- 1Media-Based Knowledge Construction—Research Methods in Psychology, Computer Science and Applied Cognitive Science, Faculty of Engineering, University of Duisburg-Essen, Duisburg, Germany
- 2Educational Technology, Institute of Education, Faculty of Arts and Social Sciences, University of Zurich, Zurich, Switzerland
Cognitive load theory assumes effort may only lead to comprehension if the material-induced load leaves enough resources for learning processes. Therefore, multimedia materials should induce as little non-relevant load as possible. Metacognition research assumes that learners tap into their memory processes to generate a mental representation of their comprehension to regulate learning. However, when judging their comprehension, learners need to make inferences about actual understanding using cues such as their experienced mental load and effort during learning. Theoretical assumptions would assume both to affect understanding and its metacognitive representation (metacomprehension). However, the question remains how perceived effort and load are related to metacomprehension judgments while learning with multimedia learning material. Additionally, it remains unclear if this varies under different conditions of multimedia design. To better understand the relationship between perceived mental load and effort and comprehension and metacomprehension under different design conditions of multimedia material, we conducted a randomised between-subjects study (N = 156) varying the design of the learning material (text-picture integrated, split attention, active integration). Mediation analyses testing for both direct and indirect effects of mental load and effort on metacomprehension judgments showed various effects. Beyond indirect effects via comprehension, both mental load and effort were directly related to metacomprehension, however, this seems to vary under different conditions of multimedia design, at least for mental effort. As the direction of effect can only be theoretically assumed, but was not empirically tested, follow-up research needs to identify ways to manipulate effort and load perceptions without tinkering with metacognitive processes directly. Despite the limitations due to the correlative design, this research has implications for our understanding of cognitive and metacognitive processes during learning with multimedia.
1 Introduction
Research on multimedia learning aims at examining the influence of differences in the design of learning materials on learning outcomes (for an overview, see Li et al., 2019). For example, the inclusion of signaling cues (i.e., the highlighting of learning-relevant information or the structure of a learning material; Schneider et al., 2018) or the segmentation of learning materials into meaning-related sections (Rey et al., 2019) were found to foster learning by highlighting relevant and coherent concepts of a learning material. However, not all materials are well designed or activate learners to build a coherent mental model leading to huge learning differences. One major explanation for learning differences is based on the cognitive load theory (Sweller, 1994; Sweller et al., 2011). According to this theory, learners experience a cognitive load when processing the information presented in a multimedia learning material. Cognitive load, also often referred to as mental load is, thus, said to be task-related and reflects the cognitive resources needed to cope with the complexity of the learning material.
Cognitive load can be distinguished into two groups of processes: learning-relevant (productive) and learning-irrelevant (unproductive) cognitive load processes (Kalyuga and Singh, 2016). While productive cognitive load refers to all cognitive processes that are needed to reach a learning goal, unproductive cognitive load refers to all cognitive processes that occur due to design-induced information search processes. For example, decorative (learning-irrelevant) pictures integrated into multimedia learning material may distract learners’ attention away from learning-relevant information (for an overview, see Schneider et al., 2016). When both textual and pictorial information are learning relevant and relate to each other, their spatial distance is of major importance for the amount of cognitive load since spatially distant representation lead to a learning-hindering split-attention effect (for an overview, see Schroeder and Cenkci, 2018). In this case, the integration of textual information into the pictorial information source is found to enhance complex learning (i.e., the spatial contiguity principle; Mayer and Moreno, 2003; for an overview, see Schroeder and Cenkci, 2018) by a reduction of unproductive cognitive load (Schroeder and Cenkci, 2020). Another possibility to foster learning is not to reduce learning-irrelevant processes, but to induce learning-relevant processes. Similar to a generation effect (see Bertsch et al., 2007), asking learners to actively integrate pictorial and symbolic sources of information in multimedia learning material seems to support them in building coherent mental representations and fosters learning (Bodemer et al., 2004; Bodemer et al., 2005). Thus, within such an active-integration procedure, learners are asked to integrate disintegrated material assuming the load induced by the split attention is gradually reduced by actively integrating the material. During this process it gets gradually replaced by the additional productive cognitive load that supports building coherent mental models. Thus, while the load imposed is supposed to be quite high, such “desirable difficulties” (see Bjork and Bjork, 2011) should ultimately be beneficial for learning as has been found for other instructions designed to induce germane cognitive processes, for example self-explanation prompts (e.g., Berthold and Renkl, 2009; Renkl et al., 2009).
While the terms “mental load” and “cognitive load” are sometimes used interchangeably, other conceptualizations differentiate the concept. In these, cognitive load is not seen as a unidimensional construct based on task-induced affordances, but also includes the effort learners assign to task-processing (Paas and van Merriënboer, 1994). As described with the above examples, cognitive load is imposed by the demands from the learning environment to perform a certain learning task. However, not all learners will achieve the same learning under the same task conditions. Learners allocate a different amount of cognitive resources for a given task demand (i.e., their cognitive engagement in the task). This allocation of cognitive resources is known as mental effort (Orru and Longo, 2019). Mental effort reflects a second dimension of possible assessment factors besides the measurement of (task-driven) mental load and the actual learning performance and can be described as a second indicator for possible learning differences by addressing the human-centered dimension of cognitive load (Scheiter et al., 2020). Thus, mental effort refers to learners’ actually invested cognitive resources while processing information of a learning material (Paas and van Merriënboer, 1994).
The relationship between mental load and mental effort and their relation to learning processes has been examined to a minor degree. Some studies propose that mental effort and mental load are different concepts with unique consequences for the measurement of learning processes (e.g., Ayres and Youssef, 2008; Schmeck et al., 2015). The experienced mental load and invested mental effort are most often measured with subjective rating scales (e.g., Naismith et al., 2015; Anmarkrud et al., 2019). When using such a subjective measurement, researchers assume that learners are able to access and assess their own invested cognitive resources and load imposed by a learning task (Paas et al., 2008).
If insights into mental processes and resources like mental load and mental effort are available to learners, we must assume that these learners are also able to use this information for metacognitive regulation purposes. While there clearly is more to (successful) regulation than metacognitive monitoring (e.g., using monitoring outcomes to control learning; Schnaubert and Bodemer, 2017), from a learner’s perspective, internal experiences may be used to guide regulation attempts (independent of their successfulness). Metacognitive processes are deemed vital for understanding how learners approach and process learning material (Schraw et al., 2006). While this may apply for memory processes like word-pair or vocabulary learning, this also extends to comprehending complex expository material (Wiley et al., 2005). Not only do learners need to plan what to study, but also divert attention towards relevant sources and invest effort in processing and integrating the content, for example when integrating texts and graphics during multimedia learning (e.g., Burkett and Azevedo, 2012). To do so, according to metacognition theories, learners monitor their learning-related cognitive processes and outcomes (e.g., their comprehension) and—by comparing it to standards—evaluate the need for further studying or a change of tactics or strategies (e.g., Nelson and Narens, 1990; Winne and Hadwin, 1998) and may thus actively steer their learning processes to successfully foster learning (e.g., Thiede, 1999; Metcalfe, 2009).
Thus, monitoring learning and comprehension is a crucial part of learning. To support learners in doing so, it is critical to understand what affects their metacognitive monitoring judgments, which is the evaluation of own learning, memory and comprehension. It is widely assumed that learners have no direct access to their memory or comprehension strength, but that metacognitive judgments rely on cues and are inferential in nature. Cue utilization theory (Koriat, 1997) assumes that learners use a number of relevant cues to judge their learning and comprehension. A crucial part of these are mnemonic cues, that is cues relating to perceiving memory processes such as the ease with which information is processed, encoded or retrieved from memory (e.g., Begg et al., 1989; Benjamin et al., 1998). Such experience-based cues inform metacognitive judgments and are in turn influenced by intrinsic and extrinsic factors, for example, material complexity or prior exposure (Koriat, 1997; Koriat et al., 2008). However, as not all cues are equally diagnostic to learning in all circumstances, their usage may result in low monitoring accuracy (i.e., the relationship between actual comprehension and metacomprehension), which is often found to be quite poor for metacomprehension (Dunlosky et al., 2005; Dunlosky and Lipko, 2007). While there are various aspects of memory and cognition learners may monitor, our research is focused on the monitoring of own levels of comprehension. In this context, metacomprehension is defined as the metacognitive representation of own comprehension as a result of metacognitive monitoring (Maki and Berry, 1984; Wiley et al., 2005) rather than a valid understanding of own comprehension (metacomprehension accuracy). Thus, while a lot of multimedia-focused research focusses rather on the accuracy of metacognitive judgments, this is a rather instructional design perspective (as it relates it to learning outcomes from an outside perspective). From a metacognition perspective, it is of equal importance to understand what affects a learner’s judgment itself as this forms the basis for self-regulatory processes (e.g., Koriat, 1997). The subjective experience and evaluation of own learning (not their accuracy) is key in regulating behavior and form the basis of control decisions (Nelson and Narens, 1990; Son and Schwartz, 2002), for example regarding study time allocation (e.g., Son and Metcalfe, 2000). While both the subjective monitoring judgments and their accuracy ultimately contribute to learning and are worthwhile studying, the learner-centred view aims at understanding the learners’ experience. This is the perspective taken within this study. From a metacognitive self-regulation perspective, it is of vital importance to understand how learners judge their comprehension and form metacomprehension judgments and how this relates to cognitive processing. From a multimedia-learning perspective, it is important to understand how this is affected by the design of (multimedia) learning material.
De Bruin and van Merriënboer (2017) made a first explicit attempt to connect self-regulated learning and cognitive load theory. While they focused on differences and similarities between the theories, concepts and measurement rather than the actual relationship between the specific cognitive and metacognitive constructs in question, within their special issue, there are some studies relating cognitive load and metacognitive judgments empirically. For example, Schleinschok and colleagues (2017) found a strong negative correlation between prospective judgments-of-learning (JOLs) and cognitive load. They further performed regression analyses finding cognitive load not contributing to explaining test performance when JOLs were included in the analyses. Baars and colleagues (2017), on the other hand, assume that under various task conditions varying in cognitive load, learners may use their invested mental effort as cue for forming metacognitive judgments. While this hypothesis needs empirical validation, the authors previously found strong correlations between mental effort and metacognitive judgments, although the impact of actual learning was not included in these calculations (Baars et al., 2013). A current meta-analysis found a substantial negative overall relationship between perceived mental effort and monitoring judgments (Baars et al., 2020). This negative relationship, however, defused for self-agent ratings (i.e., ratings stressing the effort put willingly into learning rather than the effort necessary to solve a task; Koriat, 2018). Thus, it can be assumed that mental effort only negatively relates to monitoring judgments, when it reflects a need rather than a choice to invest effort. Our study relates to the latter conceptualisation, as it distinguishes task-induced load and effort invested willingly.
While cue utilization has been researched extensively within metacognition research, multimedia research provides insights into cognitive processes relevant for processing complex learning material. Within cognitive load research, it is commonly assumed that learners are able to access information about and thus validly judge their mental load and effort (Paas et al., 2008). However, judging own mental effort may also be viewed from a metacognitive perspective as it entails monitoring own cognitive processes (Scheiter et al., 2020). Thus, it seems logical that learners may use these insights into their cognitive processes as cues to form metacognitive judgments about their learning; perceived mental load and effort may therefore affect metacognitive judgments (Baars et al., 2017). Consequently, in our study, we want to investigate if learners’ mental effort and mental load are related to metacomprehension judgments beyond their effect on the learner’s actual level of comprehension.
De Bruin and colleagues related cues, monitoring judgments, and learning by cue utilization, diagnosticity of the cues for learning, and monitoring accuracy (de Bruin et al., 2017). Their (metacognitive) model resembles a mediation model predicting learning or performance based on monitoring judgments. This is in line with other studies, for example, Schleinschok and colleagues (2017), who regressed JOLs and cognitive load on test performance. While we understand the reasons for predicting performance based on prospective JOLs, when directly targeting metacomprehension, i.e., learners’ mental representation of their comprehension, learners judge their current state of learning not their later task performance. Such presumably subtle difference in assessment may have severe impact on the outcome of a metacognitive judgment (Kelemen, 2000). Thus, in our model, metacomprehension is following comprehension not preceding it (although it may precede its assessment if it can be assumed that comprehension is relatively stable and not affected by the process of judging one’s comprehension on a metacomprehension scale; see Section 2.3). We additionally argue that while the value of mental load and mental effort for predicting learning and resulting comprehension may diminish when metacognitive judgments are involved, that does not mean that they are not affecting the judgments themselves and the strong intercorrelation found in Schleinschok and colleagues’ study (2017) indicates there might be more to this relationship. To sum it up, while we are aware of the different approaches to these relationships, we assume mental processes are not only predictive for actual comprehension, but learners’ awareness of these processes may be used as mnemonic cues for metacognitive judgments as well. Thus, we assume that learning and comprehension precedes monitoring said comprehension and consequently, in our model, comprehension is the mediator while metacomprehension is the criterion with (perceived) mental load and mental effort as predictors (Figure 1).
Based on multimedia research, we assume high diagnosticity for perceived mental effort (positive) and perceived mental load (negative) for predicting comprehension (a paths). Additionally, based on metacognition research, we assume a high positive relationship between comprehension and metacomprehension judgments (b path). While this relationship is termed “monitoring accuracy” and can theoretically be modelled as such, please note that within the statistical model used in our empirical study (see Section 2), a between-subject relationship between comprehension and metacomprehension judgments does not reflect monitoring accuracy as it does not reflect how individual learners monitor their cognitions and differentiate between high or low comprehension (see Schraw, 2009 and Schraw et al., 2013 for measures of monitoring accuracy). It remains unclear, whether perceived mental load and mental effort are predictive for metacomprehension judgments (c paths) and especially whether they thus affect metacomprehension judgments beyond their actual effects on comprehension (c’ paths). Based on de Bruin’s model (de Bruin et al., 2017), these paths are termed “cue utilization” describing a theoretical relationship between those concepts. Considering the above discussed literature (e.g., Koriat, 2018; Baars et al., 2020) as well as the above assumed relationships (a and b paths), we assume (perceived) mental effort to be rather positively and (perceived) mental load to be negatively related to metacomprehension judgments. The positive relationship of mental effort hereby refers to a conceptualization of effort that includes elements of self-agency and thus a choice to invest effort rather than a need to invest.
We know from multimedia and cognitive load theory and research that while load and effort contribute to comprehension, their value for judging comprehension depends strongly on the target processes involved and if they ultimately are relevant or not relevant for learning and comprehension. As described above, within multimedia learning, this may strongly depend on the design of the learning material. For example, learning-irrelevant load may be induced by a split attention format whereby an active integration format may induce load positively related to learning. This begs the question if learners—who may use mnemonic cues like the perceived mental load put upon them by the learning material and the effort applied to process the content to judge their comprehension (Baars et al., 2017)—are aware whether the processes conducted are directly related to learning or not. Thus, we further want to know if these mechanisms apply to different design versions of multimedia material similarly or if designs that evoke unproductive load (like a split attention format) or additional productive learning processes (like active integration) or reduce load (like an integrated material) have differential effects. For example, the ease with which integrated material may be processed may lead to learners judging their comprehension to be quite high while processing that requires more effort may be judged as less understood (ease-of-processing hypothesis; Undorf and Erdfelder, 2011). While this may be reasonable when the load imposed is based on unfortunate design of the multimedia material (e.g., split attention format), this may not hold true while actively integrating material, which may induce a high load, but may ultimately be rewarding (comparable to desirable difficulties, Bjork and Bjork, 2011). Thus, the actual diagnosticity of (perceived) mental load and effort for comprehension may vary under various load-inducing conditions and it remains unclear, if cue utilization differs accordingly. Thus, we will use a variety of different design mechanisms to find out if the relationship between mental load or effort and metacomprehension differs for these conditions and if—by affecting diagnosticity—they may hamper the relationship between comprehension and metacomprehension judgments. Thus, although effects on mental load and mental effort caused by the multimedia design are assumed, the main target of the paper is not to assess the effect of multimedia design on mental load or effort as this has been done extensively in the past (e.g., Xie et al., 2017; Mutle-Bayraktar et al., 2019), but to investigate if the multimedia design and its potential effects on the relationship between load, effort and learning effectiveness (i.e., comprehension) affect metacomprehension judgments and their relationship with mental load or effort as well. Consequently, the study aims to investigate if a potential direct and indirect relationship between perceived load and effort and metacomprehension judgments differs between conditions of multimedia design.
Taken together, we assume that overall, (perceived) mental effort is positively related to metacomprehension judgments (hypothesis 1) while (perceived) mental load is negatively related to metacomprehension judgments (hypothesis 2). With regard to the precise relationship and also the effect of multimedia design, our research questions are: 1) Is learners’ perceived mental effort and load related to learners’ judgments of comprehension (beyond actual effects on comprehension), and 2) does this vary under different load-imposing multimedia conditions (i.e., split attention, integrated, active integration condition)? While in line with Baars and colleagues (2017) we assume learners to use mental effort and load as cues for their metacognitive judgments, please be advised that intentional cue usage is not in the focus of our research, but the relationship between the constructs.
While the research basis with regard to the effect of the multimedia design on metacomprehension judgments (vs. metacomprehension accuracy) is too scarce to form explicit hypotheses and we thus chose a more exploratory approach to that respect, some effects seem more likely than others. For example, based on research on generative activities and its relation to monitoring accuracy (e.g., Prinz et al., 2020; van Gog et al., 2020), it may be assumed that effort and load within active integration affect metacomprehension judgments rather indirectly via comprehension rather than directly (without relating to comprehension). With regard to split attention, one may assume that a possible effort-metacomprehension-relationship may not be mediated by comprehension as the effort-comprehension relationship may be hampered by investing effort in overcoming the split-attention rather than germane learning activities (e.g., Beege and Colleagues, 2019). However, as the research basis for these assumptions is not yet solid enough to form distinct hypotheses (apart from the direction of the general relationship between perceived mental load or effort and metacomprehension judgments), we chose a more exploratory approach to take a first step towards understanding how (perceived) mental load and mental effort are related to metacognitive monitoring of learning under varying conditions of multimedia design.
While our study focusses on assumptions about the relationship between mental effort, mental load, comprehension, and metacomprehension under varying conditions of multimedia design, we further assume to replicate effects frequently found in multimedia research. Based on the literature on multimedia learning, we assume the multimedia design to affect comprehension with a split attention format rather hampering learning (unnecessary effort needs to be invested into integrating text and graphics; e.g., Schroeder and Cenkci, 2018) and active integration rather fostering learning (generative activity; e.g., Bodemer et al., 2004). Further, we assume multimedia design to affect especially mental load, with the potentially load inducing conditions (split attention and active integration) resulting in higher perceived mental load (e.g., Schroeder and Cenkci, 2020). However, as these issues are not in the focus of the study, we did not include them in the formal hypotheses.
2 Materials and Methods
The study (study-ID: psychmeth_2019_MMMC_66) was conducted November 2019 to December 2019 at the University of Duisburg-Essen and approved by the local ethics committee (ethics votum-ID: 1910PFYE114). It was not officially pre-registered.
2.1 Sample
The sample consisted of 156 university students, most of them (145) studying applied cognitive and media science or loosely related subjects (seven psychology, one applied informatics, one engineering, one applied language science, one did not provide a course of study). They had a mean age of 21.00 years (SD = 3.35). Most of them (121) identified as female, 34 as male, and one as non-binary. Altogether, the sample can be described as quite homogeneous: predominantly females in their early 20 s studying applied cognitive and media science at a German university. The participants were randomly assigned to one of three conditions with a different multimedia design (see Section 2.2): integrated format (n = 51), split attention format (n = 52) and active integration format (n = 53). Due to an assignment error, not all pre-test data to describe sample characteristics could be confidently traced back, but a rigorously cleaned minimal dataset with 137 participants showed a medium interest in the topic of information transmission within the nervous system (M = 4.09, SD = 1.59 on a scale from 1 = “very low” to 7 = “very high”) and rather low self-assessed prior knowledge on the nervous system (M = 3.09, SD = 1.37 on a scale from 1 = “very poor” to 7 = “very well”) and ability to explain the difference between a excited und inhibited synapse (M = 2.47, SD = 1.52 on a scale from 1 = “very poor” to 7 = “very well”).
2.2 Design
Within this study, we assessed all variables (predictors, mediator, criterion) throughout the sample. Additionally, we randomly assigned each participant to one of three multimedia design conditions. The experimentally varied factor (design of the multimedia learning material) thus had three factor levels varying the design of the learning material to induce various types of cognitive load. On level one (integrated format), the material, consisting of text and picture, was presented in an integrated format with textual annotations attached to the pictorial content to decrease cognitive load. On level two, the text was presented below the picture with numerical indicators of what each text described (split attention format) to induce search processes irrelevant for learning. On level three, the text and picture were presented like in level two, but without the numbers indicating where each text belonged and learners could drag and drop the text blocks into blanks within the picture, thereby actively generating an integrated format (active integration format). For more details see Section 2.4.
2.3 Procedure
The study took place in research laboratories that seated up to three participants simultaneously. After welcoming, participants were seated on a computer screen each with a 24″ monitor. The setup was identical for all participants. After an introduction into the study and giving informed consent, participants received a short pre-study questionnaire assessing rough indicators of self-assessed prior knowledge and topic-specific interest (see Section 2.1).
Afterwards, participants received multimedia learning material on stimulus transduction at a synapse (text and graphic) from Florax and Plötzner (2010). It was presented in an integrated format and explained basic functions and processes of synaptic transduction (see Section 2.4). Participants had 4 minutes to familiarise themselves with the process. Afterwards they were redirected to their respective learning material according to condition. The learning material was identical except for the integration of the material (see Section 2.4) and consisted of a multimedia representation of an inactive, excited and inhibited synapse. Learners had 7 minutes to study the information before they were redirected to another questionnaire page.
For questionnaires, they first filled out a short motivational questionnaire based on Wilde and colleagues et al. (2009) assessing interest/enjoyment, perceived competence and pressure/tension. As the information is not relevant for the purpose of this study, results are reported in Table 1 but not discussed further. Afterwards, learners were asked to provide various metacognitive judgments. They were asked how many items they expected to answer correctly on a 30-item test. This is consistent with a judgment-of-learning as used by Wiley and colleagues (Wiley et al., 2008). Further and on the same questionnaire page, they were asked to provide metacomprehension judgments (comparable to Thiede et al., 2003), first on the overall topic (information transmission within the nervous system) and then separately for processes regarding an inactive, an excited and an inhibited synapse. The last three were later combined to assess metacomprehension (see Section 2.5.2). As we were interested in how the learners judged their current level of comprehension of the material rather than how they predicted their future performance, we used the metacomprehension judgments for our analyses. Both measures (judgment-of-learning and metacomprehension judgments) were highly interrelated (ρ = 0.716, p < 0.001). Data on the judgments-of-learning are included in Table 2 for reference, but please note that the measures (including monitoring accuracy) only provide very rough indicators as they are based on one prediction of a performance in an unknown test. Thus, this data may provide background information, but will not be considered for further analyses. After providing metacomprehension ratings, participants were asked to fill out the StuMMBE-Q by Krell (2015), a 12-item instrument to assess mental effort and mental load (see Section 2.5.1). Learners were then asked to conduct a 30-item comprehension test adapted from Beege and Colleagues (2019). Finally, learners filled out a demographic’s questionnaire, assessing age, gender and course of study. Afterwards, they were thanked and were able to receive course credit for participation. The procedure is depicted in Figure 2.
While at first glance, the procedure may seem inappropriate for the assumed mediation model as it is widely agreed upon (and usually a good strategy) that in mediation and regression analyses, predictors should be assessed prior to mediators and criterion variables, we chose to change the order of assessment. This has various reasons. First, the order of assessment is rather less relevant to theoretical assumptions as overtly sometimes implied, but it is the actual order of occurrence of the true processes that matter. While assessing predictors before a criterion (or mediator) ensures the order of assessment matches the order of occurrence, more stable predictors not fazed by timely changes do not need to be assessed prior to a criterion variable if it can be validly argued that they preceded the criterion. While we not necessarily expect comprehension or (self-assessed) mental load or effort to be stable over a longer period of time, no relevant learning and rehearsal processes will take place after the learning phase and especially comprehension should thus be relatively stable for the short amount of time it takes to fill out a few short metacomprehension questions. Second, some variables are much more susceptible to influence than others and the order of assessment may severely affect results and mask true relationships between constructs. While asking for metacognitive judgments may act as metacognitive prompts during or before learning (e.g., Schnaubert and Bodemer, 2017), these are unlikely to affect either mental load or mental effort (or their assessment) or comprehension when there is no further study phase. On the other hand, metacomprehension judgments are assumed to be highly susceptible to assessing other variables. For example, testing for comprehension may severely alter metacognitive judgments as learners may use their experience during testing as indicators for their actual comprehension (e.g., Maki, 1998). If testing is just a means to an end (to assess comprehension) rather than part of the studied scenario, assessing metacomprehension after conducting a comprehension test would have blurred all prior existing connections. Similarly, the assessment of mental effort or mental load may impact metacognitive judgments. As we assume those to be used as indicators during learning, assessing them before metacomprehension might act as a self-fulfilling prophecy with no meaning for real-life processes. Thus, we opted to assess the criterion before the predictors and mediator.
2.4 Learning Material and Independent Variable
The learning material consisted of two webpages. On the first webpage, an introduction to the learning topic “Functioning of a synapse” was given. This introduction consisted of definitions and explanations of the nervous system and its components obtained from Florax and Plötzner (2010). The second webpage included a graphic of a synapse with three segments. This graphic was also obtained from Florax and Plötzner (2010). The second webpage was prepared with three different versions of the graphic. The first version of the graphic showed a synapse that explained the processes at a non-active synapse, the processes at an excited synapse, and the processes at an inhibited synapse. Overall, 21 synaptic sub-processes were displayed within the graphic, and every subprocess had an associated text label. In this version of the graphic, all verbal explanations of processes were displayed in rectangular boxes close to the place that stands for the process in the graphic. This version of the graphic is further called “Integrated format”. A second version of the graphic showed the same graphic but the verbal explanations were exchanged by numbers. Then, under the graphic, all the (numbered) explanations were listed one by one. This version is further called “Split-Attention format”. The third version of the graphic was designed similar to the graphic in the first version. In contrast, the verbal explanation boxes did not contain any information but a white box. Similar to the second version, under the graphic, all the explanations were listed one by one. In contrast to the second version, learners were able to drag the verbal explanations to the appropriate boxes. All boxes were programmed to accept the placement of correct explanations only so that learners did not combine an explanation box with a false box place. When learners dragged an explanation onto an incorrect place, the explanation automatically returned to the list under the graphic. This third interactive version of the graphic is further called “Active Integration format”. In all versions of the second webpage, a timer was integrated. This timer was set to 7 minutes in order to regulate the learning time of students. After this timer expired, learners were directed to the next webpage.
2.5 Dependent Variables
2.5.1 Mental Load and Mental Effort
Mental load and mental effort were measured with the StuMMBE-Q, a questionnaire developed by Krell (2015), Krell (2017). The questionnaire consists of 12 items. Six items assessed mental load (Cronbach’s α = 0.839; e.g., “The contents of the tasks were complicated”). Another six items assessed mental effort (Cronbach’s α = 0.780; e.g., “I have given my best to complete the tasks”). Please note that the items for mental effort include asking participants to judge whether they tried hard to solve the task, and the measure thus contains elements of self-agency which is a somewhat different conceptualization than the one-item scale by Paas (1992). Students had to rate these items using a 7-point equidistant response format as within the original conceptualization ranging from “not at all” to “totally”. While we are aware of the research indicating a 3-point format may ease distinction between categories (Krell, 2015), for the models assumed we feared a 3-point scale may not be adequately used to assume more than ordinal level of information (Leppink, et al., 2013). We used mean answers as estimates for (perceived) mental load and mental effort. Intercorrelation between both scales was low and non-significant (r = −0.028, p = 0.730).
2.5.2 Metacomprehension
In line with a method used by Thiede and colleagues (e.g., Thiede et al., 2003) and based on previous work by Glenberg and Epstein (1985), metacomprehension was measured by asking learners to rate their understanding of the learning content using a 7-point equidistant response format from “very poorly” (1) to “very well” (7). We asked them separately for their understanding with regard to the processes on an inactive, an excited and an inhibited synapse and used their mean metacomprehensionrating as indicator for the level of metacomprehension. Cronbach’s α was at 0.909.
2.5.3 Comprehension
Comprehension was measured with 30 multiple-choice questions (Cronbach’s α = 0.739) adapted from Beege and Colleagues (2019). These questions were 5-answer single-choice questions with “I don’t know” as one option. Some questions contained verbal answer options, some graphical answer options, some a combination of both. In order to answer these questions, participants needed to remember and understand the verbal explanations and the processes displayed in the graphic. If participants marked the correct answer of one question, one point was given. We used the number of correctly answered questions as estimator for comprehension. Overall, learners could achieve a maximum of 30 points.
2.6 Statistical Models
To answer our research questions, we conducted a number of mediation models with (perceived) mental load and mental effort as predictors, comprehension as the mediator and metacomprehension as criterion (all standardised; Figure 1). We used the jamovi 1.1.9.0 JAMM package and 10,000 percentile bootstrapping and 95% CI to estimate beta. We first used all data for an overall model and then computed one model for each multimedia condition. α was set at 5%.
3 Results
Before we report the model results, we report relevant descriptive statistics and the results of testing for differences in the variables between the conditions.
3.1 Descriptive Statistics and Group Differences
Table 3 shows the descriptive statistics for the relevant variables by condition. We can see that—in contrast to prior assumptions assuming active integration fosters comprehension—students in the active integration condition performed worst at the comprehension test and accordingly also judged their comprehension lowest.
We conducted Welch’s ANOVA to test for differences between the groups in terms of (perceived) mental effort, (perceived) mental load, comprehension or metacomprehension (cf. Table 3). We found mental effort and mental load not to differ significantly. Thus, although the conditions were meant to induce various levels of cognitive load, the overall level did not differ. However, this was not the case for comprehension [F (2,101) = 5.75, p = 0.004] or metacomprehension [F (2,101) = 3.19, p = 0.045]. Games Howell post-hoc test confirmed a difference between the learners working with the active integration material and those using integrated material [t (98.1) = 3.24, p = 0.005] for comprehension with learners with the active integration material scoring significantly worse in the comprehension test, but not between learners working with the active integration material and those learning with split-attention material or between those with split-attention and integrated material. For metacomprehension, the picture was similar. Again, learners with the active integration material had significantly lower ratings than learners with integrated material [t (99.7) = 2.53, p = 0.034], but not than learners with split attention material and the latter groups did also not differ. See Table 4 for the full results of the post-hoc tests.
We further conducted correlation analyses (cf. Table 5 for full results). As expected, comprehension and metacomprehension showed a rather high correlation (r = 0.571). Mental effort (r = 0.260) and mental load (r = −0.291) rather weakly correlated with comprehension. They did not correlate with each other significantly (r = −0.028). Metacomprehension, however, showed a rather weak correlation with mental effort (r = 0.267) but a considerably stronger, albeit negative, association with mental load (r = −0.512).
As the active integration condition was not informationally equivalent to the other conditions due to the missing link between text boxes and correct placements, we additionally checked how many of the students managed to correctly connect textual and pictorial information. Results showed that by the end of the learning phase, 39 (74%) participants had correctly placed at least 20 text boxes in the respective fields (meaning all assignments were ascertained), 11 (21%) had not, and 3 (6%) could not be confidently analysed due to a logging failure.
3.2 Mediation Analyses
The mediation analyses were conducted using jamovi’s JAMM package. We used z-standardised variables and used 10,000 percentile bootstrapping to estimate beta-coefficients.
3.2.1 Mediation Model (all Conditions)
To assess if and how perceived mental effort and load affected metacomprehension judgments (beyond their actual effects on comprehension), we first computed one mediation model over all conditions, disregarding possible differences. We found all paths to be highly significant and both direct and indirect effects of mental effort (positive) and mental load (negative) on metacomprehension (Table 6 and Figure 3). While the indirect effects of effort and load seem to be comparable in size (although not direction) due to the comparable effects of load and effort on comprehension, the direct effect of load seems considerably larger than the effect of effort, indicating that learners’ metacognitive judgments seem to be more sensitive to (perceiving) mental load than mental effort. In general, perceived mental effort affected metacomprehension positively (β = 0.25, p < 0.001) and perceived mental load negatively (β = −0.51, p < 0.001), thus confirming hypotheses 1 and 2.
3.2.2 Mediation Models per Condition
To see if the effects vary under different load-imposing multimedia conditions, we then computed mediation models for each condition separately. An overview of the results can be seen in Figure 4 (active integration format), Figure 5 (split attention format), and Figure 6 (integrated format), the full results in Table 7. As can be seen, within all conditions, there was a clear effect of comprehension on metacomprehension (b) and although not exactly equal, the size of the effect is roughly comparable, albeit descriptively a bit larger for learners using integrated material. There was also a direct negative effect of mental load on metacomprehension (c1), descriptively larger for learners using active integration material, even when indirect effects were eliminated (c1’; cue utilization). However, as mental load seems to not be very indicative of comprehension for learners with the split attention and integrated format (a1; diagnosticity), indirect effects of mental load on metacomprehension via comprehension were only confirmed for learners within the active integration condition (partial mediation).
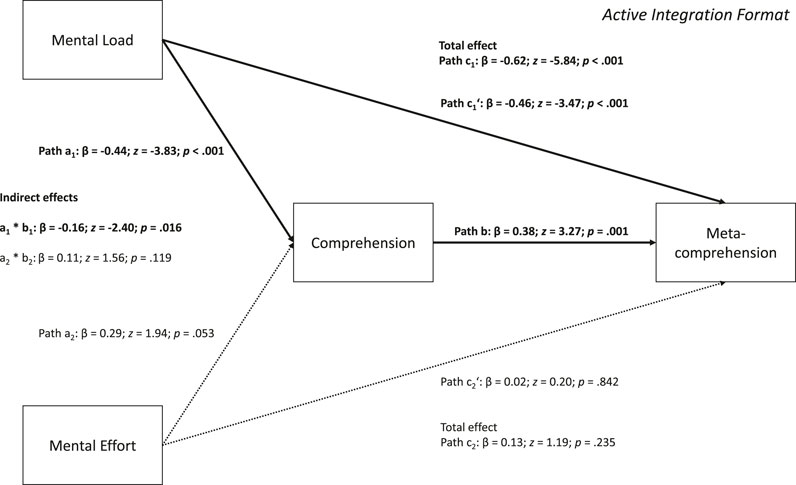
FIGURE 4. Mediation model active integration format (statistically significant effects in bold font).
For mental effort, this looked somewhat different. Here, mental effort only seems to be indicative of comprehension for learners provided with the integrated material (a2) and thus, the indirect effect was statistically significant only for those. However, while the indirect effect fully explained the effect of mental effort on metacomprehension for learners with integrated material, learners with split attention material showed a significant direct effect of mental effort on metacomprehension not explained by comprehension (c2’). Thus, even lacking diagnosticity for the latter, for learners with split attention material mental effort seems to be a relevant factor for metacomprehension judgments. For learners with active integration material, mental effort seems to be less relevant.
As statistically securing smaller effects may have been hampered by the lower power of the separate mediation models and the described differences may not be generalised beyond the sample, we conducted further post-hoc analyses (see Section 3.2.3).
3.2.3 Post-Hoc Comparisons of the Models
To test if the (direct) effects between mental load and effort on metacomprehension differed significantly between the conditions, we integrated condition as a moderator on the c’ path using 10,000 percentile bootstrapping. We contrasted all conditions separately and used bonferroni corrected alpha-levels (0.017) to adjust for alpha-error inflation. Results for the moderations showed a significant moderation effect only for the split attention versus active integration contrast (for full results Table 8) with the direct effect of mental effort on metacomprehension being highly significant within the split attention condition and substantially smaller and non-significant within the active integration condition (moderation effect: β = 0.19, z = 3.04, p = 0.002). While the integrated conditions’ effect descriptively ranged somewhere in between (Table 7), the difference was not statistically significant between the integrated and any of the other conditions. There were no significant moderation effects on the c’ path between mental load and metacomprehension.
Please note that even though mental load and mental effort did not differ significantly between our experimental conditions in the study and the predictors showed no sign of multicollinearity, possible influences of the conditions (moderator) on the predictors cannot be completely discarded and the results of the moderation models thus need to be treated with caution and may only provide a first indicator on possible moderation effects.
4 Discussion
4.1 Effects on Comprehension
In general, as expected, we found the relationships between (perceived) mental effort or mental load and metacomprehension to be positive for the former and negative for the latter (hypotheses 1 and 2) and a positive relationship between comprehension and metacomprehension. Comparable to other research, mental effort and load were not related (e.g., Minkley et al., 2021). Although the effects of mental load and mental effort on comprehension were not large, the relationship for both but especially for mental load seems to be comparable to findings in the literature (e.g., Krell, 2017; Minkley et al., 2021). This confirms basic research on cognitive load and multimedia learning, but also highlights the relevance of mental effort exerted by learners as a distinct construct apart from mental load (e.g., Paas and van Merriënboer, 1994). Thus, it is important to separately measure and include both when studying (multimedia) learning. While this did not hold true for each experimental condition separately and thus may vary under various load-inducing designs of learning material, a lack of statistical power may have contributed to these observed differences.
In the two load-inducing conditions (split attention and active integration), mental effort seems to have less effect on comprehension (less diagnosticity) and although smaller effects might have been statistically secured with more power to the analyses, especially for the split attention condition, effort seems to be rather futile. This is especially interesting as the level of mental effort and mental load did not differ between the condition, so all groups reported to have exerted similar effort, but to different avail. It seems that if learning material is designed to reduce cognitive load, the effort learners put into the learning process shows the greatest effect, while it is less effective when learners are asked to actively integrate the information and least effective under a split attention format, presumably because most of the effort exerted is wasted on processes not directly relevant for learning (unproductive).
For mental load, the picture was different. Here, (negative) effects on comprehension were especially strong within the active integration condition and were smaller with the split attention format and smallest in the integrated format. It seems that if actively integrating text and graphics put extra load on the learner, comprehension suffered. This is interesting, as it was assumed that actively integrating information would rather induce productive learning processes and successful conduction would thus foster comprehension (cf. Bodemer et al., 2004; Bodemer et al., 2005). However, the active integration condition showed lower comprehension after the learning phase (a descriptive difference which was also statistically confirmed in comparison with the integrated format) and it is thus possible, that due to the complexity of the material, they never overcame the split attention format (without proper assignment) and just did not manage to benefit from the design. Although most participants managed to integrate the information (at least physically), a considerable percentage did not manage to integrate the information within the given timeframe. This gave these learners a further disadvantage as without correct assignments, the conditions were not informationally equivalent.
4.2 Effects on Metacognition
As for metacomprehension, we did confirm effects of comprehension on metacomprehension and although somewhat stronger in the integrated format, the relationship between comprehension and metacomprehension was in general consistent with effects found in metacognition research (e.g., Schleinschok et al., 2017).
However, apart from comprehension, other factors contribute to explaining metacomprehension variance, for example mental effort. The first important observation is that the relationship between mental effort and metacomprehension is positive. This would be expected assuming learners are aware of the positive influence invested mental effort has on comprehension and learning. However, Baars and colleagues (2013) found (strong) negative correlations between judgments of learning and mental effort using the one-item scale by Paas (1992). Explicitly differentiating between mental load imposed and mental effort invested by using the instrument by Krell (2015) may have led to a more refined picture, shifting the focus more clearly on effort as a voluntary activity [“I have given my best (…)”] as opposed to task difficulty [“The tasks were easy (…)”]. However, this also means that a differential view is necessary to understand how learners form metacognitive judgments. A recent meta-analysis by Baars et al. (2020) comes to a similar conclusion: They find that the usually found negative relationship between metacognitive judgment and effort vanished when considering rating scales promoting self-agency. Thus, when effort regulation is goal-driven, said effort may be interpreted positive, while this may revert when it is data-driven (Koriat, 2018; de Bruin et al., 2020). The scale by Krell (2015) used in our study arguably conceptually aligns with a self-agent view and thus, the found relationship seems to align with findings in literature.
Learners in the integrated format condition seem to have (rightfully) used their mental effort as an indicator for comprehension. While our research design did not allow to ascertain cue usage in an intentional way, the effect of mental effort on metacomprehension is mediated by actual comprehension. However, for learners learning with material in a split attention format effort also seems to influence metacomprehension, although it does seem less diagnostic for comprehension. Thus, while these learners judge their comprehension higher when exerting more effort, actual comprehension was rather unfazed. Such mechanisms could potentially lead to misjudgments and lower monitoring accuracy, which may severely hamper self-regulated learning attempts when making study decisions (Thiede et al., 2003; Schnaubert and Bodemer, 2017). For learners using an active integration format, mental effort was not significantly related to metacomprehension. Due to the design providing feedback during learning (explanation did only stick to correct places), learners may have experienced their efforts being more or less fruitful during the learning process. While this warrants further investigation, feedback has previously been found to not only correct faulty assumptions, but also metacognitive errors (e.g., Butler et al., 2008). The difference between the split attention and active integration condition with regard to the effect of mental effort was also confirmed by the post-hoc moderation analyses which showed a significant effect of condition on the direct relationship between mental effort and metacomprehension (when the mediation effect was excluded). While these results have to be treated with caution, it seems that multimedia design may affect the relationship between mental effort invested and metacomprehension reported.
Mental load is strongly connected to metacomprehension under all conditions of learning material provided, and this relationship is even higher than the one between comprehension and metacomprehension. This may be an indicator for learners using mental load as a cue for judging their comprehension. While at least for active integration, a significant part of this is mediated by comprehension, a large portion of (negative) effect is unwarranted. Learners may overestimate the negative effect of load on comprehension, especially when learning with integrated, theoretically less load-inducing multimedia learning material. Again, this could lead to severe misjudgments and hamper self-regulated learning.
4.3 Mental Load, Mental Effort and Metacognition
Overall, these results show that mental load and mental effort are distinct concepts related differently to metacomprehension and should be studied in line with other cognitive experiences regarded as cues for metacognitive judgments like ease-of-processing or ease-of-retrieval (e.g., Benjamin and Bjork, 2014 see also Koriat and Ma’ayan, 2005). While the causal relationship cautiously assumed in our models are based on theoretical assumptions rather than experimental design, there is merit in combining research on metacognition with the vast amount of research regarding cognitive load and multimedia learning. However, there are some conceptual issues that may need specific attention when doing so (e.g., Sweller and Paas, 2017). One of these is the perspective taken on the constructs involved. Cognitive load research is mostly concerned with (working) memory processes and resources. Thereby, cued self-report is a means to gather information about cognitive processes assuming these are not only accessible, but also transformable to a given scale (for more detail, see Paas et al., 2008). This is inherently different for metacognition research. Here, the subjective view on cognitive constructs (like comprehension) is not a measurement issue, but inherent in the concept (Nelson and Narens, 1990; Nelson and Narens, 1994). Within metacognition research, metacomprehension scales are not meant to measure comprehension but an individual’s unique perception of their own comprehension. What would a metacognitive view on cognitive load look like? If we assume mental load and mental effort to be directly accessible for learners (as assumed when using self-report to measure it), is awareness of this as a metacognitive construct just an epiphenomenon with no impact or does it actually affect learning processes? Our research as well as other current approaches viewing mental effort through a metacognitive lens (e.g., Scheiter et al., 2020) suggest that monitoring mental load and mental effort is more than just a fall-out, but may actually be relevant for forming metacognitive judgments. Although requiring further empirical research, in line with the cyclic model of metacognitive regulation assumed in metacognition theory (e.g., Nelson and Narens, 1990), this means that learners may use this information to regulate their learning processes, for example by exerting effort, allocating study time and diverting attention. This brings us to another understudied yet highly discussed question of how learners regulate mental effort itself and how this may depend on their perception of cognitive load (de Bruin and van Merriënboer, 2017; see also de Bruin et al., 2020). Including multimedia research and building on well-established multimedia design effects may help to strategically design further studies to investigate not only how perceived mental load and effort affect metacognitive judgments, but how sensitive these relationships are to differences in diagnosticity of perceived mental load and effort for actual learning gains.
While our research showed first connections between perceived mental load, perceived mental effort, comprehension and metacomprehension under various load-inducing design conditions of multimedia learning material, there are several open questions that need further discussion. First, while the various effects under specific design conditions all need replications with different material and substantial statistical power to validly generalise the effects for more or less demanding content, especially the results of active integration seem puzzling. While the lack of beneficial effects of active integration may have been due to learners not performing mental activities while behaviorally integrating the material, active integration as a means to induce productive learning processes may also be more or less effective under various conditions relating to the content or the learners. Although within our sample, learners did not provide ratings indicative of “overload”, it should be taken into consideration that these productive learning processes that were attempted to be triggered did not take place or were even hindered due to the complexity of the task or low prior knowledge (prior knowledge was rated quite low within our sample, see Section 2.1). As with other multimedia effects (e.g., imagination effect; Lin et al., 2017), there could be reversed effects depending on prior knowledge (i.e., expertise reversal; Kalyuga, 2007) and thus, prior knowledge should be taken into consideration in further studies. Second, other interindividual differences may need to be considered as well. Cue utilization theory does not make specific assumptions about interindividual differences, but research has found that not all learners use the same cues. Although self-reported cue use needs to be viewed with caution, Thiede and colleagues (2010), for example, found especially high-risk readers reported using more surface level cues (i.e., relating to surface properties of a text) than typical readers. This strengthens the notion that not only do learners vary in their cue use, but also that this may be trainable (Wiley et al., 2016). A current meta-analysis found that interventions supporting learners’ use of situation-model cues (i.e., cues relating to the learners’ situation model constructed during text comprehension; cf. Kintsch, 1994) positively and considerably affect metacomprehension accuracy (Prinz et al., 2020). While we were rather interested in how perceived mental load and effort affected metacomprehension judgments under various load-inducing conditions, a next step would be to assess how this affects metacomprehension accuracy within students.
4.4 Limitations
As with all research, there are limitations to this study. First of all, our sample size especially for the single mediation models was too small to confirm smaller effects. While we can draw some conclusions about the processes involved, a final verdict needs careful replications of the found differences between the conditions to confirm and potentially generalise the effects. Additionally, we could not confirm differences in (perceived) mental effort or mental load between conditions, which could be due to incorrect assumptions about the relationship between treatment and cognitive load (which is improbable for split attention and the integrated format, but may hold true for the less studied active integration format). However, it could also be due to the assessment of load and effort, which may have been invariant to more subtle changes, especially since it was measured with a delay. However, the instrument had shown sensitivity to instructional variations before (Krell, 2017) and we did find expected (albeit rather small) relationships with comprehension giving at least small indications of valid assessment. Additionally, differences in how learners’ effort and load was related to metacomprehension between conditions indicate some sensitivity of the (perceived) mental load and mental effort measurement to changes. While there are other measures of cognitive load, we opted for the questionnaire by Krell (2015), as it differentiates between mental load imposed and effort deliberately invested, which are both central when studying self-regulatory processes in multimedia learning. Due to the intricate relationship between the requirements of a task and regulatory processes by the learner, the relationship between cognitive load and metacognition is a source for some debate (e.g., de Bruin and van Merriënboer, 2017; Seufert, 2020). Thus, it seems vital to differentiate between material-induced mental load and invested mental effort (see also Seufert, 2020) as the latter may be regulated by learners (de Bruin et al., 2020) while the former much more relies on the instructional design (although both may affect each other). Further studies may put more focus on the type of load imposed by the material and the reasons for investing mental effort in the learning task to distinguish more or less beneficial load conditions [see also discussion by Seufert (2020)], possibly linking more active (effort) and more passive (load) aspects of cognitive load to the assumed tripartite nature of the cognitive load concept (see Klepsch and Seufert, 2021). As the assessment (and structure) of cognitive load is subject to an ongoing debate (see for example Kirschner et al., 2011), a combination of multiple measures may prove useful, and while objective measures may provide reliable information for instructional design and multimedia research (e.g., Korbach et al., 2017), subjective measures additionally provide insights about subjective judgments of effort or load (Scheiter et al., 2020) that are relevant when the aim is to understand how learners themselves view their learning process and regulate their behaviour. Additionally, various subjective measures focus on different aspects of load and effort that may lead to very different empirical results (e.g., elements of self-agency, Baars et al., 2020).
Rather than a limitation, a further open question concerns the role of restricting study time on the results found. It is not uncommon that multimedia effects are more pronounced under conditions of system-paced time pressure (e.g., Rey et al., 2019). While a defined time frame allowed us to minimise effects of self-regulatory processes (i.e., study time decisions) to affect comprehension and thus fostered experimental scrutiny within our setup, Baars and colleagues’ (2020) meta-analysis found the (negative) relationship between mental effort and monitoring judgments to diffuse under time pressure. Following de Bruin and colleagues (2020) argumentation, our setup could have reinforced a positive interpretation of effort by fostering a more goal-driven approach. Coupled with our use of a mental effort scale including elements of self-agency (see above), this may have reinforced the positive association and may not be generalisable to settings allowing for self-paced study.
Another limitation to be discussed are the potentially incorrect assumptions about the use of the active integration format. While we did assume learners to actively integrate the material, which had previously been shown to be beneficial for learning (e.g., Bodemer et al., 2004) and roughly 3/4 of learners did manage to correctly assign the boxes, learners may have just actively dragged and dropped the boxes without mentally integrating the content (trial and error). Such mere behavioural activity however (correctly placing the boxes) is not in itself conducive to learning, but has to be accompanied by cognitive activity (mentally connecting concepts and building coherent mental representations) to show the assumed positive effects (Mayer, 2001). Thus, apart from participants simply not managing to integrate the information (See Section 4.1), participants focussing on behavioural (versus cognitive) activities may have contributed to the findings in our study and explain why test performance was worst for the active integration condition.
One further and central limitation of the study is that we cannot rule out that further variables affect the found relationships or that the direction of effect may be different than assumed. While we based our model on theoretical assumptions about (causal) processes, our empirical model can only confirm the relationship rather than the mechanisms involved. While we randomised participants’ allocation to experimental conditions and found differences between metacomprehension ratings as a result, that does not exclude the possibility that learners perceiving themselves as more potent and therefore provide higher metacomprehension ratings may have exerted more mental effort during learning rather than using effort as a cue to judge their comprehension. Further, additional variables may affect the found results. For example, Zu and colleagues found that prior knowledge may influence how learners respond to cognitive load items affecting the factor structure (Zu et al., 2021), which may simultaneously affect metacomprehension. Although the authors used a different survey that was designed to measure the tripartite nature of cognitive load, prior knowledge may have affected our measures of cognitive and metacognitive processes as well. While load imposed by the design of learning material may be manipulated in experimental settings, disentangling metacognitive monitoring and invested effort is harder to accomplish as it is assumed learners actively regulate their mental effort during learning (de Bruin and van Merriënboer, 2017), but would give further insights into the relationship between cognitive processes during multimedia learning and metacognitive regulation. Directly manipulating mental load and effort without running the risk of the intervention affecting metacomprehension simultaneously may be a hard to accomplish goal, but would be pertinent to ascertain causality as implied by the theoretical model assumptions underlying the cue utilization model proposed. Additionally, while we decided upon the order of assessing our variables with possible sequence effects in mind, we cannot fully exclude possible interferences. For example, the motivational questionnaire may have had unexpected effects when learners judge their perceived competence or interest. Allowing for more scrutiny, follow-up research may use within-subject variations of multimedia-material, on-time measurements of effort and load and additional metacognitive measures and explicitly test for sequence effects or randomise the order of assessment to provide further insights into how learners take variations of multimedia design into account when monitoring their comprehension during multimedia learning.
5 Conclusion
Our research suggests that the subjective perceptions of mental load and mental effort are not epiphenomenons only to be considered for assessment purposes, but that these perceptions may impact learners’ self-regulatory processes. Although it would be inappropriate to conclude an intentional cue usage from the data collected in this study, independent of the validity of the subjective measures (i.e., their relation to actual mental load and mental effort), experiences of mental load and invested effort may inform learners and may be used as cues when making monitoring judgments. As put by Nelson and Narens (1990, p .128): “A system that monitors itself (even imperfectly) may use its own introspections as input to alter the system’s behavior”. We argue that while the validity of subjective measures may be a major concern for research on cognitive load, the value of information about the subjective experience of those processes is underrated and warrants further empirical (and possibly experimental) research. Thus, with validity of assessments referring to interpretations and usage of scores (Kane, 2013), a metacognitive perspective on cognitive load shifts the focus from assessing cognitive processes to assessing their subjective experience by learners (please note that the term “experience” in this context refers to conscious perceptions, but is also being used to differentiate passive and active forms of load; e.g., Klepsch and Seufert, 2021). The found relationships between perceived mental load, perceived mental effort and metacomprehension indicate not only that perceived mental load and effort are distinct concepts, but that they may play an additional role for learning which hinges on their subjective experience by learners. Although the direction of effect cannot conclusively be established within this study, a metacognitive view on cognitive load has implications for the interpretation of subjective measures of cognitive load. While studying the relationship between subjective and objective measures of cognitive load may be a matter of validating assessment strategies (e.g., Minkley et al., 2021), it also establishes a relationship between cognitive processes and their idiosyncratic experience. Applying Nelson and Narens’ view on metacognition (Nelson and Narens, 1994), misalignment between the two is a distortion providing insights into how learners perceive their mental processes and may thus be studied in terms of metacognitive accuracy. Thus, validly interpreting subjective measures needs to consider their subjectivity explicitly while studying possible distortions. Validity hinges on the interpretation of a score rather than the score itself (e.g., Kane, 2013). Consequently, when studying self-regulatory processes during (multimedia) learning, carefully implementing subjective rating scales may be more appropriate to capture the subjective experience than physiological measures. While this does not mean subjective rating scales may validly assess experiences of effort and load per se and for example scale characteristics (Ouwehand et al., 2021) or the framing with regard to self-agency (Koriat, 2018) may influence results, it stresses the need to differentiate between cognitive load and their impact on learning processes and its subjective experience, which may impact metacognitive regulation. Our study provided some indications for the relevance of experiences of mental load and effort for learning and thus calls for a conceptual rather than methodological differentiation between cognitive processing and their idiosyncratic experience when research targets learning regulation rather than working memory capacity.
Data Availability Statement
The raw data supporting the conclusion of this article will be made available by the authors, without undue reservation.
Ethics Statement
The studies involving human participants were reviewed and approved by the Ethics Committee of the division of Computer Science and Applied Cognitive Sciences at the Faculty of Engineering at the University of Duisburg-Essen, Germany. The patients/participants provided their written informed consent to participate in this study.
Author Contributions
LS: planned and supervised experiment, conducted majority of statistical analyses, wrote majority of the manuscript (focus on metacognition). SS: technical realisation of experiment, supported data analyses, wrote parts of the manuscript (focus on multimedia), proofreading.
Conflict of Interest
The authors declare that the research was conducted in the absence of any commercial or financial relationships that could be construed as a potential conflict of interest.
Publisher’s Note
All claims expressed in this article are solely those of the authors and do not necessarily represent those of their affiliated organizations, or those of the publisher, the editors and the reviewers. Any product that may be evaluated in this article, or claim that may be made by its manufacturer, is not guaranteed or endorsed by the publisher.
Acknowledgments
We want to thank Ebru Yilmaz and Sonja Glantz for their support in planning the study and collecting data, Peter Bellstedt for programming especially the active integration website, and Mareike Florax and Rolf Plötzner for the permission to use and adapt the learning material. We acknowledge support by the Open Access Publication Fund of the University of Duisburg-Essen.
References
Anmarkrud, Ø., Andresen, A., and Bråten, I. (2019). Cognitive Load and Working Memory in Multimedia Learning: Conceptual and Measurement Issues. Educ. Psychol. 54, 61–83. doi:10.1080/00461520.2018.1554484
Ayres, P., and Youssef, A. (2008). “Investigating the Influence of Transistory Information and Motivation during Instructional Animations,” in Proceedings of the 8th International Conference of the Learning Sciences. Editors P. A. Kirschner, F. Prins, V. Jonker, and G. Kanselaaer (Utrecht, Netherlands: International Society of the Learning Sciences), Vol. 1, 68–75.
Baars, M., van Gog, T., de Bruin, A., and Paas, F. (2017). Effects of Problem Solving after Worked Example Study on Secondary School Children's Monitoring Accuracy. Educ. Psychol. 37 (7), 810–834. doi:10.1080/01443410.2016.1150419
Baars, M., Visser, S., Gog, T. v., Bruin, A. d., and Paas, F. (2013). Completion of Partially Worked-Out Examples as a Generation Strategy for Improving Monitoring Accuracy. Contemp. Educ. Psychol. 38 (4), 395–406. doi:10.1016/j.cedpsych.2013.09.001
Baars, M., Wijnia, L., de Bruin, A., and Paas, F. (2020). The Relation between Students' Effort and Monitoring Judgments during Learning: A Meta-Analysis. Educ. Psychol. Rev. 32 (4), 979–1002. doi:10.1007/s10648-020-09569-3
Beege, M.Colleagues (2019). Spatial Continuity Effect vs. Spatial Contiguity Failure. Revising the Effects of Spatial Proximity between Related and Unrelated Representations. Front. Educ. 4. doi:10.3389/feduc.2019.00086
Begg, I., Duft, S., Lalonde, P., Melnick, R., and Sanvito, J. (1989). Memory Predictions Are Based on Ease of Processing. J. Mem. Lang. 28 (5), 610–632. doi:10.1016/0749-596X(89)90016-8
Benjamin, A. S., Bjork, R. A., and Schwartz, B. L. (1998). The Mismeasure of Memory: When Retrieval Fluency Is Misleading as a Metamnemonic index. J. Exp. Psychol. Gen. 127 (1), 55–68. doi:10.1037//0096-3445.127.1.55
Benjamin, A. S., and Bjork, R. A. (2014). “Retrieval Fluency as a Metacognitive Index,” in Implicit Memory and Metacognition. Editor L. M. Reder (New York, NY: Psychology Press), 321–350. doi:10.4324/9781315806136-19
Berthold, K., and Renkl, A. (2009). Instructional Aids to Support a Conceptual Understanding of Multiple Representations. J. Educ. Psychol. 101 (1), 70–87. doi:10.1037/a0013247
Bertsch, S., Pesta, B. J., Wiscott, R., and McDaniel, M. A. (2007). The Generation Effect: A Meta-Analytic Review. Mem. Cognit 35 (2), 201–210. doi:10.3758/BF03193441
Bjork, E. L., and Bjork, R. A. (2011). “Making Things Hard on Yourself, but in a Good Way: Creating Desirable Difficulties to Enhance Learning,” in Psychology and the Real World: Essays Illustrating Fundamental Contributions to Society (New York, NY, US: Worth Publishers), 56–64.
Bodemer, D., Ploetzner, R., Bruchmüller, K., and Häcker, S. (2005). Supporting Learning with Interactive Multimedia through Active Integration of Representations. Instr. Sci. 33 (1), 73–95. doi:10.1007/s11251-004-7685-z
Bodemer, D., Ploetzner, R., Feuerlein, I., and Spada, H. (2004). The Active Integration of Information during Learning with Dynamic and Interactive Visualisations. Learn. Instruction 14 (3), 325–341. doi:10.1016/j.learninstruc.2004.06.006
Burkett, C., and Azevedo, R. (2012). The Effect of Multimedia Discrepancies on Metacognitive Judgments. Comput. Hum. Behav. 28 (4), 1276–1285. doi:10.1016/j.chb.2012.02.011
Butler, A. C., Karpicke, J. D., and Roediger, H. L. (2008). Correcting a Metacognitive Error: Feedback Increases Retention of Low-Confidence Correct Responses. J. Exp. Psychol. Learn. Mem. Cogn. 34 (4), 918–928. doi:10.1037/0278-7393.34.4.918
De Bruin, A. B. H., Dunlosky, J., and Cavalcanti, R. B. (2017). Monitoring and Regulation of Learning in Medical Education: The Need for Predictive Cues. Med. Educ. 51 (6), 575–584. doi:10.1111/medu.13267
de Bruin, A. B. H., Roelle, J., Carpenter, S. K., and Baars, M. (2020). Synthesizing Cognitive Load and Self-Regulation Theory: A Theoretical Framework and Research Agenda. Educ. Psychol. Rev. 32 (4), 903–915. doi:10.1007/s10648-020-09576-4
de Bruin, A. B. H., and van Merriënboer, J. J. G. (2017). Bridging Cognitive Load and Self-Regulated Learning Research: A Complementary Approach to Contemporary Issues in Educational Research. Learn. Instruction 51, 1–9. doi:10.1016/j.learninstruc.2017.06.001
Dunlosky, J., and Lipko, A. R. (2007). Metacomprehension. Curr. Dir. Psychol. Sci. 16 (4), 228–232. doi:10.1111/j.1467-8721.2007.00509.x
Dunlosky, J., Rawson, K. A., and Middleton, E. L. (2005). What Constrains the Accuracy of Metacomprehension Judgments? Testing the Transfer-Appropriate-Monitoring and Accessibility Hypotheses. J. Mem. Lang. 52 (4), 551–565. doi:10.1016/j.jml.2005.01.011
Florax, M., and Ploetzner, R. (2010). What Contributes to the Split-Attention Effect? the Role of Text Segmentation, Picture Labelling, and Spatial Proximity. Learn. Instruction 20, 216–224. doi:10.1016/j.learninstruc.2009.02.021
Glenberg, A. M., and Epstein, W. (1985). Calibration of Comprehension. J. Exp. Psychol. Learn. Mem. Cogn. 11 (4), 702–718. doi:10.1037/0278-7393.11.1-4.702
Kalyuga, S. (2007). Expertise Reversal Effect and its Implications for Learner-Tailored Instruction. Educ. Psychol. Rev. 19 (4), 509–539. doi:10.1007/s10648-007-9054-3
Kalyuga, S., and Singh, A.-M. (2016). Rethinking the Boundaries of Cognitive Load Theory in Complex Learning. Educ. Psychol. Rev. 28, 831–852. doi:10.1007/s10648-015-9352-0
Kane, M. T. (2013). Validating the Interpretations and Uses of Test Scores. J. Educ. Meas. 50 (1), 1–73. doi:10.1111/jedm.12000
Kelemen, W. L. (2000). Metamemory Cues and Monitoring Accuracy: Judging what You Know and what You Will Know. J. Educ. Psychol. 92 (4), 800–810. doi:10.1037/0022-0663.92.4.800
Kintsch, W. (1994). Text Comprehension, Memory, and Learning. Am. Psychol. 49 (4), 294–303. doi:10.1037/0003-066X.49.4.29410.1037//0003-066x.49.4.294
Kirschner, P. A., Ayres, P., and Chandler, P. (2011). Contemporary Cognitive Load Theory Research: The Good, the Bad and the Ugly. Comput. Hum. Behav. 27 (1), 99–105. doi:10.1016/j.chb.2010.06.025
Klepsch, M., and Seufert, T. (2021). Making an Effort versus Experiencing Load. Front. Educ. 6, 56. doi:10.3389/feduc.2021.645284
Korbach, A., Brünken, R., and Park, B. (2017). Measurement of Cognitive Load in Multimedia Learning: a Comparison of Different Objective Measures. Instr. Sci. 45 (4), 515–536. doi:10.1007/s11251-017-9413-5
Koriat, A. (2018). Agency Attributions of Mental Effort during Self-Regulated Learning. Mem. Cognit 46 (3), 370–383. doi:10.3758/s13421-017-0771-7
Koriat, A., and Ma’ayan, H. (2005). The Effects of Encoding Fluency and Retrieval Fluency on Judgments of Learning. J. Mem. Lang. 52, 478–492. doi:10.1016/J.JML.2005.01.001
Koriat, A. (1997). Monitoring One's Own Knowledge during Study: A Cue-Utilization Approach to Judgments of Learning. J. Exp. Psychol. Gen. 126 (4), 349–370. doi:10.1037/0096-3445.126.4.349
Koriat, A., Nussinson, R., Bless, H., and Shaked, N. (2008). “Information-Based and Experience-Based Metacognitive Judgments” in Handbook of Metamemory and Memory. Editors J. Dunlosky, and R. A. Bjork (New York, NY: Psychology Press), 117–135. doi:10.4324/9780203805503.ch7
Krell, M. (2017). Evaluating an Instrument to Measure Mental Load and Mental Effort Considering Different Sources of Validity Evidence. Cogent Edu. 4 (1), 1280256. doi:10.1080/2331186X.2017.1280256
Krell, P. (2015). Evaluating an Instrument to Measure Mental Load and Mental Effort Using Item Response Theory. Sci. Edu. Rev. Lett., 1–16. doi:10.5771/9783845263991-1
Leppink, J., Paas, F., Van der Vleuten, C. P., Van Gog, T., and Van Merriënboer, J. J. (2013). Development of an Instrument for Measuring Different Types of Cognitive Load. Behav. Res. Methods 45 (4), 1058–1072. doi:10.3758/s13428-013-0334-1
Li, J., Antonenko, P. D., and Wang, J. (2019). Trends and Issues in Multimedia Learning Research in 1996-2016: A Bibliometric Analysis. Educ. Res. Rev. 28, 100282. doi:10.1016/j.edurev.2019.100282
Lin, L., Lee, C. H., Kalyuga, S., Wang, Y., Guan, S., and Wu, H. (2017). The Effect of Learner-Generated Drawing and Imagination in Comprehending a Science Text. J. Exp. Edu. 85 (1), 142–154. doi:10.1080/00220973.2016.1143796
Maki, R. H., and Berry, S. L. (1984). Metacomprehension of Text Material. J. Exp. Psychol. Learn. Mem. Cogn. 10 (4), 663–679. doi:10.1037/0278-7393.10.4.66310.1037//0278-7393.10.4.663
Maki, R. H. (1998). “Test Predictions over Text Material,” in Metacognition in Educational Theory and Practice. Editors D. J. Hacker, J. Dunlosky, and A. C. Graesser (Lawrence Erlbaum Associates Publishers), 117–144.
Mayer, R. E. (2001). “A Cognitive Theory of Multimedia Learning,” in Multimedia Learning. Editor R. E. Mayer (Cambridge, UK: Cambridge University Press), 41–62.
Mayer, R. E., and Moreno, R. (2003). Nine Ways to Reduce Cognitive Load in Multimedia Learning. Educ. Psychol. 38 (1), 43–52. doi:10.1207/S15326985EP3801_6
Metcalfe, J. (2009). Metacognitive Judgments and Control of Study. Curr. Dir. Psychol. Sci. 18 (3), 159–163. doi:10.1111/j.1467-8721.2009.01628.x
Minkley, N., Xu, K. M., and Krell, M. (2021). Analyzing Relationships between Causal and Assessment Factors of Cognitive Load: Associations between Objective and Subjective Measures of Cognitive Load, Stress, Interest, and Self-Concept. Front. Educ. 6, 53. doi:10.3389/feduc.2021.632907
Mutlu-Bayraktar, D., Cosgun, V., and Altan, T. (2019). Cognitive Load in Multimedia Learning Environments: A Systematic Review. Comput. Edu. 141, 103618. doi:10.1016/j.compedu.2019.103618
Naismith, L. M., Cheung, J. J., Ringsted, C., and Cavalcanti, R. B. (2015). Limitations of Subjective Cognitive Load Measures in Simulation-Based Procedural Training. Med. Educ. 49, 805–814. doi:10.1111/medu.12732
Nelson, T. O., and Narens, L. (1990). “Metamemory: A Theoretical Framework and New Findings,” in Psychology of Learning & Motivation. Editor G. H. Bower (Academic Press), 26, 125–173. doi:10.1016/s0079-7421(08)60053-5
Nelson, T. O., and Narens, L. (1994). “Why Investigate Metacognition? Metacognition: Knowing about Knowing. Editors J. Metcalfe, and A. Shimamura (MIT Press), 1–25.
Orru, G., and Longo, L. (2019). “The Evolution of Cognitive Load Theory and the Measurement of its Intrinsic, Extraneous and Germane Loads: A Review,” in Human Mental Workload: Models and Applications. Editors L. Longo, and M. C. Leva (Springer International Publishing), 23–48. doi:10.1007/978-3-030-14273-5_3
Ouwehand, K., Kroef, A. v. d., Wong, J., and Paas, F. (2021). Measuring Cognitive Load: Are There More Valid Alternatives to Likert Rating Scales? Front. Educ. 6, 370. doi:10.3389/feduc.2021.702616
Paas, F., Ayres, P., and Pachman, M. (2008). “Assessment of Cognitive Load in Multimedia Learning: Theory, Methods and Applications,” in Recent Innovations in Educational Technology that Facilitate Student Learning. Editors D. H. Robinson, and G. Schraw (Charlotte, NC: Information Age Publishing, Inc), 11–35.
Paas, F. G. W. C. (1992). Training Strategies for Attaining Transfer of Problem-Solving Skill in Statistics: A Cognitive-Load Approach. J. Educ. Psychol. 84 (4), 429–434. doi:10.1037/0022-0663.84.4.429
Paas, F. G. W. C., and Van Merriënboer, J. J. G. (1994). Instructional Control of Cognitive Load in the Training of Complex Cognitive Tasks. Educ. Psychol. Rev. 6 (4), 351–371. doi:10.1007/BF02213420
Prinz, A., Golke, S., and Wittwer, J. (2020). To what Extent Do Situation-Model-Approach Interventions Improve Relative Metacomprehension Accuracy? Meta-Analytic Insights. Educ. Psychol. Rev. 32 (4), 917–949. doi:10.1007/s10648-020-09558-6
Renkl, A., Hilbert, T., and Schworm, S. (2009). Example-Based Learning in Heuristic Domains: A Cognitive Load Theory Account. Educ. Psychol. Rev. 21 (1), 67–78. doi:10.1007/s10648-008-9093-4
Rey, G. D., Beege, M., Nebel, S., Wirzberger, M., Schmitt, T. H., and Schneider, S. (2019). A Meta-Analysis of the Segmenting Effect. Educ. Psychol. Rev. 31, 389–419. doi:10.1007/s10648-018-9456-4
Scheiter, K., Ackerman, R., and Hoogerheide, V. (2020). Looking at Mental Effort Appraisals through a Metacognitive Lens: Are They Biased? Educ. Psychol. Rev. 32 (4), 1003–1027. doi:10.1007/s10648-020-09555-9
Schleinschok, K., Eitel, A., and Scheiter, K. (2017). Do drawing Tasks Improve Monitoring and Control during Learning from Text? Learn. Instruction 51, 10–25. doi:10.1016/j.learninstruc.2017.02.002
Schmeck, A., Opfermann, M., van Gog, T., Paas, F., and Leutner, D. (2015). Measuring Cognitive Load with Subjective Rating Scales during Problem Solving: Differences between Immediate and Delayed Ratings. Instr. Sci. 43, 93–114. doi:10.1007/s11251-014-9328-3
Schnaubert, L., and Bodemer, D. (2017). Prompting and Visualising Monitoring Outcomes: Guiding Self-Regulatory Processes with Confidence Judgments. Learn. Instruction 49, 251–262. doi:10.1016/j.learninstruc.2017.03.004
Schneider, S., Beege, M., Nebel, S., and Rey, G. D. (2018). A Meta-Analysis of How Signaling Affects Learning with media. Educ. Res. Rev. 23, 1–24. doi:10.1016/j.edurev.2017.11.001
Schneider, S., Nebel, S., and Rey, G. D. (2016). Decorative Pictures and Emotional Design in Multimedia Learning. Learn. Instruction 44, 65–73. doi:10.1016/j.learninstruc.2016.03.002
Schraw, G. (2009). A Conceptual Analysis of Five Measures of Metacognitive Monitoring. Metacognition Learn. 4 (1), 33–45. doi:10.1007/s11409-008-9031-3
Schraw, G., Crippen, K. J., and Hartley, K. (2006). Promoting Self-Regulation in Science Education: Metacognition as Part of a Broader Perspective on Learning. Res. Sci. Educ. 36 (1–2), 111–139. doi:10.1007/s11165-005-3917-8
Schraw, G., Kuch, F., and Gutierrez, A. P. (2013). Measure for Measure: Calibrating Ten Commonly Used Calibration Scores. Learn. Instruction 24, 48–57. doi:10.1016/j.learninstruc.2012.08.007
Schroeder, N. L., and Cenkci, A. T. (2020). Do measures of Cognitive Load Explain the Spatial Split-Attention Principle in Multimedia Learning Environments? A Systematic Review. J. Educ. Psychol. 112, 254–270. doi:10.1037/edu0000372
Schroeder, N. L., and Cenkci, A. T. (2018). Spatial Contiguity and Spatial Split-Attention Effects in Multimedia Learning Environments: A Meta-Analysis. Educ. Psychol. Rev. 30, 679–701. doi:10.1007/s10648-018-9435-9
Seufert, T. (2020). Building Bridges between Self-Regulation and Cognitive Load-An Invitation for a Broad and Differentiated Attempt. Educ. Psychol. Rev. 32 (4), 1151–1162. doi:10.1007/s10648-020-09574-6
Son, L. K., and Metcalfe, J. (2000). Metacognitive and Control Strategies in Study-Time Allocation. J. Exp. Psychol. Learn. Mem. Cognlearning, Memory, Cogn. 26 (1), 204–221. doi:10.1037/0278-7393.1.20410.1037//0278-7393.26.1.204
Son, L. K., and Schwartz, B. L. (2002). “The Relation between Metacognitive Monitoring and Control,” in Applied Metacognition. Editors T. J. Perfect, and B. L. Schwartz (Cambridge University Press), 15–38. doi:10.1017/CBO9780511489976.003
Sweller, J. (1994). Cognitive Load Theory, Learning Difficulty, and Instructional Design. Learn. Instruction 4, 295–312. doi:10.1016/0959-4752(94)90003-5
Sweller, J., and Paas, F. (2017). Should Self-Regulated Learning Be Integrated with Cognitive Load Theory? A Commentary. Learn. Instruction 51, 85–89. doi:10.1016/j.learninstruc.2017.05.005
Thiede, K. W. (1999). The Importance of Monitoring and Self-Regulation during Multitrial Learning. Psychon. Bull. Rev. 6 (4), 662–667. doi:10.3758/BF03212976
Thiede, K. W., Anderson, M. C. M., and Therriault, D. (2003). Accuracy of Metacognitive Monitoring Affects Learning of Texts. J. Educ. Psychol. 95 (1), 66–73. doi:10.1037/0022-0663.95.1.66
Thiede, K. W.colleagues (2010). Poor Metacomprehension Accuracy as a Result of Inappropriate Cue Use. Discourse Process. 47 (4), 331–362. doi:10.1080/01638530902959927
Undorf, M., and Erdfelder, E. (2011). Judgments of Learning Reflect Encoding Fluency: Conclusive Evidence for the Ease-Of-Processing Hypothesis. J. Exp. Psychol. Learn. Mem. Cogn. 37 (5), 1264–1269. doi:10.1037/a0023719
van Gog, T., Hoogerheide, V., and van Harsel, M. (2020). The Role of Mental Effort in Fostering Self-Regulated Learning with Problem-Solving Tasks. Educ. Psychol. Rev. 32 (4), 1055–1072. doi:10.1007/s10648-020-09544-y
Wilde, G., Bätz, K., Kovaleva, A., and Urhahne, D. (2009). Überprüfung einer Kurzskala intrinsischer Motivation (KIM) [Testing a short scale of intrinsic motivation]. Z. Für Didaktik Der Naturwissenschaften 15 (15/2009), 31–45.
Wiley, J., Griffin, T. D., Jaeger, A. J., Jarosz, A. F., Cushen, P. J., and Thiede, K. W. (2016). Improving Metacomprehension Accuracy in an Undergraduate Course Context. J. Exp. Psychol. Appl. 22 (4), 393–405. doi:10.1037/xap0000096
Wiley, J., Griffin, T. D., and Thiede, K. W. (2005). Putting the Comprehension in Metacomprehension. J. Gen. Psychol. 132 (4), 408–428. doi:10.3200/GENP.132.4.408-428
Wiley, J., Griffin, T. D., and Thiede, K. W. (2008). To Understand Your Understanding You Must Understand what Understanding Means. Proc. Cogn. Sci. Soc. 30. Available at: http://csjarchive.cogsci.rpi.edu/Proceedings/2008/pdfs/p817.pdf.
Winne, P. H., and Hadwin, A. F. (1998). “Studying as Self-Regulated Learning,” in Metacognition in Educational Theory and Practice. Editors D. J. Hacker, J. Dunlosky, and A. C. Graesser (Lawrence Erlbaum), 277–304.
Xie, H., Wang, F., Hao, Y., Chen, J., An, J., Wang, Y., et al. (2017). The More Total Cognitive Load Is Reduced by Cues, the Better Retention and Transfer of Multimedia Learning: A Meta-Analysis and Two Meta-Regression Analyses. PLOS ONE 12 (8), e0183884. doi:10.1371/journal.pone.0183884
Keywords: metacomprehension judgments, multimedia learning, mental load, mental effort, cue utilization
Citation: Schnaubert L and Schneider S (2022) Analysing the Relationship Between Mental Load or Mental Effort and Metacomprehension Under Different Conditions of Multimedia Design. Front. Educ. 6:648319. doi: 10.3389/feduc.2021.648319
Received: 31 December 2020; Accepted: 10 December 2021;
Published: 10 January 2022.
Edited by:
Fred Paas, Erasmus University Rotterdam, NetherlandsReviewed by:
Tina Seufert, University of Ulm, GermanyLisette Wijnia, Open University of the Netherlands, Netherlands
Copyright © 2022 Schnaubert and Schneider. This is an open-access article distributed under the terms of the Creative Commons Attribution License (CC BY). The use, distribution or reproduction in other forums is permitted, provided the original author(s) and the copyright owner(s) are credited and that the original publication in this journal is cited, in accordance with accepted academic practice. No use, distribution or reproduction is permitted which does not comply with these terms.
*Correspondence: Lenka Schnaubert, bGVua2Euc2NobmF1YmVydEB1bmktZHVlLmRl