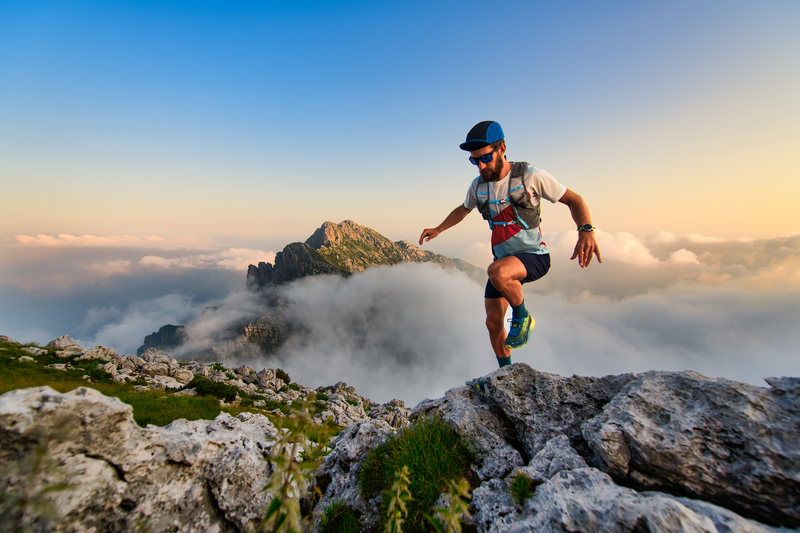
95% of researchers rate our articles as excellent or good
Learn more about the work of our research integrity team to safeguard the quality of each article we publish.
Find out more
ORIGINAL RESEARCH article
Front. Educ. , 28 April 2021
Sec. Educational Psychology
Volume 6 - 2021 | https://doi.org/10.3389/feduc.2021.645284
This article is part of the Research Topic Recent Approaches for Assessing Cognitive Load from a Validity Perspective View all 13 articles
In cognitive load theory (CLT), the role of different types of cognitive load is still under debate. Intrinsic cognitive load (ICL) and germane cognitive load (GCL) are assumed to be highly interlinked but provide different perspectives. While ICL mirrors the externally given task affordances which learners experience passively, germane resources are invested by the learner actively. Extraneous affordances (ECL) are also experienced passively. The distinction of passively experienced load and actively invested resources was inspired by an investigation where we found differential effects of a learning strategy training, which in fact resulted in reduced passive load and increased actively invested effort. This distinction is also mirrored in the active and passive forms for effort in German language: “es war anstrengend” (it has been strenuous) vs. “ich habe mich angestrengt” (I exerted myself). In two studies, we analyzed whether we could distinguish between these active and passive aspects of load by using these phrases and how this distinction relates to the three-partite concept of CLT. In two instructional design studies, we included the active and passive items into a differentiated cognitive load questionnaire. We found the factor structure to be stable, with the passive item loading on the ICL factor and the active item loading on the GCL factor. We conclude that it is possible to distinguish between active and passive aspects of load and that further research on this topic could be constructive, especially for learning tasks where learners act in a more self-regulated way and learner characteristics are taken into account.
During the last two decades, the concept of cognitive load has been widely discussed. The basic assumption that cognitive processes of schema construction require mental resources is noncontroversial. However, the aspects that contribute to these requirements while learning, and particularly, the role of different types of load, are still under debate. In its original account, cognitive load theory (CLT) differentiated between three different types of cognitive load (Sweller et al., 1998). Two of them are widely acknowledged: 1) intrinsic cognitive load (ICL) represents the complexity of the learning task itself, determined by the number of elements and their interrelations. Intrinsic load can only be altered by either changing the learning task or when learners can use their prior knowledge. Existing schemata would ease to relate the given elements and would thus reduce the intrinsic affordances. 2) Extraneous cognitive load (ECL) arises from an inappropriate design and poses load onto the learner, which is not task-relevant (e.g., search processes between different representations). This type of load was addressed in a vast amount of studies on instructional design (e.g., Paas et al., 2003). Particularly, in multimedia learning contexts, various design guidelines have been established which recommend design features which would reduce the extraneous affordances of the learning environment and thus reduce extraneous load (e.g., Mayer, 2014). The third type, that is, 3) germane cognitive load (GCL), is controversial. GCL arises when learners actively allocate resources to deal with the task, for example, to build cognitive schemata. In other words, learners use resources, which are germane while dealing with the intrinsically given the requirements of the task. Germane load therefore was also labeled as germane resources (Kalyuga, 2011). This description makes it obvious that intrinsic load and germane load are highly interlinked.
Nevertheless, if self-regulation is taken into account, that is, learners actively regulate their learning processes, ICL and GCL provide different perspectives. While ICL mirrors the externally given task affordances which learners experience rather passively, germane resources are invested by the learner actively (Seufert, 2018). The distinction of passively experienced load vs. actively invested resources could be an interesting approach to better understand the effects of how learners deal with or use their effort. This idea of different perspectives on cognitive load is actually not new and has already been reflected by Paas and van Merriënboer (1994). Besides the causal effects which determine cognitive load, they differentiate between different assessment factors which mirror the experienced load for each individual learner, namely, mental load, mental effort, and performance. The first two factors match the abovementioned distinction of actively vs. passively experienced load. While mental load reflects the task-centered dimension, which is determined externally by the task, mental effort is human-centered, and thus determined internally and dependent on learner’s decisions and characteristics. Not only the location of the determination is crucial but also the possibility to control these aspects. While mental load is not controllable and due to Paas and van Merriënboer “constant for a given task in a given environment,” mental effort is the “amount of controlled processing the individual is engaged in” (1994). Scheiter et al. (2020) referred to this distinction in their review on how to measure cognitive load and link it to an analog distinction which is made in the metacognitive literature. When learners rate their mental effort while metacognitively monitoring their learning process, they can also take these two perspectives as outlined by Koriat et al. (2006). When monitoring their effort, learners can on the one hand take into account the effort which is intrinsic to the task and thus externally triggered, which would be a data-driven appraisal of the experienced load in accordance with the concept of mental load. On the other hand, they could refer to their deliberately invested engagement based on their own goals, which is thus called a goal-driven appraisal of the experienced load in accordance with the concept of mental effort.
Not only contemporary approaches of linking research on cognitive load with research on self-regulation refer to the distinction of invested effort vs. experienced load (see also Schnaubert and Schneider, 2020) but also current research on measurement issues of cognitive load. Krell (2017), for example, developed a scale to measure mental effort and mental load. In a recent study, it could be shown that the distinction between load and effort could be even linked to objective measures of load, like the heart rate, which was only related to self-reported mental effort, but not to mental load (Minkley et al., 2021).
In the context of metacognitive monitoring, it becomes obvious that learners are in agency of their effort experience when it comes to effort appraisals, while they are not with load appraisals. This is what led us to the terms active and passive (or re-active as the activation is triggered externally) load. The use of the terms active and passive load—instead of mental effort and mental load—is especially motivated by the passive and active use of the word effort in German language: “es war anstrengend” (it has been strenuous) vs. “ich habe mich angestrengt” (I exerted myself). As there are already different items in use to measure mental effort and mental load (see, e.g., Paas, 1992; Krell, 2017), we refer to the name active and passive load as long as there is no comparison with the given items. The passive and active phrases of effort in the German language could be applied to measure the active and passive aspects of cognitive load, which we did in a study on the effects of learning strategies’ training for children (Taxis et al., 2010). We compared a group with training to a control group without training regarding their strategy use, learning outcomes, and cognitive load. As children usually have problems in understanding the item to measure load—“My mental effort was…”—we used the abovementioned German phrases in active and passive forms for measuring load. The training comprised step-by-step instructions on reading strategies and metacognitive strategies. Besides the expected effects of the training on strategy use and learning outcomes, we found evidence for differentiated effects of the two load items. While the children experienced a reduced passive load as the training provided explicit guidelines for learning, they reported an increase in their actively invested effort. This study provided a first hint that the activation of cognitive processes can enhance and reduce different aspects of cognitive load at the same time.
We started our discussion of different perspectives on cognitive load with the classical three-partite description of intrinsic, extraneous, and germane load. Thus, we now must ask how the two-fold perspective of active and passive load would align to these three load types. As argued above, ICL and GCL are closely connected as they refer to the same affordances given by a task. While ICL is data-driven, externally determined, and thus passively experienced, GCL is goal-driven, internally determined, and thus actively invested. But how about extraneous affordances of a task (ECL), like intricate navigation in an online-learning environment or other unnecessary, that is, task-irrelevant search processes? As they are also determined externally and are usually not under control of the learner, we would expect them to also relate to passive aspects of cognitive load. However, it seems also plausible that learners may also manage this kind of extraneous load by adapting their strategies, and thus are no longer passive. This self-management of load is described in an article of Eitel et al. (2020), who also link the concept of cognitive load with self-regulation in learning. They describe that learners can enhance their effort in selecting relevant aspects when confronted with irrelevant seductive details.
Based on Kane’s (2013) argument-based approach for validity, we formulate the following interpretation/use argument (IUA): We plan to measure cognitive load after learning, using an item on active as well as on passive load to assess learners’ perceived invested effort and experienced load during learning: all for the purposes of discerning the effects differences in learning settings or through learner characteristics. This IUA results in different inferences (scoring, generalization, and extrapolation) that are described in the following paragraphs and will be discussed in the end.
In order to analyze the relations between the classical three-partite load types and active and passive load, we refer to well-researched design principles or instructional design effects. With their specific theoretical assumptions on how they would affect cognitive load and performance, we can deduce specific assumptions on the three classical types of cognitive load. This leads to a scoring inference which will be operationalized as hypotheses below: If active load is associated with GCL and passive load with ICL and eventually also with ECL, then the effects on all those measures should be in accordance. As we aim at analyzing the active and passive aspects of load, we use established variations of tasks, their design, and learners’ aptitudes to elicit ICL, ECL, and GCL as a starting point. If we are using already established multimedia principles within classical experimental designs—as typical for studies on cognitive load—as well as often examined learner characteristics, then this enables us to assume some generalization inference for the found results. Given that if, for example, we can find an effect in one worked-example study, then we are hopefully able to find it in another, as there is already broad evidence for the worked-example effect.
To elicit intrinsic load effects, one could alter the complexity of the task (element interactivity, e.g., Sweller et al., 1998). Alternatively, one could use the learner-based variation of existing schemata, which would also affect the perceived ICL. Learners with higher expertise would rate a complex task less intrinsically loading than a learner with low expertise (Artino, 2008). Therefore, a scoring inference is as follows: Whenever ICL increases, the measure of passive load should also increase. Moreover, if ICL increases in a way that it exceeds learners’ working memory capacity, this should also be indicated by a decrease in learning performance.
For inducing effects on ECL, well-researched design principles could be used, like the multimedia principle, the modality principle, the split-attention principle, the worked-example principle, or the redundance principle (for an overview, see Mayer, 2014). The split-attention principle, which is also called the spatial contiguity principle, states that instructional material which needs to be integrated should be placed nearby or even integrated, like words printed into a picture instead of separately in a text (Ayres and Sweller, 2014). Thus, learners do not need extraneous resources for searching for corresponding elements, and with the freed-up resources, learners can engage in mental integration which would be germane to the task. The same ECL-reducing effect can be assumed when implementing worked examples (for an overview, see Renkl, 2014). Learners are provided with a problem and an additional example on how to solve this problem before starting to solve the same or comparable problems on their own. With the guidance of the worked-example, learners do not need to spend extraneous resources on inadequate problem-solving strategies (Van Gog et al., 2008) and improve their learning outcomes. Van Gog et al. (2008) also argue that with the freed-up resources, learners could then invest more mental effort which would be germane to the task. Thus, worked examples may trigger both, a decreased ECL and an increased GCL. Such interdependences of the three load types have been also examined by Park (2010) in her study program on the additivity assumption of load. She could demonstrate that the enhancing effects of different instructions for learning or cognitive load cannot simply be added but that they might interact. This complex interplay has also been revealed by Wirzberger et al. (2016). So, the next scoring assumption is as follows: If ECL decreases by matters of design, then the score on the passive item should also decrease.
Regarding GCL, there are also several techniques proposed, which intend to foster generative activities. Moreno and Mayer (2010) analyzed the effects of personalization: guided activities like prompts to elaborate on the learning content, feedback, or self-explanation prompts. Their review provides strong evidence for improved learning outcomes when these techniques were used. But can these techniques enhance germane cognitive load? In their review on the effects of journal writing for learning, Nückles et al. (2020) provided evidence for a germane load–enhancing effect but they also emphasized that such an enhancing effect would depend on the learner’s ability to apply the requested generative activity. This is also confirmed in a study of Park et al. (2016), where prompting learners to mentally animate a complex scenario only led to higher mental effort when learners had the necessary spatial abilities to perform these mental animations. Despite triggering generative activities and thus germane load externally, one could also analyze whether learners with task-specific abilities and particularly with strategic skills would invest more germane resources. Thus, the impulse to invest germane resources would be elicited internally. However, none of these studies used a specific measure for germane load but only deduced their interpretation by combining measures of mental effort with learning outcome measures. Only in two studies reported by Klepsch and Seufert (2020), effects of germane load–inducing instructions were revealed by using the germane cognitive load scale of the differentiated questionnaire by Klepsch et al. (2017). This leads to the assumption that if GCL increases, then the scores on the active load item should also increase, which gives us another scoring inference.
Overall, there are several instructional means with which the different types of load can be elicited. Besides the theoretical arguments, there are also empirical studies confirming these effects, but only a few measured the effects with differentiated measures of load (e.g., Klepsch and Seufert, 2020).
The goal of the present studies is to investigate the concept of passive load, that is, experiencing load and active load, that is, investing effort in relation to the classical three-partite types of load based on CLT. From a theoretical point of view, one would assume that the passively experienced load should be linked to those aspects of load that come along with the task affordances and its presentation, that is, ICL and ECL. The active load on the other hand would link to the actively invested germane resources. The purpose of the studies is to examine whether this theoretically assumed mapping of active and passive load with the concepts of intrinsic, extraneous, and germane load can be substantiated empirically. In addition, we use a simple measure for active and passive load by using the German active and passive linguistic forms of experiencing load. With such an easy-to-apply—and even for younger kids easy-to-understand—measure, the concepts of active and passive load could be investigated easily instead of or in addition to other differentiated measures (e.g.,Leppink et al., 2013; Klepsch et al., 2017; Krell, 2017). If the scores on the active and the passive load item are given, then the scores of other cognitive load measurements could be predicted, as well as learning outcome, which would allow an extrapolation inference.
In two experimental studies, we address the following questions: 1) Can we distinguish between these active and passive aspects of load and 2) relates this distinction to the three-partite concept of CLT. With classical instructional design variations, we analyze 3) the prognostic validity of these measures based on the abovementioned theories and studies on how to elicit the different types of load.
In the first study, we used worked examples in comparison to problem-solving as an external factor to elicit extraneous load and combined it with self-explanation prompts as an external factor to elicit germane load. In the second study, we used a split-source format in comparison to an integrated format to externally elicit extraneous load and learners’ cognitive style to either process information in a holistic or serialistic way, which can elicit germane processes internally. We did not vary ICL as a between-subjects factor but considered learners’ prior knowledge as an internal variation of ICL in both studies. In both studies, learners must rate their perceived intrinsic, extraneous, and germane load with the differentiated questionnaire for cognitive load by Klepsch et al. (2017) and their active and passive load with the additional items “ich habe mich angestrengt” (I exerted myself) and “es war anstrengend” (it was strenuous). With a factor analysis and additional correlations, the structural interrelation between the two lines of concepts is analyzed. Moreover, the expected main effects and interactions for the instructional variations were analyzed for validating the respective measures. Regarding the abovementioned research questions, we have the following hypotheses.
(H1) We expect that making an effort (active) in an explorative factor analysis is loading on the GCL factor and that experiencing load (passive) is loading either on the ICL or ECL factor.
(H2) We expect to find correlations between making an effort (active) and GCL and between experiencing load (passive) and ICL and/or ECL.
(H3) In terms of a validation of both measures, we expect making an effort (active) and germane cognitive load to increase when generative learning activities are triggered by the design (H3_active). We expect ECL to decrease when the design is optimized regarding unnecessary processes, and we expect no group differences for ICL, as we do not vary complexity of the learning content in our studies. Experiencing load (passive) should be either more in line with ICL or ECL (H3_passive). Additionally, learning outcome between groups will differ (H3_learning_outcome).
To examine these hypotheses, we conducted two studies, where we analyzed in which way the concept of active and passive load, measured by the active and passive German forms of effort (Supplementary Appendix A), relates to the three types of cognitive load measured by a differentiated measurement instrument (Supplementary Appendix A; Klepsch et al., 2017).
In order to elicit different levels of different aspects of cognitive load, study 1 used the worked-example effect and the prompting principle. Learners had to deal with two mathematical topics, that is, extremum problems and Taylor polynomial tasks.
Based on theoretical assumptions and empirical findings, we assume for study 1 effects of worked examples and prompts on ICL, ECL, GCL, and the passive and the active item. While worked examples are meant to reduce the perceived ECL, prompts should enhance GCL. As we did not change the content to be learned, there should not be any difference in perceived ICL. The assumptions for the active and passive load should correspond with the effects for either ICL and/or ECL (passive) or for GCL (active). We additionally analyze the effects on learning outcomes of both instructional variations.
In Table 1, the experimental conditions of study 1 and number of participants in these conditions are listed.
We collected data from 73 learners. Participants were at average 22.34 (SD = 4.27) years old, and 13.70% were male. They were students from a German university.
We conducted a 2 × 2 between-subject study with four experimental conditions (Table 1). Independent variables are based on the worked-example effect (problem-solving vs. worked examples) and the prompting principle (no prompts vs. self-explanation prompts). As dependent variables, we assessed cognitive load in a differentiated way, the active and passive parts of load and learning outcome. As control variables, age, sex, and prior knowledge have been assessed.
At the beginning, learners were informed about the procedure of the study they participated in and signed an informed consent form. All participants were aware that they could withdraw their data at any point in the study without having any disadvantage. Then, each participant was randomly assigned to one of the experimental groups. As a next step, each participant filled out a questionnaire asking for demographic data. Then, prior knowledge was assessed (described in Material). Afterward, participants had to deal with the learning material either with or without worked examples (described in Material). If they were in one of the experimental conditions with self-explanation prompts, learners then were presented the prompts (described in Material). After learning, the cognitive load questionnaire (Supplementary Appendix A; Klepsch et al., 2017) had to be answered, as well as the items to assess the active and passive parts of load (Supplementary Appendix A). In the end, learning outcome was measured; for each topic, two tasks which are structurally similar to those in the learning material and one transfer task with an unknown structure had to be solved.
Each learner had to deal with two types of mathematical learning material: 1) extremum problems and 2) Taylor polynomial tasks. Prior knowledge was assessed after participants received an example task for each type of material: in two questions, they had to answer on a 7-point Likert-type scale, whether they were familiar with such tasks, and in a second step, they had to write down how they would solve the task. Afterward, the learning phase started with a short introduction on the topic, followed by two example tasks. To realize differences in ECL as an independent variable, problem-solving or worked examples were included. In the problem-solving group, the correct solution was presented, but no information on how the solution could be calculated was given. In the worked-example group, the solution steps for calculating the correct answer for the example tasks were provided. Participants had 5 min time for each domain to learn the content and another 15 min to conduct the post test. Performance was measured by a posttest for each domain containing two analog tasks (similar in structure to the example tasks in the learning material), and one complex transfer task with an unknown problem structure. For each task, six points could be reached, and therefore, as a maximum, 36 points could be reached in the posttest. Task performance was calculated by summing up points given for correct answers.
The self-explanation prompts were presented after working through the learning material. We asked participants to reflect what is new for them in the learning material. They should write down in own words how they would solve problems like those presented in the learning material and reflect if there is something, they do not yet understand.
Cognitive load was assessed through a differentiated questionnaire (Supplementary Appendix A; Klepsch et al., 2017). For the active and passive aspects of load, two items (Supplementary Appendix A) were used: “es war anstrengend” (“It has been strenuous,” passive) vs. “ich habe mich angestrengt” (“I exerted myself,” active).
For all three scales of the differentiated questionnaire, reliability was estimated by using McDonald’s ω. A principal component analysis (PCA) was conducted to provide evidence that the items are forming the intended factors. Then, the items on the active and passive aspects of load were included into the factor analysis, to show that the active items fit into the GCL factor and the passive item fits into the ICL or ECL factor. Then, correlations were conducted to again provide evidence if especially the passive item correlates with load resulting from the material (ICL and/or ECL), and the active item correlates with the GCL scale.
Additionally, we analyzed group differences regarding the control variables, prior knowledge, and age to check for differences despite the randomization. Also, correlations between the control variables and the dependent variables have been calculated. Whenever a significant difference between groups or a significant correlation could be found, the affected variable was included in the following analyses of variance as a covariate. To identify group differences, AN(C)OVAS were conducted with ICL, ECL, GCL, the active or the passive item, or learning outcomes as dependent variables.
We used McDonalds ω to assess the reliability of the three scales of the differentiated cognitive load questionnaire. For ICL and GCL, two items, and for ECL, three items were included, and we could find sufficient levels of McDonalds ω (ICL: 0.80, ECL: 0.85, and GCL: 0.77).
The principal component analysis (PCA) was conducted with the items of the differentiated questionnaire with orthogonal rotation (varimax). The Kaiser–Meyer–Olkin measure verified the sampling adequacy for the analysis (KMO = 0.59). Barlett’s test of sphericity (χ2 (21) = 206.34, p < 0.001) indicated that correlations between items were sufficiently large for PCA. An initial analysis was run to obtain eigenvalues for each component in the data. Three components had eigenvalues over Kaiser’s criterion of 1 and in combination explained 81.76% of the variance. Table 2 (a) shows the factor loadings after rotation. The items that cluster on the same components suggest that component 1 represents ECL, component 2 ICL, and component 3 GCL.
TABLE 2. Rotated PCA (a) with items from Klepsch et al. (2017) and (b) including the passive and active items for study 1.
Including the active and passive items into the PCA resulted in the following outcome: The Kaiser–Meyer–Olkin measure verified the sampling adequacy for the analysis (KMO = 0.64). Barlett’s test of sphericity (χ2 (36) = 349.47, p < 0.001) indicated that correlations between items were sufficiently large for PCA. An initial analysis was run to obtain eigenvalues for each component in the data. Three components had eigenvalues over Kaiser’s criterion of 1 and in combination explained 78.48% of the variance. Table 2 (b) shows the factor loadings after rotation. The items that cluster on the same components suggest that component 1 represents ICL including the passive item, component 2 ECL, and component 3 GCL including the active item.
We found significant relationships between the active item and the original two-item GCL scale (r = 0.77, p (one-tailed) < 0.001) as well as the original two-item ICL scale (r = 0.21, p (one-tailed) = 0.03), and between the passive item and the original two-item ICL scale (r = 0.63, p (one-tailed) <. 001) as well as the original three-item ECL scale (r = 0.44, p (one-tailed) < 0.001). All correlations can be found in Supplementary Appendix B.
Concerning the control variables, we could not find any group differences for the variables age (F < 1, n.s.) and prior knowledge (F (1,69) = 2.47, p = 0.12, η2 = 0.04). Correlations between these variables and dependent variables showed that age is significantly correlated with learning outcome (r = −0.41, p < 0.001) and prior knowledge is significantly correlated with learning outcome (r = 0.32, p < 0.01), ECL (r = −0.24, p = 0.04), and the passive item (r = −0.29, p = 0.01), and were thus integrated as covariates in the respective analyses. All correlations can be found in Supplementary Appendix C.
All means and standard deviations of the dependent variables can be found in Table 3, and effects are visualized in Figure 1.
FIGURE 1. Effects on the dependent variables for study 1. Note: ps = problem-solving, we = worked examples; * (simple) main effect with p ≤ 0.05.
For ICL, we found no significant main effect of worked examples (F < 1, n.s.) or prompts (F < 1, n.s.), neither an interaction (F (1,69) = 1.39, p = 0.24, η2 = 0.02): ICL was reported to be equal in the experimental groups.
For ECL, we found a significant main effect of worked examples (F (1,69) = 13.57, p < 0.001, η2 = 0.17), no main effect of prompts (F > 1, n.s.), and no interaction (F > 1, n.s.): ECL was reported to be lower in the groups with worked examples.
For GCL, we found no main effect of worked examples (F (1,69) = 1.99, p = 0.16, η2 = 0.03), a significant main effect of prompts (F (1,69) = 6.26, p = 0.02, η2 = 0.08), and no interaction (F > 1, n.s.): GCL was reported to be higher in the groups without prompts.
For the passive item, we found no main effect of worked examples (F < 1, n.s.), no main effect of prompts (F (1,68) = 1.58, p = 0.21, η2 = 0.02), but an interaction (F (1,68) = 4.49, p = 0.04, η2 = 0.06): Simple main effects show that in the worked-example group, there is a significant difference if a learner gets prompts or gets no prompts (F (1,68) = 6.25, p = 0.02, η2 = 0.08), with getting a prompt resulting in higher passive load. Thus, this result pattern does not fully correspond to those of either ICL or ECL.
For the active item, we found no significant main effect of worked examples (F < 1, n.s.) or prompts (F < 1, n.s.), neither an interaction (F < 1, n.s.): The active item was reported to be equal in the experimental groups. Thus, this result pattern does also not fully correspond to that of GCL.
For learning outcome, we found a significant main effect of worked examples (F (1,67) = 10.137, p < 0.01, η2 = 0.13), no main effect of prompts (F (1,67) = 1.38, p = 0.25, η2 = 0.02), and no interaction (F (1,67) = 2.63, p = 0.11, η2 = 0.04): Learning outcome was higher, when worked examples were given.
Regarding the relation of the active and passive load measures with the three classical types of cognitive load, the PCA revealed that the active item is loading on the GCL factor and the passive item is loading on the ICL factor (H1). We also found the expected correlations between the active item and the original two-item GCL scale and between the passive-item and the original two-item ICL scale (H2). These results will be discussed more in depth in the general discussion.
Regarding the validation of the different load measures in the different instructional settings, we could not find any effects on ICL, which we expected, as we did not change the learning content between conditions. But we found a main effect of worked examples on ECL. In H3, we assumed that the passive item would correspond with either the ICL or the ECL scale, but the passive item is not aligned with the patterns for either ICL or ECL. Instead, we found a significant interaction effect, which is defined by the difference of learners with or without prompts in the worked-example group: Learners report higher passive load when provided with a prompt. The combination of worked examples and prompts seems to result in additional affordances that are necessary to link the worked examples with the prompts, which is in fact an alteration and increase in task affordances, that is, more interactive elements to deal with. The increase of passive load reported by this group thus indicates in our view that the passive item would be more closely linked to the concept of ICL than to ECL (H3_passive).
In this first study, we used prompts to elicit GCL, but the GCL items and the active item did not reveal corresponding results: We assumed a main effect of prompting on GCL and active load, which we could only find on GCL but not on active load. However, the main effect of prompts on GCL revealed unexpected results with higher levels of GCL for learners without prompts. This may be explained with the fact that they deliberately invested more mental effort as a human-centered dimension, as they have not been guided with hints. Assuming that self-regulation also results in cognitive load (Seufert, 2018), giving prompts might have reduced the amount of needed self-regulation, and therefore can result in lower GCL. These arguments would be in line with seeing the missing prompts as a desirable difficulty (Bjork, 2017). However, as the learning outcomes did not increase for learners without prompts, we rather appraise missing prompts as a difficulty, which the participants in our study were willing to compensate with increased germane load. In the worked-example condition where they were relieved from additional load, this investment paid off, and learning outcomes were comparable to those of learners with prompts. For the active load measure, we could not find such effects.
To complete, we could find an effect of worked examples on learning outcome, which was better when worked examples were provided (H3_learning_outcome). For learning outcome, we could neither find an effect of prompts nor an interaction of worked examples and prompts, which was unexpected, but is in line with the found results on GCL and the active load item, as already mentioned, and will be discussed in the general discussion.
As already mentioned, study 2 deals with the split-attention effect and Pask’s learning styles (Pask, 1976). Learners had to deal with a biological topic about the structure and functions of the human kidney.
Based on theoretical assumptions and empirical findings, we assume effects of split-attention and learning style on ICL, ECL, GCL, and passive load and active load for study 2. While the integrated format in contrast to the split format should reduce the perceived ECL, learners’ style of processing learning material in an either serialistic or holistic way should enhance GCL when it matches the presentation format. Learners with a holistic learning style tend to grasp the overall picture of the learning content and to integrate the most relevant parts while serialistic learners focus on specific details without trying to integrate them into an overall picture (Pask, 1976). Thus, no main effect but an interaction effect is hypothesized with more germane load for holistic learners in the integrated format, which helps them to get an overview, and for serialistic learners in the split format, which helps them to concentrate on details. As we did not change the learning content, there should not be any difference in perceived ICL. The assumptions for the active and passive load should correspond with the effects for either ICL and/or ECL (passive) or for GCL (active). We again analyzed the effects on learning outcomes: We assume that serialists reach higher levels of learning outcome when provided with the split-source material, whereas holists reach higher levels of learning outcome when provided with the integrated material
In Table 4, the experimental conditions of study 2 and the number of participants in these conditions are listed.
We collected data from 72 learners. Participants were at average 22.99 (SD =3.98) years old, and 16.70 % were male. They were students from a German university.
We conducted a 2 × 2 between-subject aptitude–treatment interaction study with four experimental conditions. Independent variables are related to the split-attention principle (split-source format vs. integrated format) and participants’ learning style (serialist vs. holist). As dependent variables, we assessed cognitive load in a differentiated way, the active and passive parts of load and learning outcome. As control variables, age, sex, and prior knowledge have been assessed.
As in study 1, at the beginning, learners were informed about the procedure of the study they participated in and signed an informed consent form. All participants were aware that they could withdraw their data at any point in the study without having any disadvantage. As a next step, each participant filled out a questionnaire asking for demographic data. Then, they filled out the questionnaire for assessing their learning type (described in Material). Also, in this study, prior knowledge was assessed (described in Material). Afterward, participants had to deal with the learning material either with a split-source format or an integrated format (described in Material), which was randomly assigned. After learning, the cognitive load questionnaire (Supplementary Appendix A; Klepsch et al., 2017) had to be answered, as well as the items to assess the active and passive parts of load (Supplementary Appendix A). In the end, the knowledge test had to be answered (described in Material).
Prior knowledge about the human kidney was assessed through 10 open questions, covering content of the following learning material. The learning material itself was about the structure and functions of the kidney. It consisted of four pictures and seven corresponding texts. As a dependent variable, ECL was varied through either split-source material or integrated material. In the split-source material, the text was on the left side of the paper and the four pictures on the right side. References connecting the pictures with the text were included through corresponding numbers in the text and the pictures. In the integrated format, the connections between the four pictures were made clear by integrating the four pictures into one overall picture, giving the frame with the four detailed pictures like a zoom-in in different parts of the kidney. In addition, each text was placed near the corresponding part in the pictures. The aim of this approach was to minimize search processes between text and pictures. Each participant had 30 min to learn the content. To assess learning performance, a posttest had to be filled in consisting of 13 tasks. Altogether, 35 points could be reached.
Pask’s learning styles (Pask, 1976) were assessed with the questionnaire of Ford (1985). Based on the calculated value, learners were either categorized as serialists or holists.
Cognitive load was again assessed with a differentiated questionnaire (Supplementary Appendix A; Klepsch et al., 2017). For the active and passive aspects of load, the same two items (Supplementary Appendix A) were used as in study 1, namely, “es war anstrengend” (“It has been strenuous,” passive) vs. “ich habe mich angestrengt” (“I exerted myself,” active).
For all three scales of the differentiated questionnaire, reliability was estimated by using McDonald’s ω. A principal component analysis (PCA) was conducted to provide evidence that the items are forming the intended factors. Then, the items on the active and passive aspects of load were included into the factor analysis, to show that the active items fit into the GCL factor and the passive item fits into the ICL or ECL factor. Additionally, correlation analysis was conducted to provide evidence again if especially the passive item correlates with load resulting from the material (ICL and/or ECL), and the active item correlates with the GCL scale.
Again, group differences were analyzed regarding the control variables such as prior knowledge and age. Also, correlations between these variables and the dependent variables have been calculated. Whenever a significant difference between groups or a significant correlation could be found, the affected variable was included in the following analyses of variance as a covariate. To identify group differences, AN(C)OVAS were conducted with ICL, ECL, GCL, passive load or active load, and learning outcome as dependent variables.
We used McDonalds ω to assess the reliability of the three scales of the differentiated cognitive load questionnaire. For ICL and GCL, two items, and for ECL, three items were included, and we could find satisfying levels of McDonalds ω (ICL: 0.72, ECL: 0.75, and GCL: 0.76).
The PCA was conducted with the items of the differentiated questionnaire with orthogonal rotation (varimax). The Kaiser–Meyer–Olkin measure verified the sampling adequacy for the analysis (KMO = 0.63). Barlett’s test of sphericity (χ2 (21) = 157.54, p < 0.001) indicated that correlations between items were sufficiently large for PCA. An initial analysis was run to obtain eigenvalues for each component in the data. Three components had eigenvalues over Kaiser’s criterion of 1 and in combination explained 78.71% of the variance. Table 5 (a) shows the factor loadings after rotation. The items that cluster on the same components suggest that component 1 represents ECL, component 2 GCL, and component 3 ICL.
TABLE 5. Rotated PCA (a) with items from Klepsch et al. (2017) and (b) including the passive and active items for study 2.
Including the active and passive items into the PCA resulted in the following outcome: The Kaiser–Meyer–Olkin measure verified the sampling adequacy for the analysis (KMO = 0.70). Barlett’s test of sphericity (χ2 (36) = 258.17, p < 0.001) indicated that correlations between items were sufficiently large for PCA. An initial analysis was run to obtain eigenvalues for each component in the data. Three components had eigenvalues over Kaiser’s criterion of 1 and in combination explained 75.21% of the variance. Table 5 shows the factor loadings after rotation. The items that cluster on the same components suggest that component 1 represents ECL, component 2 GCL including the active item, and component 3 ICL including the passive item.
We found significant relationships between the active item and the original two-item GCL scale (r = 0.59, p (one-tailed) < 0.001) as well as the original two-item ICL scale (r = 0.23, p (one-tailed) = 0.03), and between the passive item and the original two-item ICL scale (r = 0.60, p (one-tailed) < 0.001) and the original three-item ECL scale (r = 0.27, p (one-tailed) = 0.01). All correlations can be found in Supplementary Appendix B.
Concerning the control variables, we could not find any group differences for the variables such as age (F < 1, n.s.) and prior knowledge (F (1,68) = 1.14, p = 0.29, η2 = 0.02). Correlations between these variables and dependent variables showed that prior knowledge was significantly correlated with learning outcome (r = 0.40, p < 0.01), ICL (r = −0.26, p = 0.03) and the passive item (r = −0.25, p = 0.04), and therefore included as a covariate in the respective analyses. All correlations can be found in Supplementary Appendix C.
All means and standard deviations of the dependent variables can be found in Table 6, and effects are visualized in Figure 2.
FIGURE 2. Effects on the dependent variables for study 2. Note: ssm = split-source material, im = integrated material; * (simple) main effect with p ≤ 0.05.
For ICL, we found no main effect of learning style (F < 1, n.s.) or material (F < 1, n.s.), neither an interaction (F < 1, n.s.): ICL was reported to be equal in the experimental groups.
For ECL, we found no main effect of learning style (F < 1, n.s.), a significant main effect of material (F (1,68) = 5.55, p = 0.02, η2 = 0.08), and no interaction (F > 1, n.s.): ECL was reported to be higher with split-source material than integrated material.
For GCL, we found no main effect of learning style (F < 1, n.s.), or material (F (1,68) = 3.34, p = 0.07, η2 = 0.05), but a significant interaction (F (1,68) = 5.82, p = 0.02, η2 = 0.05): Simple main effects showed that with split-source material, serialists reported higher GCL than holists (F (1,68) = 4.28, p = 0.04, η2 = 0.06), and holists reported higher GCL with integrated material than split-source material (F (1,68) = 7.89, p < 0.01, η2 = 0.10).
For the passive item, we found no main effect of learning style (F < 1, n.s.), material (F < 1, n.s.), or interaction (F (1,68) = 2.89, p = 0.09, η2= 0.04): The responses to the passive item were equal in the experimental groups. Thus, this result pattern corresponds to that of ICL.
For the active item, we found no main effect of learning style (F (1,68) = 3.50, p = 0.07, η2 = 0.05), or material (F < 1, n.s.), but a significant interaction (F (1,68) = 4.89, p = 0.03, η2 = 0.07): Simple main effects showed that with split-source material, serialists reported higher active investment than holists (F (1,68) = 8.39, p < 0.01, η2 = 0.11), and holists reported higher GCL with integrated material than split-source material (F (1,68) = 3.91, p = 0.05, η2 = 0.05). Thus, this result pattern corresponds to that of GCL.
For the overall learning outcome, we found no main effect of learning style (F (1,67) = 1.34, p = 0.25, η2 = 0.02) or of material (F < 1, .s.), but the expected significant interaction (F (1,67) = 4.86, p = 0.03, η2 = 0.07): Simple main effects showed that with split-source material, serialists reached higher levels of overall learning outcome than holists (F (1,67) = 5.79, p = 0.02, η2 = 0.08).
Also, for study 2 with the PCA, we could show that the active item is loading on the GCL factor and the passive item is loading on the ICL factor (H1). We also found the expected correlations between the active item and the original two-item GCL scale and between the passive item and the original two-item ICL scale (H2). These results will be discussed more in depth in the general discussion.
The results of the second study show evidence for H3: We found the same effects on GCL and active load, where we categorized learners based on their learning style. A significant interaction of learner’s aptitude and the treatment shows that learners with different prerequisites benefit from different treatments. This effect could be found for the GCL scale as well as for the active item (H3_active).
In line with our hypotheses, we could not find any effects, neither of the independent variables or their interaction with ICL. For the ECL measure, however, we were able to find a main effect of split attention. For the passive item, we cloud not find any main effects and no interaction, which indicates that the passive item is rather related to ICL and not to ECL (H3_passive).
The goal of the present studies was to investigate the concept of passive load, that is, experiencing load, and active load, that is, investing effort, in relation to the classical three-partite types of load based on CLT. Therefore, in a first step, we discuss the results of the two studies based on hypotheses and address the question if reported active and passive load can be connected to reported ICL, ECL, and GCL measured with the differentiated questionnaire of Klepsch et al. (2017). Next, strengths and weaknesses of the studies as well as of the concept of active and passive load in general are discussed, and further directions are addressed. Finally, we discuss the usefulness of the concepts of active and passive load, especially the theoretical and practical implications, and the need for further research. The stated inferences (scoring, generalizations, and extrapolation) will also be discussed to provide broader evidence for validity.
Based on our findings, we conclude that it is possible to distinguish between active and passive aspects of load and that the concept can be related to the three types of cognitive load, ICL, ECL, and GCL measured with the differentiated questionnaire by Klepsch et al. (2017).
We could find sufficient evidence to underline our first two hypotheses (H1 and H2) and therefore also for the scoring inference that active load is associated strongly with GCL and passive load strongly with ICL and weakly with ECL. First, we could show that in both studies, PCA of the items for the three original load types results in the same factor structure as reported by Klepsch et al. (2017). Thus, reliability of the scales is given, which is a necessary but not sufficient condition for validity. Including the active and passive items into the PCA showed that the active item extends the GCL scale and the passive item extends the ICL scale. This was the case in both studies. In a second step, the correlation analysis showed that in both studies, the active item has a moderate-to-strong positive relationship with the GCL scale and that the passive item has a moderate-to-strong positive relationship with the ICL scale. Thus, we can state that these links between the active item with GCL and the passive item with ICL are rather stable and replicable in two independent studies. However, the active item also correlated weakly with ICL in both studies, which underpins the idea that ICL and GCL might be highly interlinked (Kalyuga, 2011). But as the strengths of the correlations highly differ—the active item is much stronger related to GCL than to ICL—we can state that active investment is more clearly linked to germane load. However, learners can and must only invest additional effort if the complexity of the task is sufficiently high, that is, when ICL is high. The role of ECL is not that clearly linked to the active–passive construct. We found a moderate correlation of the passive item with ECL in both studies. Thus, the extraneous affordances do play a role for learners' appraisal of passive load, but in our studies, it was less considerable than ICL. However, whether this would also be the case in settings where ECL is remarkably higher overall and would be thus more striking and disrupting, the impact of ECL for passive load appraisals might increase in relation to ICL.
Overall, we can state that experienced load can be differentiated with the two items for measuring active and passive load. This distinction becomes important as soon as learners act in a self-regulated way and decide to invest effort in processing learning content.
In the third hypothesis, we assumed to find corresponding result patterns for the active load item and GCL whenever generative learning activities are triggered by the design (i.e., all measures should increase) (H3_active), and also corresponding result patterns for the passive load item with ICL or ECL (H3_passive).
In study 2, we could show that generative learning activities result in differences in GCL and the active load item, but we could not show the same pattern for study 1.
But why did learners rate the active load item in accordance with the GCL items in the second study but not in the first? Perhaps prompts, which aims at triggering germane load externally, and internally available task-specific abilities—like strategy skills to process information in a holistic or serialistic way—have different effects on perceived active investment. Applying internally available abilities in learning settings that matches these abilities, for example, providing holists with integrated material, is directly experienced as active investment. Externally triggered investment, in our case prompting, might not be experienced as one’s own active investment. Whether this argument is generalizable has to be proven in future studies with measures of active load in situations with more successful triggers for generative activities. Nevertheless, the role of learners’ aptitudes in interaction with different instructional settings as an internal trigger of germane investment seems to be promising and should be analyzed in a study with a direct comparison to an externally triggered germane investment. With reference to the postulated scoring inference on GCL (H3_active; where we stated that if GCL increases, then also the scores on the active load items should increase), we can state that we could find evidence for that.
At least one of the stated scoring inferences on ICL and ECL cannot be assured, as we could not find a stable link between ECL and the passive item. In contrast, in both studies, we could show that the passive item is more in line with ICL (H3_passive). But we are not able to fully substantiate this, as our studies do not include variations in ICL. Therefore, at the moment, we can only state that we have found a promising link between ICL and the passive item through PCA and the conducted correlation analyses. Based on the given design of the studies, we are not able to provide evidence whether rated passive load would increase if ICL of the task increases. This should be investigated in further studies.
Overall, interesting to mention is that all the design effects, which are reflected in ECL, cannot be found for either the passive or active load measure. To complete the picture of the instructional design effects, we found the expected effect of worked examples with better learning outcomes when worked examples were provided. Unfortunately, we could not confirm positive effects on learning outcomes when prompts were provided. As we already discussed, this might be due to the additional perceived passive load when prompts and worked examples were given because learners might have felt overwhelmed in self-explaining. For the split-attention effect in the second study, we found the expected interaction with learners’ style to deal with either holistic (integrated) or serially (split) presented material. Overall, the implemented design effects can be largely seen as valid means to trigger the expected effects. Thus, these results substantiate the validity of the result patterns of the load measures and the correspondence of the passive load measure with the ICL scale and the active load measure with the GCL scale.
Overall, we can state that both learning materials in the two studies have been rather complex and difficult. This is also reflected in learning outcome. In study 1, learners did hardly reach two-thirds of the points, whereas in study 2, most learners did not even reach half of the points in the posttest. In study 2, the internal complexity is also reflected in reported high ICL and passive load. In study 1, reported ICL and passive load are not that pronounced, but still high. Also, important to mention, both studies are classical experimental studies conducted with students at a German university, and both include many more female than male participants, which were fortunately randomized equal over experimental groups. Thus, despite the evidence of the studies which allows some generalization, the generalization inference is not fully proven yet. Results should be replicated with broader and more balanced samples. Moreover, the samples in both studies have been rather small. We had at least 15 participants in each experimental condition, but we are aware that this results in power restrictions. Therefore, it would be of interest to conduct studies with larger samples and in a more realistic learning setting, for example, in school. Using a school setting would additionally allow to analyze whether younger kids and teens would be able to understand the items for active and passive load, as we would assume, which would even provide more proof of generalization.
The concept of active and passive load comes with some positive but also some negative aspects that should be considered. On the one hand, active and passive load in their operationalization should be easy to understand even for younger kids and teens. They can be answered rather quickly, and therefore can be used for repeated online measures of cognitive load during a learning phase, without interfering with learning. On the other hand, we could not find any evidence that design aspects in the learning material are reflected in passive or active load. Therefore, these items seem not to be useful if one is especially interested in differences in the design effects of learning materials. However, as soon as learner characteristics are considered, the concept of active and passive load seems to become important, which is addressed in the next section.
As already mentioned, ICL mirrors the externally given task affordances, and GCL can be seen as the actively invested resources by the learner (Seufert, 2018). Our results show that this can be linked to passive and active load. Passive load shows in study 2 a similar pattern like ICL. In study 1, the overall pattern is also similar, but we found a simple main effect of prompts in the worked-example group. As the prompt was new for the learners, it might have resulted in passive rather than active load—as intended by us—because the prompt belongs to the task-centered dimension (Paas and van Merriënboer, 1994) or can be seen as data-driven (Scheiter et al., 2020). Further studies should have a closer look at prompts and other active load–enhancing techniques, like asking learners to use special learning strategies. Based on the presented results, we would assume that all these active load–enhancing techniques at the beginning result in more passive load and over time—when learners are used to the techniques and can use them more automatically—the techniques should result in higher active load instead of passive load (see also mathemathantic effect; Clarck, 1990). Whether prompts for generative activities can be fruitful for learners and are thus experienced as active and germane rather than hindering and passive, highly depends on learners’ prerequisite. Learners’ prior knowledge or their strategy skills should be taken into account (see, e.g., Nückles et al., 2020; Seufert, 2020). Study 2 also provides evidence that learner characteristics, which are internally given (even per se or through training) in interaction with the instructional design, can result in differences in active load. Only when the external affordances match the internal abilities germane resources are invested, that is, learners report germane load as well as active load investment. In our case, in study 2, split-source material would fit more to learners with a serialistic approach of learning, whereas integrated material would fit more to learners with a more holistic approach of learning. The fact that this fit is also crucial for being successful with this engagement can be seen with the same effects mirrored in learning outcomes. In future studies, the complex interplay of learner characteristics, tasks affordances, and design aspects should be addressed.
As already mentioned, the two items for active and passive load can be easily used to assess load online, by asking learners repeatedly during learning to rate their perceived active and passive load. This would be particularly relevant when intrinsic load is changing over time, for example, when learning content gets more complex with each step, a learner moves forward or when learners gain expertise. Especially an observation over longer periods of time, when, for example, a training on learning strategies is implemented, would be of interest to provide evidence if the use of a new learning strategy shifts from passively experienced load to active investment as soon as the strategy is internalized and therefore more easily used automatically.
Generally, the two items that measure for active and passive load could be used to either substitute or extend other measures of cognitive load. It would be particularly interesting to analyze the items in combination with other differentiated measures of cognitive load by Leppink et al. (2013) or by Krell (2017) to substantiate its validity. Doing so would also help to provide more evidence for the stated extrapolation inference to get evidence if one measure can predict another and especially if learning outcomes in further tasks could be predicted. In the two studies, we analyzed the relations between the active and passive items and the cognitive load questionnaire of Klepsch et al. (2017). If measurement methods of cognitive load have predictive validity, it would be of interest to have a closer look on different methods of measurement and their mutual prediction of each other. Also, the extrapolation of learning outcome should be surveyed more closely. Using the items repeatedly during a study could, for example, be used to predict if the next task will be performed correctly or with errors.
We found interesting evidence for the concept of active and passive load and its connection to the classic three-partite concept of cognitive load. However, there is still research needed to investigate active and passive load more in depth. Based on the interplay between the two approaches, the active–passive aspects and the original three load types, further studies should be conducted and analyzed, to discuss the concept of active and passive load on a theoretical level. This is especially necessary when considering learner’s active role in self-regulatory learning (Seufert, 2018; Seufert, 2020) and trying to connect the concept of active and passive load with analogous concepts like the human- or task-centered dimension (Paas and van Merriënboer, 1994) or the concept of data-driven and goal-driven appraisals of cognitive load as discussed at the crossroad between CLT and self-regulated learning research (De Bruin et al., 2020).
The raw data supporting the conclusions of this article will be made available by the authors, without undue reservation.
Ethical review and approval was not required for the studies on human participants in accordance with the local legislation, institutional requirements and due to the recommendations of the German Research Association: No subject was in risk of physical or emotional pressure, we fully informed all subjects in all studies about the goals and processes of the study they participated in, and none of the subjects were patients, minors, or persons with disabilities. In all studies, participation was voluntary, and all subjects signed a written informed consent and were aware that they had the chance to withdraw their data at any point of the study.
MK and TS contributed to the conception and design of the studies. MK developed the used material and questionnaires and led data collection for studies. MK analyzed and interpreted the data. TS and MK drafted the work. All authors provided approval of the final submitted version of the manuscript and agreed to be accountable for all aspects of the work by ensuring that questions related to the accuracy or integrity of any part of the work were appropriately investigated and resolved.
The authors declare that the research was conducted in the absence of any commercial or financial relationships that could be construed as a potential conflict of interest.
We like to thank our former students involved in one of the studies. They contributed to this research as part of their curriculum, in form of a bachelor or master thesis, or as student research assistants.
The Supplementary Material for this article can be found online at: https://www.frontiersin.org/articles/10.3389/feduc.2021.645284/full#supplementary-material.
Artino, A. R., (2008). Cognitive load theory and the role of learner experience. An abbreviated review for educational practitioners. AACE J. 16, 425–439.
Ayres, P., and Sweller, J. (2014). in “The split-attention principle in multimedia learning,” in the Cambridge handbook of multimedia learning. Editor R. E. Mayer. Second edn (New York, NY: Cambridge University Press), 206–226.
Bjork, R. A. (2017). “Creating desirable difficulties to enhance learning,” in In progress. Editors I. Wallace, L. Kirkman, and L. Vergne (Bethel, CT: Crown House Publishing)), 81–89.
Clarck, R. E. (1990). “When teaching kills learning: research on mathemathantics,” in analysis of complex skills and complex knowledge domains: learning and instruction,” in European research in an international context. Editors H. Mandl, E. De Corte, N. Bennett, and H. F. Friedrich (Oxford, UK: Pergamon Press), 1–22.
De Bruin, A. B. H., Roelle, J., Roelle, J., Carpenter, S. K., and Baars, M. (2020). Synthesizing cognitive load and self-regulation theory: a theoretical framework and research agenda. Educ. Psychol. Rev. 32, 903–915. doi:10.1007/s10648-020-09576-4
Eitel, A., Endres, T., and Renkl, A. (2020). Self-management as a bridge between cognitive load and self-regulated learning: the illustrative case of seductive details. Educ. Psychol. Rev. 32, 1073–1087. doi:10.1007/s10648-020-09559-5
Ford, N. (1985). Learning styles and strategies of postgraduate students. Br. J. Educ. Technol. 16, 65–77. doi:10.1111/j.1467-8535.1985.tb00483.x
Kalyuga, S. (2011). Cognitive load theory: how many types of load does it really need? Educ. Psychol. Rev. 23, 1–19. doi:10.1007/s10648-010-9150-7
Kane, M. T. (2013). Validating the interpretations and uses of test scores. J. Educ. Meas. 50, 1–73. doi:10.1111/jedm.12000
Klepsch, M., Schmitz, F., and Seufert, T. (2017). Development and validation of two instruments measuring intrinsic, extraneous, and germane cognitive load. Front. Psychol. 8, 1997. doi:10.3389/fpsyg.2017.01997
Klepsch, M., and Seufert, T. (2020). Understanding instructional design effects by differentiated measurement of intrinsic, extraneous, and germane cognitive load. Instr. Sci. 48, 45–77. doi:10.1007/s11251-020-09502-9
Koriat, A., Ma’ayan, H., and Nussinson, R. (2006). The intricate relationships between monitoring and control in metacognition: lessons for the cause-and-effect relation between subjective experience and behavior. J. Exp. Psychol. Gen. 135, 36–69. doi:10.1037/0096-3445.135.1.36
Krell, M. (2017). Evaluating an instrument to measure mental load and mental effort considering different sources of validity evidence. Cogent Edu. 4, 1280256. doi:10.1080/2331186X.2017.1280256
Leppink, J., Paas, F., van der Vleuten, C. P. M., van Gog, T., and Van Merriënboer, J. J. G. (2013). Development of an instrument for measuring different types of cognitive load. Behav. Res. 45, 1058–1072. doi:10.3758/s13428-013-0334-1
Moreno, R., and Mayer, R. E. (2010). “Techniques That Increase Generative Processing in Multimedia Learning: Open Questions for Cognitive Load Research,” in Cognitive Load Theory. Editors J. L. Plass, R. Moreno, and R. Brünken (Cambridge, New York:Cambridge University Press), 153–177.
Minkley, N., Xu, K. M., and Krell, M. (2021). Analyzing Relationships Between Causal and Assessment Factors of Cognitive Load: Associations Between Objective and Subjective Measures of Cognitive Load, Stress, Interest, and Self-Concept. Front. Educ. 6, 632907. doi:10.3389/feduc.2021.632907
Minkley, N., Xu, K., and Krell, M. (2021). Analyzing relationships between causal and assessment factors of cognitive load: associations between objective and subjective measures of cognitive load, stress, interest, and self-concept. Front. Educ. doi:10.3389/feduc.2021.632907
Nückles, M., Roelle, J., Glogger-Frey, I., Waldeyer, J., and Renkl, A. (2020). The self-regulation-view in writing-to-learn: using journal writing to optimize cognitive load in self-regulated learning. Educ. Psychol. Rev. 32, 1089–1126. doi:10.1007/s10648-020-09541-1
Paas, F. G. W. C. (1992). Training strategies for attaining transfer of problem-solving skill in statistics: a cognitive-load approach. J. Educ. Psychol. 84, 429–434. doi:10.1037/0022-0663.84.4.429
Paas, F. G. W. C., and Van Merriënboer, J. J. G. (1994). Instructional control of cognitive load in the training of complex cognitive tasks. Educ. Psychol. Rev. 6, 351–371. doi:10.1007/BF02213420
Paas, F., Renkl, A., and Sweller, J. (2003). Cognitive load theory and instructional design: recent developments. Educ. Psychol. 38, 1–4. doi:10.1207/S15326985EP3801_1
Park, B., Münzer, S., Seufert, T., and Brünken, R. (2016). The role of spatial ability when fostering mental animation in multimedia learning: an ATI-study. Comput. Hum. Behav. 64, 497–506. doi:10.1016/j.chb.2016.07.022
Park, B. (2010). “Testing the additivity hypothesis of cognitive load theory,”. M.S Dissertation, Saarbrücken, (Germany): Universität Des Saarlandes.
Pask, G. (1976). Styles and strategies of learning. Br. J. Educ. Psychol. 46, 128–148. doi:10.1111/j.2044-8279.1976.tb02305.x
R. E. Mayer (Editors) (2014). The Cambridge handbook of multimedia learning. Second edition (New York, NY: Cambridge University Press).
Renkl, A. (2014). “The worked examples principle in multimedia learning,”in the Cambridge handbook of multimedia learning. Editor R. E. Mayer. Second edn (New York, NY: Cambridge University Press), 391–412.
Scheiter, K., Ackerman, R., and Hoogerheide, V. (2020). Looking at mental effort appraisals through a metacognitive lens: are they biased? Educ. Psychol. Rev. 32, 1003–1027. doi:10.1007/s10648-020-09555-9
Schnaubert, L., and Schneider, S. (2020). How learners use mental load and mental effort as indicators for metacomprehension under different load-inducing conditions of multimedia design.
Seufert, T. (2018). The interplay between self-regulation in learning and cognitive load. Educ. Res. Rev. 24, 116–129. doi:10.1016/j.edurev.2018.03.004
Seufert, T. (2020). Building bridges between self-regulation and cognitive load-an invitation for a broad and differentiated attempt. Educ. Psychol. Rev. 32, 1151–1162. doi:10.1007/s10648-020-09574-6
Sweller, J., Van Merriënboer, J. J. G., and Paas, F. G. W. C. (1998). Cognitive architecture and instructional design. Educ. Psychol. Rev. 10, 251–296. doi:10.1023/a:1022193728205
Taxis, S.-S., Gutmann, C., Herzmann, P., and Seufert, T. (2010). “Effects of a learning strategy training for children,” in “Instructional Design for motivated and competent learning in a digital world”: program Book. Editors M. Hopp, and F. Wagner, (Ulm, Germany: Ulm University), 44–46.
Van Gog, T., Paas, F., and Van Merriënboer, J. J. G. (2008). Effects of studying sequences of process-oriented and product-oriented worked examples on troubleshooting transfer efficiency. Learn. Instr. 18, 211–222. doi:10.1016/j.learninstruc.2007.03.003
Keywords: cognitive load theory (CLT), differentiated measurement, self-regulated learning (SRL), assessing cognitive load, active vs. passive cognitive load, measurement, validity
Citation: Klepsch M and Seufert T (2021) Making an Effort Versus Experiencing Load. Front. Educ. 6:645284. doi: 10.3389/feduc.2021.645284
Received: 22 December 2020; Accepted: 11 February 2021;
Published: 28 April 2021.
Edited by:
Fred Paas, Erasmus University Rotterdam, NetherlandsReviewed by:
Slava Kalyuga, University of New South Wales, AustraliaCopyright © 2021 Klepsch and Seufert. This is an open-access article distributed under the terms of the Creative Commons Attribution License (CC BY). The use, distribution or reproduction in other forums is permitted, provided the original author(s) and the copyright owner(s) are credited and that the original publication in this journal is cited, in accordance with accepted academic practice. No use, distribution or reproduction is permitted which does not comply with these terms.
*Correspondence: Melina Klepsch, bWVsaW5hLmtsZXBzY2hAdW5pLXVsbS5kZQ==
Disclaimer: All claims expressed in this article are solely those of the authors and do not necessarily represent those of their affiliated organizations, or those of the publisher, the editors and the reviewers. Any product that may be evaluated in this article or claim that may be made by its manufacturer is not guaranteed or endorsed by the publisher.
Research integrity at Frontiers
Learn more about the work of our research integrity team to safeguard the quality of each article we publish.