- 1Tilburg University, Tilburg, Netherlands
- 2Cito, Arnhem, Netherlands
- 3International Baccalaureate, Cardiff, United Kingdom
Comparative judgment is a method that allows measurement of a competence by comparison of items with other items. In educational measurement, where comparative judgment is becoming an increasingly popular assessment method, items are mostly students’ responses to an assignment or an examination. For assessments using comparative judgment, the Scale Separation Reliability (SSR) is used to estimate the reliability of the measurement. Previous research has shown that the SSR may overestimate reliability when the pairs to be compared are selected with certain adaptive algorithms, when raters use different underlying models/truths, or when the true variance of the item parameters is below one. This research investigated bias and stability of the components of the SSR in relation to the number of comparisons per item to increase understanding of the SSR. We showed that many comparisons are required to obtain an accurate estimate of the item variance, but that the SSR can be useful even when the variance of the items is overestimated. Lastly, we recommend adjusting the general guideline for the required number of comparisons per item to 41 comparisons per item. This recommendation partly depends on the number of items and the true variance in our simulation study and needs further investigation.
Introduction
Comparative judgment is a method that allows measurement of a competence by comparison of items. When items are compared in pairs, comparative judgment is also known as pairwise comparison. This method has been used in different contexts ranging from sports to marketing to educational assessment, with different models for each context (e.g., Agresti, 1992; Böckenholt, 2001; Maydeu-Olivares, 2002; Maydeu-Olivares and Böckenholt, 2005; Böckenholt 2006; Stark and Chernyshenko, 2011; Cattelan, 2012; Brinkhuis, 2014). In educational measurement, where comparative judgment is becoming an increasingly popular assessment method (Lesterhuis et al., 2017; Bramley and Vitello, 2018), items are mostly students’ responses to an assignment or an examination. The assignment or the examination is used to measure a competence of the students, and the students’ responses give an indication of their competence level. The method has been used in a variety of contexts, ranging from art assignments (Newhouse, 2014) to academic writing (Van Daal et al., 2016) and mathematical problem solving (Jones & Alcock, 2013). These contexts have in common that the competencies are difficult to disentangle into sub-aspects together defining the competencies. Therefore, they are difficult to measure validly using analytical scoring schemes such as rubrics or criteria lists (Van Daal et al., 2016), which are conventional measurement methods used in education. In contrast to these analytic measurement methods, which assume that a competence can be operationalized by means of a list of sub-aspects and evaluate each aspect separately, comparative judgment is a holistic measurement method where a competence is evaluated as a whole (Pollitt, 2012); simply asking which of two items scores higher on the competence of interest suffices.
For complex competencies like art assignments, academic writing, and mathematical problem solving, it is possible that a higher validity can be obtained using comparative judgment instead of rubrics or criteria lists (Pollitt, 2012; Van Daal et al., 2016) because of its holistic character and the greater possibility of raters to use their expertise in their judgments compared to rubrics or criteria lists. In addition to the claim of higher validity of comparative judgment, Pollitt (2012) claimed that comparative judgment also results in higher reliability compared to using rubrics or criteria lists. However, later research has shown that this claim is likely to be too optimistic for the reported numbers of comparisons per item (e.g., Bramley, 2015; Bramley and Vitello, 2018; Crompvoets et al., 2020; Crompvoets et al., 2021), and that the extent to which high reliability that can be obtained using comparative judgment is limited (Verhavert et al., 2019).
To explain why Pollitt’s (2012) claim is too optimistic, we first define two types of reliability in the context of comparative judgment: the benchmark reliability (Crompvoets et al., 2020, 2021) and the Scale Separation Reliability (SSR; e.g., Bramley, 2015; Crompvoets et al., 2020). Both forms of reliability are based on parameters of the Bradley-Terry-Luce (BTL; Bradley and Terry, 1952; Luce, 1959) model. This model is defined as follows. Let
We interpret
The benchmark reliability is only known in simulated data and is computed as the squared correlation between the true (simulated) item parameters and the item parameter estimates. Let
This definition of reliability corresponds with the definition of reliability as
The SSR is an estimate of reliability that is based on the Index of Subject Separation formulated by Andrich and Douglas (1977, as cited in Gustafsson, 1977) and is computed as follows. We assume that items are compared in pairs and that the location parameters of these items on the latent competence scale are of interest. Let
The SSR can then be written as
where
that is, the observed variance minus an error term (Bramley, 2015).
Research (Bramley, 2015; Bramley and Vitello, 2018; Crompvoets et al., 2020) has shown that the SSR might overestimate reliability (Eq. 2) in certain situations. These include the use of certain adaptive algorithms to select the pairs that raters have to compare. Pollitt’s (2012) claim that comparative judgment results in higher reliability than using rubrics or criteria lists is based on a study using an adaptive algorithm to select the pairs that are compared in combination with the SSR. Other situations in which the SSR may overestimate benchmark reliability are when raters behave inconsistent amongst each other, which would be reflected in the BTL model by different parameters for the same items, and perhaps when the true variance of the item parameters is below 1 as well (Crompvoets et al., 2021). The result that the SSR may overestimate reliability suggests why Pollitt’s (2012) claim that comparative judgment results in higher reliability is likely too optimistic. Moreover, the result that the SSR may overestimate reliability is problematic because 1) reliability estimates should provide a lower bound to reliability to avoid reporting reliability that is too high and therefore promises too much (Sijtsma, 2009; Hunt and Bentler, 2015) and 2) most recommendations about the number of required comparisons are based on achieving at least a user-defined value of the SSR (e.g., Verhavert et al., 2019).
To the best of our knowledge, no one has thoroughly investigated and reported the positive bias of the SSR. Previous research that reported the bias of the SSR has stopped at the conclusion that the SSR was biased (Bramley, 2015; Bramley and Vitello, 2018) or has only led to speculations about the meaning of the bias due to either adaptive pair selection (Crompvoets et al., 2020), different rater probabilities, or small true variances (Crompvoets et al., 2021).
One might reason that the behavior of the SSR needs no investigation, because its value can easily be derived from the two components
Because all quantities needed to estimate the SSR (Eq. 3) are based on the parameter estimates
The goal of this study was to gain insight into the bias and stability of the parameter estimates and the SSR of comparative judgment in educational measurement from two perspectives. In addition, we aimed to use this information either to support the guideline about the number of required comparisons per item from Verhavert et al. (2019) or to provide a new guideline based on the results from this study. First, we adapted the guideline for the required number of observations to obtain stable results for the one-parameter item response model or Rasch model (Rasch, 1960) for regular multiple choice tests to the BTL model used for comparative judgment. Second, we investigated the bias and stability of the parameter estimates and SSR of comparative judgment in a simulation study. In the discussion, we will reflect on the two perspectives.
Sample Size Guideline Adaptation to the Bradley-Terry-Luce Model
To determine the required number of observations to obtain stable model parameters, most researchers and test institutions use experience as their guide. One reason for this may be that the literature about sample size requirements to obtain stable model parameters is sparse and seems limited to conference presentations (Parshall et al., 1998), articles that were not subjected to peer review (Linacre, 1994), a framework used to assess test quality written in a non-universal language (Evers et al., 2009), or a brief mention in a book (Wright and Stone, 1979, p. 136). Parshall et al. (1998) and Evers et al. (2009) describe the guideline that for the one-parameter item response model, at least 200 observations per item are required to obtain stable item location parameter estimates. Wright and Stone (1979) suggest using 200 observations for test linking using the Rasch model, although they, and Linacre (1994), also mention that fewer observations may be sufficient to obtain sufficiently stable parameter estimates for some purposes. When the model parameters are considered sufficiently stable depends on the context. Because we encountered the guideline of 200 observations per item for several purposes and it is used often in practice, we used this guideline as a starting point.
The literature about guidelines for the Rasch model may be sparse, but for the mathematically related (Andrich, 1978) BTL model, no guidelines exist that describe how many observations are required in educational measurement for obtaining stable item parameter estimates. In this section, we first describe how the Rasch model and the BTL model are related, and then adapt the guideline from the Rasch model to the BTL model. In the Discussion section, we will evaluate this guideline in relation to the outcomes of the simulation study from the next section and in relation to the literature.
The Rasch model is defined as follows. Let
We note that although mathematically it would have made sense to use
Even though the Rasch model and the BTL model have different parametrization (Verhavert et al., 2018), Andrich (1978) showed that the equations for the Rasch model and the BTL model are equivalent. This means that a person-item comparison in the Rasch model is mathematically equivalent to an inter-item comparison in the BTL model. Therefore, it makes sense to adapt the guideline for the Rasch model about the required number of observations for stable model estimates to the BTL model.
Our starting point for the guideline adaptation is the item, since items are present in both the Rasch model and the BTL model. In addition, the guideline Parshall et al. (1998) suggested aims at obtaining stable item parameter estimates. We assume that the number of items in the test that the Rasch model analyzes is the same as the number of items in the set of paired comparisons that is analyzed by means of the BTL model. However, the manner in which we obtain additional observations for an item differs between the models. Each observation for an item in the Rasch model is obtained from a person belonging to a population with many possible parameter values, whereas each observation for an item in the BTL model is obtained from an item in the fixed set of items under investigation. Therefore, for the BTL model, the information obtained from one observation may depend on the item parameters in the set, which is different for the Rasch model, where the information also depends on the sample of persons.
There are two ways to adapt the guideline from the Rasch model for use with the BTL model. The first adaptation is to equate the number of required observations per item for the BTL to the required number for the Rasch model; that is, 200 observations per item (Guideline 1). Since each comparative judgment/observation for the BTL model contains information about two items, this adaptation means that compared to the Rasch model, we need half of the total number of observations. We illustrate this with an example. Suppose we have 20 items in both models. The guideline of 200 observations per item for the Rasch model means that we need
The second possibility is to equate the total number of observations for a set of items instead of the number of observations of one item. Continuing the example from the previous paragraph, 4,000 observations are required for a set of 20 items for the Rasch model to obtain stable item parameter estimates using Parshall et al.’s (1998) guideline. Adapted to the BTL model following the second guideline (i.e., equating the total number of observations for a set of items), this would mean that 4,000 paired comparisons in total are required to get stable item parameter estimates, which would mean
For the BTL model, the limited number of unique comparisons implies that the number of items in the set influences which numbers are compared, even though the number of observations per item does not change for different numbers of items. The number of items in the set is nonlinearly related to the number of unique comparisons in a comparative judgment setting. This means that the number of times each unique comparison is made differs for different numbers of comparisons. Table 1 illustrates this: using guideline 2, for 20 items, all unique comparisons should be made 21 times (on average). On the other hand, for 1,000 items, all unique comparisons should be made 0.4 times, which means that not even all unique comparisons are made.

TABLE 1. Total number of observations and number of complete designs according to the translated guideline for the BTL model for different numbers of items.
Bias and Stability of Scale Separation Reliability Components
We investigated in a simulation study: 1) How many comparisons are required to obtain a stable and unbiased variance of the parameter estimates,
Methods
Simulation Set-Up
The simulation design had two factors. First, we varied the number of items
For each of the 4 (Number of Items) x 5 (Variance of Items) = 20 design conditions, we repeated the same procedure 100 times. We first selected to item pairs (1,2) (2,3), (3,4), et cetera, until (
We determined the number of comparisons per item required for a stable and accurate estimate to be the number of comparisons where
Results
Figure 1 shows the development of
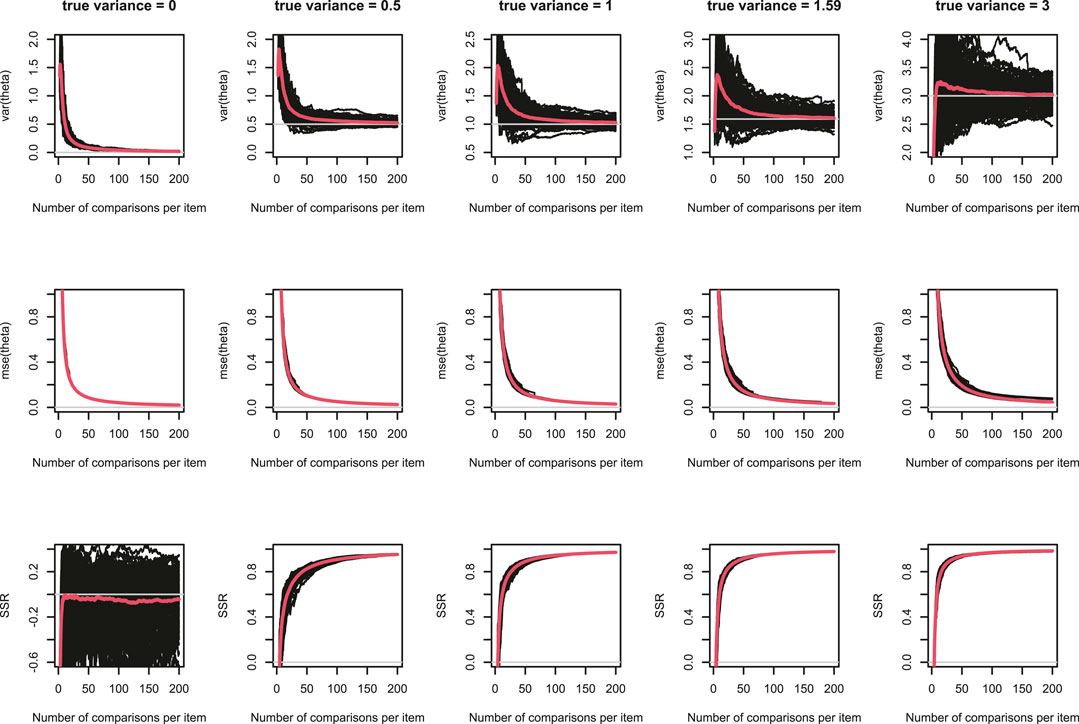
FIGURE 1. Development of
Figure 2 shows the development of bias in
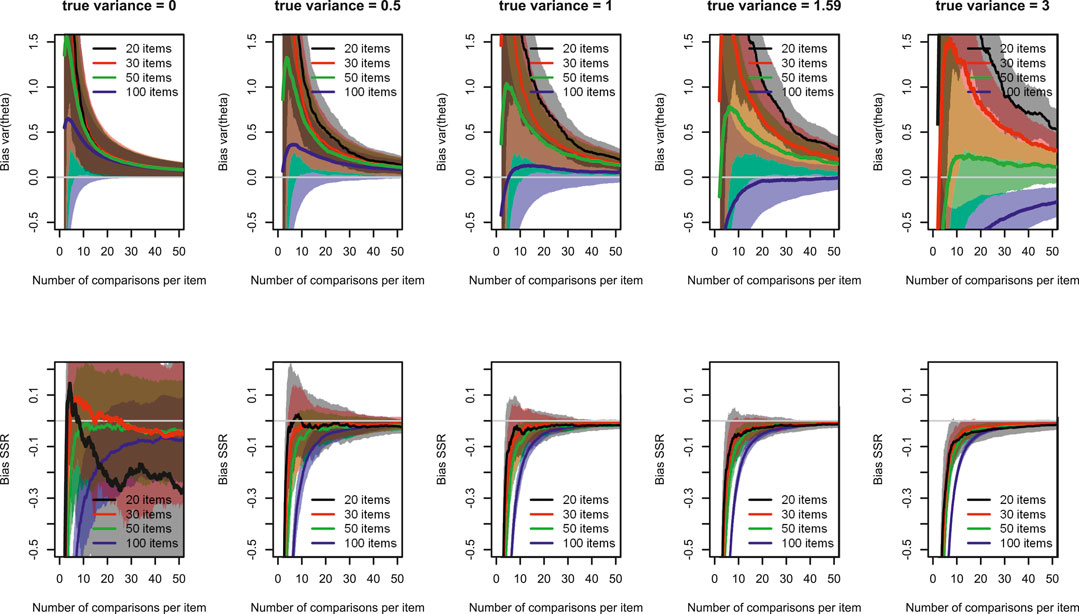
FIGURE 2. Development of bias in
We next describe the results for the other true variances. In general, the differences among the number of items conditions in
Table 2 shows the mean number of comparisons per item required for accurate
Figure 3 shows the development of the coverage of the 95% confidence intervals for the parameter estimates
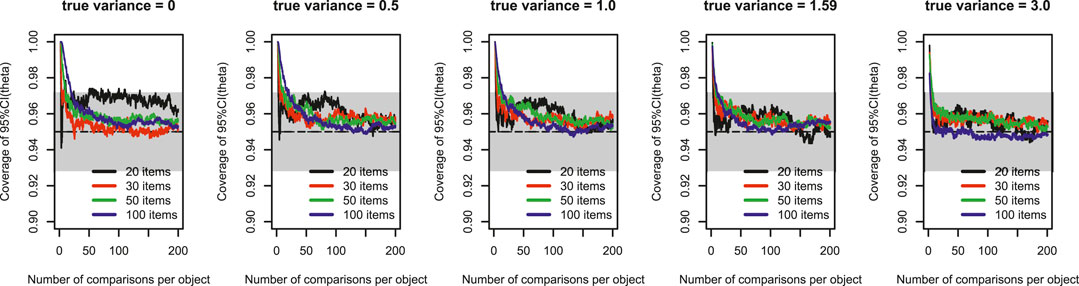
FIGURE 3. Development of the coverage of 95% confidence intervals for parameter estimates
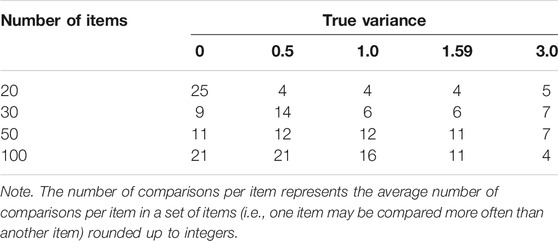
TABLE 3. Mean number of comparisons per item required for accurate coverage of 95% CI around parameter estimates.
Because the development of
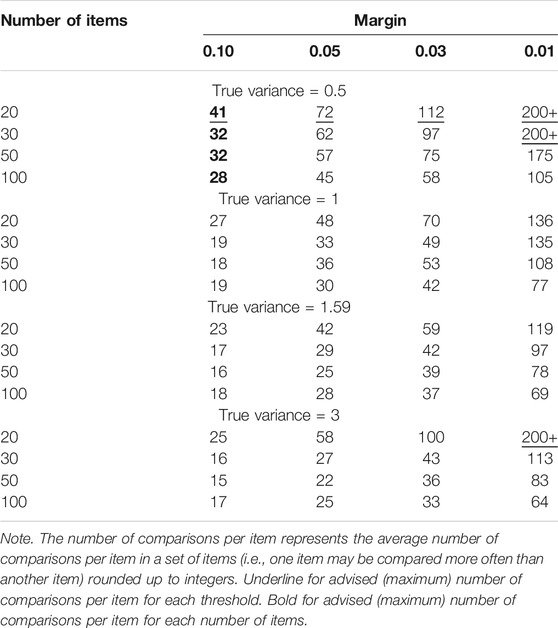
TABLE 4. Number of comparisons per item required for the SSR to estimate benchmark reliability between the benchmark reliability value and the benchmark reliability value minus the margin in 95% of the cases.
Discussion
The guideline that 200 observations per item are required for stable parameter estimates using the Rasch model (Parshall et al., 1998) was adapted for the BTL model in two ways. Guideline 1 was obtained using the number of observations per item in the Rasch model, resulting in 200 comparisons per item for the BTL model. Guideline 2 was obtained using the total number of observations in a set of items in the Rasch model, resulting in 400 comparisons per item for the BTL model.
In the simulation study, the results showed that the variation in development across simulations of both the estimated variance and the mean squared standard error were larger for larger true variance values, but the variation in development across simulations of the SSR was smaller for larger true variance values. This is interesting, because the estimated variance and the mean squared standard error are the only components of the SSR. Possibly, the variations in the estimated variance and the mean squared standard error are more aligned for larger true variances such that combining them in the SSR leads to less variation. On average, the variance was accurately estimated after 24 to 119 comparisons per item, although the number of comparisons per item differed greatly among simulations. The coverage of the 95% confidence intervals of the parameter estimates showed that the standard errors of the parameter estimates were accurate after 4 to 25 comparisons per item. The SSR could closely estimate benchmark reliability even when the variance of the parameter estimates was still overestimated. When using margins ranging from 0.10 to 0.01 to determine when the SSR closely estimated benchmark reliability, across conditions, the number of comparisons per item ranged from 15 to more than 200.
When we compare the results from the two perspectives, it seems that Guideline 2 of 400 comparisons per item is too pessimistic and overly demanding. Guideline 1 could be useful since several simulations took 200 or more comparisons per item to get stable variance estimates and it took 200 or more comparisons for the SSR to closely estimate benchmark reliability when the margin was 0.01. However, averaged across samples, the variance was accurately estimated after a maximum of 119 comparisons per item, the standard errors of the parameters and the SSR required even fewer comparisons per item, and in most conditions, the SSR closely estimated benchmark reliability after less than 50 comparisons per item. Therefore, Guideline 2 may be too demanding as well.
The alternative guideline we present here is largely based on Table 4. We recommend that comparative judgment applications require at least 41 comparisons per item based on the following considerations. In general, smaller margins led to more comparisons per item required, more items in a set led to approximately the same or fewer comparisons per item required, and larger true variances led to fewer comparisons per item required. With respect to the margin that determines how much the SSR may underestimate benchmark reliability, we are lenient by choosing the largest margin. We believe that this is justified because the benchmark reliability is usually larger than the SSR, and because Verhavert et al. (2019) indicate that the SSR already has high values with this many comparisons per item. If one prefers a smaller margin, we recommend 72 comparisons per item for a margin of 0.05, 112 comparisons for a margin of 0.03, and more than 200 comparisons for a margin of 0.01. With respect to the true variance of the item parameters, we were quite strict by choosing the largest number of comparisons, which was for a true variance of 0.5. Because one can never know the true variance in practice and because our study showed that accurate variance estimation often required many observations per item, we argue that it is best to play safe, that is, to risk performing more comparisons than required for the desired accuracy rather than risking that you do not achieve the desired accuracy by performing too few comparisons. For example, if the number of comparisons for a comparative judgment application is based on a variance of 1, but in reality the true variance is less than 1, the SSR will not be as close to the benchmark reliability as one may believe. With respect to the number of items, we also argue to be strict and play safe. Therefore, we chose the number of comparisons for 20 items for the general guideline, which requires the most comparisons per item. However, as one does know the number of items in their comparative judgment application, the required number of comparisons can be somewhat adjusted to the number of items in this set. Table 4 provides information about this adjustment, but the researcher must make the call, given that we only investigated four numbers of items.
Our guideline of 41 comparisons per item renders comparative judgment less interesting to use in practice than the guideline of 12 comparisons per item Verhavert et al. (2019) suggested. However, 41 comparisons per item are necessary for accurately determining the reliability of the measurement using the SSR. The SSR may overestimate benchmark reliability in individual samples, even when it underestimates reliability on average, especially when the number of comparisons is small. Based on Table 4, we suggest that after 41 comparisons, the risk of overestimating reliability with the SSR in individual samples is largely reduced.
Our guideline concerns reliability estimation by means of the SSR and not benchmark reliability. This means that using fewer than 41 comparisons may result in sufficient benchmark reliability (Crompvoets et al., 2020; Crompvoets et al., 2021). The problem is that we cannot determine whether this is the case based on the SSR. Therefore, if a different reliability estimate would exist for comparative judgment, the guideline might change. Measures like the root mean squared error (RMSE) may be useful in some instances, since it is related to reliability, only in terms of the original scale. However, the fact that the RMSE is scale dependent also makes it more difficult to interpret and to compare between different measurements. Therefore, a standardized measure of reliability, bound between 0 and 1, would be preferred. This is an interesting topic for future research.
In our simulation designs, we did not use adaptive pair selection algorithms or multiple raters who perceived a different truth, which are the situations in previous research where the SSR systematically overestimated benchmark reliability. The results of our study provide a baseline how the SSR and the components used to compute the SSR develop with increasing numbers of comparisons when the SSR is expected to underestimate reliability, as it should. Future research could build on our results by investigating how the components of the SSR develop with increasing numbers of comparisons in situations where the SSR might overestimate reliability. The fact that the SSR might overestimate reliability in some situations is even more reason to use a guideline that reduces the risk of overestimation due to sampling fluctuations.
Our study focused on the components of the SSR because we expected that this would show the cause of the inflation of the SSR. However, our simulation study showed that the estimated variance and standard errors of the item parameters developed differently from the SSR with increasing numbers of comparisons with respect to variation between samples, which is not what we expected. Since the components of the SSR developed differently from the SSR, they do not seem to be the cause of the inflation of the SSR. Future research could also aim at developing alternative reliability estimates to the SSR.
In conclusion, the SSR may overestimate reliability in certain situations, but it can function correctly as an underestimate of reliability even when the variance of the items is overestimated. The SSR can be used when the pairs to be compared are selected without an adaptive algorithm, when raters use the same underlying model/truth, and when the true item variance is at least 1. The variance of the items is likely to be overestimated when fewer than 24 comparisons per item were performed. An adaptation of the guideline for the Rasch model was too pessimistic. We provided a new guideline of 41 comparisons per item, with nuances concerning the number of items and the margin of accuracy for SSR estimation. Future research is needed to further investigate the SSR estimation and to develop an alternative reliability estimate.
Data Availability Statement
The datasets presented in this study can be found in online repositories. The names of the repository/repositories and accession number(s) can be found below: https://osf.io/x7qzc/.
Author Contributions
EC executed the research and wrote the manuscript. AB and KS contributed to the analysis plan and writing of the manuscript.
Conflict of Interest
EC was employed by the company Cito.
The remaining authors declare that the research was conducted in the absence of any commercial or financial relationships that could be construed as a potential conflict of interest.
Publisher’s Note
All claims expressed in this article are solely those of the authors and do not necessarily represent those of their affiliated organizations, or those of the publisher, the editors and the reviewers. Any product that may be evaluated in this article, or claim that may be made by its manufacturer, is not guaranteed or endorsed by the publisher.
References
Agresti, A. (1992). Analysis of Ordinal Paired Comparison Data. Appl. Stat. 41, 287–297. doi:10.2307/2347562
Andrich, D. (1978). Relationships between the Thurstone and Rasch Approaches to Item Scaling. Appl. Psychol. Meas. 2, 451–462. doi:10.1177/014662167800200319
Böckenholt, U. (2001). Thresholds and Intransitivities in Pairwise Judgments: A Multilevel Analysis. J. Educ. Behav. Stat. 26, 269–282. doi:10.3102/10769986026003269
Böckenholt, U. (2006). Thurstonian-based Analyses: Past, Present, and Future Utilities. Psychometrika 71, 615–629. doi:10.1007/s11336-006-1598-5
Bradley, R. A., and Terry, M. E. (1952). Rank Analysis of Incomplete Block Designs: I. The Method of Paired Comparisons. Biometrika 39, 324–345.
Bramley, T. (2015). Investigating the Reliability of Adaptive Comparative Judgement. Cambridge Assessment Research Report. Cambridge, United Kingdom: Cambridge Assessment.
Bramley, T., and Vitello, S. The Effect of Adaptivity on the Reliability Coefficient in Adaptive Comparative Judgement. Assess. Educ. Principles, Pol. Pract. (2018), 26, 43–58. doi:10.1080/0969594X.2017.1418734
Brinkhuis, M. J. S. (2014). Tracking Educational Progress. Amsterdam, Netherlands: Doctoral dissertation, University of Amsterdam. Retrieved from: https://pure.uva.nl/ws/files/2133789/153696_01_1_.pdf.
Cattelan, M. (2012). Models for Paired Comparison Data: A Review with Emphasis on Dependent Data. Statist. Sci. 27, 412–433. doi:10.1214/12-STS396
Crompvoets, E. A. V., Béguin, A. A., and Sijtsma, K. (2020). Adaptive Pairwise Comparison for Educational Measurement. J. Educ. Behav. Stat. 45, 316–338. doi:10.3102/1076998619890589
Crompvoets, E. A. V., Béguin, A. A., and Sijtsma, K. (2021). Pairwise Comparison Using a Bayesian Selection Algorithm: Efficient Holistic Measurement. Available at: https://psyarxiv.com/32nhp/.
Evers, A., Lucassen, W., Meijer, R. R., and Sijtsma, K. (2009). COTAN beoordelingssysteem voor de kwaliteit van tests [COTAN assessment system for the quality of tests]. Amsterdam, The Netherlands: Nederlands Instituut van Psychologen.
Gustafsson, J.-E. (1977). The Rasch Model for Dichotomous Items: Theory, Applications and a Computer Program. Göteborg, Sweden: Göteborg University.
Hunt, T. D., and Bentler, P. M. (2015). Quantile Lower Bounds to Reliability Based on Locally Optimal Splits. Psychometrika 80, 182–195. doi:10.1007/s11336-013-9393-6
Hunter, D. R. (2004). MM Algorithms for Generalized Bradley-Terry Models. Ann. Statist. 32, 384–406. doi:10.1214/aos/1079120141
Jones, I., and Alcock, L. (2013). Peer Assessment without Assessment Criteria. Stud. Higher Edu. 39, 1774–1787. doi:10.1080/03075079.2013.821974
Lesterhuis, M., Verhavert, S., Coertjens, L., Donche, V., and De Maeyer, S. (2017). “Comparative Judgement as a Promising Alternative to Score Competences,” in Innovative Practices for Higher Education Assessment and Measurement (Hershey, PA: IGI Global), 119–138. doi:10.4018/978-1-5225-0531-0.ch007
Linacre, J. M. (1994). Sample Size and Item Calibration Stability. Rasch Meas. Trans. 7, 328. Retrieved from: https://rasch.org/rmt/rmt74m.htm.
Lord, F. M., and Novick, M. R. (1968). Statistical Theories of Mental Test Scores. Boston, Massachusetts, United States: Addison-Wesley.
Maydeu-Olivares, A., and Böckenholt, U. (2005). Structural Equation Modeling of Paired-Comparison and Ranking Data. Psychol. Methods 10, 285–304. doi:10.1037/1082-989X.10.3.285
Maydeu-Olivares, A. (2002). Limited Information Estimation and Testing of Thurstonian Models for Preference Data. Math. Soc. Sci. 43, 467–483. doi:10.1016/s0165-4896(02)00017-3
Newhouse, C. P. (2014). Using Digital Representations of Practical Production Work for Summative Assessment. Assess. Educ. Principles, Pol. Pract. 21, 205–220. doi:10.1080/0969594X.2013.868341
Parshall, C. G., Davey, T., Spray, J. A., and Kalohn, J. C. (1998). Computerized Testing-Issues and Applications. A training session presented at the Annual Meeting of the National Council on Measurement in Education.
Pollitt, A. (2012). The Method of Adaptive Comparative Judgement. Assess. Educ. Principles, Pol. Pract. 19, 281–300. doi:10.1080/0969594X.0962012.066535410.1080/0969594x.2012.665354
Rasch, G. (1960). Probabilistic Models for Some Intelligence and Attainment Tests. Copenhagen: Danmarks Paedagogiske Institut.
Sijtsma, K. (2009). On the Use, the Misuse, and the Very Limited Usefulness of Cronbach's Alpha. Psychometrika 74, 107–120. doi:10.1007/s11336-008-9101-0
Stark, S., and Chernyshenko, O. S. (2011). Computerized Adaptive Testing with the Zinnes and Griggs Pairwise Preference Ideal point Model. Int. J. Test. 11, 231–247. doi:10.1080/15305058.2011.561459
Van Daal, T., Lesterhuis, M., Coertjens, L., Donche, V., and De Maeyer, S. (20162016). Validity of Comparative Judgement to Assess Academic Writing: Examining Implications of its Holistic Character and Building on a Shared Consensus. Assess. Educ. Principles, Pol. Pract. 26, 59–74. doi:10.1080/0969594X.2016.1253542
Van Daal, T. (2020). Making a Choice Is Not Easy?!: Unravelling The Task Difficulty of Comparative Judgement to Assess Student Work [Doctoral Dissertation. Antwerp, Belgium: University of Antwerp.
Verhavert, S., De Maeyer, S., Donche, V., and Coertjens, L. (2018). Scale Separation Reliability: What Does it Mean in the Context of Comparative Judgment? Appl. Psychol. Meas. 42, 428–445. doi:10.1177/0146621617748321
Verhavert, S., Bouwer, R., Donche, V., and De Maeyer, S. (2019). A Meta-Analysis on the Reliability of Comparative Judgement. Assess. Educ. Principles, Pol. Pract. 26, 541–562. doi:10.1080/0969594X.2019.1602027
Keywords: bias, comparative judgment (CJ), pairwise comparison (PC), reliability, stability
Citation: Crompvoets EAV, Béguin AA and Sijtsma K (2022) On the Bias and Stability of the Results of Comparative Judgment. Front. Educ. 6:788202. doi: 10.3389/feduc.2021.788202
Received: 01 October 2021; Accepted: 17 December 2021;
Published: 01 March 2022.
Edited by:
Renske Bouwer, Utrecht University, NetherlandsReviewed by:
Tom Benton, Cambridge Assessment, United KingdomKaiwen Man, University of Alabama, United States
Copyright © 2022 Crompvoets, Béguin and Sijtsma. This is an open-access article distributed under the terms of the Creative Commons Attribution License (CC BY). The use, distribution or reproduction in other forums is permitted, provided the original author(s) and the copyright owner(s) are credited and that the original publication in this journal is cited, in accordance with accepted academic practice. No use, distribution or reproduction is permitted which does not comply with these terms.
*Correspondence: Elise A. V. Crompvoets, ZS5hLnYuY3JvbXB2b2V0c0B1dnQubmw=