021.679695
United Nations (2021). Reducing Poverty and Inequality in Rural Areas: Key to Inclusive Development. New York: United Nations.
Voelkle, M. C., and Sander, N. (2008). University Dropout. J. Individual Differences 29, 134–147. doi:10.1027/1614-0001.29.3.134
Wang, C. J., Ng, C. Y., and Brook, R. H. (2020). Response to COVID-19 in Taiwan: Big Data Analytics, New Technology, and Proactive Testing. JAMA 323, 1341–1342. doi:10.1001/jama.2020.3151
World Bank (2020). The Impact of the COVID-19 Pandemic on Education Financing. Available at: https://documents.worldbank.org/en/publication/documents-reports/documentdetail/479041589318526060/The-Impact-of-the-COVID-19-Pandemic-on-Education-Financing (Accessed August 24, 2021).
Yunhua Qu, Y. (2009). “Research and Application of Diversified Model in Yardstick of Higher Education Tuition,” in 2009 International Conference on Test and Measurement, 319–322. doi:10.1109/ICTM.2009.5413043
Keywords: drop-out, COVID-19, rurality, model, economic effects, higher education
Citation: Guzmán Rincón A, Barragán Moreno S and Cala-Vitery F (2021) Rural Population and COVID-19: A Model for Assessing the Economic Effects of Drop-Out in Higher Education. Front. Educ. 6:812114. doi: 10.3389/feduc.2021.812114
Received: 09 November 2021; Accepted: 22 November 2021;
Published: 14 December 2021.
Edited by:
Adrian Castro-Lopez, University of Oviedo, SpainReviewed by:
Ishwanzya Rivers, University of Louisville, United StatesKatarzyna Rostek, Warsaw University of Technology, Poland
Copyright © 2021 Guzmán Rincón, Barragán Moreno and Cala-Vitery. This is an open-access article distributed under the terms of the Creative Commons Attribution License (CC BY). The use, distribution or reproduction in other forums is permitted, provided the original author(s) and the copyright owner(s) are credited and that the original publication in this journal is cited, in accordance with accepted academic practice. No use, distribution or reproduction is permitted which does not comply with these terms.
*Correspondence: Alfredo Guzmán Rincón, YWxmcmVkby5ndXptYW5AYXN0dXJpYXMuZWR1LmNv