- 1Department of Psychology, Education, and Child Studies, Erasmus University Rotterdam, Rotterdam, Netherlands
- 2Delft Institute of Applied Mathematics, Delft University of Technology, Delft, Netherlands
- 3School of Education/Early Start, University of Wollongong, Wollongong, NSW, Australia
Cognitive load researchers have used varying subjective techniques based on rating scales to quantify experienced cognitive load. Although it is generally assumed that subjects can introspect on their cognitive processes and have no difficulty in assigning numerical values to the imposed cognitive load, little is known about how visual characteristics of the rating scales influence the validity of the cognitive load measure. In this study we look at validity of four subjective rating scales (within groups) differing in visual appearance by participants rating perceived difficulty and invested mental effort in response to working on simple and complex weekday problems. We used two numerical scales (the nine-point Likert scale most often used in Cognitive load theory research and a Visual Analogue Scale ranging between 0–100%) and two pictorial scales (a scale consisting of emoticons ranging from a relaxed blue-colored face to a stressed red-colored face and an “embodied” scale picturing nine depicted weights from 1–9 kg). Results suggest that numerical scales better reflect cognitive processes underlying complex problem solving while pictorial scales Underlying simple problem solving. This study adds to the discussion on the challenges to quantify cognitive load through various measurement methods and whether subtleties in measurements could influence research findings.
Introduction
Cognitive load theory (CLT) centralizes the characteristics of human cognitive architecture, and especially the limitations of working memory in time and capacity (Baddeley, 1992, 2000), as a prerequisite for the optimization of learning. Cognitive-load researchers focus on instructional methods that can be used to manage working memory load (i.e., cognitive load). Cognitive load has been conceptualized as a multidimensional construct consisting of three types of cognitive load (e.g. Sweller, 2010), namely 1) intrinsic load that is imposed by the learning task itself, 2) extraneous load that is imposed by the design of the instruction, and 3) germane load that is related to the amount of cognitive resources that learners have available for learning. All three types of load have been proposed to be influenced by element interactivity (Sweller 2010); how many separate parts of information need to be integrated for learning to occur. During learning, initially separate information elements are categorized, organized and chunked into schemata in long-term memory, which after construction can be treated as one information element in working memory (Sweller, et al., 1998, 2019; van Merriënboer & Sweller, 2005). This process is called schematization and is a core mechanism underlying successful learning in CLT. When trying to successfully learn materials with high element interactivity, it is proposed that more mental effort needs to be invested by the learner than for materials with low element interactivity. Therefore, having a valid indication of cognitive load experienced/spent during a specific task or activity could provide crucial information on the development of a learning process and quality of an instruction.
The most widely used measures of cognitive load are subjective measures based on ratings of perceived mental effort and task difficulty (Paas, et al., 2003; Sweller, et al., 2019). There are two main assumptions underlying subjective measures of cognitive load. Firstly, it is assumed that all learners have similar clear understanding of what is meant by “invested mental effort” and “difficulty of a task”. Secondly, all learners are assumed to possess the metacognitive ability to monitor how much mental effort they have invested. Based on these assumptions, this common understanding or knowledge of the terms “invested mental effort” and “task difficulty” as well as the accuracy of individuals’ monitoring skills are not tested or controlled for when using the rating scales. Therefore, the reliability and validity of such subjective measures are debatable (e.g., Ayres, 2018). One of the issues that can arise with the cognitive-load rating scale concerns the way the scale is represented. We suggest that whether the scale represents symbols or numbers might affect the mental effort and task difficulty ratings, for examplr by imposing additional (extraneous) cognitive load. To investigate the effect of the symbolic/numerical representation of cognitive load in the rating scales on ratings of mental effort and task difficulty, we identified three alternatives to the original 9-point Likert rating classic 9-point scale in. One of these alternatives is also a symbolic representation, the second is a more affective one, representing the emotional aspect of effort and task difficulty, and the third is a more embodied one representing effort and task difficulty as weight. The central focus in the study is on construct validity; do the ratings (i.e., scores) on the measurement scale reflect the construct we intend to measure and are there differences between the scales?
One of the first subjective measures of cognitive load was developed by Paas (1992). In this study, learners were asked to indicate on a 9-point Likert scale “how much mental effort they have invested in a task”, ranging from 1 (very, very low mental effort) to 9 (very, very high mental effort). Subjective measures are advantageous for cognitive load research since they do not require a complicated experimental set-up and can be easily implemented and used multiple times in most research designs (Sweller and Paas, 2017). However, subjective measures of cognitive load have faced criticism, mainly for being implemented in research in an inconsistent way (Sweller et al., 2011). One of the inconsistencies concerns the verbal labels used to assess cognitive load. For example, instead of mental effort, learners were asked to rate the difficulty of the task by indicating on a 7- or 9-point scale “how difficult or easy the learning task was for them”, ranging from 1 (very, very easy) to 7 or 9 (very, very difficult). Studies have shown that subjective task difficulty ratings, like mental effort ratings, varied according to the level of element interactivity of a task (e.g., Ayres, 2006; Ouwehand et al., 2014). However, research suggested that the two verbal labels (i.e., mental effort and task difficulty) measure different aspects of the cognitive load De Leeuw and Mayer (2008). More specifically, (De Leeuw and Mayer, 2008), found that task difficulty ratings were related to intrinsic load and perceived mental effort to germane load, indicating the way verbal labels are being phrased in the rating scales can influence the measurement of cognitive load. Another inconsistency is the timing and frequency of measurement. Research showed that perceived mental effort and task difficulty were significantly higher when measured at the end of the learning phase (i.e., delayed) than when taking the average of the ratings obtained after each learning task (i.e., immediate) (van Gog et al., 2012; Schmeck et al., 2015).
Despite the variations in the way cognitive load has been subjectively measured in research (i.e., verbal labels and timing of measurement), both mental effort and task difficulty have been found to reliably reflect differences in the complexity of the instructional design in numerous studies (e.g., Hadie and Yusoff, 2016; Ouwehand et al., 2014; for an overview see; Paas et al., 2003). While previous research has focused on the type of measurement (e.g. physiological measurement and self-reports) and differences in the timing and verbal labels, little is known about whether the way in which the Likert scales are formatted influences the measurement of cognitive load. Sung and Wu (2018) argued that there are several issues inherent to the design of Likert scales, particularly the ambiguous numbers of the response categories and the response style underlying the ordinal measurement of data. Therefore, the aim of the current study was to explore the validity of alternative representations of Likert rating scales to measure subjective cognitive load.
The measurement validity was examined by the relationship between the subjective measures (i.e., mental effort and perceived difficulty) and the performance measures (i.e., accuracy and time on task) for simple and complex problems. Three alternative representation formats (i.e., Visual Analogue Scale, affective, and embodied) were investigated and compared with the original 9-point Likert scale for measuring cognitive load (Paas, 1992).
The first type of visual representation employed in this study was a Visual Analogue Scale (VAS). The VAS presents the numbers on a line continuum and participants can move a bar (or pin a point) between 0 and 100% to determine their level of cognitive load. Therefore, a VAS transforms ordinal-level measurement data from the discrete response categories in a Likert scale to continuous and interval-level measurement data (Sung and Wu, 2018). Research indicated that the VAS has a high test-retest reliability and a small measurement error (e.g., Alghadir et al., 2018). In addition, the VAS is a well-known measurement scale in the domain of judgments of learning (JoL) in which learners have to predict their future performance by indicating on a VAS how likely they think they will remember a just learned item on a future test (e.g., Rhodes, 2016). Recent research in the field of educational psychology called for an integration between cognitive load and self-regulated learning theories to better understand the dynamic relations between cognitive resources available for managing one’s own learning process and for the learning process itself. One of the challenges to the integration of theories of cognitive load and self-regulated learning is in measurement (Sweller and Paas, 2017). Therefore, findings from the current study can potentially pave the way for future research to determine whether VAS can be used as a common scale to measure concepts in cognitive load (i.e., mental effort and task difficulty) and self-regulated learning (i.e., JoL).
Besides the well-known and widely used original cognitive load scale and the VAS, we were interested in examining visual characteristics that display internal processes (i.e., mental and affective states). Numerical representations, which are characteristic of the original scale and the VAS, are a rather abstract reflection of internal processes. Given that grounding mental representations can support understanding (Barsalou, 2008, 2016), it is possible that a scale that reflects internal processes used when working on a task will improve the validity of the scale. Therefore, the first question of interest is whether a better reflection of internal processes could increase the validity of the rating scale. To this end, we designed two pictorial scales as a reflection of internal processes: an affective scale with icons to represent a range of emotions (i.e., emoticons) and an embodied scale with pictures of weights to represent a range of physical load.
Although scales with affective stimuli are frequently used in the media and medical practice (e.g., satisfaction reviews on products or services or pain rating scales), literature on the affect in learning and subjective rating in CLT research seems scarce. Interestingly, in one of the earliest lines of research on learning, using operant conditioning (e.g. Skinner, 1963), affect plays a central role in the learning process. According to the operant conditioning theory we (humans, but also other animals) learn from pleasant or unpleasant consequences of our actions. Put simply, actions with pleasant outcomes tend to be repeated and actions with unpleasant outcomes avoided. Since then, a lot of support for the operant learning theory has been gathered (for reviews see, Gordan and Amutan, 2014; Staddon and Cerutti, 2003). Mechanisms for the role of affect in learning has been extended by neuropsychological evidence of a reward circuit in the brain in which more primitive brain areas dealing with emotions highly interact with more recent brain areas more involved in higher-order cognitive processes such as executive processing (for a review, see O'Doherty et al., 2017). In a recent review by Shenhav et al. (2017), cognitive load (which these authors refer to as mental effort) is approached from an affective perspective by looking at a costs/benefits ratio of invested cognitive load. Because humans are limited in their resource capacity, they need to be efficient in their allocation of cognitive resources. In their review, these authors argue that investing (high) mental effort is a negative experience in terms of affect. As a consequence, a task requiring high mental effort would be experienced with more negative affect than a task requiring low mental effort. Following this perspective, affect can be a direct reflection of cognitive load. In line with this view, Sitzmann et al. (2010) showed that people are better at self-assessing affective processes (i.e., motivation and satisfaction) than purely cognitive processes. This suggests that human learners are more capable of defining emotional processes than purely cognitive processes. Based on these reasonings, we propose that a subjective rating scale depicting affect from negative (i.e. aversive) to positive, might represent the experience of mental effort of learners better than a more abstract numerical scale, and therefore, might be a more valid manner to measure invested mental effort and perceived task difficulty.
A second aspect we would like to explore is inspired by the embodied cognition theory. This theory states that cognition is grounded in perception and action (for a recent theoretical overview, see Barsalou, 2016). In other words, this theory claims that our cognitive processes and functions are shaped by the way we interact with our surroundings. Since the nineties a lot of evidence has been gathered, suggesting that for a substantial part, our cognition is tightly bound to how we perceive and interact in the world (Barsalou, 2008). However, there is an ongoing debate on whether embodied cognition only applies to the lower level cognitive abilities (i.e. procedural, motor learning) or also to more higher level cognitive abilities (i.e. conceptual learning) (for a critical review, see Caramazza et al., 2014). For the present research, the embodied view is still interesting, because the term mental or cognitive load, metaphorically indicates that a certain weight is related to the task (i.e., how “heavy” or “burdensome” a task is). Indeed the analogy of physical weight for mental effort is also used by Shenhav et al. (2017), to explain how effort mediates between capacity and performance. Interestingly, abstract metaphors such as the ‘heaviness’ of a task in our study are also empirically found to be connected to embodied cognition. For example, Zanolie et al. (2012) found an attentional bias for abstract concepts such as “power” on a vertical axis. In their experiment, participants were presented with a power-related word (either related to high or low power) after which they had to identify objects either presented on the top or bottom of a computer screen. It was found that target identification was faster for items that were presented on a semantically congruent location compared to an incongruent location. This result was explained by the process of mental simulation: humans tend to imagine perceptual and motoric features evoked by a stimulus in such a way that a single stimulus can elicit a rich image and or action plan for a situation in which the stimulus is normally encountered. Drawing further on these findings that metaphors can also facilitate cognitive processing, we added a scale depicting nine weights ranging from light (small 1 kg) to heavy (large 9 kg). In this way, we expressed the idea of mental “load” in a more concrete manner. For instance, a heavy problem or task (load) would correspond to a heavier weight. This might fit the experiences of mental effort and task difficulty (i.e., load on cognition) better than a more abstract numeric scale.
To investigate the construct validity of the different scales, we adopted the dominant approach used in cognitive load research; we used the relation between performance/learning and cognitive load ratings (for a meta-analysis see, Naismith and Cavalcanti, 2015). More specifically, we inspected correlation analyses between cognitive load ratings measured by the mental effort and task difficulty ratings on each of the four different scales (the original nine-point Likert scale, the VAS, an affective scale illustrated with emoticons, and an embodied scale illustrated with weights) with performance measured by accuracy of problem-solving and time on task for simple and complex problems. Although the current research is exploratory in nature, we would like to put forward some hypotheses.
First, based on cognitive load studies that showed that subjective measures are a valid way of measuring cognitive load, we hypothesized that perceived mental effort and difficulty would be rated higher for the complex problems than for the simple problem across all four measurement scales. Secondly, we explored whether there are differences between the different scales in differentiating effort and difficulty between simple and complex problem solving. Building on literature stating that affect is tightly related to the learning process (Shenhav et al., 2017), we suggest that emoticons, representing affect might reflect the perceived mental effort and difficulty better than a numeric scale and therefore correlate higher and more significantly to performance. Also, the relation is expected to be stronger for the complex problems, since these might be more arousing and frustrating. In support of the embodied cognition theory (e.g., Barsalou, 2008), we expect that pictures of increasing weights might represent a more concrete picture of “load” and therefore might represent perceived mental effort and difficulty better than a numeric scale. Following the argument that embodied cognition might be more related to more lower level cognitive abilities, it is expected that ratings on this scale correlate higher and (more) significantly with the performance on the simple problems. Finally, to gather insight into participants’ experience when using the different scales to rate their cognitive load, participants were asked to vote for their most and least favorite scale and give some reasoning on their (dis)likes.
Materials and Methods
The present study was conducted in accordance with the guidelines of the ethical committee of the host University. Below we describe our sample, design, materials, procedure and analysis plan.
Participants
Participants were 46 healthy young adults (Psychology students, 39 women; Mage= 22.4 years, SD = 2.45) who participated in this study as part of a course requirement. All participants gave written consent before participation. A power test using G*power3.1 software (Faul et al., 2009) showed that for the 2 (complexity) x 4 (scale type) within-subject design we use when aiming for an effect size of f = 0.25, power = 0.95 and α = 0.05, a sample with minimal 23 participants is required.
Design
In a 2 (Complexity: simple and complex questions) x 4 (Scale Type: original nine point, visual analogue, affect, and embodied) within-subjects design, participants were presented with simple and complex problems that they had to solve. Four types of rating scales were used to measure perceived difficulty and mental effort.
Materials
Problem-solving task. Sixteen weekday problems were used (Sweller, 1993; see also; van Gog et al., 2012) of which eight were low in element interactivity (simple problems) and eight high (complex problems). Table 1 shows an example of a simple and a complex problem in the problem-solving task. The simple problems consisted of two elements that students needed to consider when solving the problem while the complex problems consisted of five elements (i.e., elements are underlined in Table 1). The complex problems would be harder to solve than the simple problems because of the higher element interactivity.
Rating scales. Cognitive load was measured by two self-report items, one item was to assess perceived difficulty “Indicate on this scale how difficult you found the problem” and the other was to assess invested mental effort “Indicate on this scale how much mental effort it cost you to solve the problem? Four types of rating scales were investigated in this study. The first type of rating scale was the original 9-point Likert rating scale of Paas (1992) ranging from 1 (very, very easy) to 9 (very, very difficult). The second type of rating scale was a Visual Analogue Scale (VAS) in which participants could move a bar on a line representing a 0–100% continuum. The third type of rating scale, also termed as the affective scale, was a self-designed 9-point scale made up of emoticons ranging from a blue emoticon depicting a relaxed expression to a red emoticon depicting a stressed/aroused expression. The fourth type of rating scale, also termed as the embodied scale, was a self-designed 9-point scale presenting pictures of increasing weights ranging from 1–9 kg Figure 1 illustrates the four types of rating scales.
Procedure
The experiment was constructed using an online survey platform, Qualtrics (https://www.qualtrics.com/). A link to access the experiment was shared with the participants. Figure 2 illustrates the procedure of the experiment. At the start of the experiment, participants were instructed to not use paper and pencil or other external tools for the problem-solving task. They were also informed that there was a time limit to solve the problems. After this instruction, they were given a practice problem to gain familiarity with the task before proceeding to the first block of questions.
Altogether, participants had to solve four blocks of questions. In each block, participants had to solve four problems comprising two simple and two complex problems. After each problem, participants had to rate their rate mental effort and perceived difficulty (i.e., 16 times in total). The rating scale presented to the participants varied in each block (original 9-point Likert scale, VAS, emoticon, weights). The four blocks were counterbalanced so that the order in which the different type of rating scales that were presented to the participants were not the same. At the end of the experiment, participants were asked to indicate the rating scale that they liked best and the one that they liked the least out of the four types of rating scales.
Scoring of the dependent variables
Performance. Performance was measured by accuracy scores and time on task. For each correctly solved problem, one point was assigned. The mean accuracy was determined for each difficulty level (simple and complex) within each scale type (original 9-point rating scale, VAS, emoticons and depicted weights), resulting in two mean accuracy scores for each rating scale type (i.e., one for complex problems and one for simple problems). Altogether, eight mean accuracy scores were calculated for each participant. Time on task was determined by the duration (in seconds) participants took to submit their response.
Rating (invested mental effort and perceived difficulty). To make all rating scores comparable, proportion scores were calculated by dividing the obtained scores by the maximum scores: For the original 9-point rating scale, the emoticons and depicted weights (which also had nine alternatives), proportion scores were calculated by dividing the mean ratings per scale category and complexity level by 9. For the VAS, the mean percentage score was divided by 100. In this way all scores had a range between 0 and 1.
Data Analysis
Quantitative Data
Firstly, 2 (complexity; simple vs complex) x 4 (scale type; original, VAS, Emoticons, Weights) repeated measures ANOVAs were conducted for accuracy, time on task, perceived difficulty and perceived invested mental effort within subjects. Secondly, correlations were calculated between performance measures (accuracy and time on task) and the subjective ratings (perceived difficulty and mental effort) for each type of scale. To inspect validity, we tested whether significant correlations between performance measures (accuracy and time on task) and subjective ratings (perceived difficulty and mental effort) were stronger for some scales than others, significant correlations were compared by a calculation tool called cocor (Diedenhofen & Musch, 2015). This tool was also used to compare significant correlations for simple problems or complex problems per scale, to find out whether ratings on a specific type of scale was more representative of cognitive load during simple or complex problem solving. A significance level of 0.05 was used for the main analyses. On follow-up analyses a Bonferroni correction was applied. Partial eta-squared (ηp2) was calculated as a measure of effect size for F-values, with values of 0.01, 0.06, and 0.14, characterizing small, medium, and large effect sizes, respectively (Cohen, 1988). Cohen’s d was calculated as a measure of effect size for t-values, with values of 0.20, 0.50, and 0.80, characterizing small, medium, and large effect sizes, respectively (Cohen, 1988).
Qualitative Data
Finally, participants’ indication of the type of rating scale that they liked and disliked the most was analyzed. As we had no clear expectations of the qualitative data and we wanted to explore the open-ended responses to find reasoning behind preferences for the scales, an inductive approach was taken to code these responses. This approach is appropriate for exploratory purposes in the absence of clear theory-driven hypotheses on how the data would look like (Linneberg & Korsgaard, 2019). Inductive coding is data-driven in that the responses are categorized based on the content of the responses.
Two raters independently rated and categorized the participants’ responses freely (no categories were outlined before). Initially, the first rater decided on four categories (clarity, relatability, appearance, and nuance) but the second rater categorized the data in three categories (clarity, relatability, appearance). After inspection of the ratings, it seemed that the second rater initially did not distinguish between clarity and nuance. After discussion, both raters one agreed on using the four categories specified by the first rater. These answering categories were found by summarizing each given answer into keywords. The keywords were then compared to one another, and analogous keywords were combined into one category. The category “clarity” related to comments on the comprehensibility of the answering options; how unambiguous the answering options were and how easy the scale was found to be. An example of an argument classified as a remark on clarity is: ‘It is most clear as to what each answer means’. The relatability category encompassed comments on how well the participants were able to relate their feelings to the scale that was used. It could also be said that this category scored answers on how intuitive the scale was found to be. A comment marked as relatable, for example, would be: ‘Because it was a better illustration of how I felt’. In addition, especially the dislike comments contained a lot of remarks on how well a scale related to the questions. For example: ‘Incongruent with what the scale is asking’. These kinds of comments were also scored under relatability. The third category, appearance, was a recurring aspect for a lot of the scales. Comments in this category related to the aesthetic qualities of each scale. For example: ‘This scale was most visually pleasing’. Nuance, the final category, could be seen as the opposite of clarity, the first category. However, both were mentioned quite often as either a good or a bad quality of the scale. In addition, for both clarity and nuance a lot of arguments were given on why exactly this was a good quality of the scale. Whereas some participants praised a scale for its clearly defined and unambiguous answering options, other participants appreciated a scale for its grey areas and less well-defined answering options. An example of a nuance-category comment was: ‘Because the rating is not fixed, it can give flexibility to how one perceives the task’. Subsequently, every comment was classified under one of those four categories. Some elaborations were scored under more than one category, so one elaboration could be scored more than once. For example: ‘It is very ugly and not very meaningful’, was scored under appearance as well as relatability.
Supplementary Appendix A shows all responses given for the scales participants indicated to like best and B for those they indicated to like least. In the final columns it is shown how all comments were categorized by the raters and Table 2 shows the final categorization the raters agreed upon. In addition, this table shows how many of those comments related to a scale preference, and how many related to a disliking of the scale.
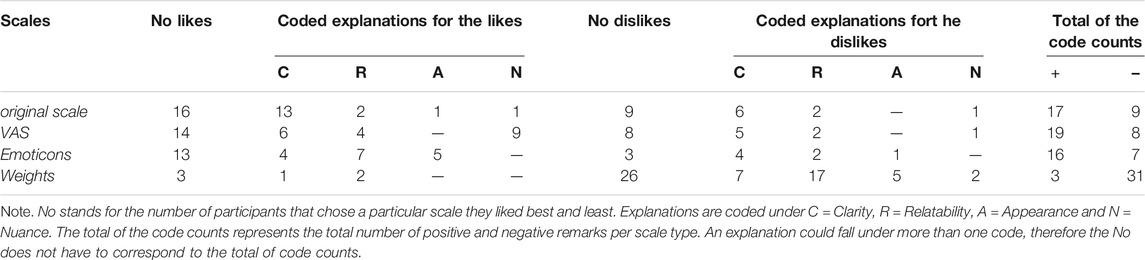
TABLE 2. Overview of Participants’ best and least Preference and Coded Explanations for the Four Types of Rating Scales.
Results
For accuracy, results showed a main effect of complexity, F (45, 1) = 218.75, p < 0.001, ηp2=0 .83, but not for scale, F (45, 1) = 2.2, p = 0.091, ηp2=0 .05, and an interaction effect, F (135, 3) = 5.41, p = 0.002, ηp2=0 .83. For time on task, results showed a main effect of complexity, F (45, 1) = 356.34, p < 0.001, ηp2=0 .89, and scale, F (45, 1) = 13.81, p < 0.001, ηp2=0 .24, and an interaction effect, F (135, 3) = 13.06, p < 0.001, ηp2=0 .23. For perceived difficulty, results showed a main effect of complexity, F (45, 1) = 1,306.63, p < 0.001, ηp2=0 .97, and scale, F (45, 1) = 36.45, p < 0.001, ηp2=0 .45, and an interaction effect, F (135, 3) = 13.64, p < 0.001, ηp2=0 .23. Finally, for perceived mental effort, results showed a main effect of complexity, F (45, 1) = 1,097.30, p < 0.001, ηp2= 0.96, and scale, F (45, 1) = 30.97, p < 0.001, ηp2= 0.41, and an interaction effect, F (135, 3) = 9.50, p < 0.001, ηp2= 0.17. All means and standard deviations of the accuracy, time on task, effort and difficulty ratings are presented in Table 3.

TABLE 3. Proportion mean scores and standard deviations of the four rating scales for accuracy, time on task, and perceived difficulty and invested mental effort.
Following up on the interaction effects between complexity and scale that was found for all dependent variables, we compared difference scores, i.e. instead of using a repeated measure for the performance and ratings on the simple and complex problems, we looked at ∆ simple - complex. By subtracting the complex performance (accuracy and time) and rating (perceived mental effort and difficulty) scores from the simple ones, we obtained one variable for the size of the effect (instead of two) which allows for a direct comparison between effect sizes. Six paired t-tests were done on these difference scores between Original and VAS (pair 1), Original-Emoticons (pair 2), Original-Weights (pair 3), VAS-Emoticons (pair 4), VAS-Weights (pair 5), and Emoticons-Weights (pair 6). Bonferroni correction was applied by adjusting the significance level to 0.05/6 = 0.008.
For readability of the text we put all statistics in Table 4 and report only the significant results in text. It was found that for accuracy the complexity effect was smaller for problems rated with the original scale than those with the weight scale, and smaller for problems rated with the emoticons than the weights scale. For time on task similar results were found with an additional finding that the effect of complexity was smaller for problems rated with the VAS than those with the Weight scale. For both perceived difficulty and perceived mental effort; the effect of complexity was significantly smaller using the original scale compared to the VAS or the original scale compared to the Weights scale. Also the effect of complexity was smaller when using the Emoticons compared to the Weights.
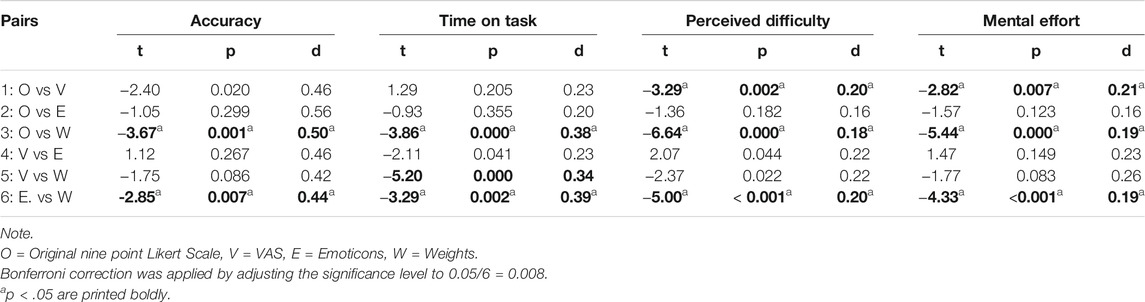
TABLE 4. Statistics of the Paired t-tests Comparing Differences in Accuracy, Time on Task, Perceived Difficulty and Mental Effort Between Complex and Simple Problems Across the Four Types of Rating Scales.
Next, correlations were calculated between performance measures (accuracy and time on task) and the ratings (difficulty and mental effort) for each scale type to examine validity of the four rating scales. For readability purposes, we present all correlations (values and significance levels) for the correlational analyses in Table 5 and report the significant ones in text. First, for all four scale types and problem complexity levels, mental effort and perceived difficulty effort were positively correlated. For analysis on the original 9-point scale, it was found that for the complex problems (but not for the simple problems), accuracy was negatively correlated with perceived mental effort and perceived difficulty and time on task was positively correlated with perceived difficulty and invested mental effort. The analysis on the VAS showed that for complex problems, accuracy was negatively correlated with perceived mental effort and time on task was positively correlated with perceived mental effort and perceived difficulty. For the simple problems, accuracy was negatively correlated with time on task. For the emoticon scale a positive correlation between time on task and perceived difficulty was found that for the complex problems. For the simple problems, time on task was positively correlated with perceived mental effort and perceived difficulty. The depicted weights scale only revealed negative correlations for simple problems between accuracy and mental effort and perceived difficulty.
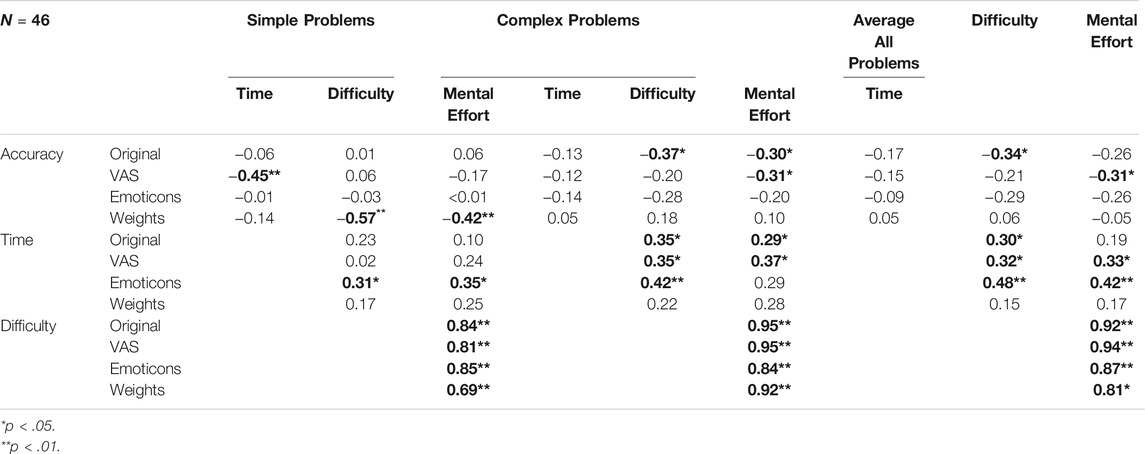
TABLE 5. Correlation table of the performance (accuracy and time on task) and subjective ratings (perceived difficulty and mental effort) of the simple and complex problems.
Because the size of the correlation was already calculated and the values of the correlations were known, the correlational differences were tested one-sided, just as a confirmative test whether the larger (or smaller) correlation was significantly larger (or smaller). For efficiency and clarity reasons, we only report significant results here in text and present all statistics in Table 6 and 7.
Correlational comparisons between scale conditions showed that for the simple problems, Weight scale ratings of perceived difficulty had a significant stronger negative relation to accuracy than any other scale. Weight scale ratings of perceived mental effort during simple problem solving were also more strongly (negatively) related to accuracy compared to the ratings using the Original and Emoticon scales. For the complex problems it was found that ratings using the original scale for both perceived difficulty and perceived mental effort correlated stronger (negatively) with accuracy than using the Weight scale. Ratings on the the Emoticon scale for perceived difficulty on the easy problems showed a stronger (positive) correlation with time on task than the VAS scale.
Noticeable from these results is that the numeric scales (original scale and VAS) seem to better reflect effort and difficulty for the complex problems (as inferred by the correlations with accuracy), while the pictorial scales (emoticons and weights) seem to better reflect effort and difficulty in for the simple problems. To test whether correlations differed depending on complexity level within scales, each significant correlation was compared to its simple or complex counterpart one-sided. For example, for the original scale, accuracy of complex, but not simple problems was significantly correlated to perceived difficulty.
In this manner, 12 correlational pairs were tested (see Table 7). All comparisons not described in text had significance levels of p > 0.16 The significant comparisons showed that for the original scale, the relation between accuracy and perceived difficulty, z = 1.98, p = 0.024, and accuracy and perceived mental effort, z = 1.83, p = 0.034, was stronger for the complex than simple problems. For the VAS, it was found that the relation between time on task and perceived mental effort was stronger for the complex than the simple problems, z = -1.70, p = 0.044. For the Weights, it was found that the relation between accuracy and perceived difficulty, z = −4.21, p < 0.001, and accuracy and perceived mental effort, z = −2.79, p = 0.003 was stronger for the simple than the complex problems.
Scale (dis)liking. Of the 46 participants, 16 participants preferred the original 9-point scale over the others, followed closely by the VAS with 14 votes, the emoticons with 13 votes and the weights with three votes. In response to the reversed question (which scale they disliked the most), 26 participants voted for the weights, nine participants for the original 9-point Likert scale, eight for the VAS and three for the emoticons. Table 2 shows an overview of the best and least preference and coded explanations for the four types of rating scales.
The original 9-point scale was preferred by 16 participants, mainly for the clarity of every answering option. As one participant stated: ‘There is little room for misinterpretation’. Interestingly, this clarity was exactly what nine dislikers criticized about the scale. They found that the scale contained too much text, which also made the answering options confusing. In addition, they stated that nine answering options did not leave enough room for nuance.
The 14 participants who favored the VAS reasoned that it was the most nuanced scale, leaving ‘more opportunity for grey areas’. Eight participants liked the VAS the least, mainly because the scale was too unclear and could leave too much room for misinterpretation. The 13 participants who favored the emoticons scale indicated that this was because the emoticons were relatable to perceived difficulty and effort and, making the scale easiest to interpret and use. Also, the scale was found to be visually the most appealing. It was liked least by three participants who indicated that it was unclear what every option represents and because it was ‘annoying’. Finally, the three participants who liked the weights-scale most indicated that this was because the weights were ‘not as abstract as the other scales’ and the differences between the answering options were most apparent. However, with 26 dislikes, this scale was disliked by the most participants out of all the scales predominantly because the weights were perceived as unrelated to the questions and it was ‘difficult to estimate the value of the weights in relation to the answer to the question’. Also, the differences between the weights were too small. Furthermore, five participants found the scale visually unpleasant and annoying to use.
Discussion
The aim of the present study was to investigate the validity of subjective rating scales measuring perceived difficulty and mental effort. More specifically, our research question was whether certain visual characteristics of a subjective rating scale intended to measure cognitive load, matter for validity (i.e. does one type of visualization elicits more valid responses than others?). By alternating the visual presentation of the scales, we compared four different types of subjective rating scales measuring perceived cognitive load (i.e., mental effort and difficulty) regarding their relation to performance (i.e., accuracy and time on task). Four scales were compared; two well-known ones, the original 9-point rating scale (Paas, 1992) and the VAS, and two specially designed for this study, using either emoticons or pictures of weights. Validity of the mental effort and difficulty ratings was estimated by correlations with performance (i.e., accuracy and time on task) and comparing correlations between difficulty levels and scale types. Also personal preference of the scales was investigated.
The results supported our first hypothesis that all scales would be able to distinguish between complexity levels of the problems for perceived difficulty and mental effort. Complex problems were rated higher than the simple ones regardless of the scale used. The second hypothesis stating that the pictorial scales might reflect cognitive load better, was partially supported. The pattern of the results of the correlations is the most striking; while numeric scales (original scale and VAS) seem to better reflect effort and difficulty for the complex problems, the pictorial scale (emoticons and weights) seems to better reflect effort and difficulty for the simple problems. More specifically, for complex problems, perceived cognitive load and difficulty as measured by the more abstract numeric scales (i.e., the original nine point Likert rating scale and the VAS) were negatively related to performance. In contrast for the simple problems, perceived cognitive load and difficulty as measured by the pictorial scales (i.e., weights and emoticons) were negatively related to performance (i.e., accuracy and time on task). This seems to suggest that for the simple problems, the pictorial scales appeared to represent experienced cognitive load better than for complex problems. Ironically, some students indicated that the differences between the weights were too small to represent the differences in difficulty they experienced. Perhaps if bigger weight increments (larger intervals, instead of 1, 2, 3 etc. 10, 20, 30 etc.) were used, the scale would be better applicable to the difficult problems. The ratings of mental effort and perceived difficulty on the affective scale were positively related with the time on task needed for the simple problems in that higher ratings were related to more time on task needed. For the complex problems, the original 9-point Likert scale was related to both the accuracy and time on task in that higher ratings were related to lower accuracy and more time on task. The VAS showed the same results as the original 9-point Likert scale, except that perceived difficulty was not (significantly) related to solution accuracy.
Therefore, it appears that the 9-point rating scale is more sensitive than the VAS scale in detecting the correlation between perceived difficulty and solution accuracy as the level of complexity in problem-solving task increases. A rating scale that is more sensitive in detecting perceived difficulty holds potential for enhancing cognitive analytics and the development of self-adaptive systems that links interaction between human and computer systems (Radanliev, et al., 2020). When comparisons between correlations was done, it was found that for the easy problems, the Weight scale provided a better reflection of perceived difficulty. However for the complex problems, the original 9-point rating scale did best.
On scale preference, it was found that the original scale was preferred most (with the VAS and emoticons closely following) and the least liked were the weights. However, likability was not a predictor for validity in terms of the association with the ratings on the scales and performance. A point for discussion might be that students were asked about their preference for a scale after a block of four problems in the order of simple-simple -complex -complex. Having made the complex problems just before being asked about scale preference might have induced a recency effect, in that the responses recorded reflect the experience of rating mental effort and difficulty on the complex problems more than that of the simple problems. In a future studie it would be interesting to ask for participants’ preference directly after they completed the simple problems and again after the complex problems.
One limitation of the present study is that the picture of the weights in the embodied scale did not fully fit onto the screen for three of the participants. Therefore, the participants had to scroll sideways to view the full length of the scale. These technical issues might have confounded the disliking of the weight scale compared to the other scales. A more theoretical limitation is that although the results showed a difference in effort and difficulty ratings for the pictorial versus numerical scales for simple versus complex problems, another factor besides the embodied cognition account may play a role in explaining these differences. In a study by Schmeck et al. (2015) that used similar week day problems, timing and topic of the ratings seemed to matter for the outcomes. Delaying effort and difficulty rating after a series or problems seemed to elicit higher scores than the average of ratings given immediately after each separate problem. The delayed ratings seemed to be better predictors of the performance on the complex problems. However, for affective components such as interest and motivation, this difference was not found. We suggest that it would be interesting to replicate the study of Schmeck et al. (2015) with the four types of scales used in this study for two reasons. First, it would be interesting to find out how the response to the affective items would differ between numeric and pictorial scales. It might be the case that the numeric scales are less sensitive to affective questions than pictorial scales and that this is the reason that these ratings were not sensitive to the timing or complexity of the problems. Second, we might find a differentiating effect depending on the timing and complexity of the problems for the affective items, using the pictorial scales.
Conclusion
In summary, it seems that the pictorial scales (i.e., emoticons and weights) seem to provide a more valid indication of mental effort and difficulty for simple tasks and the original 9-point Likert scale and the VAS more for complex tasks. This can be explained from the perspective of recent critics on the embodied cognition theory in that for lower-level abilities and functions, cognition may be well-grounded in sensory-motor processes, but that this may not (or to a lesser extent) be the case for higher order cognitive processes (i.e., Caramazza et al., 2014). On the other hand, it seems that numerical scales might be less suitable to reflect perceived mental effort and difficulty on simple problems. Practical implications from this finding would be to use more pictorial rating scales when assessing mental effort and perceived difficulty for simple tasks and more abstract numeric scales for more complex tasks. However, we strongly recommend future studies to replicate this setup, purely to see whether the similar results are found and to find out whether the results are reliable for other populations (i.e., other age group) and for other tasks. Note that we used university students for the present study. From the educational level and age of this rather homogeneous sample, we can expect that the learning capacity and working memory functioning are optimal compared to other populations such as older adults. For the present study, we manipulated task difficulty by increasing element interactivity and thereby intrinsic load (cognitive load elicited by task characteristics). However, populations with suboptimal cognitive functioning, such as older adults, might have more difficulty in general with the task because of an age-related decrease in working memory functioning and cognitive aging in general (e.g., Braver, & West, 2011), resulting in decreased germane cognitive load. In addition, in children, a population in which cognitive functioning and working memory are still developing, it has already been shown that use of pictures work well for clinical ratings such as pain (e.g. Keck, et al., 1996), nausea (Baxter, et al., 2011). In addition, promising results with children are also found for occupational self-assessment (e.g. Kramer, et al., 2010). Therefore, it would be interesting to replicate this study with a sample from a different age population.
In conclusion, in the present study, we made a start in the exploration of different types of subjective rating scales to self-assess invested mental effort and task difficulty. The main finding was that numerical type scales seem to better reflect cognitive processes for complex problem solving while the pictorial type scales for simple problem solving. Whether this finding applies to other forms of simple versus complex tasks, needs to be explored in the future.
Data Availability Statement
The raw data supporting the conclusions of this article will be made available by the authors, without undue reservation.
Ethics Statement
The studies involving human participants were reviewed and approved by the Ethics review Committee DPECS, Erasmus University Rotterdam, Netherlands. The patients/participants provided their written informed consent to participate in this study.
Author Contributions
Authors contributed in the order presented: KO coordinated the research and had the lead in writing the manuscript. JW helped along the whole process and contributed ideas and a critical review on the drafts of KO. AK gathered the data, partly analysed the data and also reviewed the manuscript drafts. FP supervised on the background initially and critically reviewed our ideas and manuscript.
Funding
The fee is funded by a special fund within the Erasmus University Rotterdam, Netherlands: the Erasmus Open Access Fund.
Conflict of Interest
The authors declare that the research was conducted in the absence of any commercial or financial relationships that could be construed as a potential conflict of interest.
Publisher’s Note
All claims expressed in this article are solely those of the authors and do not necessarily represent those of their affiliated organizations, or those of the publisher, the editors and the reviewers. Any product that may be evaluated in this article, or claim that may be made by its manufacturer, is not guaranteed or endorsed by the publisher.
Supplementary Material
The Supplementary Material for this article can be found online at: https://www.frontiersin.org/articles/10.3389/feduc.2021.702616/full#supplementary-material
References
Alghadir, A. H., Anwer, S., Iqbal, A., and Iqbal, Z. A. (2018). Test-retest Reliability, Validity, and Minimum Detectable Change of Visual Analog, Numerical Rating, and Verbal Rating Scales for Measurement of Osteoarthritic Knee Pain. J. Pain Res. 11, 851–856. doi:10.2147/JPR.S158847
Ayres, P. (2018). “Subjective Measures of Cognitive Load: What Can They Reliably Measure?” in Cognitive Load Measurement and Application: A Theoretical Framework for Meaningful Research and Practice. Editor R. Z. Zheng (Routledge/Taylor & Francis Group), 9–28.
Ayres, P. (2006). Using Subjective Measures to Detect Variations of Intrinsic Cognitive Load within Problems. Learn. Instruction 16 (5), 389–400. doi:10.1016/j.learninstruc.2006.09.001
Baddeley, A. (2000). The Episodic Buffer: a New Component of Working Memory? Trends Cogn. Sci. 4 (11), 417–423. doi:10.1016/S1364-6613(00)01538-2
Barsalou, L. W. (2008). Grounded Cognition. Annu. Rev. Psychol. 59, 617–645. doi:10.1146/annurev.psych.59.103006.093639
Barsalou, L. W. (2016). On Staying Grounded and Avoiding Quixotic Dead Ends. Psychon. Bull. Rev. 23 (4), 1122–1142. doi:10.3758/s13423-016-1028-3
Baxter, A. L., Watcha, M. F., Baxter, W. V., Leong, T., and Wyatt, M. M. (2011). Development and Validation of a Pictorial Nausea Rating Scale for Children. Pediatrics 127 (6), e1542–9. doi:10.1542/peds.2011-231210.1542/peds.2010-1410
Braver, T. S., and West, R. (2011). “Working Memory, Executive Control, and Aging,” in The Handbook of Aging and Cognition. Editors F. I. M. Craik, and T. A. Salthouse (van der, New York: Psychology Press), 311–372.
Caramazza, A., Anzellotti, S., Strnad, L., and Lingnau, A. (2014). Embodied Cognition and Mirror Neurons: a Critical Assessment. Annu. Rev. Neurosci. 37, 1–15. doi:10.1146/annurev-neuro-071013-013950
DeLeeuw, K. E., and Mayer, R. E. (2008). A Comparison of Three Measures of Cognitive Load: Evidence for Separable Measures of Intrinsic, Extraneous, and Germane Load. J. Educ. Psychol. 100 (1), 223–234. doi:10.1037/0022-0663.100.1.223
Diedenhofen, B., and Musch, J. (2015). Cocor: A Comprehensive Solution for the Statistical Comparison of Correlations. PLoS ONE 10 (4), e0121945. doi:10.1371/journal.pone.0121945
Faul, F., Erdfelder, E., Buchner, A., and Lang, A. G. (2009). Statistical power analyses using G*Power 3.1: Tests for correlation and regression analyses. Behavior Research Methods 41, 1149–1160. doi:10.3758/BRM.41.4.1149
Gordan, M., and Amutan, K. I. (2014). A Review of B. F. Skinnerâ€s ‘Reinforcement Theory of Motivationâ€. Ijrem 5 (3), 680–688. doi:10.24297/ijrem.v5i3.3892
Hadie, S. N. H., and Yusoff, M. S. B. (2016). Assessing the Validity of the Cognitive Load Scale in a Problem-Based Learning Setting. J. Taibah Univ. Med. Sci. 11 (3), 194–202. doi:10.1016/j.jtumed.2016.04.001
Keck, J. F., Gerkensmeyer, J. E., Joyce, B. A., and Schade, J. G. (1996). Reliability and Validity of the Faces and Word Descriptor Scales to Measure Procedural Pain. J. Pediatr. Nurs. 11 (6), 368–374. doi:10.1016/S0882-5963(96)80081-9
Kramer, J. M., Kielhofner, G., and Smith, E. V. (2010). Validity Evidence for the Child Occupational Self Assessment. Am. J. Occup. Ther. 64 (4), 621–632. doi:10.5014/ajot.2010.08142
Naismith, L. M., and Cavalcanti, R. B. (2015). Validity of Cognitive Load Measures in Simulation-Based Training: a Systematic Review. Acad. Med. 90 (11), S24–S35. doi:10.1097/ACM.0000000000000893
O'Doherty, J. P., Cockburn, J., and Pauli, W. M. (2017). Learning, Reward, and Decision Making. Annu. Rev. Psychol. 68, 73–100. doi:10.1146/annurev-psych-010416-044216
Ouwehand, K., van Gog, T., and Paas, F. (2014). Effects of Gestures on Older Adults' Learning from Video-Based Models. Appl. Cognit. Psychol. 29, 115–128. doi:10.1002/acp.3097
Paas, F. G. W. C. (1992). Training Strategies for Attaining Transfer of Problem-Solving Skill in Statistics: A Cognitive-Load Approach. J. Educ. Psychol. 84, 429–434. doi:10.1037/0022-0663.84.4.429
Paas, F., Tuovinen, J. E., Tabbers, H., and van Gerven, P. W. M. (2003). Cognitive Load Measurement as a Means to advance Cognitive Load Theory. Educ. Psychol. 38 (1), 63–71. doi:10.1207/S15326985EP3801_8
Radanliev, P., De Roure, D., Van Kleek, M., Santos, O., and Ani, U. (2020). Artificial Intelligence in Cyber Physical Systems. AI Soc., 1–14. doi:10.1007/s00146-020-01049-0
Rhodes, M. G. (2015). Judgments of Learning: Methods, Data, and Theory. Oxford University Press. doi:10.1093/oxfordhb/9780199336746.013.4
Schmeck, A., Opfermann, M., van Gog, T., Paas, F., and Leutner, D. (2015). Measuring Cognitive Load with Subjective Rating Scales during Problem Solving: Differences between Immediate and Delayed Ratings. Instr. Sci. 43 (1), 93–114. doi:10.1007/s11251-014-9328-3
Shenhav, A., Musslick, S., Lieder, F., Kool, W., Griffiths, T. L., Cohen, J. D., et al. (2017). Toward a Rational and Mechanistic Account of Mental Effort. Annu. Rev. Neurosci. 40, 99–124. doi:10.1146/annurev-neuro-072116-031526
Sitzmann, T., Ely, K., Brown, K. G., and Bauer, K. N. (2010). Self-assessment of Knowledge: A Cognitive Learning or Affective Measure? Amle 9 (2), 169–191. doi:10.5465/amle.9.2.zqr169
Skjott Linneberg, M., and Korsgaard, S. (2019). Coding Qualitative Data: A Synthesis Guiding the Novice. Qrj 19 (3), 259–270. doi:10.1108/QRJ-12-2018-0012
Staddon, J. E., and Cerutti, D. T. (2003). Operant Conditioning. Annu. Rev. Psychol. 54 (1), 115–144. doi:10.1146/annurev.psych.54.101601.145124
Sung, Y. T., and Wu, J. S. (2018). The Visual Analogue Scale for Rating, Ranking and Paired-Comparison (VAS-RRP): a New Technique for Psychological Measurement. Behav. Res. Methods 50 (4), 1694–1715. doi:10.3758/s13428-018-1041-8
Sweller, J., Ayres, P., and Kalyuga, S. (2011). “Measuring Cognitive Load,” in Cognitive Load Theory (New York, NY: Springer), 71–85. doi:10.1007/978-1-4419-8126-4_6
Sweller, J. (2010). Element Interactivity and Intrinsic, Extraneous, and Germane Cognitive Load. Educ. Psychol. Rev. 22, 123–138. doi:10.1007/s10648-010-9128-5
Sweller, J., and Paas, F. (2017). Should Self-Regulated Learning Be Integrated with Cognitive Load Theory? A Commentary. Learn. Instruction 51, 85–89. doi:10.1016/j.learninstruc.2017.05.005
Sweller, J. (1993). Some Cognitive Processes and Their Consequences for the Organisation and Presentation of Information. Aust. J. Psychol. 45, 1–8. doi:10.1080/00049539308259112
Sweller, J., van Merriënboer, J. J. G., and Paas, F. (2019). Cognitive Architecture and Instructional Design: 20 Years Later. Educ. Psychol. Rev. 31 (2), 261–292. doi:10.1007/s10648-019-09465-5
Sweller, J., van Merriënboer, J. J. G., and Paas, F. G. W. C. (1998). Cognitive Architecture and Instructional Design. Educ. Psychol. Rev. 10, 251–296. doi:10.1023/A:1022193728205
van Gog, T., Kirschner, F., Kester, L., and Paas, F. (2012). Timing and Frequency of Mental Effort Measurement: Evidence in Favour of Repeated Measures. Appl. Cognit. Psychol. 26, 833–839. doi:10.1002/acp.2883
van Merriënboer, J. J. G., and Sweller, J. (2005). Cognitive Load Theory and Complex Learning: Recent Developments and Future Directions. Educ. Psychol. Rev. 17, 147–177. doi:10.1007/s10648-005-3951-0
Keywords: cognitive load, measurement methodology, subjective rating scales, visualization, problem solving
Citation: Ouwehand K, Kroef Avd, Wong J and Paas F (2021) Measuring Cognitive Load: Are There More Valid Alternatives to Likert Rating Scales?. Front. Educ. 6:702616. doi: 10.3389/feduc.2021.702616
Received: 29 April 2021; Accepted: 02 September 2021;
Published: 20 September 2021.
Edited by:
Lu Wang, University of Georgia, United StatesReviewed by:
Petar Radanliev, University of Oxford, United KingdomSavio W. H. Wong, The Chinese University of Hong Kong, China
Copyright © 2021 Ouwehand, Kroef, Wong and Paas. This is an open-access article distributed under the terms of the Creative Commons Attribution License (CC BY). The use, distribution or reproduction in other forums is permitted, provided the original author(s) and the copyright owner(s) are credited and that the original publication in this journal is cited, in accordance with accepted academic practice. No use, distribution or reproduction is permitted which does not comply with these terms.
*Correspondence: Kim Ouwehand, b3V3ZWhhbmRAZXNzYi5ldXIubmw=