- 1Faculty of Artificial Intelligence in Education, Central China Normal University, Wuhan, China
- 2School of Education Science and Technology, Northwest Minzu University, Lanzhou, China
- 3School of Management, University of Science and Technology of China, Hefei, China
A growing body of work has been devoted to studying teachers’ digital competence, but not much is known about how teachers’ digital competence affects online teaching behavior. Guided by the technological pedagogical content knowledge (TPACK) framework, this study investigated how teachers’ digital competence affects online teaching behavior based on Chinese primary and secondary teachers. A total of 1833 teachers completed self-report scales measuring digital competence, online teaching behavior, use intention of online teaching, and students’ online learning difficulties using the online platform Questionnaire Star. The results indicated that the level of online teaching intentions mediated the relationship between teachers’ digital competence and online teaching behavior. Students’ learning difficulties in online learning moderated the relationship between teachers’ digital competence and the intention to use online teaching, such that the relationship became stronger as students’ online learning difficulties decreased. Similarly, the indirect relationship between teachers’ digital competence and online teaching behavior was stronger at decreased levels of students’ online learning difficulties. The findings contribute to a better understanding of the impact of teachers’ digital competence on online teaching behavior.
Introduction
Online teaching has many advantages, such as it spans time and space, and enables resource sharing, diversified learning environments, openness and collaboration, and personalized learning; however, it may also cause discomfort and panic as many instructors lack the necessary skills, resources, and didactic aptitude (Lederman, 2020). Nonetheless, the coronavirus disease (COVID-19) pandemic has forced all teachers, students, and administrators to adapt and engage in a digital learning environment—essentially ending the historical debate on whether we should teach students via online platforms (Zhai, 2021).
Teachers are now being inundated and made to learn how to effectively provide engaging and authentic learning experiences for students online (Owens & Hudson, 2021). Digital technologies can present teaching content in a variety of ways (Yang and Hu, 2014). They also allow teachers to comment on student learning and master student learning information in real time, enabling the timely guidance, encouragement, feedback, and adjustment of teaching (Zhang et al., 2016). However, teachers face many challenges when trying to integrate available technology into teaching practices.
In the traditional face-to-face learning environment, teachers and students can utilize facial expressions, body language, intonations, and other emotional cues to communicate their social, emotional, and learning needs; however, these communication tools may not be as readily available in a virtual learning setting (Owens & Hudson, 2021). When preparing for online learning, instructors must consider how to support positive socio-emotional outcomes for students, such as a sense of belonging to the learning community (Delahunty et al., 2014; Crow & Murray, 2020). This is because online learning is more distributed, socially isolated, and asynchronous than its face-to-face counterpart (Lowenthal & Dennen, 2017), and students have fewer opportunities for sustained synchronous social interactivity with peers or instructors. It can reduce social presence (Crow & Murray, 2020) and risk leaving students feeling anonymous, unvalued, and demotivated (Delahunty et al., 2014; Plante & Asselin, 2014). Without guided preparation for online teaching, students are likely to be disrupted and may end up with limited engagement and gains.
This research utilizes technological pedagogical content knowledge (TPACK) as a framework to investigate the impact of digital competence on online teaching behavior. TPACK highlights teachers’ knowledge of technology (about specific tools, software, and hardware), pedagogy (about how to manage, instruct, and guide students), and content (about the discipline or subject matter) (Mishra and Koehler, 2006). Meanwhile, TPACK emphasizes that two actors (i.e., the teacher and student), and the characteristics of teachers (such as motivation and beliefs) as well as students (such as self-management and digital literacy), affect the implementation and effect of teaching and learning (Porras-Hernández and Salinas-Amescua, 2013).
As discussed, this research focuses on the relationship between digital competence and online teaching behavior (see Figure 1). First, teaching behavior is an important manifestation of teaching methods (Mishra & Koehler, 2006). It refers to all the related behaviors that teachers use to stimulate, maintain, and promote student learning when teaching (Li, 2005), and includes primary teaching behaviors (e.g., demonstration and guidance behavior), secondary teaching behaviors (e.g., stimulating student learning motivation, training student learning motivation, and positive teacher expectations), and management behavior (e.g., discussion, behavior problem management, learning resource management, and classroom time management; Zhao et al., 2019; Shi & Cui, 1999). By showing high expectations of students, giving students clear and continuous feedback, and providing students with varied, challenging, interesting, and meaningful tasks (Fredricks et al., 2016), teachers can understand the course learning progress and problems encountered by learners, to better carry out their teaching. At the same time, students will be encouraged to develop good learning habits (Slater et al., 2012), which in turn will generate adequate learning behaviors (Chai et al., 2011; Cooper, 2014) and significantly increase academic engagement (Zhang et al., 2016; Li et al., 2020; Li et al., 2021). However, to transfer the traditional classroom online, teaching behaviors also need to be adjusted according to the characteristics of online teaching. Online teaching places new demands on teachers’ teaching behaviors, for instance, emphasizing the use of digital technologies and resources to convey special emotions to students, effective interaction, and timely, high-quality feedback (Cai, 2021). Previous research has found that teachers are likely to focus primarily on successfully transitioning face-to-face learning and activities to the online context (Hill, 2020). Therefore, it is necessary to explore ways to improve teachers’ online teaching behaviors.
Second, teachers’ digital competence may be an essential factor in online teaching behavior. Online teaching in a virtual environment is not only a change in teaching form but is also deep integration of educational technology and teaching methods and strategies. Teachers have to be digital competent because if not, they will be unable to use digital technology or resource in their day-to-day educational practice (Wastiau et al., 2013; Kabakci Yurdakul and Coklar, 2014). Therefore, teachers need some competencies: the competence to use technologies as a teacher and to decide which technology to use for a specific teaching purpose (Starkey, 2019).
Third, the intention to use online teaching plays an important role in the relationship between teachers’ digital competence and online teaching behavior. TPACK explicitly mentions that teaching competence in the model is influenced by technical competence (Mishra and Koehler, 2006), however, ignored the role of cognitive aspects. The improved TPACK model posits that teachers’ beliefs and cognitive characteristics play an important role between technological competence and instructional competence (Porras-Hernández and Salinas-Amescua, 2013). Mooij and Smeets (2001) further state that if teachers do not trust their skills or competences to handle computers, they may be reluctant to introduce technology into their classroom. In other words, the integration of technology and teaching depends on the teacher’s competence to master and use technology, and whether the teacher consciously uses technology to meet the teaching purpose. Therefore, this study sought to use online teaching as a mediation variable to investigate the impact of teachers’ digital competence on online teaching behavior.
Finally, teachers’ digital competence is related to the characteristics of the situation in which they are placed. Porras-Hernández and Salinas-Amescua, 2013 consider that the characteristics of students (e.g., digital competence, learning motivation, knowledge of student thinking and learning) have an impact on teaching behavior. At the same time, knowledge of student characteristics is fundamental to teaching (Mishra & Koehler, 2006). Considering the differences in students’ digital abilities, it is inferred that students’ digital competence will affect the role of teachers’ digital competence. Therefore, this study selected the students’ online learning difficulties perceived by teachers as the moderating variable, which could moderate the association between teachers’ digital competence and the intention to use online teaching.
Hypotheses Development
Teachers’ Digital Competence and Online Teaching Behavior
Teachers’ digital competence which is not how to use digital technology, but how to implement it for teaching and learning, refers to teachers’ competence to acquire, identify, and organize digital information related to the course (Knutsson et al., 2012); to communicate such that teachers and students may share, interact, participate, and collaborate; and to identify online resources and use technology to solve problems. In many countries, basic and higher education are developing in the direction of digitalization. It has become a common phenomenon for teachers and students to learn in various online environments (Viberg et al., 2020). Thus, using and mastering digital learning tools have become the basic literacy requirements of teachers and students (Julien & Barker, 2009). In the current COVID-19 pandemic, online teaching has become very common, and digital capabilities have become even more important for teachers.
TPACK points out that a lack of digital competence to identify online resources and use technology to solve problems directly leads to teachers’ difficulty in integrating information resources into teaching design and improving classroom structure. Many studies based on digital literacy have shown that the improvement of teachers’ digital literacy contributes to the effective integration of technology, teaching in complex learning environments (e.g., online learning, mixed online and offline learning; Ge & Han, 2017), infiltrating technology into lesson preparation, extracurricular tutoring, management (Lan et al., 2020), and providing students with personalized learning. Training aimed at improving teachers’ digital competence (such as with technology and resources) can significantly improve teachers’ competence to integrate technology and teaching practice (Santos & Castro, 2021). For example, a study by Bicer and Capraro, 2016 found that by providing teachers with continuous support for the integration of technology and mathematics courses, students’ mathematics performance could be significantly improved.
However, the digital competence of teachers in most countries is very limited (Bossu et al., 2007; Phuapan et al., 2016; Nguyen et al., 2020). More than half teachers have never used digital information or technology in the fields of curriculum development and design, teaching resources, classroom management, providing feedback, and teaching examination, among others (Perifanou et al., 2021). When teachers’ acceptance of technology is low, classroom teaching is difficult to integrate with technology (Legris et al., 2003; Jose, 2017; Bai & Gu, 2020)—resulting in the incompetence of technology to produce structural changes in teaching (Lei, 2018; Tondeur et al., 2019). Based on the theoretical and empirical evidence discussed, this study proposes the following hypothesis:
Hypothesis 1: Teachers’ digital competence is positively related to teachers’ online teaching behavior.
Mediating Effects of Use Intention of Online Teaching
Teachers’ characteristics are an important factor in the process of teacher technology integration (Porras-Hernández and Salinas-Amescua, 2013; Zhang, 2014). TPACK emphasizes the teacher’s main role as an actor in the overall theoretical framework and emphasizes that merely introducing technology to the educational process is not enough. This issue has received much attention regarding teachers’ knowledge of how to appropriately introduce technology into their teaching (Mishra and Koehler, 2006). Teachers’ intentions to use online teaching are particularly important.
The intention to use online teaching refers to the possibility that teachers rely on digital technology and resources to carry out online teaching, which is the core predictor of behavior and determines teachers’ degree of effort in integrating technology with teaching (Bai & Gu, 2020). Teachers’ intention to use digital technologies and resources is influenced by their knowledge of technology affordances. For example, Zeng and Zheng (2019) pointed out that teachers’ digital competence can reduce their cognitive load and anxiety about the use of digital technology and resources (Zhu et al., 2018), and increase their perception of the usefulness and ease of digital technology and resources—thus enhancing their intention to use online teaching (Bai & Gu, 2020).
Contrarily, low digital competence makes it more difficult for teachers to acquire, use, and integrate digital resources (Huang, 2011) and realize the deep integration of technology and teaching tasks (Zhu et al., 2018)—thereby reducing their behavior and willingness to carry out online teaching. Thus, this study proposes the following hypothesis:
Hypothesis 2: Teachers’ digital competence is positively related to their intention to use online teaching.
As for the third hypothesis, teachers tend to use technologies in ways that are consistent with their own epistemological and pedagogical beliefs and use those technologies that lie within the limits of their knowledge (Porras-Hernández et al., 2010). We propose that the intention to use online teaching can predict teachers’ online teaching behavior. As a tendency for individuals to perform specific behaviors, teachers’ intention to use online teaching is an important factor that influences their choice of technology in teaching (Goodhue & Thompson, 1995; Zhang, 2018). Online teaching behavior requires teachers to think about the methods of integrating technology and teaching content independently and actively. The more positive a teacher’s attitude toward technology, the more likely he or she is to use it (Porras-Hernández and Salinas-Amescua, 2013)— which in turn increases the likelihood of continued use of the technology and resources (Davis et al., 1989; Taylor & Todd, 1995). The intention to use online teaching is important for teachers to carry out online teaching, and may even play a decisive role in the design of teaching behavior (Zhang, 2018). Based on the above, we propose the following hypothesis:
Hypothesis 3: The intention to use online teaching plays a mediating role between teachers’ digital competence and online teaching behavior.
Moderating Effects of Students’ Online Learning Difficulties
Teachers’ digital competence does not guarantee that deep integration of technology and teaching will occur (Uerz et al., 2018). TPACK states that the impact of teachers and their knowledge of students depends on how successfully each teacher adapts to a unique context (Porras-Hernández and Salinas-Amescua, 2013). However, space and time separation of online teaching create more difficulties in teaching and learning for teachers and students, such as weak interaction between teachers and students, untimely feedback, and increased distraction for students (Wang et al., 2020; Yu & Wang, 2020). Additionally, the smooth development of online teaching also requires students to have a better digital competence, such as experience in locating and using resources (Oliver & Hannafin, 2000; Clarebout & Elen, 2006), which are essential for instructional design (Porras-Hernández and Salinas-Amescua, 2013). Therefore, online teaching requires that teachers consider students’ digital competence, attention maintenance, and self-management, among others, which are closely related to their competence to use digital technology and resources in a separate teaching environment. We refer to these factors collectively as students’ online learning difficulties.
Students’ online learning difficulties may become a prerequisite for teachers’ intention to use online teaching. In online teaching, teachers are more susceptible to the impact of student characteristics. Such as the recent COVID-19 outbreak, most colleges endeavor to make teaching online normal. However, basic education—especially in the lower levels of primary school—is difficult to carry out online as it is closely related to the characteristics of primary school students; generally, their digital skills are lacking, attention is difficult to maintain, and self-management competence is weak. Anecdotal evidence also suggests that many students who made a rapid mandated shift to online learning experienced feelings of displacement, social isolation, and demotivation (Hall & Batty, 2020; Yamin, 2020).
Teachers who assess students’ online learning difficulties are less likely to take advantage of their digital competence and have a positive attitude toward online teaching, which can lead to better online teaching behavior. Previous research has also shown that while teachers have lower digital competence, if their students’ own qualities (e.g., digital literacy and self-management) are better, teachers can also achieve deep integration of digital technology and teaching (Porras-Hernández and Salinas-Amescua, 2013). A study on the teaching behavior of pre-service teachers shows that the expert evaluation of the effectiveness of teaching plans at integrating pre-teachers’ technology and teaching is much lower than that of pre-service teachers’ self-evaluation (Chai et al., 2011). To some extent, this shows that teaching is the result of multiple interactions between teachers, students, and the learning environment, and teaching behavior not only depends on teaching methods but also the importance of perceived student characteristics. Based on this, we propose the following hypotheses:
Hypothesis 4: Students’ online learning difficulties will moderate the relationship between teachers’ digital competence and the intention to use online teaching, such that the relationship will be stronger when the online learning difficulties perceived by teachers are decreased.
Hypothesis 5: Students’ online learning difficulties will play a moderating role in regulating the mediating effect of teachers’ digital competence on online teaching behavior. The mediation effect will be weaker when the students are perceived to have increased online learning difficulties.
Materials and Methods
Participants and Procedure
All research processes were reviewed and approved by the survey and behavioral research ethics committee of the author’s university. A convenience sampling method was used to select 23 primary or secondary schools in two cities in Hubei Province, China. Local education authorities were commissioned through the primary and secondary school teachers’ QQ and WeChat groups to send a questionnaire link using the online platform Questionnaire Star. The questionnaire was automatically refreshed by the system after being filled out. Additionally, there was also an informed consent form signed by the participants, which clearly stated the purpose of the study and emphasized the principles of anonymity and confidentiality of the participants’ information. Participants could simply refuse to participate in the study after reading the informed consent form. A total of 2,440 teachers answered the questionnaire. A total of 607 questionnaires were excluded because the participants’ answer time was less than 5 s, which showed a clear tendency to respond hastily (e.g., extreme response tendency), or they did not belong to an elementary or junior high school. Thus, there were 1,833 valid questionnaires, representing an effective recovery rate of 75.12%. There were 712 men (38.8%), 1,121 women (61.2%), 1,091 teachers of primary schools (59.6%), and 742 teachers of secondary schools (40.5%). The distribution of teaching experience was as follows: 466 (25.4%) had a teaching experience of less than 5 years, 345 (18.8%) had a teaching experience of 6–15 years, and 1,022 (55.8%) had a teaching experience of more than 15 years. In terms of the spatial distribution of the schools involved, 1,151 (62.8%) and 545 (29.7%) participants were from cities/townships and villages, respectively. The educational background of the teachers was as follows: 67 (3.7%) did not attend college, 618 (33.7%) had undergone 3 years at college, and 1,118 (61%) had undergone 4 years at university.
Measurements
Teacher’s Digital Competence
This study used a teacher’s digital competence scale, which was revised from the college students’ digital competence scale compiled by Hong and Jeong (2018). It contained a total of 11 items and encompassed three dimensions: 1) using digital technology to interact (e.g., I can organize students to carry out group activities through digital technology or resources), 2) digital resource search and integration capabilities (e.g., I can integrate digital resources in the course design process), and 3) digital technology or resource application in teaching (e.g., I can use digital technology to innovate teaching evaluation methods). Answers were scored on a 5-point Likert scale (1 = “fully disagree”; 5 = “fully agree”). The model fitting results of the confirmatory factor analysis were good (χ2/df = 478.01/41 = 11.66, CFI = 0.96, TLI = 0.94, RMSEA = 0.076, SRMR = 0.032). In the current study, Cronbach’s α for using digital technology to interact, having digital resource search and integration capabilities, and digital technology or resources application in teaching were 0.88, 0.84, and 0.90, respectively. Cronbach’s α for the total scale was 0.95. The homogeneity coefficient (HC) of the scale was 0.81. The composite relicompetence (CR) for using digital technology to interact, having digital resource search and integration capabilities, and digital technology or resource application in teaching were 0.97, 0.87, 0.85, respectively. The CR for the total scale was 0.97.
Teacher’s Online Teaching Behavior
Based on the research of Shi and Cui (1999) and Zhao et al. (2019) on teachers(online teaching behavior, we proposed that the Teacher’s Online Teaching Behavior Scale contained three dimensions: 1) main teaching behavior (e.g., I ask questions that are appropriate for the student’s level in the online classroom), 2) behaviors that contribute to teaching (e.g., I can guide students to pay attention to learning), and 3) management behavior (e.g., I can make students abide by online classroom rules). The scale contained a total of 11 items and answers were scored on a 5-point Likert scale (1 = “fully disagree”; 5 = “fully agree”). The model fitting results of the confirmatory factor analysis were good (χ2/df = 157.78/41 = 3.85, CFI = 0.99, TLI = 0.98, RMSEA = 0.039, SRMR = 0.021). The load of each topic in the corresponding dimension was good (0.67–0.88). In the current study, Cronbach’s α for the main teaching behavior, behaviors that contribute to teaching, and management behavior were 0.873, 0.905, and 0.859, respectively. Cronbach’s α for the total scale was 0.944. The HC of the scale was 0.85. The CR for main teaching behavior, behaviors that contribute to teaching and management behavior were 0.85, 0.72, 0.87, respectively. The CR for the total scale was 0.95.
Teacher’s Use Intention of Online Teaching
This study used a teacher’s intention to use the online teaching scale, which was revised from the Intention to Use Digital Technology Scale compiled by Taylor and Todd (1995). The scale, which measured teachers’ intention to use online teaching, consisted of four items (e.g., If conditions permit, I will be more inclined to carry out online teaching). Answers were scored on a 5-point Likert scale (1 = “fully disagree”; 5 = “fully agree”). The model fitting results of the confirmatory factor analysis were good (χ2 = 4.98, df = 2, χ2/df = 1.49, CFI = 1.00, TLI = 0.99, RMSEA = 0.03, SRMR = 0.008). The load of each topic in the corresponding dimension was good (0.66–0.91). In the current study, Cronbach’s α was 0.86.
Teacher’s Perceived Online Learning Difficulties of Students
Using the results of existing literature as well as open interviews, we compiled a questionnaire about students’ online learning difficulties. The questionnaire consisted of five items. The guidelines of the scale stated: “The following lists the common difficulties encountered by students in online teaching.” The items were: “inattention,” “cannot interact effectively with the teacher,” “cannot attend class on time,” “insufficient preview and review,” and “equipment network failure.” The answer to each question was either “yes” or “no.” “Yes” is was as 1 point, and “no” was counted as 0 points. The model fitting results of the confirmatory factor analysis were good (χ2/df = 9.615/5 = 1.92, CFI = 0.97, TLI = 0.95, RMSEA = 0.022, SRMR = 0.015). The scores of the five items were added together to form a total score. The higher the score, the more online learning problems the teacher perceives for his or her students.
Control Variables
We included the teacher’s teaching age as a control variable because it may affect the teacher’s technical level (Hu et al., 2021). Research has shown that the higher the teaching age, the worse the teacher’s competence to integrate information technology into teaching (Chen and Lu, 2020). We also controlled for the location of the school (county/township or village). Although there is no difference in hardware equipment between urban and rural schools, there may be differences in teachers’ digital competence and teachers’ online teaching methods (Du, 2017). For example, urban teachers use both live streaming and synchronous interactive discussions. However, rural teachers only use interactive discussions, and mostly through asynchronous means (Chen and Lu, 2020; Hu et al., 2021). Finally, we controlled for primary/secondary schools and educational backgrounds. Studies have found that these two factors may affect the use and acceptance of information technology, as well as the integration of technology and teaching (Chen and Lu, 2020).
Analysis Procedure
First, we used the expectation-maximization (EM) algorithm to fill in the missing values. Harman’s single-factor method was used to assess the severity of common method variance (CMV) using IBM SPSS 25.0. Mplus Version 7.4 was then used to test the construct validity of the scale, with the estimation method used as the maximum likelihood estimation (Muthén and Muthén, 2012). The model fit was jointly measured using five indicators (χ2/df, CFI, TLI, RMSEA, and SRMR). The following values indicated a good fit: χ2/df < 3, CFI >0.90, TLI >0.90, RMSEA <0.08, and SRMR <0.08 (Marsh et al., 2004). IBM SPSS 25.0 was then used for calculating descriptive statistics of the data, correlation analysis, and Cronbach’s α. Finally, the SPSS Process macro developed by Hayes, 2013 was used to test the mediating and moderating effects.
Results
Test for Common Method Variance
The self-reporting method used to collect data from the study’s participants may have resulted in CMV. Thus, the testing process had to be strictly controlled. First, it was emphasized that the questionnaire results would only be used for academic research, and all information provided was kept strictly confidential. Second, the questionnaire was anonymously completed by the participants. To posttest the threat of CMV, Harman’s single-factor method was used to test for CMV (Podsakoff et al., 2003), and an unrotated exploratory factor analysis was performed on all questions. The results showed that the four factors had an initial eigenvalue greater than 1. The variance explained by the first factor was 48.34%. This was less than the critical value of 50%, indicating that the CMV was not obvious under Harman’s single-factor method (Hair et al., 1998).
Descriptive Statistics and Correlations Between Variables
The correlations between school location and the four study variables were not significant. School location and teaching experience were significantly and negatively correlated with the other three study variables, except for the insignificant correlation with students’ online learning difficulties. Education background was significantly and positively correlated with the four study variables. The other three study variables were significantly and positively correlated with each other, except for the significant negative correlation between students’ online learning difficulties and the other three study variables (Table 1).
Test for the Moderated Mediation Model
Use Intention of Online Teaching Played a Mediation Role Between Digital Competence and Online Teaching Behavior
The relationship between online teaching behavior, intention to use online teaching, and teachers’ digital competency was regressed using Model 4 (Model 4 hypothesis is a mediating effect test, which is consistent with the hypothesis model of this study) in the SPSS Process macro developed by Hayes, 2013 after controlling for educational background, teaching age, primary/secondary school, and school location. The results showed that after controlling for basic demographic information, teachers’ digital competence was a significant predictor of online teaching behavior (β = 0.68, t = 39.77, p < 0.001) (Table 2). Hypotheses 1 were tested. After the mediating variable (intention to use online teaching) entered the equation, teachers’ digital competence remained a significant predictor of online teaching behavior (β = 0.54, t = 29.92, p < 0.001) and was a significant predictor of intention to use (β = 0.27, t = 9.02, p < 0.001). Hypotheses 2 were tested. Intention to use was likewise a significant predictor of online teaching behavior (β = 0.23, t = 15.86, p < 0.001). The indirect effect was 0.136 (SE = 0.012) with a bootstrap 95% confidence interval without a value of 0 (95% CI [0.113, 0.161]), and the mediating effect accounted for 20% of the total effect. Hypotheses 3 were tested.
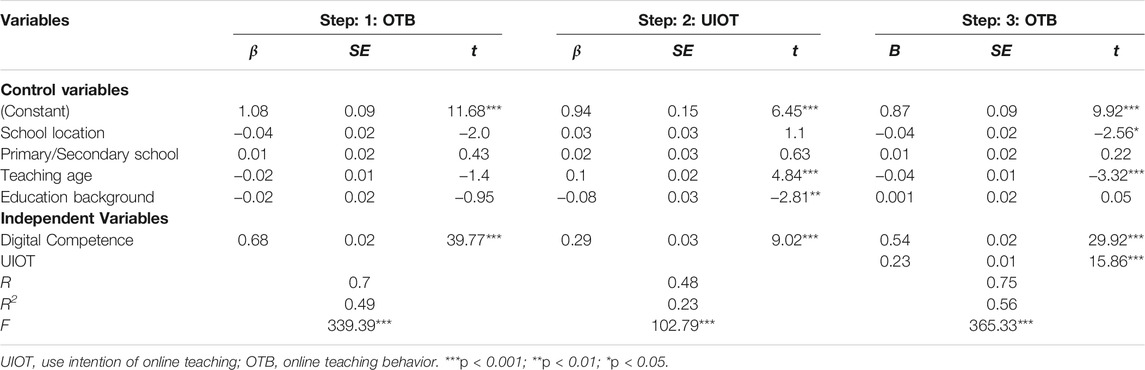
TABLE 2. Analysis of use intention of online teaching, digital competence, and online teaching behavior.
Students’ Online Learning Difficulties Played a Moderating Role in Mediating the Process From Teachers’ Digital Competence to Online Teaching Behavior
Using Model 7 in the SPSS Process macro prepared by Hayes, 2013 (Model 7 assumes the presence of moderation in the first stage of the indirect path in the mediating effect, which is consistent with the model assumed in this study), and after controlling for educational background, teaching age, school location, and school section, with students’ online learning difficulties as the moderating variable, the results showed that the moderating term of digital competency × learning online learning difficulties was a significant predictor of intention to use online instruction (β = −0.07, t = −3.11, p < 0.001) (Table 3). This indicates that online learning difficulties moderated the relationship between teachers’ digital competency and intention to use online instruction.
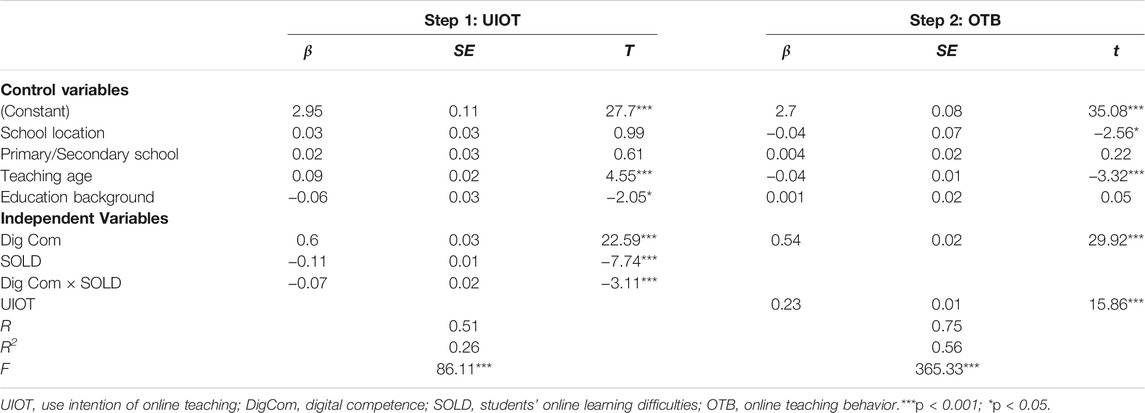
TABLE 3. Analysis of use intention of online teaching, digital competence, and online teaching behavior, students’ online learning difficulties.
A simple slope analysis using the SPSS Process 3.0 macro showed that when perceived student online learning problems were high, the effect of teachers’ digital competence on intention to use online instruction was weaker (Figure 2). Hypothesis 4 was tested.
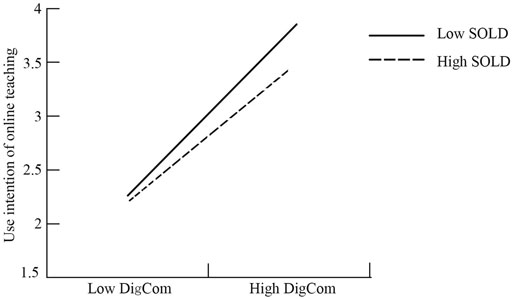
FIGURE 2. Moderating role perceived students’ online learning difficulties (SOLD) between teachers’ digital competence (DigCom) and intention to use online instruction.
Further analysis of the moderating effect of teachers’ perceived online learning difficulties of students on the indirect effect of teachers’ digital competence on teachers’ online teaching behaviors showed that the mediating effect of teachers’ online teaching use intentions was significant when students’ online learning problems took values plus or minus one standard deviation, and none of their confidence intervals contained 0 (Table 4). The indirect effect was stronger when teachers’ perceived students’ online learning difficulties were weak. Hypothesis 5 was tested.
Discussion
Summary of Results
This study draws on the TPACK theory to ascertain the relationship between teachers’ digital competence and online teaching behavior. Building on earlier research (Mishra & Koehler, 2006) and reviews (Porras-Hernández and Salinas-Amescua, 2013), we aimed to ascertain the extent to which digital competence contributes to online teaching behavior. Further, we investigated whether students’ online learning difficulties moderated the effects of digital competence on the intention to use online teaching. The theory of the TPACK model is further enriched by the interpretation of the relationship between teachers, students, pedagogy, and technology in teaching (Angeli & Valanides, 2009).
Theoretical Implications
In support of the TPACK framework, digital competence contributes to online teaching behavior. Higher digital competence among teachers is conducive to the integration of technology teaching and learning and the advantages of digital technology, such as presenting teaching content in multiple ways (Yang and Hu, 2014) and grasping students’ learning information in real time so they can guide, encourage, give feedback, and adjust teaching promptly (Zhang et al., 2016). Contrarily, when teachers are not digitally competent, difficulties will arise in personalized instructional design for online teaching—which in turn leads to the incompetence of technology to produce structural changes in teaching and learning (Jose, 2017). For example, Wang et al. (2020) noted that teachers in urban areas are more likely to use self-produced resources and live streaming, while teachers in rural areas are more likely to use resources provided at the national and county levels—which makes significant differences in teachers’ instructional design in terms of personalization of instructional resources, and can also affect students’ learning experiences. While previous studies have shown that digital competence can significantly improve teachers’ online teaching behaviors, most studies have focused on famous teachers in famous schools or massive open online course (MOOC) teaching, and have not studied the online teaching behaviors of frontline teachers. This study validated previous findings with a sample of primary and secondary school teachers.
In line with the TPACK, support for the mediating role of use intention in online teaching was evident when investigating the impact of digital competence and use intention of online teaching on online teaching behavior. The results indicated that the higher the digital competence, the better the online teaching behavior. The effect of digital competence on online teaching behavior was mediated by the intention to use online teaching. Online teaching is a reintegration of technology and teaching, which requires teachers to have an active intention to use it and actively combine the features of online teaching (e.g., richness of resources, teacher-student interaction, real-time feedback) to redesign it. Teachers must have the intention to use online teaching and actively redesign their teaching with the features of online teaching for them to have better online teaching behavior.
The TPACK theory emphasizes the role of student characteristics in the model. We argue that student characteristics can be viewed as contextual variables that influence teachers’ online instruction; that is, student factors may moderate the relationship between digital competence and intention to use online teaching. We examined the role of students’ online learning difficulties as a moderator—predicting that with decreased student online learning difficulties, the effect of digital competence on intention to use online teaching would be accentuated. Whether in an online or face-to-face setup, students are always the main subject of education, and the design of teaching behaviors for online teaching should be based on students’ characteristics as well. Yu and Wang (2020) pointed out that Student-centered classroom instruction is achieved by developing students’ competence to plan, execute, and reflect on their own. Online teaching requires not only these abilities, but also requires good digital competence and self-management.
Practical Implications
Improving Teachers’ Digital Competence to Meet the Requirements of Online Teaching
Teachers should improve their proficiency in digital technology and their competence to acquire, analyze, and integrate digital resources with teaching content to make such content more intuitive, vivid, and easily understood. Examples of this are using relevant video resources to present abstract knowledge, and using information technology to collect and analyze data on students’ learning characteristics, and adjusting and improving teaching behaviors through said data’s results (Liu G. D, 2019; Liu J. W, 2019)—all of which are inextricably linked to teachers’ digital competencies.
Cultivating Teachers’ Intention to Use Online Teaching to Promote Online Teaching Behaviors
In improving teachers’ digital literacy, while paying attention to the cultivation and improvement of their digital competence is necessary, attention should also be paid to cultivating their intention to use online teaching. Online teaching has higher requirements for teachers than traditional classroom teaching. The technology and rich digital resources brought about by the development of information technology, and different people will have different degrees of use for them. Therefore, in teacher training and teaching work, teachers should strengthen their intention to use online teaching and improve their awareness of combining information technology with their teaching activities—avoiding the mechanical transfer of offline teaching online (Zhang et al., 2020).
Improving Students’ Information Literacy to Promote Teacher-Student Online Teaching Interaction
Online teaching places more emphasis on a student-centered, which places higher demands on students’ learning behaviors and requires attention to students’ information literacy while emphasizing the strengthening of teachers’ digital capabilities and improving their intention to use online teaching. Students with high information literacy can quickly grasp and use the functions of the teaching platform and can better ask questions, discuss, and provide feedback in class. For example, students’ active completion of online reading and discussion before class can enhance the depth of classroom communication, and the discussion forum and homework review after class can provide more feedback and communication for students (Ma, 2020). Moreover, students’ increased competence to access, analyze, and integrate digital resources could also improve the depth of the discussion and knowledge taught in the teacher’s classroom.
Limitations and Future Research
This study provides unique insights into improving online teaching behaviors. However, it is not without limitations. First, although the research questions were based on a theoretical foundation, the data used was cross-sectional, making causality difficult to determine. Future research may choose to utilize experimental methods or adopt a longitudinal design to sort out the causal effects between variables. Second, the self-reporting of the respondents may have led to CMV. All data in this study were obtained from teachers’ self-assessments. However, the result of Harman’s single-factor method indicated that CMV was not obvious in this study. Nonetheless, future studies should collect data from multiple sources to address this. Third, this study focused only on the moderating role of online learning difficulties in student characteristics. However, other contextual variables influence teachers’ online teaching behaviors, such as other student characteristics (e.g., family’s socioeconomic status) and school factors (e.g., school digital infrastructure development and school digital teaching support). Thus, future research could examine other situational variables from a multilevel perspective. Finally, few studies have focused on students’ online learning difficulties, resulting in a lack of appropriate measurement instruments. We used the results of existing literature as well as open interviews, and compiled a questionnaire about students’ online learning difficulties. Future studies may consider further improvement of the instrument.
Data Availability Statement
The original contributions presented in the study are included in the article/Supplementary Material, further inquiries can be directed to the corresponding author.
Ethics Statement
The studies involving human participants were reviewed and approved by the Research Ethics Committee, Faculty of Artificial Intelligence Education, Central China Normal University. The patients/participants provided their written informed consent to participate in this study.
Author Contributions
WL: writing-original draft and formal analysis. WG: data curation, writing-review, and editing. WF: conceptualization, methodology, and investigation. CY: investigation. All authors contributed to the article and approved the submitted version.
Funding
This work was supported by Open Project Funded by the Key Laboratory of Information Technology of Chinese Ethnic Languages and Writings of the Ministry of Education (KFKT202012) and Supported by the Fundamental Research Funds for the Central Universities (31920210120).
Conflict of Interest
The authors declare that the research was conducted in the absence of any commercial or financial relationships that could be construed as a potential conflict of interest.
Publisher’s Note
All claims expressed in this article are solely those of the authors and do not necessarily represent those of their affiliated organizations, or those of the publisher, the editors and the reviewers. Any product that may be evaluated in this article, or claim that may be made by its manufacturer, is not guaranteed or endorsed by the publisher.
Supplementary Material
The Supplementary Material for this article can be found online at: https://www.frontiersin.org/articles/10.3389/feduc.2021.744950/full#supplementary-material
References
Angeli, C., and Valanides, N. (2009). Epistemological and Methodological Issues for the Conceptualization, Development, and Assessment of ICT-TPCK: Advances in Technological Pedagogical Content Knowledge (TPCK). Comput. Edu. 52, 154–168. doi:10.1016/j.compedu.2008.07.006
Bai, X. M., and Gu, X. Q. (2020). What Makes it Difficult to Use Technology to its Full Potential in the Classroom? ——A Study on the Factors Influencing Teachers' Behavioral Intention to Teach with Information Technology Based on Cognitive and Affective Perspectives. Open Edu. Res. 26, 86–94. doi:10.13966/j.cnki.kfjyyj.2020.04.010
Bicer, A., and Capraro, R. M. (2016). Longitudinal Effects of Technology Integration and Teacher Professional Development on Students' Mathematics Achievement. Eurasia J. Math. Sci. T 13, 815–833. doi:10.12973/eurasia.2017.00645a
Bossu, C., Smyth, R., and Stein, S. (2007). Academic Professional Development for Effective E-Learning: a Possible Framework for brazil. Int. J. Emerging Tech. Learn. (Ijet) 2, 48–53. http://www.online-journals.org/index.php/i-jet/article/download/139/139.
Cai, H. H. (2021). Research on the Correlation of Teachers' E-Readiness and Students'Learning Effect: the Mediation Effect of Learner Control and Academic Emotions. J. East China Normal University:Educational Sci. 7, 27–37. doi:10.16382/j.cnki.1000-5560.2021.07.003
Chai, X., Gong, S., Duan, T., Zhong, L., and Jiao, Y. (2011). A Social- Cognitive Approach to Motivational Contagion between Teacher and Student. Adv. Psychol. Sci. 19, 1166–1173. doi:10.3724/SP.J.1042.2011.01166
Chen, B., and Lu, X. Z. (2020). Are Teachers Competent to Teach in the Information Age? --A Survey from Less Developed Regions. Open Edu. Res. 5, 71–77. doi:10.13966/j.cnki.kfjyyj.2020.05.007
Clarebout, G., and Elen, J. (2006). Tool Use in Computer-Based Learning Environments: towards a Research Framework. Comput. Hum. Behav. 22, 389–411. doi:10.1016/j.chb.2004.09.007
Cooper, K. S. (2014). Eliciting Engagement in the High School Classroom. Am. Educ. Res. J. 51, 363–402. doi:10.3102/0002831213507973
Crow, J., and Murray, J. A. (2020). “Online Distance Learning in Biomedical Sciences: Community, Belonging and Presence,” in Biomedical Visualisation. Advances in Experimental Medicine and Biology. Editor P. Rea (Cham: Springer), 1235. doi:10.1007/978-3-030-37639-0_10
Davis, F. D., Bagozzi, R. P., and Warshaw, P. R. (1989). User Acceptance of Computer Technology: a Comparison of Two Theoretical Models. Manage. Sci. 35, 982–1003. doi:10.1287/mnsc.35.8.982
Delahunty, J., Verenikina, I., and Jones, P. (2014). Socio-emotional Connections: Identity, Belonging and Learning in Online Interactions. A Literature Review. Technol. Pedagogy Edu. 23, 243–265. doi:10.1080/1475939x.2013.813405
Du, Y. X. (2017). Research on the Promotion of Primary and Secondary School Teachers' Informationalized Teaching Competence Based on"Internet+. China Educ. Tech. 8, 86–92. doi:10.3969/j.issn.1006-9860.2017.08.014
Fredricks, J. A., Filsecker, M., and Lawson, M. A. (2016). Student Engagement, Context, and Adjustment: Addressing Definitional, Measurement, and Methodological Issues. Learn. Instruction 43, 1–4. doi:10.1016/j.learninstruc.2016.02.002
Ge, W. S., and Han, S. B. (2017). A Standard Framework for Teachers' Teaching Competence in the Digital Age. Mod. Distance Edu. Res. 145, 59–67. doi:10.3969/j.issn.1009-5195.2017.01.008
Gonzalez, M. J., and Gonzalez Ruiz, I. (2016). Behavioural Intention and Pre-service Mathematics Teachers' Technological Pedagogical Content Knowledge. Eurasia J. Math. Sci. T 13, 602–620. doi:10.12973/eurasia.2017.00635a
Goodhue, D. L., and Thompson, R. L. (1995). Task-technology Fit and Individual Performance. Mis Q. 19(2):213–236. doi:10.2307/249689
Hair, J. F., Anderson, R. E., Tatham, R. L., and Black, W. C. (1998). Multivariate Data Analysis. 5th ed. Upper Saddle River, NJ: Prentice-Hall.
Hall, R., and Batty, D. (2020). ‘I Can’t Get Motivated’: The Students Struggling with Online Learning. United Kingdom: The Guardian. Available at: https://www.theguardian.com/education/2020/may/04/i-cant-get-motivated-the-students-struggling-with-online-learning (Accessed May 4, 2020).
Hayes, A. (2013). Methodology in the Social Sciences: Introduction to Mediation, Moderation, and Conditional Process Analysis: A Regression‐based Approach. N.Y, Ny: Guilford Press. 51, 335–337. doi:10.1111/jedm.12050
Hill, P. (2020). Revised Outlook for Higher Ed’s Online Response to COVID-19. Retrieved from Available at: https://philo nedtech.com/revised-outlook-for-higher-eds-online-response-to-covid-19/[Accessed March 31, 2020]
Hong, A. J., and Kim, H. J. (2018). College Students' Digital Readiness for Academic Engagement (Drae) Scale: Scale Development and Validation. Asia-pacific Edu Res. 27, 303–312. doi:10.1007/s40299-018-0387-0
Hu, Y. L., Nie, J., and Gu, X. Q. (2021). From Opportunity Equity to Development Equity: A Comparative Urban- Rural Analysis of Large-Scale Online Education in Primary and Secondary Schools in China under the Epidemic. Distance Edu. China 5, 13–21. +76–77. doi:10.13541/j.cnki.chinade.2021.05.002
Huang, R. H. (2011). Education Informatization Helps Current Educational Changes:opportunities and Challenges. China Educ. Tech. 288, 36–40. doi:10.3969/j.issn.1006-9860.2011.01.005
Julien, H., and Barker, S. (2009). How High-School Students Find and Evaluate Scientific Information: a Basis for Information Literacy Skills Development. Libr. Inf. Sci. Res. 31, 12–17. Library & Information Science Research. doi:10.1016/j.lisr.2008.10.008
Kabakci Yurdakul, I., and Coklar, A. N. (2014). Modeling Preservice Teachers' TPACK Competencies Based on ICT Usage. J. Comp. Assist. Learn. 30 (4), 363–376. doi:10.1111/jcal.12049
Knutsson, O., Blåsjö, M., Hållsten, S., and Karlström, P. (2012). Identifying Different Registers of Digital Literacy in Virtual Learning Environments. Internet Higher Edu. 15, 237–246. doi:10.1016/j.iheduc.2011.11.002
Lan, G. S., Guo, Q., Zhang, Y., Kong, X. K., and Guo, X. J. (2020). Digital Literacy Framework for Educators in the European Union: Interpretation of Key Points and Insights. Mod. Distance Edu. Res. 32, 23–32. doi:10.2139/ssrn.3661054
Lederman, D. (2020). The Shift to Remote Learning: The Human Element. Retrieved from Available at: https://www.insidehighered.com/digital-learning/article/2020/03/25/how-shift-remote-learning-might-affect-students-instructors-and [Accessed March 25, 2020]
Legris, P., Ingham, J., and Collerette, P. (2003). Why Do People Use Information Technology? a Critical Review of the Technology Acceptance Model. Inf. Manage. 40, 191–204. doi:10.1016/S0378-7206(01)00143-4
Lei, W. P. (2018). Three Misconceptions of Education Information Technology Policy Research. Educ. Res. Exp. 6, 1–6. CNKI:SUN:YJSY.0.2018-06-001.
Li, S. L. (2005). Introductory Remarks on Analysis of Classroom Instructional Behaviors. Theor. Pract. Educ. 25, 48–51. CNKI: SUN:JYLL.0.2005-07-011.
Li, W., Gao, W., and Sha, J. (2020). Perceived Teacher Autonomy Support and School Engagement of Tibetan Students in Elementary and Middle Schools: Mediating Effect of Self-Efficacy and Academic Emotions. Front. Psychol. 11, 50. doi:10.3389/fpsyg.2020.00050
Li, W., Gao, W. Y., and Fu, W. D. (2021). When Does Teacher Support Reduce Depression in Students? the Moderating Role of Students' Status as Left-Behind Children. Front. Psychol. 12, 608359. doi:10.3389/fpsyg.2021.608359
Liu, G. D. (2019). Exploring Strategies for Analyzing Classroom Teaching Behaviors in an Information-Based Teaching Environment. J. Yanbian Edu. Coll. 33, 10–11. doi:10.3969/j.issn.1673-4564.2019.06.004
Liu, J. W. (2019). Strategies for Transforming Teachers' Teaching Behavior in the Era of "Internet+. West. China Qual. Edu. 5, 115–116. doi:10.16681/j.cnki.wcqe.201905072
(1998‐2015). L. K., Muthén, and B. O., Muthén .in Mplus Users’ Guide. 7th Edn. (Los Angeles, CA: Muthén & Muthén). doi:10.1016/j.ijedudev.2009.09.003
Lowenthal, P. R., and Dennen, V. P. (2017). Social Presence, Identity, and Online Learning: Research Development and Needs. Distance Edu. 38, 137–140. doi:10.1080/01587919.2017.1335172
Ma, J. (2020). A Study of the Mechanisms Influencing College Students' Learning Engagement in a Hybrid Teaching Environment - a Perspective of Teaching Behavior. Distance Edu. China 2, 57–67. CNKI: SUN: DDJY.0.2020-02-00.
Marsh, H. W., Hau, K.-T., and Wen, Z. (2004). In Search of Golden Rules: Comment on Hypothesis-Testing Approaches to Setting Cutoff Values for Fit Indexes and Dangers in Overgeneralizing Hu and Bentler's (1999) Findings. Struct. Equation Model. A Multidisciplinary J. 11, 320–341. doi:10.1207/s15328007sem1103_2
Mishra, P., and Koehler, M. J. (2006). Technological Pedagogical Content Knowledge: A Framework for Teacher Knowledge. Teach. Coll. Rec 108, 1017–1054. doi:10.1111/j.1467-9620.2006.00684.x
Mooij, T., and Smeets, E. (2001). Modelling and Supporting ICT Implementation in Secondary Schools. Comput. Edu. 36 (3), 265–281. doi:10.1016/S0360-1315(00)00068-3
Nguyen, N. T., Thai, T. V., Pham, H. T., and Nguyen, G. C. T. (2020). Cdio Approach in Developing Teacher Training Program to Meet Requirement of the Industrial Revolution 4.0 in Vietnam. Int. J. Emerg. Technol. Learn. 15, 108–123. doi:10.3991/ijet.v15i18.15517
Oliver, K., and Hannafin, M. J. (2000). Student Management of Web-Based Hypermedia Resources during Open-Ended Problem Solving. J. Educ. Res. 94 (2), 75–92. doi:10.2307/2754230810.1080/00220670009598746
Owens, J. K., and Hudson, A. K. (2021). Prioritizing Teacher Emotions: Shifting Teacher Training to a Digital Environment. Education Tech Res. Dev 69, 59–62. doi:10.1007/s11423-020-09921-y
Perifanou, M., Economides, A. A., and Tzafilkou, K. (2021). Teachers' Digital Skills Readiness during Covid-19 Pandemic. Int. J. Emerg. Technol. Learn. 16, 238–251. doi:10.3991/ijet.v16i08.21011
Phuapan, P., Viriyavejakul, C., and Pimdee, P. (2016). An Analysis of Digital Literacy Skills Among Thai university Seniors. Int. J. Emerg. Technol. Learn. 11, 24–31. doi:10.3991/ijet.v11i03.5301
Plante, K., and Asselin, M. E. (2014). Best Practices for Creating Social Presence and Caring Behaviors Online. Nurs. Educ. Perspect. 35, 219–223. doi:10.5480/13-1094.1
Podsakoff, P. M., MacKenzie, S. B., Lee, J. Y., and Podsakoff, N. P. (2003). Common Method Biases in Behavioral Research: A Critical Review of the Literature and Recommended Remedies. J. Appl. Psychol. 88, 879–903. doi:10.1037/0021–9010.88.5.879
Porras-Hernández, L. H., Hernández, M. L., and Alva, M. (2010). Integración de tic al currículum de telesecundaria: incidiendo en procesos del pensamiento desde el enfoque comunicativo funcional de la lengua. Revista Mexicana De Investigación Educativa 15, 515–551. http://www.scielo.org.mx/scielo.php?scrip- t=sci_arttext&pid=S1405-66662010000200008.
Porras-Hernández, L. H., and Salinas-Amescua, B. (2013). Strengthening Tpack: a Broader Notion of Context and the Use of Teacher's Narratives to Reveal Knowledge Construction. J. Educ. Comput. Res. 48, 223–244. doi:10.2190/EC.48.2.f
Santos, J. M., and Castro, R. D. R. (2021). Technological Pedagogical Content Knowledge (Tpack) in Action: Application of Learning in the Classroom by Pre-service Teachers (Pst). Social Sci. Humanities Open 3 (1), 100110. doi:10.1016/j.ssaho.2021.100110
Shi, L. F., and Cui, Y. K. (1999). Teaching Theory: Principles, Strategies, and Research on Classroom Teaching. Shanghai: East China Normal University Press.
Slater, H., Davies, N. M., and Burgess, S. (2012). Do Teachers Matter? Measuring the Variation in Teacher Effectiveness in England*. Oxf. Bull. Econ. Stat. 74, 629–645. doi:10.1111/j.1468-0084.2011.00666.x
Starkey, L. (2019). A Review of Research Exploring Teacher Preparation for the Digital Age. Cambridge J. Edu. 50, 37–56. doi:10.1080/0305764X.2019.1625867
Taylor, S., and Todd, P. A. (1995). Understanding Information Technology Usage: a Test of Competing Models. Inf. Syst. Res. 5, 91–108. doi:10.1287/isre.6.2.144
Tondeur, J., Scherer, R., Baran, E., Siddiq, F., Valtonen, T., and Sointu, E. (2019). Teacher Educators as Gatekeepers: Preparing the Next Generation of Teachers for Technology Integration in Education. Br. J. Educ. Technol. 50, 1189–1209. doi:10.1111/bjet.12748
Uerz, D., Volman, M., and Kral, M. (2018). Teacher Educators' Competences in Fostering Student Teachers' Proficiency in Teaching and Learning with Technology: an Overview of Relevant Research Literature. Teach. Teach. Edu. 70, 12–23. doi:10.1016/j.tate.2017.11.005
Viberg, O., Khalil, M., and Baars, M. (2020). Self-regulated Learning and Learning Analytics in Online Learning Environments. Assoc. Comput. Mach., 524–533. doi:10.1145/3375462.3375483
Wang, J. X., Wei, Y. T., and Zong, M. (2020). The Current Situation,Problems and Reflection of Online Teaching for Primary and Secondary School Teachers during a Large-Scale Epidemic——Based on the Investigation and Analysis of "Classes Suspended but Learning Continue" in Hubei Province. China Educ. Tech. 5, 15–21. doi:10.3969/j.issn.1006-9860.2020.05.004
Wastiau, P., Blamire, R., Kearney, C., Quittre, V., Van de Gaer, E., and Monseur, C. (2013). The use of ICT in education: a survey of schools in Europe. Eur. J. Edu. 48, 11–27. doi:10.1111/ejed.12020
Yamin, K. (2020). Mixed Response but Online Classes to Stay post COVID-19. Indonesia: University World News. Available at: https://www.universityworldnews.com/post.php?story=20200514121749886 (Accessed May 14, 2020).
Yang, G. X., and Hu, J. J. (2014). In the View of Ecological Sight Interpret IT Support Classroom Teaching. China Educ. Tech. 331, 100–104+110. doi:10.3969/j.issn.1006-9860.2014.08.018
Yu, S. Q., and Wang, H. M. (2020). How to Better Organize Online Learning in Extreme Situations such as Epidemics. China Educ. Tech. 5 (6–14), 33. doi:10.3969/j.issn.1006-9860.2020.05.003
Zeng, Z. M., and Zheng, A. A. (2019). Study on factors influencing learners' willingness to continuously use MOOC platform in Chinese universities. Chin. J. Ict Edu. 16, 28–33. CNKI: SUN: JYXX. 0.2019-16-008.
Zhai, X. (2021). Advancing automatic guidance in virtual science inquiry: from ease of use to personalization. Edu. Tech Res. Dev 69, 255–258. doi:10.1007/s11423-020-09917-8
Zhang, J. (2014). Connotations and Features of TPACK from Three Different Perspectives Connotations and Features of TPACK from Three Different Perspectives. Distance Edu. J. 32, 87–95. doi:10.15881/j.cnki.cn33-1304/g4.2014.01.012
Zhang, K. (2018). A study on elementary school teachers' intention to use online teaching resources. Zhengzhou: Henan University, 113107. Dissertation’s thesis
Zhang, W., Wang, Y., Yang, L., and Wang, C. (2020). Suspending Classes Without Stopping Learning: China's Education Emergency Management Policy in the COVID-19 Outbreak. Jrfm 13, 55–61. doi:10.3390/jrfm13030055
Zhang, Y., Zhu, Y., Bai, Q. Y., Li, X. Y., and Zhu, Y. H. (2016). Research of the Teaching Interaction Behavior Characteristics of Primary Mathematics in the Smart Classroom. China Educ. Tech. 353, 43–48. doi:10.3969/j.issn.1006-9860.2016.06.009
Zhao, C. L., Hu, P., Ling, Y. Z. M., Jiang, Z. H., Huang, Y., and anf Shu, F. F. (2019). Using NLP opinion mining to study teaching behaviors in open online courses. Distance Edu. China 556, 62–70+97. doi:10.13541/j.cnki.chinade.20190111.003
Keywords: online teaching, use intention of online teaching, digital competence, online teaching behavior, students’ online learning difficulties
Citation: Li W, Gao W, Fu W and Chen Y (2021) A Moderated Mediation Model of the Relationship Between Primary and Secondary School Teachers’ Digital Competence and Online Teaching Behavior. Front. Educ. 6:744950. doi: 10.3389/feduc.2021.744950
Received: 21 July 2021; Accepted: 27 August 2021;
Published: 08 September 2021.
Edited by:
Shuai (Sam) Wang, Shanghai Jiao Tong University, ChinaReviewed by:
Laura Sara Agrati, University of Bergamo, ItalySiti Mistima Maat, Universiti Kebangsaan Malaysia, Malaysia
Copyright © 2021 Li, Gao, Fu and Chen. This is an open-access article distributed under the terms of the Creative Commons Attribution License (CC BY). The use, distribution or reproduction in other forums is permitted, provided the original author(s) and the copyright owner(s) are credited and that the original publication in this journal is cited, in accordance with accepted academic practice. No use, distribution or reproduction is permitted which does not comply with these terms.
*Correspondence: Weidong Fu, ZnV3ZGNjbnVAMTYzLmNvbQ==