- 1Centre for Research in Child Development, Office of Education Research, National Institute of Education, Singapore, Singapore
- 2Macquarie School of Education, Macquarie University, Sydney, NSW, Australia
Childhood socioeconomic status (SES) is strongly predictive of math achievement in early childhood and beyond. In this study, we aimed to further our understanding of the mechanisms underpinning the SES-achievement gap by examining whether two aspects of self-regulation—executive functions (EF) and behavioral self-regulation (BSR)—mediate between SES and math achievement. Using data from a longitudinal study in Singapore (n = 1,257, 49% males), we examined the predictive link from SES to math achievement at entry to formal education (age 7), and the role of EF (child-assessed) and BSR (child-assessed and teacher-rated) as mediators of the SES-math achievement relationship. After accounting for children’s non-verbal reasoning and prior math achievement, EF and BSR (both child-assessed) emerged as significant partial mediators between SES and math. A key contribution of our study is in demonstrating that both components of self-regulation play a small role in explaining SES disparities in math achievement. Our findings further suggest that a balanced focus on enhancing EF and BSR skills of children from low-SES families may help to attenuate the SES-math achievement gap. More generally, our research contributes new insights to the ongoing debate about the theoretical distinctions between EF and BSR.
Introduction
Family socioeconomic status (SES) is negatively associated with the development of children’s mathematics skills. As mathematical skills are strongly predictive of health, wealth, and SES in adulthood (Ritchie and Bates, 2013), early math achievement gaps may perpetuate the cycle of inequality. Thus, it is important to understand the pathways by which SES is associated with mathematical skills early in development. A growing body of evidence suggests that two domain-general factors related to self-regulation, executive functions (EF; Ellefson et al., 2020; Waters et al., 2021) and behavioral self-regulation (BSR; Sektnan et al., 2010) play a mediating role between SES and math achievement. However, these studies have considered the role of EF and BSR in isolation. Given the conceptual overlap between EF and BSR, it remains unclear whether each skill uniquely mediates the SES-achievement gap in mathematics when they are considered together. In this study, we investigate the relations among family SES at age 5 (the first year of kindergarten), EF and BSR at age 6 (the second year of kindergarten), and children’s math achievement at age 7 (at entry to primary school). We also examine whether EF and BSR, representing different aspects of self-regulation, uniquely mediates the SES-achievement gap in math at entry to primary school. Findings would add to the literature about the distinction between EF and BSR, as well as our understanding of the skills that underlie early academic disparities.
Executive Functions and Behavioral Self-Regulation Skills
Executive functions (EF) refer to cognitive processes involved in intentionally controlling one’s attention, thinking, behaviors, and impulses to perform goal-directed actions (Huizinga et al., 2006). It is commonly conceptualized as a multi-componential framework including cognitive flexibility, inhibition, and working memory (Lee et al., 2013). Cognitive flexibility refers to the ability to shift between alternative sets of mental operations, inhibition is the ability to resist interference from competing or prepotent responses, and working memory refers to the ability to refresh and maintain information in the presence of new knowledge (Miyake et al., 2000). Behavioral self-regulation (BSR) is defined as “deliberately applying multiple component processes of … (EF) to overt, socially contextualized behaviors” (McClelland et al., 2014; p. 2). Within school settings, children use BSR to control their behavior, remember instructions, pay attention, and complete tasks, which facilitate the learning process (McClelland and Cameron, 2012; Blair and Raver, 2015).
Executive functions and BSR are components of self-regulation, a multi-dimensional construct that describes the ability to control thoughts, feelings, actions, and emotions (Blair and Raver, 2015). On the theoretical level, within the early childhood literature, EF and BSR are viewed as distinct, but conceptually interrelated and overlapping skills (Duncan et al., 2017; Anthony and Ogg, 2019; Compagnoni et al., 2019). For example, Compagnoni et al. (2019) conceptualized EF as a set of domain-general abilities that correlates with and supports BSR as a context-specific developing skill set; BSR is also often viewed as the behavioral manifestation of EF (e.g., Becker et al., 2014). Thus, EF can be thought of as the same set of skills or as antecedent skills that contribute towards BSR. On the other hand, the manifestation of BSR skills requires the ability to first integrate or coordinate different aspects of EF, and second, to do so in accordance with varying social contexts. Thus, BSR can arguably be considered as a separate skill set with different trajectories, predictors, and outcomes from EF.
On the operational level, EF and BSR can be distinguished in terms of their measurement tools. Typically, EF skills are assessed using laboratory-based, direct performance measures of components of EF, such as the Flanker task for inhibition (Fan et al., 2002), Dimensional Change Card Sort for cognitive flexibility (Diamond et al., 2005), and Listening Recall for working memory (Alloway, 2007). Behavioral self-regulation can be assessed using direct performance measures (e.g., the Head-Toes-Knees-Shoulders task; McClelland et al., 2014) or informant (e.g., teacher or self) ratings. The latter complements direct assessment methods by assessing children’s ability to demonstrate BSR in a social context such as the classroom (e.g., the Child Behavior Rating Scale; Bronson et al., 1995) and other everyday settings (e.g., the Behavior Rating Inventory of Executive Function; Gioia et al., 2000). In some studies, different terms are used to refer to informant ratings of children’s BSR skills, e.g., teacher ratings on children’s approaches to learning (Li-Grining et al., 2010) and learning-related behaviors (Anthony and Ogg, 2019).
Going from a task such as Listening Recall to Head-Toes-Knees-Shoulders to the Child Behavior Rating Scale increasingly taps upon the child’s ability to integrate component EF processes and to apply it appropriately according to the demands of specific social settings. These categories of measures can thus be viewed as tapping qualitatively different sets of skills, on both theoretical and operational levels. While there appears to be some agreement about the conceptual overlap between EF and BSR, the empirical literature provides mixed views about the relations among these constructs: EF and BSR have been found to share overlapping variance, though correlations between scores on these measures tend to vary in the low-to-moderate range (McClelland et al., 2014; Duncan et al., 2017; Finders et al., 2021).
During the transition to formal schooling, self-regulation skills are particularly important to help children adapt to learning in a more structured classroom setting (Neuenschwander et al., 2012). The cognitive control skills assessed by EF tasks may be beneficial for holding task-specific instructions in memory, remembering a sequence of steps to solve a problem, or inhibiting and switching between procedural strategies. In contrast, BSR likely plays a more general role in supporting engagement in learning opportunities, e.g., via persistence to continue working on difficult tasks, ignoring distractions in the classroom, and refraining from disruptive or inappropriate behaviors.
Specific to math achievement, both EF and BSR (measured using direct performance measures and teacher reports) have been identified as key factors promoting children’s concurrent and later math achievement (Duncan et al., 2007; Li-Grining et al., 2010; McClelland et al., 2014; Sasser et al., 2015; Lee and Bull, 2016; Morgan et al., 2019). For example, Morgan et al. (2019) found that all three aspects of kindergartner’s EF skills (cognitive flexibility, working memory, and inhibition) positively predicted second grader’s mathematics achievement in a longitudinal and nationally representative sample from the United States. Sasser et al. (2015) showed that pre-kindergarten BSR significantly predicted kindergarten and first grade math outcomes. Some studies have found that both EF and BSR uniquely predict children’s math achievement (Fuhs et al., 2015; Beisly et al., 2020). There is also some evidence suggesting that EF indirectly affects math achievement through BSR (Nesbitt et al., 2015), or that EF and BSR moderate the relationship between variables such as SES and math achievement in different ways (Beisly et al., 2020).
SES and Children’s Math and Self-Regulation Skills
Broadly defined, SES refers to an individual’s access to economic and social resources and the privileges that derive from these resources (McLoyd, 1998; Bradley and Corwyn, 2002). It is typically assessed using measures of parental education, income, and occupation (Duncan and Magnuson, 2012). Socio-economic status is a robust predictor of children’s developmental outcomes. Positive associations between SES and children’s cognitive and academic outcomes have been documented across a wide age range, from kindergarten until the later schooling years (Bradley and Corwyn, 2002; Noble et al., 2007; Aikens and Barbarin, 2008; Mistry et al., 2010; Lawson and Farah, 2015).
In the math domain, SES differences emerge early during preschool, with studies showing that children from low-SES backgrounds perform more poorly than their higher-SES peers on counting, adding, subtracting, and comparing magnitudes (Starkey et al., 2004; Jordan et al., 2006; Elliott and Bachman, 2018). In larger studies utilizing the Early Childhood Longitudinal Study-Kindergarten Class of 1998–99 (ECLS-K: 1999), differences of more than 1 SD in kindergarten mathematics scores were reported between children from the lowest SES quintile compared to the highest (Burkam et al., 2004; Duncan and Magnuson, 2012). Evidence also suggests that SES disparities in math during early childhood persist and widen throughout later childhood and adolescence. For example, a meta-analytic study Sirin (2005) found that student’s grade level moderated the SES-math achievement relationship, whereby effect sizes increased from kindergarten (0.19) to elementary school (0.27) and middle school (0.31). There is also evidence showing that initial SES-related math deficits at kindergarten are associated with slower growth in math abilities from first to third grade (Jordan et al., 2006).
Children’s SES is significantly associated with their EF skills (Sarsour et al., 2011; Dilworth-Bart 2012; Little, 2017), with a recent meta-analysis reporting a small-to-medium relationship (r = 0.22) in socioeconomically diverse populations (Lawson et al., 2018). These findings were obtained using composite or latent measures of EF as well as individual EF components (Hackman et al., 2015). Evidence also indicates that the strength of the SES-EF relationship is stable across early and middle childhood (e.g., Hackman et al., 2015; Lawson et al., 2018). Similarly, SES is linked to children’s BSR. McClelland and colleagues have shown that children aged 3–5 years with low BSR (assessed using direct measures and teacher ratings) were more likely to have parents with lower educational attainment compared to their peers (McClelland et al., 2000; McClelland et al., 2007). Similarly, Sektnan et al. (2010) reported a positive association between maternal education and children’s BSR (teacher-rated and parent-rated) in first grade.
The mechanisms underlying the effects of SES on children’s developmental outcomes are complex and multi-faceted. Families from low-SES backgrounds face a variety of daily stressors (e.g., lack of job stability, poor housing conditions, living in unsafe neighborhoods), which can lead to high levels of psychological distress and conflict within family members (Duncan et al., 2017). These families also lack financial and material resources because of economic hardship and financial instability (Duncan et al., 2017). The confluence of these factors creates systematic barriers that make it difficult for parents to provide enriching home and childcare environments as well as high quality, nurturing caregiving to their children (Domina and Roksa, 2012; Duncan and Magnuson, 2012). Studies have shown that parents from higher SES backgrounds are more likely to provide cognitively stimulating learning materials and activities at home, as well as more responsive and consistent caregiving (Votruba-Drzal, 2003; Raviv et al., 2004; Magnuson et al., 2009; Dilworth-Bart, 2012). These high-quality caregiving strategies and home learning environments support the development of children’s EF and math skills (Zadeh et al., 2010; Sarsour et al., 2011; Elliott and Bachman, 2018). In contrast, exposure to highly stressful environments may be associated with elevated levels of chronic stress, which contribute to lower levels of EF in children (Blair and Raver, 2012). In terms of BSR skills, it has been suggested that children from low-SES backgrounds are exposed to a variety of risk factors (e.g., fewer resources at home, unsafe neighborhoods), which reduces their exposure to learning contexts that promote BSR and opportunities to practice these skills (Evans and Rosenbaum, 2008). Sektnan et al. (2010) also proposed that growing up in low-SES, high-risk environments might lead children to develop BSR strategies that are maladaptive in classroom settings.
Self-Regulation Skills as Mediators Between SES and Math Achievement
Building on previous findings and the proposed mechanisms underlying SES differences in EF, BSR, and math skills, researchers have speculated that EF and BSR may partially explain the SES-math achievement gap. Specifically, within the context of better-quality caregiving and home learning environments, children from high-SES families have more opportunities to develop their EF and BSR skills compared to their peers from low-SES families. In turn, children with higher EF and BSR skills are likely better able to process and remember information as well as to maintain their focus, which facilitates the acquisition of math knowledge and skills in school. Consistent with this hypothesis, many studies have found that EF partially mediated the link between SES and math achievement in preschoolers, kindergartners, and adolescents (Dilworth-Bart, 2012; Fitzpatrick et al., 2014; Lawson and Farah, 2015; Waters et al., 2021). One study Nesbitt et al. (2013) found that EF fully mediated the link between SES and Grade 1 math achievement. A smaller number of studies have also found a significant mediating role for BSR (Sektnan et al., 2010; Zhang et al., 2019).
Taken together, previous findings show that SES predicts children’s EF and BSR, as well as math achievement. Each aspect of self-regulation also plays a partial mediating role between SES and children’s math achievement. However, given the overlapping variance between EF and BSR, it is surprising that few studies have considered whether each of these skills offers a unique contribution to accounting for the SES achievement gap in math. One exception is the recent study by Finders et al. (2021) which examined whether EF explained any additional proportion of the achievement gap after accounting for BSR. Finders et al. (2021) included two measures of EF as indicators of cognitive flexibility (the Dimensional Change Card Sort) and working memory (the Numbers Reversed task) as well as teacher ratings of BSR (the Child Behavior Questionnaire). The study found that both EF and BSR skills independently contributed to math achievement gaps in kindergarten, with EF continuing to predict performance gaps at third grade.
An important contribution of Finders et al. (2021) study is the finding that different aspects of self-regulation uniquely predict math achievement gaps among children from economically disadvantaged families. However, there are some outstanding issues to consider. First, only two aspects of EF were assessed (i.e., cognitive flexibility and working memory) using individual tasks. This provides a relatively imprecise and incomplete assessment of EF. Using multiple measures of EF (including tasks that tap inhibition) would provide a more precise measurement of EF skills by extracting common variance across tasks to minimize issues related to task impurity (Lee et al., 2013; Duncan et al., 2017). In the current study, we employed the latent factor approach to assess children’s EF skills.
In the studies reviewed above, SES was measured either using single indicators (e.g., household income) or composite measures (e.g., combination of income and parental education). A growing body of evidence points to parental education attainment as the most powerful predictor of children’s cognitive and academic outcomes (Magnuson et al., 2009; Harding et al., 2015; Davis-Kean et al., 2021; Waters et al., 2021). More educated parents tend to have access to a greater number of resources and provide their children with more stimulating activities, toys, books, and educational opportunities both in and outside the home (Cebolla-Boado et al., 2017; Davis-Kean et al., 2021). As evidence suggests that mothers’ education may be more influential than father’s education for early learning and development (Cebolla-Boado et al., 2017; Conway et al., 2018), we defined SES in terms of maternal education in the current study.
Recent findings suggest that the associations between SES and self-regulation skills may vary according to sociocultural contexts. For example, in a recent study with 9- to 16-year-olds, SES significantly predicted EF skills in a Western sample from United Kingdom, but not in an Asian sample from Hong Kong (Ellefson et al., 2020). In another study involving 6-year-olds, SES significantly influenced children’s BSR in the United States’ sample but not in the Norwegian sample (Lenes et al., 2020). In line with the bioecological model of development (Bronfenbrenner and Morris, 2006), these findings suggest that cultural differences in beliefs or ideologies may influence the role of sociodemographic factors on children’s socialization practices (e.g., parent’s and teacher’s expectations) and development (Lenes et al., 2020). Our study expands on this work by examining the associations between SES and self-regulation skills in an Asian sample from Singapore.
Study Aims
The current study has two aims for gaining a better understanding of the cognitive underpinnings of poor math achievement in children from low SES families. The first aim is to examine the contributions of SES, EF, and BSR to math achievement in a sample of preschool children in Singapore. The second aim is to examine whether different aspects of self-regulation uniquely mediate the SES-achievement gap in math. As suggested by Sektnan et al. (2010), we also investigated whether direct performance measures and teacher ratings of BSR each captured a unique perspective of children’s skills and independently mediated the SES-achievement gap. Whilst direct performance measures of BSR have been found to associate well with ratings of real-world self-regulatory behaviors (e.g., Howard et al., 2019), these different types of assessment may also capture something unique about children’s ability to use BSR in different contexts. Specifically, direct performance measures may capture single moments of BSR in highly structured one-to-one situations (often with an unknown researcher) whereas teacher ratings capture children’s ability to apply BSR in ecological settings across different contexts and time. Additionally, the use of a direct performance measure of BSR allows us to consider whether differences in the predictive role of EF and teacher-rated BSR might be due to the type of measurement instrument.
Our study also expands on previous work by examining whether the associations between SES and self-regulation skills in an Asian sample correspond to findings in Western and European samples (Ellefson et al., 2020; Lenes et al., 2020). Given recent evidence of cross-cultural differences in these associations, it is important to investigate whether prior findings regarding the mediating role of EF and BSR in the SES achievement gap applies to Singapore, a multicultural society which has a strong emphasis on a culture of hard work, filial piety, and respect for scholarship and learning. Such beliefs clearly influence parenting practices and expectations of children’s self-regulatory behaviors (see Schirmbeck et al., 2020). In addition, attendance at preschool in Singapore is highly subsidized for lower income families, which has helped to ensure that approximately 95% of children attend up to 2 years of preschool before formal education starts the year children turn 7 (Bull and Bautista, 2018). There are also government-level initiatives that provide community-based learning, development, and health support services to children from low-income families. For example, the KidSTART program equips parents with skills so their children can benefit from warm and nurturing relationships, achieve age-appropriate and holistic child development, and have a safe and secure home environment (KidSTART Singapore, 2020). Such a combination of shared cultural values, equitable access to preschool education of a reasonable level of quality (Tan, 2017), and targeted initiatives to help low-income families may result in weaker relationships between SES and self-regulation skills in Singapore compared to other cultural contexts.
In consideration of the literature, we hypothesized that SES, EF, and BSR skills would predict math achievement at P1. We also hypothesized that EF and BSR skills would partially mediate the association between SES and math achievement at P1. However, considering the specific context of Singapore as described above, the strength of the mediation effects may be weaker compared to the effects reported in other cultural contexts. Given the lack of previous work comparing the predictive roles of teacher-rated BSR and child-assessed BSR, we did not formulate specific hypotheses about the predictive strength of these variables.
Materials and Methods
Participants
This paper draws on data from a longitudinal study examining the impact of preschool experiences on children’s developmental outcomes in Singapore. Participating children’s parents provided informed consent. The author’s university institutional review board provided ethics approval. The total sample comprised 1,537 children recruited from 80 preschools across a range of social strata. The sample used for this study consisted of 1,257 children recruited into the study at the beginning of their first year of kindergarten; the remaining 280 children were recruited later and thus did not complete the assessments conducted in Wave 1 (see Procedure). After excluding those with the presence of learning or developmental issues (19 children), high (>42 weeks) or low (<34 weeks) gestational duration (26 children) and missing data on these variables (175 children), the final sample comprised 1,037 children. The sample generally reflected the population composition of Singapore (Department of Statistics, 2015). Of the 1,037 children, 504 (48.6%) were males. In terms of ethnicity, 63.7% were Chinese, 11.3% Malay, 19.8% Indian, and 4.7% identified as others; 1.5% did not indicate ethnicity information. The average age for children in the first year of kindergarten (K1) was 57.4 months (SD = 3.9; 0.7% missing data), in the second year of kindergarten (K2) was 69.8 months (SD = 3.8; 7% missing data), and in the first year of primary school (P1) was 81.0 months (SD = 3.7; 13% missing data). Results from Little (1988) Missing Completely at Random (MCAR) test indicated that the missing data were MCAR, X2(23) = 35.07, p = 0.051.
Measures
Executive Functioning
A suite of computerized tasks was used to assess children’s shifting, inhibition, and working memory abilities. Three working memory tasks were used. In Animal Updating (Lee et al., 2013), pictures of animals were shown one at a time. Children recalled the identities of the last two, three, or four animals that were presented. They were not told how many animals to expect to ensure that working memory was used in the task. The dependent measure was the total number of animals recalled correctly. In Backward Digit Recall (adapted from Pickering and Gathercole, 2001), children listened to a series of numbers presented through headphones and recalled the numbers in backward order. The dependent measure was the number of trials recalled correctly. In Lost Animals (adapted from Law et al., 1995), children completed a processing task, followed by a memory recall task within each trial. In the processing task, children verified the accuracy of an “addition” equation in which two line matrices were added together to form a third line matrix. Each line matrix consisted of five objects in a square (one at each corner and one in the middle), with a line connecting the objects in a variety of configurations. The objects in the matrices were presented as food or toys, and the lines depicted the paths taken by an animal to get food or toys. Following the processing task, children recalled the location of a coloured object (i.e., the target object) in the third matrix. Each block had six trials progressing from a block with one matrix to be verified and one location to be recalled to a block with three matrices to be verified and three locations to be recalled. In total, the task has 18 trials. The dependent measure was the number of object locations recalled correctly in the correct order.
Two tasks were used to assess inhibition skills. In the Flanker task (Lee et al., 2012), children were presented with a row of five arrows and asked to identify whether the middle arrow (target) was facing left or right. A neutral block displayed only one arrow that faced either left or right. In the congruent block, all the arrows faced the same direction. In the incongruent block, the off-centre arrows faced the opposite direction from the target. In the Simon task (Lee et al., 2012), a picture of a frog or a butterfly appeared on the left or right side of the computer screen. Children were asked to lead the butterfly home by pressing a button on the left, and likewise for the frog by pressing a button on the right. In the congruent block, the animals appeared on the same side as their “home” location. In the incongruent block, the animals appeared on the opposite side of their “home”. For both tasks, the dependent measures were the number of correct responses in the congruent and incongruent blocks.
Two tasks were used to assess shifting skills. In the Dimensional Change Card Sort (DCCS; Slotkin et al., 2012), children were presented with objects that varied in colour (blue and red) and shape (rabbits and boats) on the computer screen. Children sorted the objects according to its shape or colour. They completed two blocks of trials, in which the sorting rules were intermixed. The dependent measures were accuracy scores and reaction time (RT) on each trial. A combined accuracy and RT score was calculated according to the formulae provided in the NIH Toolbox Technical Manual; higher scores reflect better shifting skills. In Picture-Symbol (Lee et al., 2012), a bigram comprising a picture and a symbol appeared in one of four quadrants on the computer screen. When the bigram appeared in the top quadrants, children identified whether the picture was an animal. When it appeared at the bottom quadrants, they identified whether the symbol was a number. In the first and second block of trials, the bigrams appeared only in the top and bottom quadrants, respectively. In the final two mixed blocks, the bigrams appeared clockwise in all four quadrants. Switch and non-switch scores were computed from the mixed blocks. Switches were generated from trials at which children switch from the animal to number or from the number to animal trials. Switch accuracy was computed from the number of correct switch trials divided by the total number of mixed trials. In the same way, non-switch accuracy was computed.
Child-Assessed Behavioral Self-Regulation
The Heads-Toes-Knees-Shoulders (HTKS) task was used as a direct measure of children’s behavioral self-regulation skills (McClelland et al., 2014). Children were presented with behavioral rules where they were required to do the opposite of what the experimenter asked them to do (e.g., touch their head when told to touch their toes). The task has three parts, each comprising a set of behavioral rules and practice items. In Part One, children completed six practice trials with feedback about two rules (i.e., touch your head/touch your toes), followed by ten test trials. In Part Two, children completed five practice trials with feedback about two new rules (i.e., touch your shoulders/touch your knees), followed by ten test trials combining the rules from Part One and Two (i.e., head/toes, knees/shoulders). In Part Three, the rule pairs were switched (i.e., heads/knees, shoulders/toes). Children first completed six practice trials with feedback, followed by ten test trials. The task was discontinued if the child’s total score on the test trials for Part One or Part Two was less than 4 points. The dependent measure was the total score across all three parts; a higher score reflects better task performance.
Teacher-Rated Behavioral Self-Regulation
The 12-item Learning-related Social Skills subscale in the Child Behavior Rating Scale (CBRS; Lim et al., 2010) was used to assess children’s BSR in the classroom. Teachers rated the frequency of the behavior described in each item (e.g., returns to unfinished tasks after interruption) using a five-point Likert scale, from 1 (never) to 5 (always). The dependent measure was the average score across all items.
Math Skills
The Test of Early Mathematics Ability–3rd edition (TEMA-3; Ginsburg and Baroody, 2003) assessed children’s informal and formal mathematics knowledge. Test administration began at an appropriate entry point according to the manual. Each item was scored as 1 or zero, depending on whether the scoring criteria were met. Basal and ceiling rules were implemented according to the manual. Non-administered items before basal were scored 1 and non-administered items after ceiling were scored zero. The dependent measure was the sum of scores on all items below the ceiling. Higher scores reflect better math skills. This measure has been validated for use in Singapore (Yao et al., 2017), with evidence of convergent validity with the Number Sets Test (Geary et al., 2009), a measure of children’s ability to identify and process numerical quantities.
Socioeconomic Status
Parents provided information on home background (e.g., parent’s educational qualifications, housing type, household income) in a short questionnaire. This questionnaire was distributed to parents via their children’s kindergarten teachers and included basic demographics (e.g., age, gender, ethnicity), and other information about the child’s early development. Based on prior findings, we focused on mother’s educational attainment. For the current study, mothers’ education qualifications, which included 11 different levels, were recoded into 5 categories according to the Singapore Standard Educational Classification (SSEC; Department of Statistics, 2015): 1 = below secondary (5.9%), 2 = secondary (14.2%), 3 = post-secondary (non-tertiary; 7.0%), 4 = diploma or professional qualification (19.3%), 5 = university degree (52.2%); 1.4% did not provide information about educational qualifications. Compared to data collected from the wider population in 2015 (Department of Statistics, 2015), the current sample comprised a smaller proportion of mothers with secondary and lower qualifications (20.1% vs. 52.0% in the population) and a larger proportion of university graduates (52.2% vs. 26.1% in the population).
Non-Verbal Reasoning
Raven’s Colored Progressive Matrices (Raven et al., 1998) was used as a covariate. This task comprised three sets of 12 items. In each item, a pattern with a missing element was presented in matrix format. Children selected from a set of alternatives the element that completed the pattern. To reduce testing burden, administration of each set was terminated after four consecutive incorrect responses. The dependent measure was the total number of correct responses across all three sets.
Procedure
Child assessments of EF, BSR, and math skills were administered as part of a larger task battery at the beginning of Kindergarten 1 (K1; Wave 1), towards the end of K1 (Wave 2), at K2 (Wave 3) and at Primary 1 (Wave 4). Each task was administered individually to the child in their schools. The current study draws on data from the EF and child-assessed BSR measures at K2 (Wave 3), and math skills at waves 1 and 4 (i.e., start of K1 and at Primary 1), as well as data from the non-verbal reasoning task administered at Wave 1. Questionnaires were distributed to parents throughout the first year to gather information about SES. The Child Behavior Rating Scale was administered to K2 teachers around August to December of the study’s second year to gather information on children’s BSR in the classroom.
Analytical Plan
All descriptive and inferential statistical analyses were estimated using Mplus v8 (Muthén and Muthén, 2017). As previous studies did not clearly show three separable components of EF in young children (Lee et al., 2012; Lee et al., 2013), and existing evidence suggesting a unidimensional EF structure with young children (Willoughby et al., 2012), we specified a one-factor model1 comprising all seven EF measures (Figure 1A). In addition, we followed Lee et al. (2013) approach of regressing the incongruent or switch measures to their congruent or non-switch counterparts to obtain a purer measure of EF. This model provided good fit with the data (RMSEA = 0.06, CFI = 0.97, and SRMR = 0.03). All manifest variables loaded significantly on the latent factor (standardized coefficients range from 0.17 to 0.73). Given the very low factor loading for the Picture-Symbol task (0.17), the one-factor model was rerun without this task. The resulting model (Figure 1B) provided good fit with the data (RMSEA = 0.05, CFI = 0.95, and SRMR = 0.03), thus the EF latent factor scores were used in the main analyses.
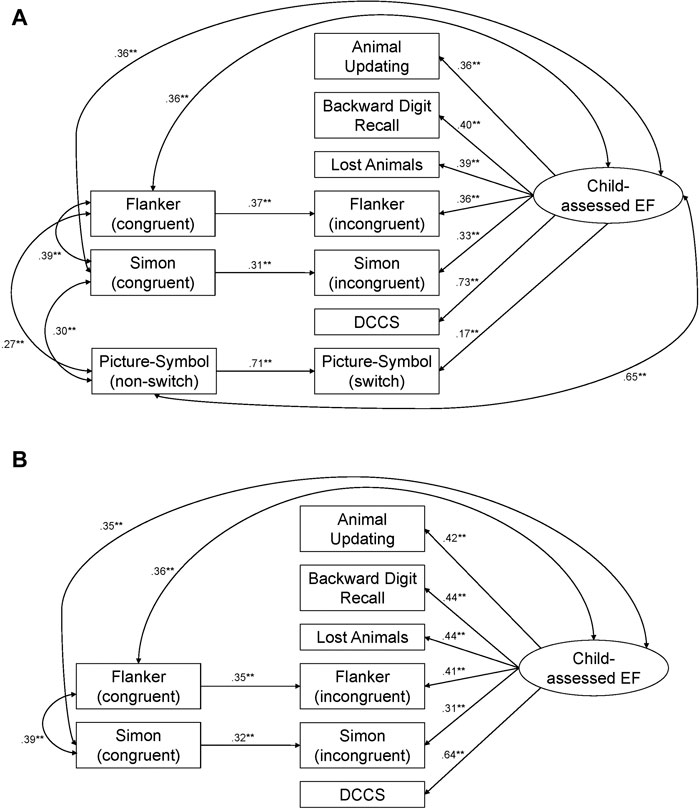
FIGURE 1. Factor structure of child-assessed executive functioning (EF). The initial and final factor structure is shown in (A) and (B), respectively. All path coefficients are standardized estimates. **p < 0.01.
To address our study aims, three path models were estimated. Given that children were nested within teachers, the TYPE = COMPLEX command was used in Mplus to account for the clustering of the data in all models. Models 1 and 2 addressed the first aim of examining the contributions of SES, EF and BSR on math achievement, and whether child-assessed and teacher-rated BSR each provides a unique perspective of children’s skills. In Model 1 (Figure 2), we assessed the contribution of SES to math achievement at Primary 1 (P1), controlling for child’s age at K1 and P1, math skills at K1, time interval between K1 and P1 assessment of math skills, and non-verbal reasoning. In Model 2 (Figure 3), we assessed the direct contributions of SES, child-assessed EF, child-assessed BSR, and teacher-rated BSR to P1 math achievement, taking into account the same covariates in Model 1. In addition, children’s non-verbal reasoning skills and age at K2 were included as covariates of child-assessed EF, child-assessed BSR, and teacher-rated BSR. The EF and BSR measures were also allowed to correlate with each other, and with math skills at K1.
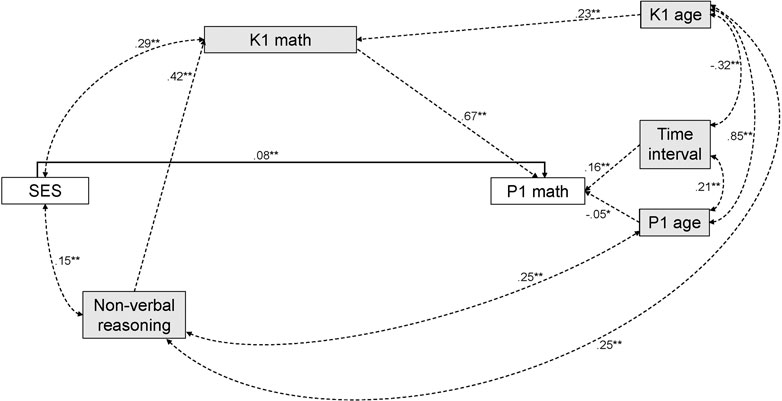
FIGURE 2. Model 1 examined the influence of socio-economic status (SES) on math achievement at Primary 1 (P1 math), controlling for child’s age at Kindergarten 1 (K1) and P1, time interval between K1 and P1 assessment of math skills, math skills at K1, and non-verbal reasoning. Dashed paths reflect predictive and correlational paths involving control variables. All path coefficients are standardized estimates. R2P1 math = 0.48, p < 0.01, *p < 0.05, **p < 0.01.
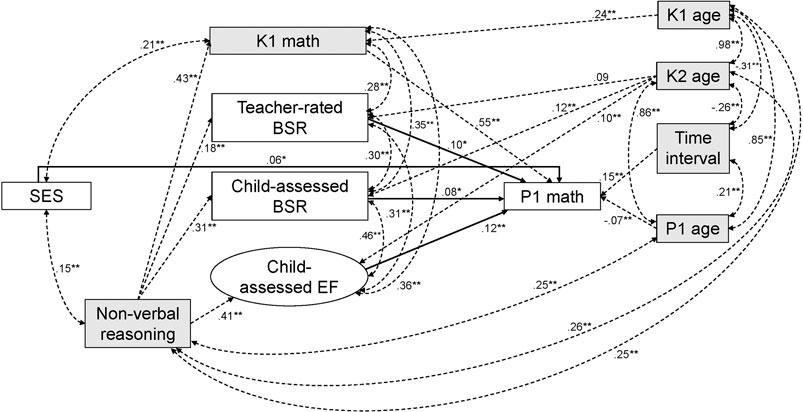
FIGURE 3. Model 2 examined the influences of socio-economic status (SES), child-assessed executive functioning (EF), child-assessed behavioral self-regulation (BSR), and teacher-rated BSR on math achievement at Primary 1 (P1 math), controlling for child’s age at Kindergarten 1 (K1), K2, and P1, time interval between K1 and P1 assessment of math skills, math skills at K1, and non-verbal reasoning. Dashed paths reflect predictive and correlational paths involving control variables. All path coefficients are standardized estimates. R2EF = 0.20, p < 0.01, R2Teacher-rated BSR = 0.05, p < 0.05, R2Child-assessed BSR = 0.13, p < 0.01, R2P1 math = 0.50, p < 0.01, *p < 0.05, **p < 0.01.
Model 3 (Figure 4) addressed the second aim of assessing whether different aspects of self-regulation uniquely mediate the SES-achievement gap in math, taking into account the same covariates in Model 2. Thus, directional paths from SES to child-assessed EF, child-assessed BSR, and teacher-rated BSR were added to the model. The residuals of all three mediators were allowed to correlate. Tests of mediation were conducted using the MODEL INDIRECT command in Mplus and bootstrapped confidence intervals.
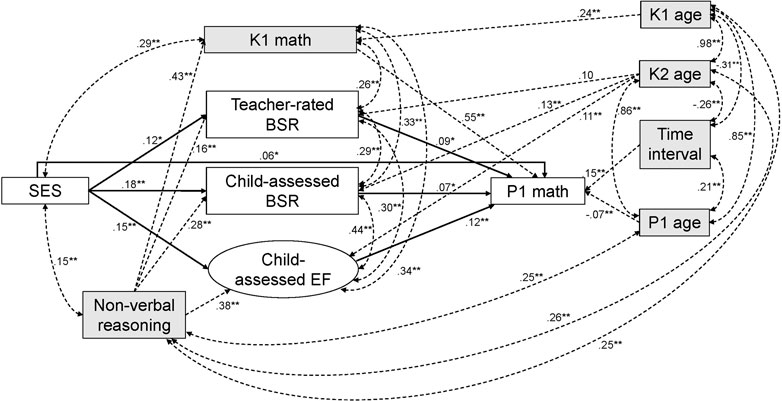
FIGURE 4. Model 3 examined the roles of child-assessed executive functioning (EF), child-assessed behavioral self-regulation (BSR), and teacher-rated BSR as mediators between socio-economic status (SES) and math achievement at Primary 1 (P1 math), controlling for child’s age at Kindergarten 1 (K1), K2, and P1, time interval between K1 and P1 assessment of math skills, math skills at K1, and non-verbal reasoning. Dashed paths reflect predictive and correlational paths involving control variables. All path coefficients are standardized estimates. R2EF = 0.22, p < 0.01, R2Teacher-rated BSR = 0.06, p < 0.05, R2Child-assessed BSR = 0.16, p < 0.01, R2P1 math = 0.51, p < 0.01, *p < 0.05, **p < 0.01.
Results
Table 1 presents the descriptives, bivariate correlations, and reliabilities for the study variables. For all the EF measures, higher scores reflected better EF ability. The EF measures, both observed and latent, positively correlated with both measures of BSR (child-assessed and teacher-rated). The EF latent factor score correlated more highly with the direct performance measure of BSR (r = 0.54) than teacher ratings of BSR (r = 0.36), as indicated by the results of the Wald test, χ2(1) = 122.03, p < 0.001. The EF latent factor and measures of BSR also correlated positively with math skills at K1 and P1. At K1, math skills correlated more highly with EF (r = 0.52) compared to child-assessed BSR [r = 0.49; χ2(1) = 25.60, p < 0.001] and teacher ratings of BSR [r = 0.36, χ2(1) = 153.28, p < 0.001]. Similarly, at P1, math skills correlated more highly with EF (r = 0.49) compared to child-assessed BSR [r = 0.44; χ2(1) = 31.38, p < 0.001] and teacher ratings of BSR [r = 0.37, χ2(1) = 125.68, p < 0.001]. These findings provide evidence to support the concurrent and predictive validity of the EF and BSR measures.
Contributions of SES, EF, and BSR to Math Achievement at P1
First, we considered the direct effects of SES, EF, and BSR on math achievement at P1, after controlling for the influences of a set of covariates (i.e., math achievement at K1, age at K1, age at K2, age at P1, time interval between K1 and P1 assessment of math skills, and non-verbal reasoning skills). As shown in Table 2 and Figure 2, results of Model 1 indicate that SES significantly predicted P1 math achievement (β = 0.08, p = 0.003). After including measures of EF and BSR in Model 2, SES was still a significant predictor of P1 math achievement (β = 0.06, p = 0.015).
Next, we considered the direct effects of EF and BSR on P1 math achievement, with SES in the same model. Results of Model 2 (see Table 2 and Figure 3) show that EF significantly contributed to P1 math achievement, even when considered together with SES and BSR (β = 0.12, p = 0.000). Teacher-rated BSR (β = 0.10, p = 0.013) and child-assessed BSR (β =0 .08, p = 0.027) also significantly contributed to P1 math achievement. Among the SES, EF, and BSR variables, EF emerged as the strongest predictor of P1 math achievement (i.e., 1 SD increase in EF is related to a 0.12 SD increase in P1 math scores) after considering the contribution of other covariates. Notably, K1 math achievement was the strongest predictor of P1 math achievement overall (β = 0.55, p = 0.000).
Do EF and BSR Mediate the Association Between SES and Math Achievement at P1?
The results of the mediation model are shown in Table 2 (see Model 3) and Figure 4. Children’s EF skills at K2 significantly and partially mediated the association between SES and P1 math achievement (β = 0.02, p = 0.008). Similarly, child-assessed BSR partially mediated the association between SES and P1 math achievement (β = 0.01, p = 0.041). The results for teacher-rated BSR were not conclusive; whereas the statistical significance test indicates that teacher-rated BSR was not a significant mediator (β = 0.01, p = 0.140), the bootstrapped confidence interval result suggests a significant mediating effect. Given these contradictory results, we take the conservative approach of concluding that teacher-rated BSR did not significantly mediate between SES and P1 math achievement. Importantly, the direct effect of SES on P1 math achievement remained statistically significant (β = 0.06, p = 0.018) after accounting for the mediating influences of EF and BSR. Overall, the effect sizes of the mediating effects involving EF and child-assessed BSR were very small (equivalent to 0.01 to 0.02 SD), compared to the direct effects of EF (0.12 SD) and child-assessed BSR (0.07 SD) on P1 math achievement.
Discussion
In this study, we examined the contributions of SES, EF, and BSR on children’s math achievement at entry to formal education (i.e., Primary 1), and the extent to which different aspects of self-regulation mediate the SES-achievement gap. We also considered whether direct performance measures (i.e., child-assessed BSR) and teacher ratings of BSR uniquely account for math achievement at Primary 1. We found that SES, EF, and both measures of BSR directly predicted math achievement at Primary 1, even after accounting for prior math achievement at kindergarten entry. We also found that EF and child-assessed BSR represent distinct constructs of self-regulation that partially mediated the relationship between SES and math achievement at Primary 1, although it should be noted that the mediation effects were very small. Our findings build on a growing literature examining the influences of early EF and BSR for children’s math achievement (Lee and Bull, 2016; Bustamante et al., 2017) and contribute to the ongoing debate regarding the distinctions between different components of self-regulation. In the following sections, we discuss each finding in detail and consider the theoretical and practical implications of our findings.
Contributions of SES, EF, and BSR to Math Achievement at P1
Mother’s educational attainment (our chosen indicator of SES) has been found to be strongly associated with children’s pre-academic skills (Duncan and Magnuson, 2003; Nguyen et al., 2016; Davis-Kean et al., 2021). Our findings agree with much of the extant literature in showing that SES significantly predicts mathematics on entry to primary school, even after controlling for prior math achievement, EF and BSR. A 1 SD increase in SES was associated with a 0.06 SD increase in later math achievement, an effect similar in size to that seen in other recent studies. For example, Finders et al. (2021) reported a 0.06 SD difference in 3rd grade math between children from high- and low-SES families, after controlling for prior achievement and other background characteristics (age, gender, and ethnic minority status). While we are not able to make a definitive statement regarding the mechanism of this SES impact on later math achievement, one explanation for this finding is that higher-educated mothers are more likely to create quantitatively and qualitatively richer interactions with their children (Votruba-Drzal, 2003; Raviv et al., 2004; Magnuson et al., 2009; Vandermaas-Peeler et al., 2009; Dilworth-Bart, 2012). Specifically, regarding mathematics, other findings from Singapore indicate that more educated mothers engage their children more frequently in advanced numeracy activities, which in turn impacted on math achievement (Munez et al., 2021).
Beyond the contribution of SES and prior math ability, both EF and BSR directly and uniquely contributed to later math achievement, supporting previous findings that these represent distinct aspects of broader self-regulation (Duncan et al., 2017; Anthony and Ogg, 2019; Compagnoni et al., 2019). A 1 SD increase in these skills resulted in an increase in later math skills ranging from 0.08 SD (child-assessed BSR) to 0.10 SD (teacher ratings of BSR) to 0.12 SD (child-assessed EF). The effect size for EF is similar to that found in recent studies. For example, Morgan et al. (2019) focusing on independent aspects of EF (measured at kindergarten) in predicting 2nd grade math reported effect sizes ranging from 0.06 (working memory) to 0.10 (inhibitory control) and 0.13 (cognitive flexibility).
Our findings also align with previous studies showing a relationship between BSR and math achievement (McClelland et al., 2014; Sasser et al., 2015; Beisly et al., 2020), although note that most previous studies have not considered different methods of assessing BSR within the same sample. A key addition from the current study is the finding that teacher ratings of BSR and child-assessed BSR (HTKS) each uniquely predicted later math achievement, suggesting that each capture different applications of BSR skills which are important for math achievement. Teacher-rated BSR skills are most aligned with context-specific learning related behaviors and skills (e.g., working cooperatively, following directions) that enable children to engage and persevere in a wide range of learning opportunities in the classroom (Beisly et al., 2020). In contrast, child-assessed BSR could be conceived as non-contextual (i.e., not specific to the classroom), focusing on children’s ability to integrate different EF skills into their behavior to complete a novel task (McClelland et al., 2014).
Do EF and BSR Mediate the Association Between SES and Math Achievement at P1?
Our study extends past research on the domain-general mediators of the SES-achievement relation by examining concurrent pathways via three facets of self-regulation: EF, child-assessed (non-contextual) BSR, and teacher-rated (classroom) BSR. As suggested by other scholars (e.g., Sektnan et al., 2010), we included both direct and other-reported assessments of BSR for a clearer examination of the role of BSR in the SES-achievement link. We found unique pathways through which the relationship between SES and math achievement is partially mediated by different aspects of self-regulation, further elucidating the SES-achievement gap. Importantly, the SES-achievement gap is under-estimated when we only consider SES on its own and neglect the additional, unique pathways through which SES further indirectly impacts achievement.
Our finding that EF partially mediates between SES and math achievement further corroborates previous work, which has shown that EF is a key factor explaining the relationship between SES and academic achievement (e.g., Fitzpatrick et al., 2014; Waters et al., 2021). Furthermore, while many studies use one task to index each EF component (e.g., Nesbitt et al., 2013), we employed a more comprehensive approach by using multiple tasks for each component. The association between SES and EF may be explained by different factors, including SES-related differences in parental scaffolding and responsiveness, as well as maternal and child language (Blakey et al., 2020). Another pathway is through poverty-related experiences of chronic stress, which lead to changes in the biological stress response that could detrimentally affect EF (Blair and Raver, 2012). The exact pathway that explains our current findings is unclear as the mechanism(s) may differ according to individual circumstances and extent of SES disadvantage (Blakey et al., 2020).
Child-assessed (non-contextual) BSR also partially accounts for the SES disparity in math achievement, with a similar effect size as the EF mediation pathway. However, teacher-rated (classroom) BSR, which assesses children’s ability to self-regulate their behavior in the classroom (e.g., remembering a classroom rule to raise hand before participating, following directions, and maintaining attention in the presence of distractors), did not mediate the relationship between SES and math achievement. It should be noted though that this latter finding was not completely conclusive, with conflicting results regarding the statistical significance of the mediation effect. One reason for the weaker, inconclusive pathway via teacher-rated (classroom) BSR could be a weaker influence of SES on this variable, relative to contributions from other factors not considered in this study. This is supported by the observation that the proportion of variance explained by the predictors (SES, non-verbal reasoning skills and age at K2) was much lower for teacher-rated BSR compared to that for EF and child-assessed (non-contextual) BSR. For instance, children may pick up learning-related skills and behaviors that contribute to classroom BSR through teacher’s scaffolding and peer modelling, hence attenuating the impact of SES. It has been suggested that the preschool environment provides opportunities for children to practice applying self-regulation, which to some extent, compensates for the purported diminished opportunities for children from disadvantaged social backgrounds (Cebolla-Boado et al., 2017; McClelland et al., 2018). While these opportunities may also enhance basic EF skills, effects may be more apparent on children’s classroom BSR, which may be more amenable to socialization from outside the home environment.
Unlike previous studies (e.g., Waters et al., 2021), SES remained as a direct predictor of math in the mediation model. This suggests that SES also operates through alternative mechanisms to impact on math outcomes. Some possibilities have been suggested in recent studies. For example, Davis-Kean et al. (2021) note that higher parental education provides the knowledge and belief system that enrichment activities outside the home are important for children’s academic development. Certainly, in the case of Singapore, the availability of external tuition is more readily accessible to higher income families (Teng, 2015). In Cebolla-Boado et al. (2017) study across 28 countries, Singapore was one of the very few where parental education and children’s time in preschool showed complementary (instead of substitution) effects on fourth-grade reading literacy. In other words, preschool attendance was more beneficial for children of more advantaged social backgrounds (i.e., more highly educated parents) compared to their less advantaged peers. These findings suggest that the mechanisms underlying the SES-achievement link may be more complex in sociocultural settings like Singapore.
Limitations and Future Directions
Certain limitations warrant discussion. Our use of multiple measures of each EF component and a latent EF factor has both strengths and limitations. On the one hand, a latent construct provides a “purer” assessment of EF by capturing the common variance of multiple tasks and aligns with developmental findings that EF’s are difficult to differentiate as unique skills in this age range (e.g., Blakey et al., 2020; Wiebe et al., 2008). However, we are not able to confirm whether this relationship of EF to later math is driven more strongly by one specific EF component. For example, Waters et al. (2021) found that only working memory mediated the association between SES and math achievement, after controlling for prior achievement, a finding supported by other studies showing differential contributions of EF skills to academic outcomes (Ahmed et al., 2019; Nguyen and Duncan, 2019). In contrast, Finders et al. (2021) found that multiple aspects of EF (attentional flexibility and working memory) each contributed to explaining the SES achievement gap. Both approaches (i.e., using one versus multiple latent factors) have their merits. Using a latent EF factor was better suited for our purpose of delineating the broader conceptual categories within self-regulation, rather than their componential competencies. It should be noted that the factor loading of the Simon task on the latent EF factor is below the commonly accepted threshold of 0.40, which suggests that this task has a smaller contribution to the latent EF factor.
In this study, we modelled EF and two aspects of BSR as concurrent mediators between SES and math achievement. However, other models have also been discussed in the literature. In one model, BSR is positioned as a mediator between EF and achievement in early childhood (Nesbitt et al., 2015; Anthony and Ogg, 2019), with some evidence for partial mediation (Anthony and Ogg, 2019). According to this model, EF operates as a distal predictor of academic achievement by fostering more effective learning-related behaviors (e.g., motivation, engagement, and attention) that promote engagement in learning opportunities in the classroom (Nesbitt et al., 2015; Sasser et al., 2015). In another model, EF and BSR are conceived as moderators in the SES-achievement link (e.g., Robinson, 2013; Beisly et al., 2020), based on findings indicating that these skills are particularly important to children from low SES backgrounds (Razza et al., 2015). Beisly et al. (2020) found that for children from lower SES families, having higher BSR skills was associated with better math outcomes and vice versa. For children from higher SES families, BSR was unrelated to math outcomes. These alternative models highlight the complex ways in which SES, self-regulation, and children’s academic achievement are interrelated (Beisly et al., 2020). Future studies may be designed to compare the viability of these models within a study using the same sample and measures to facilitate model comparisons.
Implications
The current study presents several theoretical and practical implications. First, our study contributes to a growing body of evidence indicating that individual differences in self-regulation skills explain a small piece of the SES-math achievement gap at entry to formal education. Building on previous findings, we further elucidated the SES-achievement gap by showing that two different aspects of self-regulation—EF and BSR—partially mediate between SES and math achievement. That these mediating paths are unique and independent also suggest that their effects are additive in nature. One important practical implication of this finding is that interventions focused on enhancing EF alone may not suffice; such efforts should also target BSR. We return to this point later.
Our study underscores the value of examining the influence of multiple facets of self-regulation on the SES-achievement gap within the same study. Specifically, it allowed us to tease apart the distinct influence of EF and BSR (despite their overlapping variance) as well as the contributions of different aspects of BSR. At the same time, our findings highlight important issues related to the broader debate about the conceptualization and measurement of EF and BSR (Morrison and Grammer, 2016). A variety of EF variables have been used in previous studies, including latent factors, composite variables, and individual EF task scores. In a similar vein, different measures have been employed in studies utilizing other-reported assessments of BSR (e.g., the Child Behavior Rating Scale and the Children’s Behavior Questionnaire). Not surprisingly, this has contributed to significant challenges in integrating findings from different studies. Although some of the decisions around the choice of measurement strategy is driven by contextual factors (e.g., the factor structure of EF may differ across countries and age groups), part of the problem stems from lack of agreement in terminology, levels of analysis, and measurement among related constructs across fields (Nigg, 2017).
Examining BSR using a child-assessed measure (HTKS) and teacher ratings (CBRS), together with child-assessed EF, allowed us to shed some light on this issue, in particular the ongoing contention regarding the classification of HTKS as measuring BSR (e.g., McClelland et al., 2014) or EF (e.g., Duncan et al., 2017; Compagnoni et al., 2019; Beisly et al., 2020). McClelland et al. (2014) argues that the HTKS requires the integration of EF (attention, working memory, inhibition, and cognitive flexibility) into behavioral control and thus reflects BSR. In contrast, Duncan et al. (2017) argues that HTKS is conceptually more aligned with conventional EF assessments than teacher-rated classroom BSR, where broader context-specific behaviors may come into play. We found the mediation effects involving HTKS and EF to be more similar than those between HTKS and CBRS. With the pathways maintaining unique contributions to the SES-achievement relation, it makes sense to consider HTKS and CBRS as distinct measures of self-regulation. For greater conceptual and empirical clarity in future studies, we propose using the term non-contextual BSR to refer to HTKS.
It has been argued that adult-report measures of BSR provide a better assessment of the application of authentic self-regulatory behaviors in real-world conditions compared to child-assessed BSR (Howard et al., 2019). While direct observational measures provide a non-biased assessment of children’s skills in regulating their behavior in highly structured one-on-one situations, teacher ratings capture children’s ability to independently apply their behavioral and affective self-regulatory abilities across context and time (Toplak et al., 2013). This may provide a more accurate picture of the child compared to e.g., a one-off direct assessment conducted by an adult unknown to the child. However, measures that rely on one teacher reporting on multiple children are susceptible to rater bias or shared effects of a classroom environment that induce students to behave more similarly to one another (Waterman et al., 2012; Schmitt et al., 2014). This highlights the importance of disaggregating variance at the teacher/classroom level from variance at the child level to ensure more accurate individual level estimates, as we have done in the present study.
From a practical perspective, our results suggest that enhancing children’s EF and BSR in multiple structured and unstructured contexts may help reduce the SES disparities in math achievement and would provide causal evidence for the current longitudinal findings. Our research suggests that interventions aimed at supporting children’s math achievement require a two-pronged approach. For children from disadvantaged backgrounds who tend to exhibit poorer EF skills at school entry (Lawson et al., 2016), an effective approach might be to focus on strengthening their EF to ensure they have adequate core cognitive skills for engaging in mathematical learning activities. A second approach is to improve and support children’s BSR skills. This can be achieved by providing high-quality instructional experiences as well as consistent, engaging, and well-structured learning opportunities (Duncan et al., 2018; Sung and Wickrama, 2018) that promote the use and development of BSR skills. For example, teachers could be more explicit about what is involved in active listening and paying attention and provide time for focused exploration with learning materials to help children develop self-regulation (Beisly et al., 2020). In view of recent evidence that EF-focused interventions show limited far transfer effects on either reading or math achievement (e.g., Ang et al., 2015; Jacob and Parkinson, 2015; Melby-Lervåg et al., 2016; Anthony and Ogg, 2019), an approach that combines EF training with opportunities to practice and develop BSR in the classroom might yield more promising results. This would align with McClelland et al. (2018) perspective that interventions may be more effective when they integrate multiple levels of influence across different contexts and allow repeated practice of skills that are relevant to behavior in everyday settings.
Conclusion
The achievement gap between socioeconomically advantaged and disadvantaged children is pervasive and likely plays a key role in the inter-generational transmission of poverty (Fitzpatrick et al., 2014). Using different assessments of EF and BSR, our study shows that both components of self-regulation play a small role in explaining SES disparities in math achievement. Our findings further suggest that a balanced focus on enhancing EF and BSR skills of children from low-SES families may help to attenuate the SES-math achievement gap. More generally, our research contributes new insights to the ongoing debate about the distinction between EF and BSR, as well as the value of differentiating between contextual and non-contextual aspects of BSR.
Data Availability Statement
The raw data supporting the conclusions of this article will be made available by the authors, without undue reservation.
Ethics Statement
The studies involving human participants were reviewed and approved by Nanyang Technological University Institutional Review Board. Written informed consent to participate in this study was provided by the participant’s legal guardian/next of kin.
Author Contributions
ELN and RB contributed to conception and design of the study. ELN performed the statistical analysis and wrote the first draft of the manuscript. All authors wrote sections of the manuscript. All authors contributed to manuscript revision, read, and approved the submitted version.
Funding
This study was funded by Singapore Ministry of Education (MOE) under the Education Research Funding Programme (OER 09/14 RB) and administered by National Institute of Education (NIE), Nanyang Technological University, Singapore. Any opinions, findings, and conclusions or recommendations expressed in this material are those of the author(s) and do not necessarily reflect the views of the Singapore MOE and NIE.
Conflict of Interest
The authors declare that the research was conducted in the absence of any commercial or financial relationships that could be construed as a potential conflict of interest.
Publisher’s Note
All claims expressed in this article are solely those of the authors and do not necessarily represent those of their affiliated organizations, or those of the publisher, the editors and the reviewers. Any product that may be evaluated in this article, or claim that may be made by its manufacturer, is not guaranteed or endorsed by the publisher.
Footnotes
1We also explored the suitability of a 2-factor model comprising a combined shifting and inhibition factor, and a working memory factor (Lee et al., 2013). This model produced similar fit indices as the 1-factor model (RMSEA = 0.05, CFI = 0.98, and SRMR = 0.03). However, the two latent factors were highly correlated (r = 0.75), suggesting the two factors contributed little unique explanatory power. Thus, the 1-factor model was selected for this study because of parsimony.
References
Ahmed, S. F., Tang, S., Waters, N. E., and Davis-Kean, P. (2019). Executive Function and Academic Achievement: Longitudinal Relations from Early Childhood to Adolescence. J. Educ. Psychol. 111, 446–458. doi:10.1037/edu0000296
Aikens, N. L., and Barbarin, O. (2008). Socioeconomic Differences in reading Trajectories: The Contribution of Family, Neighborhood, and School Contexts. J. Educ. Psychol. 100 (2), 235–251. doi:10.1037/0022-0663.100.2.235
Ang, S. Y., Lee, K., Cheam, F., Poon, K., and Koh, J. (2015). Updating and Working Memory Training: Immediate Improvement, Long-Term Maintenance, and Generalisability to Non-trained Tasks. J. Appl. Res. Mem. Cogn. 4 (2), 121–128. doi:10.1016/j.jarmac.2015.03.001
Anthony, C. J., and Ogg, J. (2019). Executive Function, Learning-Related Behaviors, and Science Growth from Kindergarten to Fourth Grade. J. Educ. Psychol. 112 (8), 1563–1581. doi:10.1037/edu0000447
Becker, D. R., Miao, A., Duncan, R., and McClelland, M. M. (2014). Behavioral Self-Regulation and Executive Function Both Predict Visuomotor Skills and Early Academic Achievement. Early Child. Res. Q. 29 (4), 411–424. doi:10.1016/j.ecresq.2014.04.014
Beisly, A., Kwon, K.-A., Jeon, S., and Lim, C. (2020). The Moderating Role of Two Learning Related Behaviours in Preschool Children's Academic Outcomes: Learning Behaviour and Executive Function. Early Child. Dev. Care, 1–16. doi:10.1080/03004430.2020.173236
Blair, C., and Raver, C. C. (2012). Child Development in the Context of Adversity: Experiential Canalization of Brain and Behavior. Am. Psychol. 67 (4), 309–318. doi:10.1037/a0027493
Blair, C., and Raver, C. C. (2015). School Readiness and Self-Regulation: a Developmental Psychobiological Approach. Annu. Rev. Psychol. 66, 711–731. doi:10.1146/annurev-psych-010814-015221
Blakey, E., Matthews, D., Cragg, L., Buck, J., Cameron, D., and Higgins, B. (2020). The Role of Executive Functions in Socioeconomic Attainment Gaps: Results From A Randomized Controlled Trial. Child Develop. 91 (5), 1594–1614. doi:10.1111/cdev.13358
Bradley, R. H., and Corwyn, R. F. (2002). Socioeconomic Status and Child Development. Annu. Rev. Psychol. 53 (1), 371–399. doi:10.1146/annurev.psych.53.100901.135233
Bronfenbrenner, U., and Morris, P. A. (2006). “The Bioecological Model of Human Development,” in Handbook of Child Psychology: Theoretical Models of Human Development. Editors W. Damon, and R. M. Lerner (New York, NY: Wiley), 793–828.
Bronson, M. B., Tivnan, T., and Seppanen, P. S. (1995). Relations between Teacher and Classroom Activity Variables and the Classroom Behaviors of Prekindergarten Children in Chapter 1 Funded Programs. J. Appl. Develop. Psychol. 16 (2), 253–282. doi:10.1016/0193-3973(95)90035-7
Bull, R., and Bautista, A. (2018). “A Careful Balancing Act: Evolving and Harmonizing a Hybrid System of ECEC in Singapore,” in The Early Advantage I – Early Childhood Systems that lead by Example: A Comparative Focus on International Early Childhood Education. Editor S. L. Kagan (New York, NY: Teachers College Press), 155–181.
Burkam, D. T., Ready, D. D., Lee, V. E., and LoGerfo, L. F. (2004). Social-class Differences in Summer Learning between Kindergarten and First Grade: Model Specification and Estimation. Sociol. Educ. 77 (1), 1–31. doi:10.1177/003804070407700101
Bustamante, A. S., White, L. J., and Greenfield, D. B. (2017). Approaches to Learning and School Readiness in Head Start: Applications to Preschool Science. Learn. Individ. Differ. 56, 112–118. doi:10.1016/j.lindif.2016.10.012
Cebolla-Boado, H., Radl, J., and Salazar, L. (2017). Preschool Education as the Great Equalizer? A Cross-Country Study into the Sources of Inequality in reading Competence. Acta Sociol. 60 (1), 41–60. doi:10.1177/0001699316654529
Compagnoni, M., Karlen, Y., and Maag Merki, K. (2019). Play it Safe or Play to Learn: Mindsets and Behavioral Self-Regulation in Kindergarten. Metacognition Learn. 14 (3), 291–314. doi:10.1007/s11409-019-09190-y
Conway, A., Waldfogel, J., and Wang, Y. (2018). Parent Education and Income Gradients in Children's Executive Functions at Kindergarten Entry. Child. Youth Serv. Rev. 91, 329–337. doi:10.1016/j.childyouth.2018.06.009
Davis-Kean, P. E., Tighe, L. A., and Waters, N. E. (2021). The Role of Parent Educational Attainment in Parenting and Children's Development. Curr. Dir. Psychol. Sci. 30 (2), 186–192. doi:10.1177/0963721421993116
Department of Statistics (2015). General Household Survey 2015. Singapore: Ministry of Trade and Industry. ISBN: 978-981-09-8924-8.
Diamond, A., Carlson, S. M., and Beck, D. M. (2005). Preschool Children's Performance in Task Switching on the Dimensional Change Card Sort Task: Separating the Dimensions Aids the Ability to Switch. Dev. Neuropsychol. 28 (2), 689–729. doi:10.1207/s15326942dn2802_7
Dilworth-Bart, J. E. (2012). Does Executive Function Mediate SES and home Quality Associations with Academic Readiness? Early Child. Res. Q. 27 (3), 416–425. doi:10.1016/j.ecresq.2012.02.002
Domina, T., and Roksa, J. (2012). Should Mom Go Back to School? Post-natal Educational Attainment and Parenting Practices. Soc. Sci. Res. 41 (3), 695–708. doi:10.1016/j.ssresearch.2011.12.002
Duncan, G. J., Dowsett, C. J., Claessens, A., Magnuson, K., Huston, A. C., Klebanov, P., et al. (2007). School Readiness and Later Achievement. Dev. Psychol. 43 (6), 1428–1446. doi:10.1037/0012-1649.43.6.1428
Duncan, G. J., and Magnuson, K. (2012). Socioeconomic Status and Cognitive Functioning: Moving from Correlation to Causation. Wiley Interdiscip. Rev. Cogn. Sci. 3, 377–386. doi:10.1002/wcs.1176
Duncan, G. J., and Magnuson, K. A. (2003). “Off with Hollingshead: Socioeconomic Resources, Parenting, and Child Development,” in Socioeconomic Status, Parenting, and Child Development. Editors M. H. Bornstein, and R. H. Bradley (New York, NY: Lawrence Erlbaum), 83–106.
Duncan, R. J., Schmitt, S. A., Burke, M., and McClelland, M. M. (2018). Combining a Kindergarten Readiness Summer Program with a Self-Regulation Intervention Improves School Readiness. Early Child. Res. Q. 42, 291–300. doi:10.1016/j.ecresq.2017.10.012
Duncan, R. J., McClelland, M. M., and Acock, A. C. (2017). Relations between Executive Function, Behavioral Regulation, and Achievement: Moderation by Family Income. J. Appl. Develop. Psychol. 49, 21–30. doi:10.1016/j.appdev.2017.01.004
Ellefson, M. R., Zachariou, A., Ng, F. F., Wang, Q., and Hughes, C. (2020). Do executive Functions Mediate the Link between Socioeconomic Status and Numeracy Skills? A Cross-Site Comparison of Hong Kong and the United Kingdom. J. Exp. Child. Psychol. 194, 104734. doi:10.1016/j.jecp.2019.104734
Elliott, L., and Bachman, H. J. (2018). SES Disparities in Early Math Abilities: The Contributions of Parents' Math Cognitions, Practices to Support Math, and Math Talk. Develop. Rev. 49, 1–15. doi:10.1016/j.dr.2018.08.001
Evans, G. W., and Rosenbaum, J. (2008). Self-regulation and the Income-Achievement gap. Early Child. Res. Q. 23 (4), 504–514. doi:10.1016/j.ecresq.2008.07.002
Fan, J., McCandliss, B. D., Sommer, T., Raz, A., and Posner, M. I. (2002). Testing the Efficiency and independence of Attentional Networks. J. Cogn. Neurosci. 14, 340–347. doi:10.1162/089892902317361886
Finders, J. K., McClelland, M. M., Geldhof, G. J., Rothwell, D. W., and Hatfield, B. E. (2021). Explaining Achievement Gaps in Kindergarten and Third Grade: The Role of Self-Regulation and Executive Function Skills. Early Child. Res. Q. 54, 72–85. doi:10.1016/j.ecresq.2020.07.008
Fitzpatrick, C., McKinnon, R. D., Blair, C. B., and Willoughby, M. T. (2014). Do preschool Executive Function Skills Explain the School Readiness gap between Advantaged and Disadvantaged Children? Learn. Instruc. 30, 25–31. doi:10.1016/j.learninstruc.2013.11.003
Fuhs, M. W., Farran, D. C., and Nesbitt, K. T. (2015). Prekindergarten Children's Executive Functioning Skills and Achievement Gains: The Utility of Direct Assessments and Teacher Ratings. J. Educ. Psychol. 107 (1), 207–221. doi:10.1037/a0037366
Geary, D. C., Bailey, D. H., and Hoard, M. K. (2009). Predicting Mathematical Achievement and Mathematical Learning Disability with a Simple Screening Tool: The Number Sets Test. J. Psychoeduc Assess. 27, 265–279. doi:10.1177/0734282908330592
Ginsburg, H., and Baroody, A. (2003). TEMA-3 Test of Early Mathematics Ability: Examiner's Manual (Austin, TX: Pro-Ed.
Gioia, G. A., Isquith, P. K., Guy, S. C., and Kenworthy, L. (2000). TEST REVIEW Behavior Rating Inventory of Executive Function. Child. Neuropsychol. 6 (3), 235–238. doi:10.1076/chin.6.3.235.3152
Hackman, D. A., Gallop, R., Evans, G. W., and Farah, M. J. (2015). Socioeconomic Status and Executive Function: Developmental Trajectories and Mediation. Dev. Sci. 18 (5), 686–702. doi:10.1111/desc.12246
Harding, J. F., Morris, P. A., and Hughes, D. (2015). The Relationship between Maternal Education and Children's Academic Outcomes: A Theoretical Framework. Fam. Relat. 77 (1), 60–76. doi:10.1111/jomf.12156
Howard, S. J., Neilsen-Hewett, C., de Rosnay, M., Vasseleu, E., and Melhuish, E. (2019). Evaluating the Viability of a Structured Observational Approach to Assessing Early Self-Regulation. Early Child. Res. Q. 48, 186–197. doi:10.1016/j.ecresq.2019.03.003
Huizinga, M., Dolan, C. V., and van der Molen, M. W. (2006). Age-related Change in Executive Function: Developmental Trends and a Latent Variable Analysis. Neuropsychologia 44 (11), 2017–2036. doi:10.1016/j.neuropsychologia.2006.01.010
Jacob, R., and Parkinson, J. (2015). The Potential for School-Based Interventions that Target Executive Function to Improve Academic Achievement. Rev. Educ. Res. 85, 512–552. doi:10.3102/0034654314561338
Jordan, N. C., Kaplan, D., Nabors Oláh, L., and Locuniak, M. N. (2006). Number Sense Growth in Kindergarten: A Longitudinal Investigation of Children at Risk for Mathematics Difficulties. Child. Dev. 77 (1), 153–175. doi:10.1111/j.1467-8624.2006.00862.x
KidSTART Singapore (2020). About KidSTART. Available at: https://www.kidstart.sg/about-us/ (Accessed June 23, 2021).
Law, D. J., Morrin, K. A., and Pellegrino, J. W. (1995). Training Effects and Working Memory Contributions to Skill Acquisition in a Complex Coordination Task. Learn. Individ. Differ. 7 (3), 207–234. doi:10.1016/1041-6080(95)90011-x
Lawson, G. M., and Farah, M. J. (2015). Executive Function as a Mediator between SES and Academic Achievement throughout Childhood. Int. J. Behav. Dev. 41 (1), 94–104. doi:10.1177/0165025415603489
Lawson, G. M., Hook, C. J., and Farah, M. J. (2018). A Meta-Analysis of the Relationship between Socioeconomic Status and Executive Function Performance Among Children. Dev. Sci. 21, e12529. doi:10.1111/desc.12529
Lawson, G. M., Hook, C. J., Hackman, D. A., and Farah, M. J. (2016). “Socioeconomic Status and the Development of Executive Function: Behavioral and Neuroscience Approaches,” in Executive Function in Preschool Children: Integrating Measurement, Neurodevelopment, and Translational Research. Editors J. A. Griffin, P. McCardle, and L. S. Freund (Washington DC, USA: American Psychological Association), 259–278. doi:10.1037/14797-012
Lee, K., Bull, R., and Ho, R. M. (2013). Developmental Changes in Executive Functioning. Child. Dev. 84 (6), 1933–1953. doi:10.1111/cdev.12096
Lee, K., Ng, S. F., Pe, M. L., Ang, S. Y., Hasshim, M. N., and Bull, R. (2012). The Cognitive Underpinnings of Emerging Mathematical Skills: Executive Functioning, Patterns, Numeracy, and Arithmetic. Br. J. Educ. Psychol. 82 (1), 82–99. doi:10.1111/j.2044-8279.2010.02016.x
Lee, K., and Bull, R. (2016). Developmental Changes in Working Memory, Updating, and Math Achievement. J. Educ. Psychol. 108 (6), 869–882. doi:10.1037/edu0000090
Lenes, R., Gonzales, C. R., Størksen, I., and McClelland, M. M. (2020). Children's Self-Regulation in Norway and the United States: The Role of Mother's Education and Child Gender across Cultural Contexts. Front. Psychol. 11, 566208–566217. doi:10.3389/fpsyg.2020.566208
Li-Grining, C. P., Votruba-Drzal, E., Maldonado-Carreño, C., and Haas, K. (2010). Children's Early Approaches to Learning and Academic Trajectories through Fifth Grade. Dev. Psychol. 46 (5), 1062–1077. doi:10.1037/a0020066
Lim, S. M., Rodger, S., and Brown, T. (2010). Validation of Child Behavior Rating Scale in Singapore (Part 1): Rasch Analysis. Hong Kong J. Occup. Ther. 20 (2), 52–62. doi:10.1016/S1569-18611170004-310.1016/s1569-1861(11)70004-3
Little, M. (2017). Racial and Socioeconomic Gaps in Executive Function Skills in Early Elementary School. Educ. Res. 46, 103–109. doi:10.3102/0013189X17698700
Little, R. J. A. (1988). A Test of Missing Completely at Random for Multivariate Data with Missing Values. J. Am. Stat. Assoc. 83 (404), 1198–1202. doi:10.1080/01621459.1988.10478722
Magnuson, K. A., Sexton, H. R., Davis-Kean, P. E., and Huston, A. C. (2009). Increases in Maternal Education and Young Children's Language Skills. Merrill-Palmer Q. 55, 319–350. doi:10.1353/mpq.0.0024
McClelland, M., Geldhof, J., Morrison, F., Gestsdóttir, S., Cameron, C., Bowers, E., et al. (2018). “Self-regulation,” in Handbook of Life Course Health Development. Editors N. Halfon, C. B. Forrest, R. M. Lerner, and E. M. Faustman (New York, NY: Springer International Publishing), 275–298. doi:10.1007/978-3-319-47143-3_12
McClelland, M. M., Cameron, C. E., Connor, C. M., Farris, C. L., Jewkes, A. M., and Morrison, F. J. (2007). Links between Behavioral Regulation and Preschoolers' Literacy, Vocabulary, and Math Skills. Dev. Psychol. 43, 947–959. doi:10.1037/0012-1649.43.4.947
McClelland, M. M., Cameron, C. E., Duncan, R., Bowles, R. P., Acock, A. C., Miao, A., et al. (2014). Predictors of Early Growth in Academic Achievement: The Head-Toes-Knees-Shoulders Task. Front. Psychol. 5, 599. doi:10.3389/fpsyg.2014.00599
McClelland, M. M., and Cameron, C. E. (2012). Self-Regulation in Early Childhood: Improving Conceptual Clarity and Developing Ecologically Valid Measures. Child. Dev. Perspect. 6 (2), 136–142. doi:10.1111/j.1750-8606.2011.00191.x
McClelland, M. M., Morrison, F. J., and Holmes, D. L. (2000). Children at Risk for Early Academic Problems: The Role of Learning-Related Social Skills. Early Child. Res. Q. 15 (3), 307–329. doi:10.1016/S0885-2006(00)00069-7
McLoyd, V. C. (1998). Socioeconomic Disadvantage and Child Development. Am. Psychol. 53 (2), 185–204. doi:10.1037/0003-066x.53.2.18510.1037//0003-066x.53.2.185
Melby-Lervåg, M., Redick, T. S., and Hulme, C. (2016). Working Memory Training Does Not Improve Performance on Measures of Intelligence or Other Measures of "Far Transfer". Perspect. Psychol. Sci. 11 (4), 512–534. doi:10.1177/1745691616635612
Mistry, R. S., Benner, A. D., Biesanz, J. C., Clark, S. L., and Howes, C. (2010). Family and Social Risk, and Parental Investments during the Early Childhood Years as Predictors of Low-Income Children's School Readiness Outcomes. Early Child. Res. Q. 25 (4), 432–449. doi:10.1016/j.ecresq.2010.01.002
Miyake, A., Friedman, N. P., Emerson, M. J., Witzki, A. H., Howerter, A., and Wager, T. D. (2000). The unity and Diversity of Executive Functions and Their Contributions to Complex “Frontal Lobe” Tasks: A Latent Variable Analysis. Cogn. Psychol. 41, 49–100. doi:10.1006/cogp.1999.0734
Morgan, P. L., Farkas, G., Hillemeier, M. M., Pun, W. H., and Maczuga, S. (2019). Kindergarten Children's Executive Functions Predict Their Second-Grade Academic Achievement and Behavior. Child. Dev. 90 (5), 1802–1816. doi:10.1111/cdev.13095
Morrison, F. J., and Grammer, J. K. (2016). “Conceptual Clutter and Measurement Mayhem: Proposals for Cross-Disciplinary Integration in Conceptualizing and Measuring Executive Function,” in Executive Function in Preschool-Age Children: Integrating Measurement, Neurodevelopment, and Translational Research. Editors J. A. Griffin, P. McCardle, and L. S. Freund (Washington, DC: American Psychological Association), 327–348. doi:10.1037/14797-015
Muñez, D., Bull, R., and Lee, K. (2021). Socioeconomic Status, home Mathematics Environment and Math Achievement in Kindergarten: A Mediation Analysis. Develop. Sci. doi:10.1111/desc.13135
Muthén, L. K., and Muthén, B. O. (2017). Mplus User’s Guide. Eighth Edition. Los Angeles, CA: Muthén & Muthén.
Nesbitt, K. T., Farran, D. C., and Fuhs, M. W. (2015). Executive Function Skills and Academic Achievement Gains in Prekindergarten: Contributions of Learning-Related Behaviors. Dev. Psychol. 51 (7), 865–878. doi:10.1037/dev0000021
Nesbitt, K. T., Baker-Ward, L., and Willoughby, M. T. (2013). Executive Function Mediates Socio-Economic and Racial Differences in Early Academic Achievement. Early Child. Res. Q. 28 (4), 774–783. doi:10.1016/j.ecresq.2013.07.005
Neuenschwander, R., Röthlisberger, M., Cimeli, P., and Roebers, C. M. (2012). How Do Different Aspects of Self-Regulation Predict Successful Adaptation to School? J. Exp. Child. Psychol. 113 (3), 353–371. doi:10.1016/j.jecp.2012.07.004
Nguyen, T., Watts, T. W., Duncan, G. J., Clements, D. H., Sarama, J. S., Wolfe, C., et al. (2016). Which Preschool Mathematics Competencies Are Most Predictive of Fifth Grade Achievement? Early Child. Res. Q. 36, 550–560. doi:10.1016/j.ecresq.2016.02.003
Nguyen, T., and Duncan, G. J. (2019). Kindergarten Components of Executive Function and Third Grade Achievement: A National Study. Early Child. Res. Q. 46, 49–61. doi:10.1016/j.ecresq.2018.05.006
Nigg, J. T. (2017). Annual Research Review: On the Relations Among Self-Regulation, Self-Control, Executive Functioning, Effortful Control, Cognitive Control, Impulsivity, Risk-Taking, and Inhibition for Developmental Psychopathology. J. Child. Psychol. Psychiatry. 58 (4), 361–383. doi:10.1111/jcpp.12675
Noble, K. G., McCandliss, B. D., and Farah, M. J. (2007). Socioeconomic Gradients Predict Individual Differences in Neurocognitive Abilities. Dev. Sci. 10 (4), 464–480. doi:10.1111/j.1467-7687.2007.00600.x
Pickering, S. J., and Gathercole, S. E. (2001). Working Memory Test Battery for Children. Kent, UK: Psychological Corporation.
Raven, J. C., Court, J. H., and Raven, J. E. (1998). Raven's Coloured Progressive Matrices. USA: Harcourt Assessment.
Raviv, T., Kessenich, M., and Morrison, F. J. (2004). A Mediational Model of the Association between Socioeconomic Status and Three-Year-Old Language Abilities: The Role of Parenting Factors. Early Child. Res. Q. 19 (4), 528–547. doi:10.1016/j.ecresq.2004.10.007
Razza, R. A., Martin, A., and Brooks-Gunn, J. (2015). Are Approaches to Learning in Kindergarten Associated with Academic and Social Competence Similarly?. Child. Youth Care Forum 44 (6), 757–776. doi:10.1007/s10566-015-9307-0
Ritchie, S. J., and Bates, T. C. (2013). Enduring Links from Childhood Mathematics and reading Achievement to Adult Socioeconomic Status. Psychol. Sci. 24 (7), 1301–1308. doi:10.1177/0956797612466268
Robinson, K. (2013). Early Disparities in Mathematics Gains Among Poor and Non-poor Children. Elem. Sch. J. 114 (1), 22–47. doi:10.1086/670737
Sarsour, K., Sheridan, M., Jutte, D., Nuru-Jeter, A., Hinshaw, S., and Boyce, W. T. (2011). Family Socioeconomic Status and Child Executive Functions: The Roles of Language, home Environment, and Single Parenthood. J. Int. Neuropsychol. Soc. 17 (1), 120–132. doi:10.1017/S1355617710001335
Sasser, T. R., Bierman, K. L., and Heinrichs, B. (2015). Executive Functioning and School Adjustment: The Mediational Role of Pre-kindergarten Learning-Related Behaviors. Early Child. Res. Q. 30, 70–79. doi:10.1016/j.ecresq.2014.09.001
Schirmbeck, K., Rao, N., and Maehler, C. (2020). Similarities and Differences across Countries in the Development of Executive Functions in Children: a Systematic Review. Inf. Child. Dev. 29 (1), e2164. doi:10.1002/icd.2164
Schmitt, S. A., Pratt, M. E., and McClelland, M. M. (2014). Examining the Validity of Behavioral Self-Regulation Tools in Predicting Preschoolers' Academic Achievement. Early Educ. Dev. 25, 641–660. doi:10.1080/10409289.2014.850397
Sektnan, M., McClelland, M. M., Acock, A., and Morrison, F. J. (2010). Relations between Early Family Risk, Children's Behavioral Regulation, and Academic Achievement. Early Child. Res. Q. 25 (4), 464–479. doi:10.1016/j.ecresq.2010.02.005
Sirin, S. R. (2005). Socioeconomic Status and Academic Achievement: A Meta-Analytic Review of Research. Rev. Educ. Res. 75 (3), 417–453. doi:10.3102/00346543075003417
Slotkin, J., Kallen, M., Griffith, J., Magasi, S., Salsman, J., Nowinski, C., et al. (2012). NIH Toolbox Dimensional Change Card SortNIH Toolbox Technical Manual. Available at: https://www.healthmeasures.net/images/nihtoolbox/Technical_Manuals/Cognition/Toolbox_Dimensional_Change_Card_Sort_Test_Technical_Manual.pdf. (Accessed Sep 3, 2021).
Starkey, P., Klein, A., and Wakeley, A. (2004). Enhancing Young Children's Mathematical Knowledge through a Pre-kindergarten Mathematics Intervention. Early Child. Res. Q. 19 (1), 99–120. doi:10.1016/j.ecresq.2004.01.002
Sung, J., and Wickrama, K. A. S. (2018). Longitudinal Relationship between Early Academic Achievement and Executive Function: Mediating Role of Approaches to Learning. Contemp. Educ. Psychol. 54, 171–183. doi:10.1016/j.cedpsych.2018.06.010
Tan, C. T. (2017). Enhancing the Quality of Kindergarten Education in Singapore: Policies and Strategies in the 21st century. Icep 11 (1), 1–22. doi:10.1186/s40723-017-0033-y
Teng, A. (2015). Better-educated Parents with Higher Incomes Spend more. The Straits Times Singapore. Available at: https://www.straitstimes.com/singapore/education/better-educated-parents-with-higher-incomes-spend-more (Accessed Sept 3, 2021).
Toplak, M. E., West, R. F., and Stanovich, K. E. (2013). Practitioner Review: Do Performance-Based Measures and Ratings of Executive Function Assess the Same Construct? J. Child. Psychol. Psychiatry. 54 (2), 131–143. doi:10.1111/jcpp.12001
Vandermaas‐Peeler, M., Nelson, J., Bumpass, C., and Sassine, B. (2009). Numeracy‐related Exchanges in Joint Storybook reading and Play. Int. J. Early Years Educ. 17 (1), 67–84. doi:10.1080/09669760802699910
Votruba-Drzal, E. (2003). Income Changes and Cognitive Stimulation in Young Children's Home Learning Environments. J. Marriage Fam. 65 (2), 341–355. doi:10.1111/j.1741-3737.2003.00341.x
Waterman, C., McDermott, P. A., Fantuzzo, J. W., and Gadsden, V. L. (2012). The Matter of Assessor Variance in Early Childhood Education-Or Whose Score Is it Anyway? Early Child. Res. Q. 27, 46–54. doi:10.1016/j.ecresq.2011.06.003
Waters, N. E., Ahmed, S. F., Tang, S., Morrison, F. J., and Davis-Kean, P. E. (2021). Pathways from Socioeconomic Status to Early Academic Achievement: The Role of Specific Executive Functions. Early Child. Res. Q. 54, 321–331. doi:10.1016/j.ecresq.2020.09.008
Wiebe, S. A., Espy, K. A., and Charak, D. (2008). Using Confirmatory Factor Analysis to Understand Executive Control in Preschool Children: I. Latent Structure. Dev. Psychol. 44, 575–587. doi:10.1037/0012-1649.44.2.575
Willoughby, M. T., Blair, C. B., Wirth, R. J., and Greenberg, M. (2012). The Measurement of Executive Function at Age 5: Psychometric Properties and Relationship to Academic Achievement. Psychol. Assess. 24, 226–239. doi:10.1037/a0025361
Yao, S.-Y., Muñez, D., Bull, R., Lee, K., Khng, K. H., and Poon, K. (2017). Rasch Modeling of the Test of Early Mathematics Ability-Third Edition with a Sample of K1 Children in Singapore. J. Psychoeducational Assess. 35 (6), 615–627. doi:10.1177/0734282916651021
Zadeh, Z. Y., Farnia, F., and Ungerleider, C. (2010). How home Enrichment Mediates the Relationship between Maternal Education and Children's Achievement in reading and Math. Early Educ. Dev. 21 (4), 568–594. doi:10.1080/10409280903118424
Keywords: learning-related behaviors, mathematics achievement, executive function skills, self-regulation, early childhood, socioeconomic disadvantage
Citation: Ng EL, Bull R and Khng KH (2021) Accounting for the SES-Math Achievement Gap at School Entry: Unique Mediation Paths via Executive Functioning and Behavioral Self-Regulation. Front. Educ. 6:703112. doi: 10.3389/feduc.2021.703112
Received: 30 April 2021; Accepted: 31 August 2021;
Published: 13 September 2021.
Edited by:
Megan McClelland, Oregon State University, United StatesReviewed by:
Andres Bustamante, University of California, Irvine, United StatesAndrew David Ribner, New York University, United States
Copyright © 2021 Ng, Bull and Khng. This is an open-access article distributed under the terms of the Creative Commons Attribution License (CC BY). The use, distribution or reproduction in other forums is permitted, provided the original author(s) and the copyright owner(s) are credited and that the original publication in this journal is cited, in accordance with accepted academic practice. No use, distribution or reproduction is permitted which does not comply with these terms.
*Correspondence: Ee Lynn Ng, ZWVseW5uLm5nQG5pZS5lZHUuc2c=