- 1Purdue Polytechnic Institute, Purdue University, West Lafayette, IN, United States
- 2College of Education, Curriculum and Instruction, Purdue University, West Lafayette, IN, United States
- 3School of Technology, Brigham Young University, Provo, UT, United States
Adaptive comparative judgment (ACJ) is a holistic judgment approach used to evaluate the quality of something (e.g., student work) in which individuals are presented with pairs of work and select the better item from each pair. This approach has demonstrated high levels of reliability with less bias than other approaches, hence providing accurate values in summative and formative assessment in educational settings. Though ACJ itself has demonstrated significantly high reliability levels, relatively few studies have investigated the validity of peer-evaluated ACJ in the context of design thinking. This study explored peer-evaluation, facilitated through ACJ, in terms of construct validity and criterion validity (concurrent validity and predictive validity) in the context of a design thinking course. Using ACJ, undergraduate students (n = 597) who took a design thinking course during Spring 2019 were invited to evaluate design point-of-view (POV) statements written by their peers. As a result of this ACJ exercise, each POV statement attained a specific parameter value, which reflects the quality of POV statements. In order to examine the construct validity, researchers conducted a content analysis, comparing the contents of the 10 POV statements with highest scores (parameter values) and the 10 POV statements with the lowest scores (parameter values)—as derived from the ACJ session. For the criterion validity, we studied the relationship between peer-evaluated ACJ and grader’s rubric-based grading. To study the concurrent validity, we investigated the correlation between peer-evaluated ACJ parameter values and grades assigned by course instructors for the same POV writing task. Then, predictive validity was studied by exploring if peer-evaluated ACJ of POV statements were predictive of students’ grades on the final project. Results showed that the contents of the statements with the highest parameter values were of better quality compared to the statements with the lowest parameter values. Therefore, peer-evaluated ACJ showed construct validity. Also, though peer-evaluated ACJ did not show concurrent validity, it did show moderate predictive validity.
Introduction
Design is believed to be the core of technology and engineering, which promotes experiential learning towards the development of a robust understanding (Dym et al., 2005; Atman et al., 2008). Design situates learning in real life contexts, involving ambiguity and multiple potentially viable solutions (Lammi and Becker, 2013), and thus promotes the development of students to adapt rapidly to diverse, complicated, and changing requirements (Dym et al., 2005; Lammi and Becker, 2013). Generally, design thinking in the context of technology and engineering settings follows five stages (Erickson et al., 2005; Lindberg et al., 2010): Empathy, define, ideate, prototype, and test. In the stage of empathy, students learn about the users for whom they are designing. Then, they redefine and articulate their specific design problem based on the findings from the empathy stage. Later, students brainstorm creative solutions, build prototypes of ideas, and test prototypes with the original/possible user group to assess their ideas. In the design thinking process, defining the problem is a critical step to capturing what the students are attempting to accomplish through the design. The Point-Of-View (POV) statement (Figure 1), which includes three parts (user, need, insight), is one element of problem definition; this artifact often arises during the define stage and serves as a guideline during the entire design process (Sohaib et al., 2019).
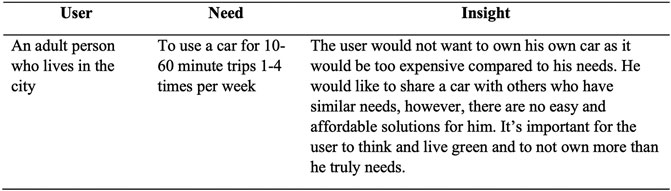
FIGURE 1. An example of a Point of View (POV) from course reading (Rikke Friis and Teo Yu, 2020).
In the context of the design thinking course in which this research took place, students worked in groups to write a POV statement to address one or more problem(s) their potential user(s) may confront, by combining user, needs, and insights into a 1-2 sentence statement. Students were instructed that a good problem statement is human-centered, reflecting specific users’ insights, broad enough for creative freedom but not too narrowly focused to explore creative ideas, and narrow enough to make it manageable and feasible within a given timeframe (Rikke Friis and Teo Yu, 2020). Hence, a good POV statement is considered a “meaningful and actionable” problem statement (Rikke Friis and Teo Yu, 2020), which guides people to foreground insights about the emotion and experiences of possible user groups (Karjalainen, 2016). It is a crucial step which defines the right challenge to situate the ideation process in a goal-oriented manner (Woolery, 2019) and inspires a team to generate multiple quality solutions (Kernbach and Nabergoj, 2018). Further, effective POV statements facilitate the ideation process by helping an individual to better communicate one’s vision to team members or other stakeholders (Karjalainen, 2016).
To encourage students to write well-defined and focused POV statements, design thinking instructors have highlighted the importance of teaching detailed, explicit criteria of good POV statements based on a specific grading rubric (Gettens et al., 2015; Riofrío et al., 2015; Gettens and Spotts, 2018; Haolin et al., 2019). Though competent use of scoring rubrics is believed to ensure reliability and validity of performance assessments, there are inherent difficulties in carrying out rubric-based assessments on summative assignments (Jonsson and Svingby, 2007). Further, this assessment becomes especially difficult in the context of collaborative, project-based design thinking assignments which demand a high level of creativity (Mahboub et al., 2004), especially in terms of organizing the content and structure of the rubric (Chapman and Inman, 2009). Bartholomew et al. have also noted that traditional teacher-centric assessment models (e.g., rubrics) are not always effective at facilitating students’ learning in a meaningful way (Bartholomew et al., 2020a) and other studies have raised questions about the reliability and validity of the rubric-based assessment, such as subjectivity bias of the graders (Hoge and Butcher, 1984), one’s leniency or severity (Lunz and Stahl, 1990; Lunz et al., 1990; Spooren, 2010), and halo effect due to the broader knowledge of some students (Wilson and Wright, 1993).
In contrast to rubrics, Adaptive comparative judgement (ACJ) has been implemented as an efficient and statistically sound measure to assess the relative quality of each student’s work (Bartholomew et al., 2019; Bartholomew et al., 2020a). In ACJ, an individual compares and evaluates pairs of items (e.g., the POV statements) and chooses the better of the two; this process is repeated—with different pairings of items—until a rank order of all items is created (Thurstone, 1927). The pairwise comparison process is iterative and multiple judges can make comparative decisions on multiple sets of work (Thurstone, 1927), with the final ordering of items—from strongest to weakest—calculated using multifaceted Rasch modeling (Rasch, 1980). In addition to a ranking, the judged quality of the items results in the creation of parameter values—which specify both the rank and the magnitude of differences between items—based on the outcome of the judgments (Pollitt, 2012b). Thus, the ACJ approach differs fundamentally from a traditional rubric-based approach in that it allows summative assessment without subjective point assigning (Pollitt, 2012b; Bartholomew and Jones, 2021).
For ACJ, there is no predetermined specific criteria like rubric-based assessments. Rather, in ACJ, holistic statement, or basis for judgment, is used. This provides the rationale for judges’ decisions and is considered a critical theoretical underpinning for reliability and validity (Van Daal et al., 2019). To achieve a level of consensus in ACJ, professionally trained judges’ with collective expertise are often considered ideal; however, studies have also demonstrated that students—with less preparation and/or expertise—can also be proficient judges with levels of reliability and validity similar to professionals (Jones and Alcock, 2014). For examples, studies investigating concurrent validity of peer-evaluated ACJ showed that the results generated by peer-evaluated ACJ had a high correlation with the results of experts (e.g., professionally trained instructors, graders) (Jones and Alcock, 2014; Bartholomew et al., 2020a). Jones and Alcock (Jones and Alcock, 2014) conducted peer-evaluated ACJ in the field of mathematics, to see the conceptual understanding of multivariable calculus. The results indicated mean peer and mean expert scores of ACJ had high correlation (r = 0.77), and also had significant correlation with summative assessments. Similarly, Bartholomew and others (Bartholomew et al., 2020a) compared the results of professional, experienced instructors’ ACJ with student-evaluated ACJ results. Though peer-evaluated ACJ showed non-normality, results suggested strong correlation between peer-evaluated ACJ and instructor-evaluated ACJ.
The present study aims to investigate whether peer-evaluated ACJ can yield sound validity in design thinking. More specifically, the validity of ACJ was studied from two perspectives: construct validity and criterion validity (as investigated through both concurrent and predictive validity). The construct validity was studied based on the holistic nature of ACJ. Three researchers with professional backgrounds evaluated POV statements, studying whether the results of ACJ (parameter values) appropriately reflected general criteria of good POV statement. Following the construct validity, criterion validity was studied. First, researchers investigated concurrent validity of peer-evaluated ACJ by studying the relationships of peer-evaluated ACJ and instructors’ rubric-based grading. Second, the researchers studied the predictive validity of peer-evaluated ACJ by studying the relationships of peer-evaluated ACJ and students’ final grades. By doing so, we explored the validity of implementing peer-evaluated ACJ in design thinking context.
Literature Review
In this section, we first will start by introducing the concept of a POV statement and the importance of a good POV statement in a design thinking context. Then, two assessments implemented to evaluate POV statements will be presented: rubric-based grading and ACJ. To explore the potential of ACJ as an effective and efficient alternative to rubric-based grading widely implemented in design thinking context, we share a brief review of existing literature on the reliability and validity prior to making our contribution to the knowledge base through this research.
Point-Of-View Statements
The problem definition stage of design thinking explores the problem space and creates a meaningful and actionable problem statement (Rikke Friis and Teo Yu, 2020). Dam and Siang asserted that a good POV statement has three major traits (Dam and Siang, 2018). First, the POV needs to be human-oriented. This means the problem statement students write should focus on the specific users, from whom they learn the needs and insights through the empathy stage. Also, a human-centered POV statement is required to be about the people who are stakeholders in the design problem rather than the technology, monetary return, and/or product improvement. Second, the problem statement should be broad enough for creative freedom meaning the problem statement should be devoid of a specific method or solution. When the statement is framed around a narrowly defined solution, or with a possible solution in mind, it restricts the creativity of the ideation process (Wedell-Wedellsborg, 2017). The final trait of a strong problem statement is that it should be narrow enough to make it viable with the available resources. The third trait complements the second trait, which suggests that the POV statements should possess appropriate parameters for the scope of the problem, avoiding extreme narrowness or ambiguity. A good POV statement, equipped with all three traits, can contribute to delivering attention, providing sound framework for the problem, motivating students working on the problem, and providing informational guidelines (Sohaib et al., 2019).
Assessment of Point-Of-View Statements With Rubrics
One trend among assessments in higher education is a shift from traditional knowledge-based tests towards assessment to support learning (Dochy et al., 2006). In order to capture students’ higher-order thinking, a credible, trustworthy assessment, which is both valid and reliable, is needed. The historic development of a rubric as a scoring tool for the assessment of students’ authentic and complex work, including what counts (e.g., user, needs, insights are what count in POV statements) and for how much, has traditionally centered on 1) articulating the expectations of quality for each task and 2) describing the gradation of quality (e.g., excellent to poor, proficient to novice) for each element (Chapman and Inman, 2009; Reddy and Andrade, 2010). Three factors are included in a rubric: evaluation criteria, quality definitions, and a scoring strategy. The analytic rubric used in the Design thinking course to grade POV statements is included below (Table 1). The rubric-based evaluation of competency is made through analytical reflections by graders, in which the representation of the ability is scored on a set of established categories of criteria (Coenen et al., 2018).
Adaptive Comparative Judgment
Adaptive comparative judgment (ACJ) is an evaluation approach accomplished through multiple comparisons. In 1927, Thurstone presented the “Law of Comparative Judgment” (Thurstone, 1927) as an alternative to the existing measurement scales, aimed at increasing reliability. Thurstone specifically argued that making decisions using holistic comparative judgments can increase reliability compared to decisions made from predetermined rubric criteria (Thurstone, 1927). Years later, based on Thurstone’s law of comparative judgement, Pollitt outlined the potential for ACJ, seeking the possibility of implementing the comparative judgment approach in marking a wide range of educational assessments (Pollitt, 2012b), with statistically sound measurements in terms of accuracy and consistency (Bartholomew and Jones, 2021). The adaptive attribute of ACJ is based on an algorithm embedded within the approach which pairs similarly ranked items as the judge makes progress in the comparative judgement process—an approach aimed at expediting the process of achieving an acceptable level of reliability (Kimbell, 2008; Bartholomew et al., 2019).
We choose to use a software titled RMCompare to facilitate adaptive comparative judgment enabling students to make a series of judgments with an outcome consisting of several helpful data, including: a rank order of the items judged, parameter values (statistical values representing the relative quality of each item), judgment time of each comparison, a misfit statistic of judges and items (showing consistency, or lack thereof, among judgments), and judge-provided rationale for the comparative decisions (Pollitt, 2012b). Previous research has shown that utilizing these data can provide educators with a host of possibilities including insight into students’ judgment criteria, consensus, and their processing/understanding of the given task. In a design thinking process scenario specifically, ACJ—though originally designed for expert assessment—has demonstrated through educational research efforts to be a helpful measure for students who participate in the task because it promotes learning and engagement (Seery et al., 2012; Bartholomew et al., 2019). Specifically, Bartholomew et al. noted that ACJ can efficiently facilitate learning among students studying design and innovation by including students as judges (Bartholomew et al., 2020a).
Validity of Adaptive Comparative Judgment
Construct Validity of Adaptive Comparative Judgment: Holistic Approach
The traditional concept of validity was established by Kelley (Kelley, 1927), who claimed that validity is the extent to which a test measures what it is supposed to measure. Construct validity pertains to “the degree to which the measure of a content sufficiently measures the intended concept” (O’Leary-Kelly and Vokurka, 1998, p. 387). The validity estimate has to be considered in the context of its use, and needs evidence of the relevance and the utility of the score inferences and actions (Messick, 1994). In other words, researchers need to take into account the context, with adequate construct validity evidence, to support the inferences made from a measure (Hubley and Zumbo, 2011).
Since ACJ requires holistic assessment, researchers examining the validity of comparative judgement have highlighted the importance of an agreed upon set of criteria (Pollitt, 2012a) and shared consensus across judges (Pollitt, 2012a; Jones et al., 2015; Van Daal et al., 2019). In terms of an agreed upon criteria for judgment, in some instances, rather than following a predetermined specific criterion for the assessment, judges in ACJ have followed a general description regarding the assessment. For instance, Pollitt (Pollitt, 2012a) used the “Importance Statements” published on England’s National Curriculum to assess design thinking portfolios:
In design and technology pupils combine practical and technological skills with creative thinking to design and make products and systems that meet human needs. They learn to use current technologies and consider the impact of future technological developments. They learn to think creatively and intervene to improve the quality of life, solving problems as individuals and members of a team.
Working in stimulating contexts that provide a range of opportunities and draw on the local ethos, community and wider world, pupils identify needs and opportunities. They respond with ideas, products and systems, challenging expectations where appropriate. They combine practical and intellectual skills with an understanding of aesthetic, technical, cultural, health, social, emotional, economic, industrial, and environmental issues. As they do so, they evaluate present and past design and technology, and its uses and effects. Through design and technology pupils develop confidence in using practical skills and become discriminating users of products. They apply their creative thinking and learn to innovate. (QCDA., 1999).
The shared consensus among judges, facilitated through the ACJ process, underpins the validity of ACJ, because each artifact is systematically evaluated in various pairings across multiple judges. Through the process of judgement, a shared conceptualization of quality and collective expertise of judges is then reflected in the final rank order (Van Daal et al., 2019). Though the majority of studies initially limited the judges to trained graders/instructors, recent work has explored students’ (or other untrained judges’) competence as judges in ACJ (Rowsome et al., 2013; Jones and Alcock, 2014; Palisse et al., 2021). Findings suggest that, in many cases, students—and even out-of-class-professionals (e.g., practicing engineers; see Strimel et al., 2021) can reach similar consensus to that reached by trained judges or classroom teachers suggesting a shared quality consensus across different judge groups.
Considering the curriculum, goals, and educational setting of design thinking, our research team postulated that when implementing ACJ to assess POV statements of the students in the design thinking course, the high score of parameter values should reasonably be interpreted as one’s ability to write a good POV statement, while a low score of parameter values can be understood as one’s low ability, or lack of ability, to write a good POV statement.
Validity of Adaptive Comparative Judgment: Criterion Validity
In classical views of validity, criterion validity concerns “the correlation with a measure and a standard regarded as a representative of the construct under consideration” (Clemens et al., 2018). If the measure shows a correlation with an assessment in the same time frame, it is termed concurrent validity. If the measure shows a correlation with a future assessment, it is termed predictive validity. The criterion validity evidence is related to how accurately one measure predicts the outcome of another criterion measure. Criterion validity is useful for predicting performance of an individual in different context (e.g., past, present, future) (Borrego et al., 2009).
Although the unique, holistic characteristics of ACJ provides meaningful insights, concurrent validity of ACJ also has been studied with great importance (Jones and Alcock, 2014; Jones et al., 2015; Bisson et al., 2016). There has been several efforts to establish criterion validity of ACJ, which mostly concentrated on the concurrent validity (Jones and Alcock, 2014; Jones et al., 2015; Bisson et al., 2016). These studies compared the results of ACJ with the results of other validated assessments to investigate the conceptual understanding. Examining the criterion validity is crucial to implement ACJ in various educational contexts as an effective alternative. Considering that ACJ can be rapidly applied to target concepts, it has the potential to effectively and efficiently evaluate various artifacts in a wide range of contexts with high validity and reliability (Bisson et al., 2016).
Informed by previous studies, this study examines the validity of peer-evaluated ACJ in design thinking context. Though it has relatively high and stable reliability, coming from its adaptive nature, empirical evidence regarding ACJ’s predictive validity is limited (Seery et al., 2012; Van Daal et al., 2019). Delving into predictive validity is necessary for demonstrating the technical adequacy and practical utility of ACJ (Clemens et al., 2018). Therefore, investigating the validity of ACJ may provide another potentially strong peer assessment measure in design thinking context, where most of the assignments are portfolios, thus hard to operationalize explicit assessment criteria using traditional rubric based approaches (Bartholomew et al., 2020a). Not only may ACJ be a viable assessment tool but, it may also be a valuable learning experience for students who engage in the peer evaluation process (Bartholomew et al., 2020a).
Research Question
The ACJ-produced rank order and standardized scores (i.e., parameter values) reflect the relative work quality of students’ POV statements according to the ACJ judges. Therefore, researchers assumed that POV statements with higher parameter values were better in quality when compared to the POV statements with lower parameter values. The first research question investigated in this study will qualitatively explore how students’ shared consensus reflects the general and broad criteria of good POV statement.
RQ 1. What is the construct validity of ACJ? Does peer-reviewed ACJ reflect general criteria of good POV statements?
Taking its effectiveness and efficiency into consideration, studies already explored ACJ’s theoretical promise in educational setting as a new approach with acceptable statistical evidence (Jones and Alcock, 2014; Bartholomew et al., 2020a). This study aims to investigate the criterion validity of ACJ. More specifically, concurrent validity and predictive validity of ACJ were examined by comparing the results of ACJ with rubric-based grading.
RQ 2. What is the criterion validity of ACJ? Does peer-reviewed ACJ correlate with existing assessment?
RQ 2-1. What is the concurrent validity of ACJ? Does peer-reviewed ACJ correlate with instructors’ rubric-based grading on the same assignment?
RQ 2-2. What is the predictive validity of ACJ? Does peer-reviewed ACJ predict instructors’ rubric-based grading on the key final project deliverable?
Methods
Participants
Study participants were 597 technology students out of 621 students enrolled in a first-year Design Thinking Course at a large Midwestern university in the United States during Spring 2019. These students are subset of entire Polytechnic population (N = 4,480). This research was approved by the university’s Institutional Research Board. Sociodemographic information of the participants is provided in Table 2.
Research Process
Research Design
The research design of this study is graphically depicted by Figure 2. First, students wrote the POV statements during the project 3 as a team. Researchers collated and anonymized the total 124 POV statements. Followed by this process, students performed ACJ on their peer’s POV statements (Assessment 1, peer-evaluated ACJ). Concurrently, instructors graded the same POV statement using rubrics (Assessment 2, Table 1). After project 3, instructors, who worked as graders assigned grades to final deliverables of project 3 (Assessment 3). To study the construct validity, researchers qualitatively analyzed ACJ statements using content analysis. Before analyzing the criterion validity, we analyzed the descriptive statistics of all three assessments. For the concurrent validity, we studied correlation between the peer-evaluated ACJ (Assessment 1) and instructors’ grading based on rubric (Assessment 2). Finally, for the predictive validity, we examined if peer-evaluated ACJ (Assessment 1) predicts final deliverables (Assessment 3).
Study Context and Point-Of-View Statement Writing
In the semester-long, three credit design thinking course, 597 students from 14 sections designed and developed solutions to real problems, voluntarily forming 124 groups in alignment with their current interests or major within each section of the course. During the course, students fostered their own foundational understanding of design thinking by participating in three projects, in which they could create, optimize, and prepare innovative solutions for people. The first project was designed to provide overview and theoretical descriptions with simple hands-on projects about the design thinking process and lasted about a week. The second course project was a more real-life based group project, and took approximately 4 weeks, following the five stages of design thinking: empathize, define the problem, ideate, prototype, and test (retest).
The final project spanned about 8 weeks and engaged students in addressing a problem related to a self-selected grand challenge of engineering (National Academy of Engineering, 2008). In this study, we observed the “define” stage of the third project, when we hypothesized that students would have had enough experience with the design thinking process, including the POV statements, to work comfortably through the designing approach. At this point in class these students had already written four POV statements, two as an individual during the first project, and two as a team during the second project. As a part of the define stage during the third project, the course instructors utilized one 50-min class concentrating on POV creation, highlighting essential components of quality POV statements (user, needs, and insights), structures of POV statements, essential criteria for producing a good POV statement, and importance of writing a good POV statement for this project. During and after this class session, the students wrote a definition of their problem as a team using a provided format for POV statements [User . . . (descriptive)] needs [need . . . (verb)] because [insight. . . (compelling)].
Measures
This study used three types of assessments: peer-evaluated ACJ of POVs (Assessment 1), rubric-based grading of POV(Assessment 2), and rubric-based grading of final deliverables (Assessment 3). First, we compared two types of assessments: Assessment 1 and Assessment 2. For both rubric based and ACJ based assessments, all the POV statements from the 124 teams written at the beginning of the final project were included in the dataset. Then, researchers included the rubric-based grading of final deliverables (Assessment 3) to see if the peer-evaluated ACJ can predict the future achievements.
Assessment 1. Peer-Evaluated ACJ of the POV Statements.
For the peer-evaluated ACJ, the POV statements were collated, anonymized, and uploaded into the ACJ software called RMCompare for evaluation. Near the end of the final project, in preparation for presenting their design projects, students were challenged to evaluate the POV statements using the RMCompare interface by selecting the POV statement they believed was holistically better between the pairs displayed to them. For the holistic judgment prompt, students were reminded of general qualities of good POV statements (Rikke Friis and Teo Yu, 2020), which were already familiar to them. Students previously used these same criteria (Rikke Friis and Teo Yu, 2020) as class material to learn the notion of POV statement. Each student (550 of 597) compared approximately 8 pairs of POV statements written by their peers. The subsequent ACJ judgments resulted in all 124 POV statements being compared at least 12 times to other increasingly similarly ranked POV statements in line with the adaptive nature of the software. As a result, the rank and parameter value for each POV statement was automatically calculated using the embedded Rasch multifaceted model (see Pollitt, 2012b; Pollitt, 2015 for more details).
Assessment 2. Instructor’s Rubric-Based Grading of the POV Statements.
Rubric based grading was performed based on assigned criteria (Table 1). Graders are currently working as course instructors of design thinking course, who were pursuing a MS or Ph.D. degree in relevant fields (e.g., engineering, polytechnic, or education) at the time of study. Each grader assessed two sections, in which around 40 students enrolled. As a result, the numerical grading value (total 15 pts) were provided.
Assessment 3. Final Project Deliverables.
Student teams submitted their final prototypes as one of the significant final project deliverables. They plan, implement, and reflect on testing scenarios for their prototypes, and present prototypes for the purpose of receiving feedback from the peers. Instructors (same as Assessment 2) grade the prototypes as a key final deliverable based on assigned criteria (see Table 3). As a result, the numerical grading value (total 35 pts) were provided.
Analysis
Construct Validity
Qualitative Content Analysis (QCA)
Content analysis is an analytic method frequently adopted in both quantitative and qualitative research for the systematic reduction of text or video data (Hsieh and Shannon, 2005; Mayring, 2015). Qualitative content analysis, QCA is one of the recognized research methods in the field of education. It is a method for “the subjective interpretation of the content of text data through the systematic classification process of coding and identifying themes or patterns” (Hsieh and Shannon, 2005, p. 1278). We used directive (qualitative) content analysis to extend the findings of ACJ, therefore enriching the findings (Potter and Levine-Donnerstein, 1999). The focus of current study was on validating ACJ from analyzing the key concepts of POV statements (e.g., structure, user, needs, and insights). Researchers began the research by identifying the key concepts POV statements. Then, researchers begin coding immediately with the predetermined codes. We articulated four categories based on the discussion: framework (alignment, logic), user, needs, and insights.
Two major approaches are frequently used for the validity and reliability of QCA: Quantitative and qualitative (Mayring, 2015). Quantitative approach measures inter-coder reliability and agreement using the quantitative methods (Messick, 1994). Qualitative approach adopts a consensus process in which multiple coders independently code the data, compare their coding, and discuss and resolve discrepancies when they arise, rather than measuring them (Schreier, 2012; Mayring, 2015). The qualitative validation approach is preferred to the quantitative research because it provides reason with reflexivity, the critical thinking of researchers’ own assumptions and perspective (Schreier, 2012). This is particularly important during the negotiation process because coders meet to discuss their own rationale used in coding. In this study context, researchers compared, reviewed, and revisited coding process before reaching consensus on the codes (Hsieh and Shannon, 2005; Forman and Damschroder, 2007; Schreier, 2012).
Sample Selections of Point-Of-View Statements
To provide validation to ACJ data (parameter values), researchers selectively analyzed 20 POV statements out of the 124 POV statements as was done in a previous related study (Bartholomew et al., 2020b). Based on ACJ, we selectively analyzed the 10 POV statements with the highest parameter values and the 10 POV statements with the lowest parameter values to provide contrasting cases. Using the rubrics implemented in the grading system (Table 1), researchers analyzed whether the parameter values were aligned with the criteria for a strong POV statement. More specifically, in an effort to explore the construct validity of the ACJ results, we investigated if the 10 POV statements with high parameter values better reflect the required criteria for good POV statements and if the 10 POV statements with low parameter values fail to meet the criteria required of the student groups.
Criterion Validity Analysis
The software program RStudio Version 1.3.959 was used for our criterion validity analysis.
Preliminary Data Analysis
Prior to running the statistical analysis, researchers screened the data for missing values and outliers. Participants with missing data on a variable were excluded from the analysis. For instance, if there was a missing value either in grader’s grading in POV statements or final deliverables, the data were not included in the statistical analysis. As a result, 26 participants were removed from data. Values greater than 4 SD from the mean on any measures were considered as outliers and thus removed. The results of ACJ demonstrated a high level of interrater reliability (r = 0.94), with none of the judges showing significant misalignment.
Descriptive Statistics
We analyzed the rubric based grading of POV statements (POV Grading), ACJ on the same POV statements (ACJ), and rubric-based grading on the final deliverables (Final Deliverable) (Table 4).
Correlation and Regression Analysis
Specifically, both Spearman’s
Results
Construct Validity of Peer-Evaluated Adaptive Comparative Judgment
The POV statements with the highest parameter values (Table 5) and the lowest parameter values (Table 6) are presented based on their rank order and referenced in the following discussion.
Framework of Point-Of-View Statements
Structure and Length
To articulate their user, needs, and insights to solve the current challenges users are facing, the assignment required students to make a POV statement using the sentence structure: [User . . . (descriptive)] needs [need . . . (verb)] because [insight. . . (compelling)] (Rikke Friis and Teo Yu, 2020). Though most of the POV statements with high parameter values followed the basic structures, some of the POV statements with low parameter values deviated from the basic POV statement structure. For instance, the POV #117 and #119 statements omitted insights resulting in their POV statements not leading to an actionable statement. The #120 statement included unnecessary background information prior to the POV statement which may be distracting and hinder the readers’ understanding of the POV statement itself. In the #123 statement, a specific solution was presented instead of the POV statement and a problem statement like this, framed with a certain solution in mind, might restrict the creativity of problem-solving (Wedell-Wedellsborg, 2017). Therefore, based on our analysis, the judges perceived that good POV statements should include the required information with all the necessary components (i.e., user, needs, insights) in a concise manner with the necessary details.
In terms of the length, researchers found the POV statements of low parameter values were notably shorter than the POV statements with high parameter values, except for the statement #120. It provides insights to the researchers that the students produced POV statements with lower parameter values are not clearly specifying the user, need and insight. Therefore, short length reflects the lack of thorough description to understand the context in which the POV statements are based on. Also, when we took a more detailed analysis on the statement #120, we found that this statement included introductory sentence as part of their POV statement. The inclusion of introductory sentences can either be interpreted as students’ misunderstanding of the structure of POV statement, or lack of writing skills to integrate all the necessary detailed information in the structure of POV statement.
Alignment and Logic
The user, needs, and insights should be aligned and actionable to increase the likelihood of success during the follow-up designing process. Well-aligned POV statements enhance the team’s ability to assist the users in meeting their goals and objectives in an efficient and effective way (Wolcott et al., 2021). Compared to the high parameter value statements, our research team agreed that the low parameter value statements typically showed less logically aligned user, needs, and insights. In most of the cases, the less cohesive POV statements came from stating the user and needs in a manner that was too broad, vague, or less clarified. Statement #121, #122, #124 were direct examples of this problem. For instance, the statement #121 fell short of a detailed illustration about why “people who live in urban areas” needed a “sustainable source of foods”. Too broad of a user group, like “people live in urban areas”, was not cohesively related to the need of “sustainable foods”, and this statement did not articulate what were the “sustainable foods”. Thus, it appeared difficult to determine whether it was hard to gain sustainable sources of food in urban areas, or whether the struggles were due to the socio-economic status of the residents in urban districts that more sustainable sources of food were needed. Moreover, the insights did not clarify the range and definition of “imports”, and why it was important and/or positive to decrease the reliance on imports.
POV statements lacking alignment between the user, need and insight were not logical and/or easy to follow. These kinds of statements appeared unfounded or unsupported. For instance, statement #117, #119, #120, #121, and #122 could face rebuttal because the user group was not well aligned with the needs. As an example, the statement #122 insisted that the “Food industry” “waste less”, to prevent “excessive usage of natural resources”. Not only were the contents of this statement not written in the way POV statements required, but it also lacked a logical explanation of why the food industry needed to waste less, while there could be many possible factors/ subjects excessively wasting natural resources. Overall, not including the components of a POV statement (user, need and insight) or including them in ways that are not well aligned yield POV statements that are marginally actionable and vague. Additionally, the lower quality POV statements often framed the users’ needs as oriented towards a specific solution rather than focusing on the problem at hand.
Components of Point-Of-View Statements
User
Although these were broad in some senses, the user defined in both the POV statements with high parameter values and low parameter values were narrowed down with descriptive explanations, though the degree of specification differed from statement to statement. Specifically, some of the POV statements with low parameter values revealed limitations when defining users. For instance, the statement #115 defined “People” as a user group but did not narrow down the user and not provide any illustrated details about the user group they are targeting. The user group of the statement #118 was “pedestrians”, which was not any different from “people”, failing to narrow it down enough. The statement #123 did not designate any user group, therefore making the targeted user group remain unspecified. By failing to define user groups from the specific user’s perspective in the problem-solving, these teams fell short of solutions with quantity and higher quality.
Needs
The needs are something essential or important, and are required for targeted users (Interaction Design Foundation, 2020). Though it still could have been improved, compared to the low parameter value statements, most of the high parameter value statements incorporated adjectives and details specific to the user group. For instance, the statement #1 and #2 proposed the needs pertinent to the user group. The statement #1 proposed a need for an “accessible, personalized and interactive” method for safety meetings. When limited to the user and needs, this statement did not seem to provide sufficient information due to the vague depiction of the user group. However, considering their insights illustrated the current situation of the statement #1 user group, it seemed to reflect the current needs the user group was confronting. The statement #2 also showed needs of “reducing the CO2 emissions” relevant to the user group utilizing the automobiles and transportation vehicles. Also, the user group of #6 was students who had constraints on time and accessibility on campus. The needs of these user groups were stated as a “means to achieve a healthier lifestyle without spending too much extra time and money”. The proposed need of an efficient, healthy lifestyle was well aligned with the busy user group on campus.
Compared to the high parameter value statements, the low parameter statements were less pertinent to the user group because either the user group was too general and not specified enough or the needs were too broad and vague. For the statements like #115 and #119, it was hard to connect the user and needs because the user was “people” or “anyone involved in scientific or technology labs”. Like these two statements, either too broad or user groups without any detailed information, hindered the cohesive alignment of user group and their needs. Statement #122 and #124 showed the examples of too vague and broad needs: “To waste less (#122)’ and ‘to be improved (#124)” lacked adjectives and details to enhance the needs. For the needs of the statement #122, missing details of “what” was wasted and “how much” it should or could be less wasted made the statement less strong. The statement #124 was not only less related to the user group in that it did not provide how the infrastructure(s) could be improved, but also the user, “infrastructure at (The name of University)” was not clarified enough among the broad notion of infrastructure (e.g., system or organization, clinical facilities, offices, centers, communities) (Longtin, 2014).
The high parameter value POV statements identified the user groups’ needs and goals in, or with, a verb form so that users could see the choices they could make and choose among the options. In contrast, some of the low parameter value statements’ needs provided the needs in a noun form, which described the solution relying on technology, money/funding, a product (specifications), and/or a system (e.g., #117, #118, #119, #120, #121). Although these statements proposed possible solutions, those were limited, predetermined solutions from the perspectives of the writers, not allowing the alternatives from the user’s stance. For example, the statement #118 suggested “signage” as a need of their user group to reduce the risk of accidents in the bike lanes. However, this need was a solution and did not include various other possible solutions and the actual needs designers might consider, obviously excluding the possibility that the signage itself might not be the only best solution for the pedestrians.
Another problem found in the low parameter value statements was the interpretation of “need” itself. While most of the high parameter value statements concentrated on the goals and needs user groups experience, some of the low parameter value statements regarded the needs of user groups according to the dictionary definition, as a requirement, necessary duty, or obligation instead of user’s goals. This particular type of need misinterpretation can be found in statement #115, #122, and #124. For example, statement #115 highlighted a necessary moral, educational duty of people to be culturally sensitive, statement #122 also emphasized that the user group (food industry) waste less to protect the environment, and statement #124 called for the upgrade of the infrastructure to resolve the overcrowded campus issue. These examples of misinterpretation appeared to affect the insights. Specifically, these misinterpretations appear to lead to a misunderstanding of the problems and current issues specific to the insights for the users.
Insights
A good insight provides the result of meeting the needs, which should be based on the empathy (Gibbons, 2019). It provides the goals user groups can accomplish by solving the current needs, among the multiple possible solutions (Pressman, 2018). In terms of insights, both the high parameter value statements and the low parameter value statements mostly provided the current problem without resolving their current needs, except for statements #2, #3, #5, and #120. These statements provided the positive side the user group could achieve when finding the appropriate solution of the user needs. However, other statements failed to meet this criterion and got high parameter scores regardless of the contents of their insights. For instance, the statement #1 proposed “currently the users lack motivation and different levels of complexity within the class environment” as their insights. However, this was the problem the current situation reveals, not the goal the user group (the school of aviation and transportation technology) are trying to accomplish. The low parameter value statements provided positive goals the user group could achieve but showed the lower parameter value compared to the statement #1. Based on these findings it appeared that, when judging the POV statements, there was a high chance the students did not take the notion of good insights into account. Thus, in terms of insights, the parameter value was not always aligned with the actual quality of the insights.
Summary of the Findings From Construct Validity Analysis
Table 7 provides the summary of the findings from construct validity analysis.
Criterion Validity of Adaptive Comparative Judgment
Concurrent Validity of Adaptive Comparative Judgment
To measure concurrent validity, a correlation was run between the parameter values from conducting the peer reviewed ACJ assessment and the instructors’ rubric based grade assignments on the POV statements. The peer-evaluated ACJ was not significantly correlated (r = 0.08, p = 0.51) with graders’ grading based on rubric. Therefore, the potential concurrent validity of peer-evaluation using ACJ with POV statements is not supported by these results in the context of design thinking.
Predictive Validity of Adaptive Comparative Judgment
As seen in Table 8, A simple linear regression was calculated to predict grades of final deliverables (Assessment 3) based on the parameter values of peer-evaluated ACJ (Assessment 1). A significant regression was found (F (1, 575) = 63.057, p < 0.001), with an
Discussion
Our research questions guiding the inquiry were: 1) What is the construct validity of ACJ? Does peer-reviewed ACJ reflect general criteria of good POV statements? 2) What is the criterion validity of ACJ? By doing so, this study aimed to validate peer-evaluated ACJ in the design thinking education context. First, this study analyzed ten high parameter value statements and ten low parameter value statements based on the criteria of “good” POV statements (Interaction Design Foundation, 2020; Rikke Friis and Teo Yu, 2020) to examine the construct validity of ACJ. Second, this study examined criterion validity: Concurrent validity and predictive validity. Concurrent validity was studied using correlation between the parameter values and grades on the same POV assignment. Then, the study on the predictive validity was followed to see the parameter values on POV statement can predict future achievement of students, the grades of final deliverables.
The results revealed that peer-evaluated ACJ demonstrated construct validity. The parameter values reflect the quality of POV statements in terms of content structure, needs, user, and insights. The POV statements with higher parameter values showed better quality compared to the POV statements with lower parameter values. This finding is aligned with the findings from previous studies, which reported that ACJ completed by students can be a sound measure for evaluation of self and peer work (Jones and Alcock, 2014; Bartholomew et al., 2020a). Further, the results suggested that peer-evaluated ACJ had predictive validity, but not concurrent validity. When assessing the same POV statements, the results of peer-evaluated ACJ (parameter values) and rubric-based grading by instructors did not show significant correlation. However, the results of peer-evaluated ACJ moderately predicted students’ final grades in project 3.
As mentioned in previous studies, peer-evaluated ACJ is not proficient nor professional enough compared to instructors’ ACJ (Jones and Alcock, 2014). This may potentially affect the lack of correlation between peer-evaluated ACJ and rubric-based grading of instructors. The lack of correlation between peer evaluated ACJ results and the instructors’ rubric based grading may potentially be due to the distributions of the variables as opposed to a lack of concurrent validity. We note that the instructors’ rubric based scores are negatively skewed—which we attribute to the criterion-referenced evaluation. Thus, many POV statements may have scored high and similarly to each other on the rubric while in fact there was a noticeable difference between them as discussed in our criterion validity analysis. The ACJ approach yields a norm referenced output which includes a normal distribution regardless of the POV statements meeting the quality standards (or not).
ACJ offers researchers and practitioners in design thinking an effective quality assessment tool that is valid and reliable. As could be seen in the comparison between two groups (i.e., POV statement with high parameter values and POV statements with low parameter values), the results of ACJ displayed the quality of student assignments in a more conspicuous way. The outlier POV statements, such as those generated by teams who failed to progress or high-achiever groups were more notable when using the ACJ, due to its rank system. Early detection of struggling students (or groups) is important for both supporting student’s academic achievement in following task and keeping students from dropping out. Instructors could provide timely educational intervention to the student groups who received low parameter values in their task. For instance, if the instructor could support student groups who were struggling in POV statement, he or she could facilitate iteration and revision before student group make a progress using poor-quality POV statement, which might deleteriously affect following design thinking process. Additionally, instructors also could benefit from evaluating the quality of formative assessment during the design projects because goal-oriented, competitive students who were interested in developing one’s project in a more excellent manner would be motivated from the results of ACJ.
This study is not without limitations. First, while ACJ provided reliable and valid assessment method, the parameter value highly depends on the relative quality/level of the objects which were being assessed compared. If everyone performs well in the assignment, some students will get low parameter value and rank although the submission successfully meet overall criteria of good POV statements. Therefore, educators should bear the learning objectives and expected outcomes in mind when using ACJ and pay attention to the difference between the higher and lower ranked items. Second, the goal of assessment should be clarified. The rubric based assessment yielded a measure comparing work against a minimum standard where every team could have succeeded. The ACJ measure provided a rank order where one team’s POV was strongest, while another weakest. This means that both the strongest and weakest POV’s may or may not have met the minimum standards for a good POV statement. Further, peers are students and may not be as proficient as trained graduate students or instructors though they were nearly finished with the course at the time of assessment and the previously-noted work has pointed to the potential for students to complete judgments similarly to experts.
Future Implications
We suspect that an additional benefit of ACJ during the design thinking process was the opportunity for students to learn from both 1) the judgment process and 2) the POV statement examples of their teammates. During the comparative judgment of the POV statements, students had to cognitively internalize criteria to select “better” POV statement and applied those perceptions of quality. Also, the process required students to take a careful look at other students’ works as examples of POV statements. Examples resemble the given task and illustrate how the POV-writing task can be completed in the form of near transfer (Eiriksdottir and Catrambone, 2011). Studies revealed that simply being exposed to good examples did not lead to actual transfer (e.g., specify the criteria of good POV statement, explicitly articulate the principles of good POV statement, produce a good POV statement based on what student(s) learn from the POV statements) because learners often do not actively engage in cognitive strategies which help them learning better (Eiriksdottir and Catrambone, 2011). In other words, simply providing good POV examples to the students may not lead to the ability to judge or produce a good POV statement, because students did not use the knowledge from the examples to direct their POV judging/writing process. Educators who were interested in implementing ACJ in the course were required to adopt teaching strategies to enhance transfer of learning from examples such as emphasizing subgoals (Catrambone, 1994; Atkinson et al., 2000) (e.g., articulate main components of POV statements, narrow down the user, set insights as ultimate goal of users), self-explanation (e.g., add detailed explanation about their judging criteria) (Anderson et al., 1997) and group discussion (Olivera and Straus, 2004; Van Blankenstein et al., 2011) (e.g., discuss comparative judgement criteria with peers).
Data Availability Statement
The datasets presented in this article are not readily available because data is restricted to use by the investigators as per the IRB agreement. Requests to access the datasets should be directed to bm1lbnR6ZXJAcHVyZHVlLmVkdQ==.
Ethics Statement
The studies involving human participants were reviewed and approved by the Purdue University Institutional Review Board. Written informed consent for participation was not required for this study in accordance with the national legislation and the institutional requirements.
Author Contributions
NM contributed to the research implementation and methodology of this project. WL contributed to the writing, literature review, and statistical analysis. SB contributed to the overall research design and expertise in adaptive comparative judgment.
Funding
This material is based on work supported by the National Science Foundation under Grant Number DRL-2101235.
Conflict of Interest
The authors declare that the research was conducted in the absence of any commercial or financial relationships that could be construed as a potential conflict of interest.
Publisher’s Note
All claims expressed in this article are solely those of the authors and do not necessarily represent those of their affiliated organizations, or those of the publisher, the editors and the reviewers. Any product that may be evaluated in this article, or claim that may be made by its manufacturer, is not guaranteed or endorsed by the publisher.
References
Anderson, J. R., Fincham, J. M., and Douglass, S. (1997). The Role of Examples and Rules in the Acquisition of a Cognitive Skill. J. Exp. Psychol. Learn. Mem. Cogn. 23, 932–945. doi:10.1037//0278-7393.23.4.932
Atkinson, R. K., Derry, S. J., Renkl, A., and Wortham, D. (2000). Learning from Examples: Instructional Principles from the Worked Examples Research. Rev. Educ. Res. 70, 181–214. doi:10.3102/00346543070002181
Atman, C. J., Kilgore, D., and McKenna, A. (2008). Characterizing Design Learning: A Mixed-Methods Study of Engineering Designers' Use of Language. J. Eng. Educ. 97, 309–326. doi:10.1002/j.2168-9830.2008.tb00981.x
Bartholomew, S. R., Jones, M. D., Hawkins, S. R., and Orton, J. (2021). A Systematized Review of Research with Adaptive Comparative Judgment (ACJ) in Higher Education. Int. J. Technol. Des. Educ., 1–32. doi:10.5296/jet.v9i1.19046
Bartholomew, S. R., Mentzer, N., Jones, M., Sherman, D., and Baniya, S. (2020a). Learning by Evaluating (LbE) through Adaptive Comparative Judgment. Int. J. Technol. Des. Educ. 2020, 1–15. doi:10.1007/s10798-020-09639-1
Bartholomew, S. R., Ruesch, E. Y., Hartell, E., and Strimel, G. J. (2020b). Identifying Design Values across Countries through Adaptive Comparative Judgment. Int. J. Technol. Des. Educ. 30, 321–347. doi:10.1007/s10798-019-09506-8
Bartholomew, S. R., Strimel, G. J., and Yoshikawa, E. (2019). Using Adaptive Comparative Judgment for Student Formative Feedback and Learning during a Middle School Design Project. Int. J. Technol. Des. Educ. 29, 363–385. doi:10.1007/s10798-018-9442-7
Bisson, M.-J., Gilmore, C., Inglis, M., and Jones, I. (2016). Measuring Conceptual Understanding Using Comparative Judgement. Int. J. Res. Undergrad. Math. Ed. 2, 141–164. doi:10.1007/s40753-016-0024-3
Borrego, M., Douglas, E. P., and Amelink, C. T. (2009). Quantitative, Qualitative, and Mixed Research Methods in Engineering Education. J. Eng. Educ. 98, 53–66. doi:10.1002/j.2168-9830.2009.tb01005.x
Catrambone, R. (1994). Improving Examples to Improve Transfer to Novel Problems. Mem. Cognit. 22, 606–615. doi:10.3758/bf03198399
Chapman, V. G., and Inman, M. D. (2009). A Conundrum: Rubrics or Creativity/metacognitive Development? Educ. Horiz., 198–202.
Clemens, N. H., Ragan, K., and Christopher, P. (2018). “Predictive Validity,” in The SAGE Encyclopedia of Educational Research, Measurement, and Evaluation. Editor B. B. Frey (Thousand Oaks: California: SAGE), 1289–1291.
Coenen, T., Coertjens, L., Vlerick, P., Lesterhuis, M., Mortier, A. V., Donche, V., et al. (2018). An Information System Design Theory for the Comparative Judgement of Competences. Eur. J. Inf. Syst. 27, 248–261. doi:10.1080/0960085x.2018.1445461
Dam, R., and Siang, T. (2018). Design Thinking: Get Started with Prototyping. Denmark: Interact. Des. Found.
Dochy, F., Gijbels, D., and Segers, M. (2006). “Learning and the Emerging New Assessment Culture,” in Instructional Psychology: Past, Present, and Future Trends. Editors L Verschaffel, F Dochy, M. Boekaerts, and S. Vosniadou (Amsterdam: Elsevier), 191–206.
Dym, C. L., Agogino, A. M., Eris, O., Frey, D. D., and Leifer, L. J. (2005). Engineering Design Thinking, Teaching, and Learning. J. Eng. Educ. 94, 103–120. doi:10.1002/j.2168-9830.2005.tb00832.x
Eiriksdottir, E., and Catrambone, R. (2011). Procedural Instructions, Principles, and Examples: How to Structure Instructions for Procedural Tasks to Enhance Performance, Learning, and Transfer. Hum. Factors 53, 749–770. doi:10.1177/0018720811419154
Erickson, J., Lyytinen, K., and Siau, K. (2005). Agile Modeling, Agile Software Development, and Extreme Programming. J. Database Manag. 16, 88–100. doi:10.4018/jdm.2005100105
Forman, J., and Damschroder, L. (2007). “Qualitative Content Analysis,” in Empirical Methods For Bioethics: A Primer Advances in Bioethics. Editors L. Jacoby, and L. A. Siminoff (Bingley, UK: Emerald Group Publishing Limited), 39–62. doi:10.1016/S1479-3709(07)11003-7
Gettens, R., Riofrío, J., and Spotts, H. 2015, “Opportunity Thinktank: Laying a Foundation for the Entrepreneurially Minded Engineer.” in ASEE Conferences, Seattle, Washington, June 14-17, 2015. doi:10.18260/p.24545
Gettens, R., and Spotts, H. E. (2018). “Workshop: Problem Definition and Concept Ideation, an Active-Learning Approach in a Multi-Disciplinary Setting”, in ASEE Conferences, Glassboro, New Jersey, July 24-26, 2018. Available at: https://peer.asee.org/31440.
Gibbons, S. (2019). User Need Statements: The ‘Define’ Stage in Design Thinking. Available at: https://www.nngroup.com/articles/user-need-statements/.
Haolin, Z., Alicia, B., and Gary, L. (2019). “Full Paper: Assessment of Entrepreneurial Mindset Coverage in an Online First Year Design Course.” in 2019 FYEE Conference, Penn State University, Pennsylvania. July 28-30, 2019
Hoge, R. D., and Butcher, R. (1984). Analysis of Teacher Judgments of Pupil Achievement Levels. J. Educ. Psychol. 76, 777–781. doi:10.1037/0022-0663.76.5.777
Hsieh, H. F., and Shannon, S. E. (2005). Three Approaches to Qualitative Content Analysis. Qual. Health Res. 15, 1277–1288. doi:10.1177/1049732305276687
Hubley, A. M., and Zumbo, B. D. (2011). Validity and the Consequences of Test Interpretation and Use. Soc. Indic. Res. 103, 219–230. doi:10.1007/s11205-011-9843-4
Interaction Design Foundation (2020). Point of View - Problem Statement. Available at: https://www.interaction-design.org/literature/topics/problem-statements.
Jones, I., and Alcock, L. (2014). Peer Assessment without Assessment Criteria. Stud. Higher Edu. 39, 1774–1787. doi:10.1080/03075079.2013.821974
Jones, I., Swan, M., and Pollitt, A. (2015). Assessing Mathematical Problem Solving Using Comparative Judgement. Int. J. Sci. Math. Educ. 13, 151–177. doi:10.1007/s10763-013-9497-6
Jonsson, A., and Svingby, G. (2007). The Use of Scoring Rubrics: Reliability, Validity and Educational Consequences. Educ. Res. Rev. 2, 130–144. doi:10.1016/j.edurev.2007.05.002
Karjalainen, J. (2016). “Design Thinking in Teaching: Product Concept Creation in the Devlab Program”, European Conference on Innovation and Entrepreneurship, Karjalainen, Janne, September 18, 2016. (Academic Conferences International Limited), 359–364.
Kernbach, S., and Nabergoj, A. S. (2018). “Visual Design Thinking: Understanding the Role of Knowledge Visualization in the Design Thinking Process”, 2018 22nd International Conference Information Visualisation (IV), Fisciano, Italy, July 10-13, 2018 (IEEE), 362–367. doi:10.1109/iv.2018.00068
Lammi, M., and Becker, K. (2013). Engineering Design Thinking. J. Technol. Educ. 24, 55–77. doi:10.21061/jte.v24i2.a.5
Lindberg, T., Meinel, C., and Wagner, R. (2010). “Design Thinking: A Fruitful Concept for IT Development?” in Design Thinking. Understanding Innovation (Berlin: Springer), 3–18. doi:10.1007/978-3-642-13757-0_1
Longtin, S. E. (2014). Using the College Infrastructure to Support Students on the Autism Spectrum. J. Postsecond. Educ. Disabil. 27, 63–72.
Lunz, M. E., and Stahl, J. A. (1990). Judge Consistency and Severity across Grading Periods. Eval. Health Prof. 13, 425–444. doi:10.1177/016327879001300405
Lunz, M. E., Wright, B. D., and Linacre, J. M. (1990). Measuring the Impact of Judge Severity on Examination Scores. Appl. Meas. Edu. 3, 331–345. doi:10.1207/s15324818ame0304_3
Mahboub, K. C., Portillo, M. B., Liu, Y., and Chandraratna, S. (2004). Measuring and Enhancing Creativity. Eur. J. Eng. Edu. 29, 429–436. doi:10.1080/03043790310001658541
Mayring, P. (2015). “Qualitative Content Analysis: Theoretical Background and Procedures,” in Approaches to Qualitative Research in Mathematics Education (Berlin: Springer), 365–380. doi:10.1007/978-94-017-9181-6_13
Messick, S. (1994). The Interplay of Evidence and Consequences in the Validation of Performance Assessments. Educ. Res. 23, 13–23. doi:10.2307/1176219
National Academy of Engineering (2008). Grand Challenges for Engineering. Available at: http://www.engineeringchallenges.org/challenges.aspx.
O’Leary-Kelly, S. W., and Vokurka, R. J. (1998). The Empirical Assessment of Construct Validity. J. Oper. Manag. 16, 387–405.
Olivera, F., and Straus, S. G. (2004). Group-to-Individual Transfer of Learning: Cognitive and Social Factors. Small Group Res. 35, 440–465. doi:10.1177/1046496404263765
Palisse, J., King, D. M., and MacLean, M. (2021). Comparative Judgement and the Hierarchy of Students' Choice Criteria. Int. J. Math. Edu. Sci. Tech., 1–21. doi:10.1080/0020739x.2021.1962553
Pollitt, A. (2012a). Comparative Judgement for Assessment. Int. J. Technol. Des. Educ. 22, 157–170. doi:10.1007/s10798-011-9189-x
Pollitt, A. (2015). On ‘Reliability’ Bias in ACJ. Camb. Exam Res. 10, 1–9. doi:10.13140/RG.2.1.4207.3047
Pollitt, A. (2012b). The Method of Adaptive Comparative Judgement. Assess. Educ. Principles, Pol. Pract. 19, 281–300. doi:10.1080/0969594x.2012.665354
Potter, W. J., and Levine‐Donnerstein, D. (1999). Rethinking Validity and Reliability in Content Analysis. J. Appl. Commun. Res. 27, 258–284. doi:10.1080/00909889909365539
Pressman, A. (2018). Design Thinking: A Guide to Creative Problem Solving for Everyone. Oxfordshire: Routledge.
QCDA (1999). Importance of Design and Technology Key Stage 3. Available at: http://archive.teachfind.com/qcda/curriculum.qcda.gov.uk/key-stages-3-and-4/subjects/key-stage-3/design-and-technology/programme-of-study/index.html.
Rasch, G. (1980). Probabilistic Models for Some Intelligence and Attainment Tests. expanded edition. Chicago: The University of Chicago Press.
Reddy, Y. M., and Andrade, H. (2010). A Review of Rubric Use in Higher Education. Assess. Eval. Higher Edu. 35, 435–448. doi:10.1080/02602930902862859
Rikke Friis, D., and Teo Yu, S. (2020). Stage 2 in the Design Thinking Process: Define the Problem and Interpret the Results. Interact. Des. Found. Available at: https://www.interaction-design.org/literature/article/stage-2-in-the-design-thinking-process-define-the-problem-and-interpret-the-results.
Riofrío, J., Gettens, R., Santamaria, A., Keyser, T., Musiak, R., and Spotts, H. (2015, “Innovation to Entrepreneurship in the First Year Engineering Experience.” in ASEE Conferences, Seattle, Washington, June 14-17, 2015. doi:10.18260/p.24306
Rowsome, P., Seery, N., and Lane, D. (2013). “The Development of Pre-service Design Educator’s Capacity to Make Professional Judgments on Design Capability Using Adaptive Comparative Judgment”. in 2013 ASEE Annual Conference & Exposition, Atlanta, Georgia, June 23-26, 2013. 1–10.
Seery, N., Canty, D., and Phelan, P. (2012). The Validity and Value of Peer Assessment Using Adaptive Comparative Judgement in Design Driven Practical Education. Int. J. Technol. Des. Educ. 22, 205–226. doi:10.1007/s10798-011-9194-0
Sohaib, O., Solanki, H., Dhaliwa, N., Hussain, W., and Asif, M. (2019). Integrating Design Thinking into Extreme Programming. J. Ambient Intell. Hum. Comput 10, 2485–2492. doi:10.1007/s12652-018-0932-y
Spooren, P. (2010). On the Credibility of the Judge: A Cross-Classified Multilevel Analysis on Students’ Evaluation of Teaching. Stud. Educ. Eval. 36, 121–131. doi:10.1016/j.stueduc.2011.02.001
Strimel, G. J., Bartholomew, S. R., Purzer, S., Zhang, L., and Ruesch, E. Y. (2021). Informing Engineering Design Through Adaptive Comparative Judgment. Eur. J. Eng. Educ. 46, 227–246.
Thurstone, L. L. (1927). A Law of Comparative Judgment. Psychol. Rev. 34, 273–286. doi:10.1037/h0070288
Van Blankenstein, F. M., Dolmans, D. H. J. M., van der Vleuten, C. P. M., and Schmidt, H. G. (2011). Which Cognitive Processes Support Learning during Small-Group Discussion? the Role of Providing Explanations and Listening to Others. Instr. Sci. 39, 189–204. doi:10.1007/s11251-009-9124-7
Van Daal, T., Lesterhuis, M., Coertjens, L., Donche, V., and De Maeyer, S. (2019). Validity of Comparative Judgement to Assess Academic Writing: Examining Implications of its Holistic Character and Building on a Shared Consensus. Assess. Educ. Principles, Pol. Pract. 26, 59–74. doi:10.1080/0969594x.2016.1253542
Wilson, J., and Wright, C. R. (1993). The Predictive Validity of Student Self- Evaluations, Teachers' Assessments, and Grades for Performance on the Verbal Reasoning and Numerical Ability Scales of the Differential Aptitude Test for a Sample of Secondary School Students Attj7Ending Rural Appalachia Schools. Educ. Psychol. Meas. 53, 259–270. doi:10.1177/0013164493053001029
Wolcott, M. D., McLaughlin, J. E., Hubbard, D. K., Rider, T. R., and Umstead, K. (2021). Twelve Tips to Stimulate Creative Problem-Solving with Design Thinking. Med. Teach. 43, 501–508. doi:10.1080/0142159X.2020.1807483
Woolery, E. (2019). Design Thinking Handbook. Available at: https://www.designbetter.co/design-thinking April.
Keywords: adaptive comparative judgement, comparative judgement, design education, validity and reliability, technology and engineering education
Citation: Mentzer N, Lee W and Bartholomew SR (2021) Examining the Validity of Adaptive Comparative Judgment for Peer Evaluation in a Design Thinking Course. Front. Educ. 6:772832. doi: 10.3389/feduc.2021.772832
Received: 08 September 2021; Accepted: 09 November 2021;
Published: 16 December 2021.
Edited by:
Tine Van Daal, University of Antwerp, BelgiumReviewed by:
Jessica To, Nanyang Technological University, SingaporeRosemary Hipkins, New Zealand Council for Educational Research, New Zealand
Copyright © 2021 Mentzer, Lee and Bartholomew. This is an open-access article distributed under the terms of the Creative Commons Attribution License (CC BY). The use, distribution or reproduction in other forums is permitted, provided the original author(s) and the copyright owner(s) are credited and that the original publication in this journal is cited, in accordance with accepted academic practice. No use, distribution or reproduction is permitted which does not comply with these terms.
*Correspondence: Nathan Mentzer, bm1lbnR6ZXJAcHVyZHVlLmVkdQ==