- Department of Educational Psychology, Baylor University, Waco, TX, United States
Person-centered methodologies generally refer to those that take unobserved heterogeneity of populations into account. The use of person-centered methodologies has proliferated, which is likely due to a number of factors, such as methodological advances coupled with increased personal computing power and ease of software use. Using latent class analysis and its extension for longitudinal data, [latent transition analysis (LTA)], multiple underlying, homogeneous subgroups can be inferred from a set of categorical and/or continuous observed variables within a large heterogeneous data set. Such analyses allow researchers to statistically treat members of different subgroups separately, which may provide researchers with more power to detect effects of interest and closer alignment between statistical modeling and one’s guiding theory. For many educational and psychological settings, the hierarchical structure of organizational data must also be taken into account; for example, students (i.e., level-1 units) are nested within teacher/schools (i.e., level-2 units). Finally, multilevel LTA can be used to estimate the number of latent classes in each structured unit and the potential movement, or transitions, participants make between latent classes across time. The transitions/stability between latent classes across time can be treated as the outcome in and of itself, or the transitions/stability can be used as a correlate or predictor of some other, distal outcome. The purpose of the paper is to discuss multilevel LTA, provide considerations for its use, and demonstrate variance decomposition, which requires numerous steps. The variance decomposition steps are presented didactically along with a worked example based on analysis from the Social Rating Scale of ECLS-K.
1 Introduction
Efforts to classify individual cases into homogeneous groups have long been used in order to better understand complex sets of information. Classification of cases into homogeneous groups has important implications in the social sciences, such as education, medicine, psychology, or economics, where identifying smaller subsets of like cases may be of particular interest. Person-centered methodologies generally refer to those that take unobserved heterogeneity of populations into account. That is, rather than treat all individuals as if they originated from a single underlying population, as is true with variable-centered methodologies, person-centered methodologies allow for multiple subpopulations to underlie a set of data. The challenge with these methods is identifying the correct number (i.e., frequency) of subpopulations, or classes, and the parameters (i.e., form) associated with each, when the frequency and form are not known a priori (Nylund et al., 2007; Tofighi and Enders, 2008; Morgan, 2015).
Mixture modeling, generally, refers to the family of statistical procedures for identifying homogeneous subpopulations of cases from one large, heterogeneous data set (McLachlan and Peel, 2004; Collins and Lanza, 2009). The analysis assumes that an observed dataset is a mixture of observations collected from a finite number of mutually exclusive classes, each with its own characteristics. These procedures have been referred to in the literature under many different names, such as mixture likelihood approach to clustering (McLachlan and Basford, 1988; Everitt, 1993) and model-based clustering (Banfield and Raftery, 1993). Depending on the metric level of the variables included in the study, other terms used to describe this methodology are latent class analysis, latent profiles analysis, latent class clustering, or model-based clustering.
Many advances have occurred in mixture modeling as an analytic methodology, which now includes models like factor mixture, growth mixture, diagnostic classification, and latent Markov models. Moreover, mixture modeling is now being applied in fields ranging from education to brain imaging and geosciences to robotics. Despite the proliferation of models and applications that fall within the mixture modeling framework, there are still new areas and angles to explore and better understand in order to more fully realize the strengths of this analytic framework. One such area where limited research has been disseminated involves nested data structures that are collected longitudinally. That is, multilevel mixture models are available to researchers although they have not been discussed as extensively as other cross-sectional and longitudinal mixture models. Asparouhov and Muthén (2008) and Kaplan et al. (2011) presented findings from applications of this type of model, but additional guidance on the use of these models may help users better understand their data structures and, ultimately, make better decisions about their research questions. One important consideration when using these models is the ability of the researcher to understand the magnitude and sources of effects through the decomposition of variance. This is especially true in models, such as the ones we present in the next section, that have nested data structure collected across time.
This is precisely the purpose of this paper. That is, we seek to 1) present and discuss multilevel latent transition analysis, 2) describe considerations for the use of this model, and 3) demonstrate a multi-step variance decomposition. The variance decomposition steps are presented didactically along with a worked example based on an analysis from the Social Rating Scale (SRS) of Early Childhood Longitudinal Survey - Kindergarten (ECLS-K). Several additional notes are important due to the didactic nature of this paper. First, the latent class analysis and latent profile analysis differ on the basis of the metric level of the indicator variables, yet these are conceptually similar analyses. Latent categorical variables are often referred to as latent classes regardless of the metric level of the indicator variables. As such, there are instances where we use “class” and “profile” interchangeably. For this paper, we are modeling continuous indicators so the term “latent profile” is most precise, but the discussion and procedures we present apply to model with categorical and/or continuous indicators. Second, we demonstrate the procedures for variance decomposition with a two-class model for didactic reasons; therefore, any substantive conclusions about the specific variables or participants used in the example should be avoided. Third, we used the Grades 3 and 5 SRS scores from restricted-use ECLS-K 1998 datafile; the scores for Grades 3 and 5 were respectively collected in Spring 2002 and Spring 2004.
2 Introduction to Latent Transition Analysis
When using latent class analysis and its extension for longitudinal data, [latent transition analysis (LTA)], multiple underlying, homogeneous subgroups can be inferred from a set of categorical and/or continuous observed variables within a large heterogeneous data set. Such analyses allow researchers to statistically treat members of different subgroups separately, which may provide researchers with more power to detect effects of interest and closer alignment between statistical modeling and one’s guiding theory.
In latent class analysis (LCA), membership in one of the underlying populations is conceptualized as a latent, categorical variable that is not directly observed. Instead, latent class membership must be measured using two or more observed, or indicator, variables, taken as a manifestation of latent variables. The number of latent profiles underlying a dataset is not known a priori, and thus, has to be uncovered (Collins and Lanza, 2009). The process typically involves fitting models that specify different numbers of profiles in order to determine which model best approximates the heterogeneous set of data. Each case is assigned a probability of belonging to each profile based on the alignment between the characteristics (e.g., response probabilities, means, variances, covariances) between each case and each profile. When the characteristics of a case are similar to those of a given profile, the case has a high probability of being a member of the subpopulation. When the characteristics of a case are dissimilar to those for a stated profile, the case has a low probability of belonging to the profile. Generally, cases are assigned to the profile to which they have the highest probability of belonging, which is called modal assignment (Collins and Lanza, 2009). Ideally, the classification probability for each person will be high for one and only one profile. An optimal solution will have high classification probabilities for each latent class, illustrating that the classes are distinct.
The procedures described above can be applied to cross-sectional data or data collected at multiple points in time. LTA, the longitudinal extension of LCA, allows the stability of an LCA solution to be examined across time. Furthermore, LTA allows researchers to examine transition patterns among latent classes across time using one of several strategies. The first strategy is to regress latent class membership at time
The modeling strategy chosen has important implications on the structure of the latent transition matrix, which contains probabilities of transitioning to another latent class conditioned on latent class membership at 1) time 1 if only two waves of data collection occurred or 2)
2.1 Multilevel Latent Transition Analysis for Longitudinal Nested Data
Although LTA accounts for the collection of data from the same individuals across time (i.e., time nested within person), the model can also be extended to account for individuals being nested within higher level units, such as schools, hospitals, organizations, etc. In education research, statistical methods are commonly used that model students nested within schools, which is the context for the illustration in this paper. In such cases, the hierarchical structure of organizational data must be taken into account because independence between observations is not tenable; that is, students (i.e., level-1 units) are nested within and share influence of schools (i.e., level-2 units). Thus, multilevel LTA can be used to estimate the number of latent classes in each structured unit and the transitions participants make between classes across time. Finally, the transitions/stability between latent classes across time can be treated as the outcome in and of itself, or the transitions/stability can be used as a correlate or predictor of some other, distal outcome.
The multilevel LTA can be expressed as a series of multinomial logistic regressions at level-1 and as a linear regression at level-2 (Asparouhov and Muthén, 2008). To illustrate, consider the model below that has two latent classes across two time points. At level-1 the multinomial logistic regression for the latent classification variable at time 1,
where
where
The multinomial autoregression model above is akin to what is used in single-level LTA; however, a unique contribution of multilevel LTA is the incorporation of a latent regression model of latent class intercepts over time. At level-2, the random effects of latent class size across schools can be explained as part of a series of latent linear regression models such as
The regression in Eq. 3 models the difference in latent class size across schools at time 1 where
The multilevel LTA model has many parameters to describe the process which generated the differences in observed characteristics across time, and some of the model features are directly interpretable whereas other features are less easily interpreted. In order to help explain the complex features of the model, various
where
Asparouhov and Muthén (2008) used these other
One potential limitation of the
2.2 Considerations for Using Multilevel Latent Transition Analysis
There are several considerations specific to multilevel LTA that extend beyond those associated with LCA and LTA. First, one must consider whether the research question posed requires the multilevel aspect of the data explicitly incorporated into a mutlilevel LTA model. Not all questions require that the nested nature of the data be explicitly modeled (McNeish et al., 2017). For example, a researcher primarily interested in transitions of students among latent classes over time may not need to explicitly account for a school effect if differences among schools does not influence the students’ transitions. Instead, the multilevel aspect of the data can be incorporated implicitly through the use of sampling weights (Stapleton, 2013) or alternatives such as cluster-robust standard errors (McNeish et al., 2017). However, the use of multilevel LTA is likely warranted when researchers believe that characteristics of the group or school are related to differences in latent class membership. This is commonly encountered in education and healthcare applications where between-school and between-hospital differences, respectively, influence large groups of participants simultaneously.
In additional to the nested feature of one´s data, another important consideration is the time scale in which data were collected. The time scale of data collection may, or may not, adhere time scale of the transitions that individual may experience. Collins and Lanza (2009, p. 209–211) expressed how the transition structure estimated may reveal only chance transitions due to a underlying structure that transitions very rapidly (e.g., the example of indicators of depression in the last week but data were collected one year apart). Therefore, researchers must think carefully about how observed transitions among latent classes are related to transitions in the underlying construct of interest. In multilevel LTA, in particular, an additional consideration is whether the time scale of the transition is equal across level-2 units, such as schools. In healthcare settings, for example, the time scale of transitioning among depression latent classes may depend in part on the care received across different clinics if clinics were to have a general approach to helping patients with, say, depressive symptoms. As noted above, these considerations should be applied in addition to those important considerations that have been identified for LCA and LTA, such as model selection (Nylund et al., 2007; Tofighi and Enders, 2008; Morgan, 2015), label switching (Chung et al., 2004; Tueller et al., 2011), nature of the latent variables (Lubke and Neale, 2008), and incorporation of distal outcome (Lanza et al., 2013; Bakk and Vermunt, 2016; Nylund-Gibson et al., 2019). An excellent collection of applied and methodological papers using these procedures can be found on the Mplus website (www.statmodel.com/paper.shtml).
Next, we illustrate the use of multilevel LTA and explicitly model the multilevel nature of the data.
3 Application of Multilevel Latent Transition Analysis
3.1 Sample
The data used are a subset of the ECSL-K national dataset (Tourangeau et al., 2009). The analytic sample for this demonstration was approximately 7,080 students nested within approximately 1,100 schools (sample sizes have been rounded to the nearest 10 in compliance with federal restricted-use data reporting guidelines). Prior to estimating the multilevel LTA model, we subset the ECLS-K data file on the students who remained in the same school from at least Grade 3 to Grade 5. The average number of students per school was 6.4 (
3.2 Instrumentation
In order to demonstrate the model output and subsequent decomposition of the model variance, we used the five Social Rating Scale subscales from the Early Childhood Longitudinal Survey–Kindergarten (ECLS-K) data. The five major constructs of interests are: Approaches to Learning (AtL), Self-Control (SC), Interpersonal Skills (IPS), Externalizing Problem Behaviors (EPB), and Internalizing Problem Behaviors (IPB) (Tourangeau et al., 2009). These five constructs of child behaviors/characteristics are modeled as being reflective of a child’s need for possible additional behavioral intervention. The reliability estimates (coefficient α) for these constructs in the full ECLS-K in spring of fifth grade ranged from 0.77 (Internalizing Problem Behaviors) to 0.91 (Approaches to Learning). Reading teachers were asked to report how frequently students exhibited the social skill or behavior identified by each item. The response scale used a four-point frequency scale ranging from 1 (Never) to 4 (Very Often). The same 26 SRS items administered in Grade 3 and 5. A summary of these raw subscale scores is shown in Table 1.
The raw subscale scores were computed as the average of the responses to the items on each subscale.
3.3 Procedures
The model was estimated using maximum likelihood estimator with robust standard errors (MLR) in Mplus v8.4 (L. Muthén and Muthén, 2017) using 2,000 random starting values and 50 final stage optimizations. For illustrative purposes, we estimated only a two-class solution. In practice, additional class enumeration models would be estimated and compared. For this demonstration, we elected to not use sampling weights to reduce the complexity of the example analysis. All inferences from the following model are restricted to this sample of students and is not necessarily a representation of the characteristics of students more broadly.
The path diagram for the multilevel LTA model is presented in Figure 1. We should note that the path diagram includes variance components to aid in interpretation of variance decomposition discussion below.
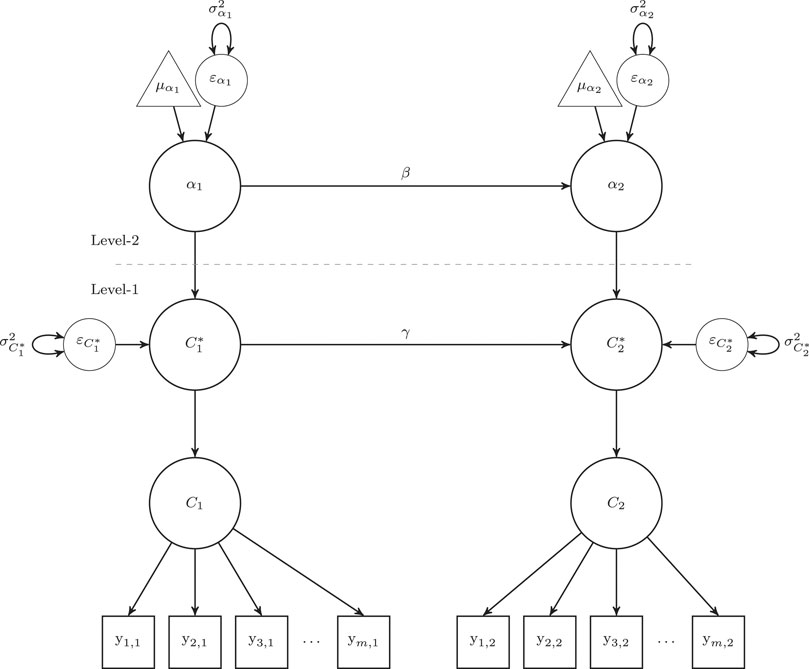
FIGURE 1. Path diagram of a multilevel latent transition model proposed for ECLS-K Social Rating Scale over two waves. Note. Subscripts indicate the timepoint of the data. AtL = Approaches to Learning; SC = Self-control; IPS = Interpersonal Skills; EPB = Externalizing Problem Behaviors; IPB = Internalizing Problem Behaviors;
The major inferential goals are the evaluation of the transition parameters (
3.4 Results
The resulting latent class patterns are shown in Table 2. In the estimation, the latent class structure was fixed to be invariant across time. Latent class 1 is characterized by students who had lower ratings on the three positive constructs (i.e., AtL, SC, and IPS) and higher scores on the constructs reflecting problem behaviors (i.e., EPB and IPB). Latent class 2 was characterized as having higher scores on the three positive constructs (i.e., AtL, SC, and IPS) and lower ratings on the problem behavior constructs (i.e., EPB and IPB).
The structural model parameters are described in Table 3. At Time 1, Class 1 was the smaller of the two latent classes, making up about 32% of the sample, whereas Class 2 made up about 68% of the sample. Due to the multilevel nature of the data, the parameter estimate,
The transition component of the multilevel LTA model is characterized by the parameters γ (
As alluded to above, there are numerous calculations necessary to extract important modeling results that guide interpretation. The intraclass correlation (ICC) estimate for this model indicates that about 16% proportion of variability in class assignment at Time 1 can be explained by the school effect. Using the variance decomposition, 10.4% of the variability in latent class membership at Time 2 can be accounted for by the school effect at Time 1. However,15.8% of the variability in latent class membership at Time 2 can be accounted for by the school effect at Time 2. The incorporation of school level covariates into Eq. 3 and Eq. 4 could give insight into what school characteristics are associated with latent class membership at Time 2 by investigating the change in the previous two
4 Multilevel Latent Transition Analysis Variance Decomposition
Clearly, as indicated above, examining the proportion of variability that can be attributed to each component of the model can aid in interpreting the model effects. Although the parameter estimates provides some indication of the magnitude of model effects, the scale can make them difficult interpret. Furthermore, it is customary in traditional regression to report the proportion of variability explained by the model, and in multilevel models reporting the proportion of variability that is attributable to higher- and/or lower-level units can greatly inform inferences about the magnitude of effects of those units on the outcome(s) of interest. In this didactic model, for example, the estimated regression weight for the effect that latent class membership in Grade 3 had on latent class membership in Grade 5, controlling for school-level effects, was 2.93. Is this effect small, moderate, or large? It is difficult to make such a determination with the effect on this metric. Decomposing the variance and reporting the effect as a percentage makes the effect much easier to interpret. That is, the proportion of variability of
Due to the didactic nature of this paper, we refrain from commenting on any substantive conclusions regarding the size of this effect; rather, we seek to demonstrate how the variance decomposition produces a more intuitive, or at least familiar, effect size estimate. That said, certain
4.1 Variance Component Derivations
To derive the variance components, the structural equations associated with the path diagram are needed. The structural equation are:
The variances associated with these structural component are defined as follows. The variance of
The remaining pieces are slightly more complex. For the variance of the random effect at time 2, a long form derivation is
It should be noted that we assumed that the covariance between the time 1 random effect and the time 2 random effect is 0.
The variance of the latent response tendency variable relative to the reference class 2 is defined as follows.
Again, we assumed that the error terms between the random effect at level 2 and the latent response tendency variable residual variance for the logistic regression have a covariance of 0. The residual variance of the latent response residual variance is a known constant of
Lastly, the variance of the latent response tendency variable for time 2 is defined as
Again, the assumption of a covariance of 0 among the terms is imposed. The unique part of obtaining the variance of the latent response variable at time 2 is that an indicator function is a part of the structural equation. An indicator function,
To summarize, the variance components are
4.2 Compute -Like Measures
The
The ICC above will be a useful component to disentangle the variance of the latent response tendency variable at time 1. The
The estimate of the proportion of variance in latent class membership at time 2 (
The estimate of the proportion of variance in latent class membership at time 2 (
The estimate of the proportion of variance in (
The estimate of the proportion of variance in
The proportion of variance in
Lastly, the proportion of variance in
It should be noted that similar decomposition is possible for higher number of latent classes at each time point. However, the decomposition is more involved given the complexity of more transitions and random effects at level-2. Methods for expanding the results described above to k-class solutions are built on ideas similar to the random effects models for multinomial outcomes (Hedeker, 2003). We are currently developing the extension to three latent classes and intend to identify some concise patterns that will allow for relatively straightforward variance decomposition with more latent classes.
5 Conclusion
In this paper, we have described multilevel latent transition analysis as an approach to investigating heterogeneous, nested data. This model has only recently seen increased use in psychological and educational research, but its use is still rather scarce. Asparouhov and Muthén (2008) introduced the multilevel LTA model more than a decade ago and have made recent contributions with LTA models that incorporate random intercepts (Muthén and Asparouhov, 2020). Thus, advances are being made with models and parameterizations to accommodate more complex data structures, nested longitudinal data from multiple underlying subpopulations (i.e., mixtures). When considering alternative models, the choice of modeling approach should, of course, be determined by one’s guiding theoretical expectation(s) about the variables of interest. That said, models are also useful to the extent that they are interpretable. As noted, analysis of one’s data using multilevel LTA can also help researchers classify individual cases into homogeneous groups in order to better understand complex sets of information. The use of classification of cases into homogeneous groups is important in the social sciences where identifying smaller subsets of like cases may be of particular interest. In presenting multilevel LTA, our goal was to increase researchers’ knowledge and confidence in using these models because nested data are ubiquitous in many educational and psychological research settings.
In order for this goal to be realized, the mechanics of the model and effect size estimation must be transparent. We believe this paper has served an important role in this respect because reporting the results in terms of proportions of variance explained by the various parts in the model is consistent with regression analysis, including multilevel modeling, and thus more familiar to a broader research audience. The contribution of this detailed decomposition of the variance components gives researchers another dimension for interpreting the results from multilevel LTA. The decomposition shown here also adds to the limited research of nested longitudinal data structures by providing guidance on how to understand one’s complex data structure.
Being able to interpret the model results and effect size estimates is the necessary foundation for using multilevel LTA to study a broader set of phenomena. The model demonstrated here included two classes across two waves of data collection, which may generalize to the many research studies that use pre-post study designs in the social sciences, for example. The use of the multilevel LTA could also be expanded to include other types of relationships, such as using the smaller subsets of homogeneous groups as an outcome or predictor for more investigations (Nylund-Gibson et al., 2019; Bakk and Kuha, in press). That is, latent class membership could be used to predict a distal outcome. For example, latent class membership could be modeled as a predictor of, say, high school graduation or academic achievement to investigate how early identification of problem behaviors relates to key educational milestones. In summary, multilevel LTA can be useful for investigating a longitudinal nested data structures. Researchers can then use the methods we described here to gain even more information about the within- and cross-level relationships among level-1 latent class membership and level-2 cluster effects. Future work is needed to provide relatively straightforward variance decomposition or models with more latent classes and across more timepoints.
Data Availability Statement
The data analyzed in this study is subject to the following licenses/restrictions: “Restricted-Use Data from U.S. Department of Education”. Requests to access these datasets should be directed to aWVzZGF0YS5zZWN1cml0eUBlZC5nb3Y=.
Author Contributions
All authors listed have made a substantial, direct, and intellectual contribution to the work and approved it for publication.
Conflict of Interest
The authors declare that the research was conducted in the absence of any commercial or financial relationships that could be construed as a potential conflict of interest.
Publisher’s Note
All claims expressed in this article are solely those of the authors and do not necessarily represent those of their affiliated organizations, or those of the publisher, the editors and the reviewers. Any product that may be evaluated in this article, or claim that may be made by its manufacturer, is not guaranteed or endorsed by the publisher.
References
Asparouhov, T., and Muthén, B. (2008). “Multilevel Mixture Models,” in Advances in Latent Variable Mixture Models. Editors G. R. Hancock, and K. M. Samuelsen (Charlotte, NC: Information Age), 27–52.
Bakk, Z., and Kuha, J. (in press). Relating Latent Class Membership to External Variables: An Overview. Br. J. Math. Stat. Psychol. 74, 340–362. doi:10.1111/bmsp.12227
Bakk, Z., and Vermunt, J. K. (2016). Robustness of Stepwise Latent Class Modeling with Continuous Distal Outcomes. Struct. Equation Model. A Multidisciplinary J. 23 (1), 20–31. doi:10.1080/10705511.2014.955104
Banfield, J. D., and Raftery, A. E. (1993). Model-based Gaussian and Non-gaussian Clustering. Biometrics 49 (3), 803–821. doi:10.2307/2532201
Blumen, I., Kogan, M., and Mccarthy, P. J. (1955). The Industrial Mobility of Labor as a Probability Process. Ithaca, NY: Cornell University.
Chung, H., Loken, E., and Schafer, J. L. (2004). Difficulties in Drawing Inferences with Finite-Mixture Models. The Am. Statistician 58 (2), 152–158. doi:10.1198/0003130043286
Collins, L. M., and Lanza, S. T. (2009). Latent Class and Latent Transition Analysis: With Applications in the Social, Behavioral, and Health Sciences. Hoboken, NJ: John Wiley & Sons.
Goodman, L. A. (1961). Statistical Methods for the Mover-Stayer Model. J. Am. Stat. Assoc. 56 (296), 841–868. doi:10.1080/01621459.1961.10482130
Hedeker, D. (2003). A Mixed-Effects Multinomial Logistic Regression Model. Stat. Med. 22 (9), 1433–1446. doi:10.1002/sim.1522
Kaplan, D., Kim, J.-S., and Kim, S.-Y. (2011). “Multilevel Latent Variable Modeling: Current Research and Recent Developments,” in The Sage Handbook of Quantitative Methods in Psychology. Editors R. E. Millsap, and A. Maydeu-Olivares (Thousand Oaks, California: SAGE Publications), 592–612.
Lanza, S. T., Tan, X., and Bray, B. C. (2013). Latent Class Analysis with Distal Outcomes: A Flexible Model-Based Approach. Struct. Equ Model. 20 (1), 1–26. doi:10.1080/10705511.2013.742377
Lubke, G., and Neale, M. (2008). Distinguishing between Latent Classes and Continuous Factors with Categorical Outcomes: Class Invariance of Parameters of Factor Mixture Models. Multivariate Behav. Res. 43 (4), 592–620. doi:10.1080/00273170802490673
McLachlan, G. J., and Basford, K. E. (1988). Mixture Models: Inference and Applications to Clustering. New York, NY: M. Dekker.
McNeish, D., Stapleton, L. M., and Silverman, R. D. (2017). On the Unnecessary Ubiquity of Hierarchical Linear Modeling. Psychol. Methods 22 (1), 114–140. doi:10.1037/met0000078
Morgan, G. B. (2015). Mixed Mode Latent Class Analysis: An Examination of Fit index Performance for Classification. Struct. Equation Model. A Multidisciplinary J. 22 (1), 76–86. doi:10.1080/10705511.2014.935751
Muthén, B., and Asparouhov, T. (2020). Latent Transition Analysis with Random Intercepts (RI-LTA). Psychol. Methods. doi:10.1037/met0000370
Nylund, K. L., Asparouhov, T., and Muthén, B. O. (2007). Deciding on the Number of Classes in Latent Class Analysis and Growth Mixture Modeling: A Monte Carlo Simulation Study. Struct. Equation Model. A Multidisciplinary J. 14 (4), 535–569. doi:10.1080/10705510701575396
Nylund-Gibson, K., Grimm, R. P., and Masyn, K. E. (2019). Prediction from Latent Classes: A Demonstration of Different Approaches to Include Distal Outcomes in Mixture Models. Struct. Equation Model. A Multidisciplinary J. 26 (6), 967–985. doi:10.1080/10705511.2019.1590146
Stapleton, L. M. (2013). “Multilevel Structural Equation Modeling with Complex Sample Data,” in Structural Equation Modeling: A Second Course. Editors G. R. Hancock, and R. O. Mueller. 2nd (Information Age Publishing), 521–562.
Tofighi, D., and Enders, C. K. (2008). “Identifying the Correct Number of Classes in Growth Mixture Models,” in Advances in Latent Variable Mixture Models. Editors G. R. Hancock, and K. M. Samuelson (Greenwich, CT: Information Age), 317–341.
Tourangeau, K., Nord, C., Lê, T., Sorongon, A. G., and Najarian, M. (2009). Early Childhood Longitudinal Study, Kindergarten Class of 1998–99 (ECLS-K), Combined User’s Manual for the ECLS-K Eighth-Grade and K–8 Full Sample Data Files and Electronic Codebooks (NCES 2009–004). Washington, D.C.: National Center for Education Statistics, Institute of Education Sciences, U.S. Department of Education.
Tueller, S. J., Drotar, S., and Lubke, G. H. (2011). Addressing the Problem of Switched Class Labels in Latent Variable Mixture Model Simulation Studies. Struct. Equation Model. A Multidisciplinary J. 18 (1), 110–131. doi:10.1080/10705511.2011.534695
Vermunt, J. K., Langeheine, R., and Bockenholt, U. (1999). Discrete-Time Discrete-State Latent Markov Models with Time-Constant and Time-Varying Covariates. J. Educ. Behav. Stat. 24 (2), 179–207. doi:10.3102/1076998602400217910.2307/1165200
Keywords: multilevel, latent transition, mixture, education, ECLS-K
Citation: Morgan GB and Padgett RN (2021) Multilevel Latent Transition Mixture Modeling: Variance Decomposition and Application. Front. Educ. 6:634528. doi: 10.3389/feduc.2021.634528
Received: 27 November 2020; Accepted: 05 July 2021;
Published: 05 August 2021.
Edited by:
Katerina M. Marcoulides, University of Minnesota Twin Cities, United StatesReviewed by:
Ryan Grimm, SRI International, United StatesJason Rights, University of British Columbia, Canada
Copyright © 2021 Morgan and Padgett. This is an open-access article distributed under the terms of the Creative Commons Attribution License (CC BY). The use, distribution or reproduction in other forums is permitted, provided the original author(s) and the copyright owner(s) are credited and that the original publication in this journal is cited, in accordance with accepted academic practice. No use, distribution or reproduction is permitted which does not comply with these terms.
*Correspondence: Grant B. Morgan, Z3JhbnRfbW9yZ2FuQGJheWxvci5lZHU=