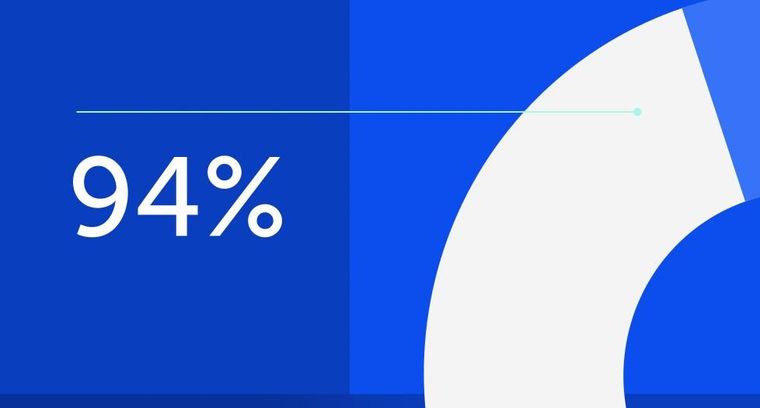
94% of researchers rate our articles as excellent or good
Learn more about the work of our research integrity team to safeguard the quality of each article we publish.
Find out more
ORIGINAL RESEARCH article
Front. Educ., 12 April 2021
Sec. Assessment, Testing and Applied Measurement
Volume 6 - 2021 | https://doi.org/10.3389/feduc.2021.619319
This article is part of the Research TopicExploring Classroom Assessment Practices and Teacher Decision-makingView all 12 articles
Governments expect teachers to be able to make sense of and take action on data at various levels of aggregation. In our research we collaborated with 13 teachers from six primary schools and one intermediate school to use a Data Conversation Protocol to analyze and act on mathematics assessment data generated through a standardized assessment tool—the Progressive Achievement Test (PAT). Our intention was to optimize teacher use of this data for pedagogical decision making and action. At team meetings, the teachers co-constructed then refined a taken-as-shared definition for teacher data literacy for instructional action, which acted to inform and anchor our collaborative research. Data were collected in all teacher meetings and via interviews. Initial findings indicate that a ‘Data Conversation Protocol’ is helping teachers to slow down the process of considering, interpreting and making a judgement about their students’ understanding thereby opening up a space for deeper consideration of the range of possible reasons for student responses to assessment items. Students responded positively to teachers’ data informed small group teaching, gaining in understanding and confidence. Teachers considered this confidence translated to more positive engagement with mathematical ideas. Patterns and trends in student responses emerging from the teachers’ collaborative analysis of standard data supported a shift from viewing student responses as linked to student or school characteristics to critical analysis of how their teaching approaches might have contributed to student answers/misunderstandings. This finding has implications for how we might challenge assumptions about students through a willingness to engage critically with student achievement data. The importance of teachers having a rich pedagogical content knowledge as a basis for this was clearly evident.
Day to day teachers in New Zealand, and other jurisdictions that adopt a non-prescriptive or framework approach to curriculum, enjoy considerable agency in matters such as choice of teaching approaches, the detail of program design and how they assess their students. Given this, the basis and nature of teacher decision-making is of crucial importance. Over the last decade the expectations for teacher use of data as a basis for instructional decision-making have increased (Pierce and Chick, 2011; Schildkamp and Poortman, 2015). Teacher assessment literacy, data-based/data informed decision making and data literacy have emerged as foci for policy and professional development. In this paper our focus is on data literacy. While there is no definitive definition of data literacy it is generally considered to involve teachers establishing a purpose for then collecting, analyzing and interpreting data, and using the insights gained to take instructional action as part of focused inquiry (Datnow and Hubbard, 2015; Gummer and Mandinach 2015; Mandinach and Gummer 2016; Kippers et al., 2018a). This is a complex task, and there is a substantial body of evidence that describes the challenges that teachers face in using data for instructional decision-making and action (e.g., Means et al., 2011; Wayman and Jimerson, 2014; Mandinach and Jimerson, 2016; Schildkamp et al., 2017, ; Visscher, 2020). Moreover, there is evidence that teachers in New Zealand, which is the context of this study, also experience challenges in using data to inform their instructional decision making (Brown and Harris, 2009; Education Review Office ERO, 2018; Edwards and Ogle, 2021; Peter et al., 2017). While intervention research tends to be dominated by studies based in the United States (see Park and Datnow, 2008; Datnow et al., 2012; Marsh, 2012; Athanases et al., 2013), there is evidence of interest elsewhere (Brown and Harris, 2009; Edwards and Ogle, 2021; Kippers et al., 2018a; Kippers et al., 2018b; Lai and McNaughton, 2013a; Lai and McNaughton, 2013b). This work typically positions data literacy as an inquiry sequence similar to that detailed above with data literacy development and enactment relying on a multiplicity of interacting knowledges (statistical, subject content, pedagogical content, curriculum, student, assessment task), teacher mindset and or commitments, and sociocultural/environmental factors (resources, leadership, school culture).
While there has been a sustained emphasis on classroom assessment for formative purposes using teacher generated data (Bell and Cowie, 2001; Black et al., 2003; Ruiz-Primo and Furtak, 2007; Shepard, 2019), changes in technology and increased accountability expectations and measures mean teachers now have access to a wide range of standardized assessment tools and data for classroom use. Research on teacher use of this data is inconclusive, even negative, in terms of its use and impact on classroom level decision making (Stobart, 2008; Lai and Schildkamp, 2013; Volante et al., 2020) suggesting the potential value of this resource is worthy of further investigation.
In this paper we explore teacher consideration and use of data from standardized assessments of student mathematical understanding. Thirteen teachers from seven primary schools and one intermediate school came together to enhance their data literacy skills and explore the instructional potential of data from a widely-used standardized assessment tool. The research project explored the efficacy of a Data Conversation Protocol (DCP) for mediating and supporting processes that embody the principles of productive data analysis, decision-making and instructional action. We illustrate the way the DCP facilitated teacher decision-making and action and conclude that its use permitted teachers to make better founded pedagogical decisions based on root causes rather than symptoms of misconceptions in mathematics. We also detail how the patterns and trends in student responses that emerged from the teachers’ collaborative analysis of standard data supported a shift from viewing student responses as linked to student or school characteristics to critical analysis of how their teaching approaches might have contributed to student answers/misunderstandings. These findings have implications for how we might challenge assumptions about students through a willingness to engage critically with student data and support teachers to make greater/more effective use of standardized student achievement data.
Three lines of research provide the framing for findings and discussion in this paper. These are:
(1) The definition and importance of teacher data literacy.
(2) Teacher access to and understanding of different kinds of assessment approaches and tools.
(3) The use of standardized data for pedagogical decision-making and instructional action.
Teachers are experiencing increasing pressure from accountability systems, focused on evidence-based teaching and or data-based/informed decision making, with this emphasis designed to address equity and achievement gaps (Means et al., 2011; Klenowski and Wyatt-Smith, 2013; Mandinach and Schildkamp, 2020). Within this agenda the press for teachers to have data literacy skills can be traced to 2001 and the No Child Left Behind initiative in the United States, which emphasized the notion of accountability for student learning outcomes based on standardized test data (Wiener and Hall, 2004). Subsequently, the 2015 Every Student Succeeds Act (ESSA) has provided more flexibility in student achievement tracking but the Act still requires the use of overall accountability measures (U.S. Department of Education, 2015). Currently, a number of states in the United States require school leaders and teachers be evaluated, at least in part, by student achievement data (Ross, 2017). Given this history it is unsurprising that researchers from the United States have been at the forefront of scoping the definition of and practices for data literacy with both the definition and practice still evolving.
Broadly speaking, data literacy can be considered as subsuming, overarching and or distinct from the notion of assessment literacy. Data literacy can be theorized as an individual capacity and one that individuals need to acquire and exercise. It can also be theorized as a collective capacity and set of constantly evolving interconnected practices, grounded in the local context and achieved through collaborative endeavor (Peter et al., 2017). In this paper we view data literacy as a metaconcept (Reeves and Honig, 2015; Cowie and Cooper, 2017; Beck et al., 2020) with the definition by Mandinach and Gummer (2016) providing the theoretical grounding for our discussion. The Mandinach and Gummer definition is adopted because of its explicit focus on the use of data for instructional action and its expansive view of the kinds of data that can inform this. It also takes account of the breadth of capabilities teachers need to take data-informed instructional action. The definition states:
Data literacy for teaching is the ability to transform information into actionable instructional knowledge and practices by collecting, analyzing, and interpreting all types of data (assessment, school climate, behavioral, snapshot, longitudinal, moment-to-moment, etc.) to help determine instructional steps. It combines an understanding of data with standards, disciplinary knowledge and practices, curricular knowledge, pedagogical content knowledge, and an understanding of how children learn (2016, 367)
While Mandinach and Gummer are concerned with data literacy to inform and enhance instruction there is ample evidence that teachers experience a tension between this agenda and the broader accountability agenda. Brown and colleagues over a number of country contexts (e.g. Brown, 2008; Deneen and Brown, 2016; Brown et al., 2019) have found that while teachers and student teachers consider that assessment can play a productive role in supporting teaching and learning they also view it as having an evaluative function, a negative impact and or as irrelevant. The teachers in the Brown (2008) study identified an improvement focus and a student evaluative function to do with appraising student performance against standards, assigning scores/grades and awarding qualifications. School and teacher evaluative functions were also identified. Irrelevance was associated with rejecting assessment as having a meaningful connection to learning and or believing it to be bad for students. Brown’s proposition, and the proposition underpinning the research reported here is that how teachers conceptualize the purpose of assessment and data literacy is important because this influences their practice (Brookhart, 2011; Deneen and Boud, 2014; Barnes et al., 2015; Fulmer et al., 2015). Therefore it is important that interventions focused on developing teacher data literacy for instructional purposes help teachers to reflect on their conceptions of and visions of assessment (Deneen and Brown, 2016). Additionally, a teacher’s understanding of the goals and principles underlying a practice are critical because this facilitates the complex, highly situated judgments they need to make without specifying the judgments themselves (Spillane, 2012). Resultant adaptive decisions will always involve, for example, tailoring practice for different groups of students, in specific contexts, as they are engaging with specific kinds of subject matter in order to assist them to achieve valued learning objectives.
There are a plethora of models for the sequence of processes that together lead to data literacy in action/practice, and for how to develop teacher capacity and inclination to work through these. Data literacy interventions typically include an elaboration of the nature of each of the constructs, processes and activities scoped in the Mandinach and Gummer (2016) definition including deciding a focus/goal, data generation methods, data analysis and interpretation, and planning for, taking and reflecting on action, often as an iterative process. Research tends to highlight that teachers can struggle to or may not take instructional action (Kippers et al., 2018a) but there is also evidence that teachers may not have the confidence or knowledge to analyze data in depth (Datnow and Hubbard, 2016; Cowie and Cooper, 2017; Peter et al., 2017; Edwards and Ogle, 2021). Working in the United States, Herman et al. (2015) identified this was the case even for teachers who had access to data from established, high-quality assessments. Van Gasse et al. (2020) and others have identified the tendency to move from data to action with limited consideration of potential causes and or teachers’ own assumptions (e.g., Hoover and Abrams, 2013; Jimerson, 2014; Abrams et al., 2015; Bryk et al., 2015; Schildkamp and Poortman, 2015). They recommend paying specific attention to each of the elements of data literacy (see also Bertrand and Marsh, 2015; Farrell and Marsh, 2016). Van Gasse and colleagues point out that each of the elements requires different knowledge and skills. For example, in our study, in order to make decisions about what to focus on based on a summary report of standardized mathematics data for their class, teachers needed to understand how to read data displays; whereas in order to make decisions about how to teach multiplicative thinking (an identified area of weakness), teachers needed to understand multiplication and the range of ways their students might conceptualize multiplication. In this way each aspect of the data use cycle involves a different kind of knowledge and decision about meaning and priority.
Collaboration among teachers where this includes examining student data together is a commonly recommended strategy for developing and supporting teacher data literacy (Love et al., 2008; Hubbard et al., 2014; Bertrand and Marsh, 2015; Reeves and Honig, 2015; Van Gasse et al., 2017; Visscher, 2020). Specifically, professional collaboration around data use is most productive when it is guided by a broader purpose such as providing equitable and excellent education for students (Datnow and Park, 2019; Visscher, 2020). The proposition is that collaboration can help address the challenges individual teachers face in interpreting data, diagnosing problems and formulating action (Gummer and Mandinach, 2015; Datnow and Hubbard, 2016). Through discussion teachers can revisit their initial explanations for poor student results and reflect upon how these results might be linked to their instruction (Bertrand and Marsh, 2015). This said, there is evidence that collaboration is fraught with complexities of power, trust, and diverse priorities (e.g. Daly, 2012). Teacher attitudes towards and motivations for data use along with their self-efficacy and mental models for data use have been identified as influencing their willingness to collaborate (Datnow et al., 2012; Hubbard et al., 2014; Jimerson, 2014; Van Gasse, et al., 2017). It is therefore important to establish a shared understanding of both the instructional action goal of data literacy and the norms for social interaction around data, such as no blame, collective responsibility, mutual respect (Schildkamp and Poortman, 2015). Teachers need to feel free to take risks and learn from their mistakes in the knowledge they will be supported in the process of experimentation and exploration (Datnow and Park, 2019). Datnow (2020) argues that this kind of professional collaboration is grounded in a mindset of teacher learning, which also provides for emotional support.
A number of studies have identified the value of tools and routines for supporting the development of individual data literacy and of a collaborative culture for data use. For example, Gearhart and Osmundson (2009) identified the value of protocols that embed a clear and specific process for data use and reflection. Others have demonstrated that protocols can support teacher dialogue and data analysis, interpretation, and use within teacher inquiry (Love et al., 2008; Nelson and Slavit, 2008). When practices, tools, and language are shared among teachers, they can much more readily appreciate and learn from one another because they have a common framework for sense-making and goals for participation and learning (Windschitl et al., 2019). In New Zealand, Lai and McNaughton (2013b) demonstrated the value of shared artefacts such as data-interpretation/analysis resources (e.g., PowerPoint slides of graphs and tables that summarized data comparing school achievement data with national data or that displayed relative performances of groups of students which served as templates for schools to use when analyzing their own data) and of the value of schools establishing partnerships with external experts to assist in the development and use of these resources.
To this point we have focused on the data-use cycle as a whole. Here we turn our attention to the nature of data generation as an element that is often taken for granted. Looking beyond education, increasingly people have access to a range of personal health data sourced from wearable technologies/devices. Fors and Pink (2017) argue that the pedagogic importance of personal data lies in ‘how they participate in the constitution of new possibilities that enable people to learn about, and configure, their everyday health in new ways” (59). Put another way they suggest that rather than trying to use data to change behavior, people should use it to expand what it is possible to know, do, and imagine. They propose it is more productive for people pursue what possibilities data open up for them to learn and know differently about elements of their lives that they are already familiar with. Connecting this idea to our research, we are interested in how teachers might collaborate around already familiar standardized mathematics achievement data to open up new possibilities for understanding student learning and informing pedagogical decision-making and instructional action. However, studies from a number of country contexts indicate teachers only make limited use of data generated through externally developed tools to inform classroom decision-making (Schildkamp and Kuiper, 2010; Vanlommel et al., 2017; Volante et al., 2020). Volante et al. (2020), based on their review of teacher use of large scale assessments in seven international jurisdictions (United States, Canada, Australia, England, Germany, Finland, and Singapore), suggest three reasons why there is a lack of good formative use of large scale assessments, that is, of national or state-wide compulsory test or examination data. These are: that separate levels of authority promote different use of tests and the data they generate; that large scale tests are often designed and used for accountability purposes so are limited in their scope, and that large scale and classroom assessment remain separated because no-one is advocating for their integration. Other more practical reasons for teachers making limited use of data may be the time elapsed between data collection and data use and issues of curriculum and pedagogical alignment. Additionally, the nature of sampling and complex administration involving multiple tests to different within-class/school participants can mean that it is difficult to disaggregate data derived from large scale tests for use at classroom level. In other words, not all large scale assessments may be fit for purpose in terms of data use at classroom level. On the other hand Anderson (2006) discusses how analysis of question item responses on a national test in Australia can inform pedagogical decision making and action. Pierce and Chick (2011), in their study of Australian Mathematics and English teachers’ intentions to engage with externally produced statistical data, found that most teachers considered the data could be used to identify weak students and some teachers (mostly mathematics teachers) thought that they could help to identify curriculum topics that needed attention. In a teacher study in New Zealand, Caldwell and Hawe (2016) concluded that a systematic approach to standardized data was needed for students, teachers, schools and other stakeholders to gain full benefit from the data. A challenge in our research was to know more about the processes and supports needed for teachers to learn with and through data and to exploit any opportunities this might offer to create new and productive opportunities for improving student learning and achievement.
New Zealand policy documents have consistently emphasized that the primary purpose of assessment is to support learning and teaching (Ministry of Education, 1993; Ministry of Education, 2011; Ministry of Education, 2019). This purpose has consistently been a focus for professional development (Crooks, 2011). Research has emphasized the role of informal on-the-fly and in-the-moment generation of information and action on what students know and can do and might do next (Bell and Cowie, 2001). Planned and more formal assessment has been recognized as having a role to play in interaction with informal and on-the-fly approaches to provide information on whole class and individual student understandings. Classroom based teacher summative assessments (for example, teacher-designed tests and assignments) are recognized as trustworthy and used for reporting and accountability purposes. As noted above, there is recent and increasing interest in teacher data literacy with this being identified as a requirement for high quality assessment practice (Education Review Office ERO, 2018).
The project from which the data for this paper is drawn is a two and a half year government funded Teaching and Learning Research Initiative (TLRI) project (2019–2021) in New Zealand (http://www.tlri.org.nz/tlri-research/research-progress). The TLRI project is using a design-based implementation research (DBIR) approach (Penuel et al., 2011). DBIR research has a focus on persistent problems of practice and a concern with developing theory related to learning and implementation through collaborative design and systematic inquiry, with a longer term aim of developing capacity for sustaining systematic change. The persistent problem of practice that is the research focus is on how to optimize data use for mathematics teaching and learning purposes through a combination of zooming in and out on data at the level of the individual student, class, school and cluster of schools. A second research focus is on TLRI project teachers working as data coaches with their colleagues to develop colleagues' capability to use data for instructional purposes.
In New Zealand teachers can design and or choose what assessment tasks they use, and there is evidence they access a wide range of sources. There are no compulsory national level assessments at the primary school level but the government has made available a number of assessment tools, the New Zealand Ministry of Education supported and New Zealand Council of Education Research developed Progressive Achievement Tests [PATs] being one of these tools. PATs at a range of levels are available for school years 4–10 in reading comprehension and reading vocabulary, in years 3–10 in listening comprehension and in years 3–10 in mathematics. Tests comprise 30–45 multiple choice items depending on the test level. Most questions have alternative conceptions or distractors built in as option choices. The tests are available in paper-based and adaptive online formats. Teachers can access individual question data and class and individual student data reports. Scale scores and stanine information mean a student’s level of achievement can be tracked from year to year. For the online version, class individual question response data can be compared with national data (NZCER, n.d.). The project teachers are exploring the potential of PAT mathematics data to inform their instructional decision making and working with colleagues as data coaches to develop their colleagues’ capability to use this data. This paper reports on the first year of the project and teacher data-informed action with their classes only.
Teachers from a 16-school Community of Learning | Kāhui Ako (a government funded initiative in which groups of schools in the same area work together to help their students achieve their full potential) were invited to participate in the study with the active consent of their principals. Thirteen teachers from seven different schools volunteered to take part. Ten of the teachers had over ten years teaching experience, the others had taught for between 5 and 10 years. Around a third were the mathematics leader in their school, a third were not, and the remaining third had previously been a mathematics leader in their school.
Four of the schools are full primary schools (Years 0–8), two are contributing schools (Years 0–6) and one is an intermediate school (Years 7–8). Two of the schools were rated within the low end of the socioeconomic ratings in the New Zealand context, two were rated mid-level and three high. The number of students ranged from 118 to 300 for the primary schools. The intermediate school had around 770 students. School student demographics were generally consistent with those New Zealand wide.
In the first year of the project, seven teacher meetings were held, two per term for the first three terms, and one in the final term. At these meetings teachers discussed and then developed a shared definition of data literacy to inform and anchor our collaborative research. Teachers were introduced to a Data Conversation Protocol at the first meeting of the year. They used this to analyze, take action on then report on their class PAT mathematics data.
The Data Conversation Protocol (Table 1) was adapted from that developed by Dalton and Anderson (2016). The research team added the “So then?” question to ensure teachers were prompted to reflect on the impact of their pedagogical decisions and instructional actions.
Meetings were audio-recorded and field notes taken. Teacher powerpoint presentations on the results of their inquiries were collected as were any materials produced during the meetings. Teachers participated in one-to-one end of year reflective interviews. Interview data was transcribed in full. Audio from teacher meetings was selectively transcribed. Data were collated and analyzed thematically (Braun and Clarke, 2006). Data analysis and findings presentation for this paper reflects the three lines of research that frame this paper with regard to teacher use data to inform practice (consistent with the first research question of the TLRI project).
In the next section we set out findings related to the study definition of data literacy and its evolution. We also report on the use of a Data Conversation Protocol to support teacher inquiry/analysis of and action on student data. We then outline an example of the impact of these processes on teacher decision making.
Research emphasises the need for and challenge of developing a shared understanding of goals when teachers undertake research and learning related to data literacy (Jimerson et al., 2020; Mandinach and Schildkamp, 2020). In the TLRI research, teachers and researchers together reviewed available definitions and then co-developed a project definition for data literacy. This was revisited and revised at each meeting. Revisiting and revisioning was deemed necessary because the construct is challenging to define and because it was important that the group had at least a taken-as-shared consensual or compatible understanding (Cobb et al., 1992) of what we were researching together given we were devoting considerable time and effort to the project (Ball et al., 2009; Windschitl et al., 2019). The discussions took place as described below.
The first meeting began with a focus on what counted as data. This was stimulated by the question: When you think about ‘data’ what comes to mind? Data were described as “numbers, information, trends, results, graphs,” but also as “more than just numbers.” Teachers questioned whether the data they had access to were always a true reflection of a child’s understanding and progress. They discussed whether they would ‘trust’ data, depending on when and how it was collected and analyzed. An additional prompt was: What sort of ‘data’ did you use when you made an overall teacher judgement (OTJ). [Until 2010 teachers were expected to collate a range of data to make an OTJ about their students’ mathematics achievement relative to a set of nationally mandated standards and this language and thinking has persisted since the requirement was revoked in 2017].
The next prompt asked teachers to consider: To you, what is data literacy? Groups discussed and contributed definitions. Examples included: “Data literacy is being able to read and understand data, look for trends and patterns, look for validity and critique, then use data effectively”; “Data literacy is to be able to collect, understand, reproduce and utilize worthwhile data”, and “To gather, analyze, act on and reflect on these actions related to data”.
Two definitions were then shared:
A process that integrates the analysis of educational data to support decisions intended to improve teaching and learning at the school and classroom levels. (Means et al., 2010).
Data literacy is the ability to understand and use data effectively to inform decisions. It is composed of a specific skill set and knowledge base that enables educators to transform data into information and ultimately into actionable knowledge. (Mandinach and Gummer, 2013).
Teachers were asked to discuss if and how the definitions were consistent with their own ideas. They considered they were. The group then came together to negotiate the definition of data literacy they would use to inform their collaboration in the project. This definition was crafted by recording and adjusting dictated sentences on a whiteboard. The following statement was agreed at the end of this meeting:
Data literacy involves collecting/gathering data, analyzing and understanding it and then using this understanding to take action. It includes the knowledge needed to decide if data is worthwhile and or valid and the ability to share information to different groups (Children, other teachers, principal, Boards of Trustees [School governance board] etc.)
This was the taken-as-shared definition for teacher data literacy. The inclusion of an explicit mention of taking action on data echoed teachers initial ideas and the focus of the definitions shared with them. Teacher individual interviews indicated that the project commitment to action had been important in them volunteering to be part of the project. The second sentence was a source of more debate. The teachers designed it to encapsulate their concern to maintain a critical stance towards data and their view that information on student learning was of interest and value to a range of decision-makers. This focus was exemplified in the comment, “Parents are interested in their children’s progress and we need to be able to communicate this to them”. This focus is in line with New Zealand assessment policy (Ministry of Education, 2007; Ministry of Education, 2011).
In the second meeting on 24 May, the teachers were reminded of the co-constructed definition and encouraged to refine it. In this session they were talking about data literacy in the context of their own work, and critiquing each other’s thinking and the assessment tools they used themselves. For instance, as teachers discussed key issues that examination of PAT data revealed, they discussed the interaction of validity and assessment task design, asking, “What knowledge is needed to unpack a question?” Again, they noted the need to critique both data and the questions that led to it. Commenting, on some of the PAT questions they pointed out that “students can’t answer a question involving time differences on an analogue clock if they can’t read an analogue clock” and “it is difficult to estimate volume if students are unsure of context or whether to use milliliters or liters”.
During the third workshop on 19 June the team again revisited their data literacy definition. The definition was on display and edited publicly with teachers offering suggestions for additions and refinements. On this occasion the discussion focused on what counted as data in concert with exploring the implications of a holistic view of students and of teachers’ responsibility towards their students as ‘whole people’. That is, the discussion encompassed the need for teachers to understand how, when and why different data was produced and raised questions about what could and should be considered as relevant for data and data generation when a teacher’s goal is to assist students in their learning. The holistic vision of the student as a learner that the teachers endorsed is consistent with current policy within New Zealand (Ministry of Education, 2007). It is also in line with international policy, for example the Every Student Succeeds Act (2015). The teachers’ recognition of the need to draw on different types of data and to have an expansive focus when the student group is diverse is supported by research (Gipps and Murphy, 1994; Stobart, 2008; Bernhardt, 2018) and is congruent with system, school, teacher and family interest in the behavioral, affective, and cognitive dimensions of learning and being a learner. As part of their discussion the teachers again raised and discussed the validity of the assessment data they might use: “Had they [students] ever been through this type of question, with this wording? Testing that is too hard provides poor data as when students find the test too hard they give up”. They concluded that they needed to interrogate assessment questions and student responses. Other points for consideration were whether the questions actually tested what students need to know. The teachers concluded that students needed to have an opportunity to show that they knew and that perhaps this would mean changing some questions.
After several iterations the discussion converged on the following description for data:
For us ‘data’ is a wide range of information including student learning conversations, perceptions, observations, and products of learning, school processes, student demographics (afterBernhardt, 2018) and includes different levels of aggregation.
Teacher revisiting of the definitions for data literacy has been ongoing, and in 2020 dimensions of vision and data literacy culture have been added to the definition by the teachers. These are the focus of another paper. The taken-as-shared definition for data and for teacher data literacy for instructional action have provided common reference points for teachers when they collaboratively work and talk together. The definitions provide a framework within which the teachers are comfortable to work.
The research team introduced a Data Collection Protocol (DCP) to the teachers at the first project meeting in anticipation it would lead to a taken-as-shared way of talking about how they might work with data. The first step of the DCP above prompts teachers to reflect systematically on the data they have generated using the ‘Here’s what?’ prompt. As noted above, teacher focus in the first year of the study was on PAT mathematics data. The mathematics PAT assessments include questions on number knowledge, number strategies, algebra, geometry, and measurement and statistics. The teachers brought their class PAT data to the first meeting. This included individual student responses for each question and test totals with associated scale scores. This information was in tabular form for individuals and scatter plots for classes. No statistical analysis was included. The teachers also had access to national item and item option response distributions for questions for comparison.
The ‘Here’s what’ prompt stimulated a robust discussion on ‘What counts as data and for what purposes?’ We were all surprised at the level of debate this question generated as discussion probed matters to do with validity, reliability, equity and consequences. Teachers listed and critiqued the nature and potential meanings of the results of commercial tests, of teacher generated tasks, of classroom-based observations and dialogue as well as data on attendance and student mobility across schools. They identified gaps and variations in their individual knowledge in terms of different assessment tools and knowledge of what data analysis support was available with tools such as PAT. The group then discussed and negotiated a meaning for the other three prompts. Following this, teachers formed small groups and analyzed the class PAT data they had brought to the meeting by looking for patterns within and across their classes and nationally for individual PAT item results. This process included thinking about what actions they could/would take; the “Now what?“.
Teachers were then charged to select and take action with 2–5 ‘target’ students. The group decided these students would be selected on the basis that data identified a common misconception or a need for strategy development. For example, one teacher selected target students who had incorrect responses to approximately two thirds of the measurement/geometry questions in PAT Mathematics Test 4. At the next meeting the teachers reported back on their actions with their target students.
In what follows we outline key themes and insights that arose during the two first year meeting discussions based on the DCP. In essence, the key insights that teachers discussed were the value of taking time to analyze data, of questioning data as evidence of student learning, of considering possible underlying/conceptual reasons for student responses, and of planning for follow up action including revisiting concepts to develop student understanding and confidence.
We have already noted that the teachers critiqued the data they generated through their own classroom based assessment/OTJs and the data generated by commercial sources such as PAT. They concluded that ultimately, “The data is the starting point but we have to know the students” with this point flowing naturally into the “So what?” step of the DCP. Consideration of this step ‘slowed down’ the teachers’ analysis and interpretation of the data they had in front of them. It included consideration of how previous experiences and understanding might be implicated in student responses as in the following teacher comment: “There is a group of students who are flatlining—why are they like that? This can mean going back to previous data to find gaps in understanding.” Through comments such as these teachers can be seen to be questioning the data as robust evidence of student learning. The group public consensus was that it was important to not make assumptions about what students did know and could do and what they did not know and could not do nor to assume that skills were in place when they might not be on the basis of one data source. Here teacher data interpretation and critique is in line with Raffe et al. (2019) assertion that, “targeting outcomes without understanding the context or procedural mechanisms that produce them yields constrained insight into how to support and enhance teachers’ data use practices.” (94). It also suggests that standardized data offers a snapshot of learning and should not be used in isolation or considered as the sole basis to judge learner achievement.
The teachers commented that prior to the TLRI project they might not have analyzed their class PAT data. It was seen as having relevance only for their principal. The following teacher comment is representative of the group view: “Before I would have just marked the PATs, got a stanine to give to my Principal and gone, ‘OK’”. The teacher continued, “But this time I actually looked at it and thought, ‘Oh, measurement...still work to do.’ And it has informed my teaching for this term—we’re going to go back and revisit measurement this term before they move on next year.”
Teacher analysis of possible reasons for student responses to particular questions involved them in taking the time to consider what might be the basis for student answers and to plan for teaching. One teacher noted: “Often you look at the data and we just say they [students] need to work on their addition and subtraction strategies without really narrowing down to look at what do we actually need to work on.” A representative comment was: “I’ve never delved as deeply into it [data] before.” We can see here that the teachers thought that taking time allowed them to analyze the data more deeply and to think about the implications of the teaching approaches they used: “We’re all good teachers - but this has given me the time to think about my teaching.” The teachers came to the view there was value in taking “a little bit extra time to get proportionately more value”. This is an important realisation given evidence from elsewhere that teachers tend to spend very little time and do not consistently analyze student work in depth (Herman et al., 2015). Also important was that slowing down in this way interrupted teacher habits to do with, “This is how I teach this”. Careful analysis at the “So what?” step meant for one teacher that, “I can actually hone in on students’ [ideas and or misconceptions] and ensure planning in relation to that.” Through thorough analysis teachers, “Could pin down the issue - and pin down what next”. As another teacher explained, “Careful analysis of one question from the PAT test allowed me to really focus my planning and my teaching on the (concept)”. Teachers were emphatic that their focused work with small groups of selected students, the “Now what?” element of the DCP, was better targeted and more productive. One teacher commented, “Without taking the time to look closely at this data I may have spent less time teaching (the concept) to these students (because I was) assuming that it was more of a calculation error rather than a lack of knowledge.”
Typically, teachers’ deeper analysis and follow up actions led to their revisiting earlier ideas. In the words of one teacher “I had to go right back but going back helped students move forward.” Student responses alerted teachers to the idea that, “We need to take time to cement learning before moving on.” They came to appreciate, in line with a number of studies (in the New Zealand context: Alton-Lee, 2003; Nuthall, 2007) that students benefit from encountering ideas multiple times and in multiple contexts: “The process highlighted for me the importance of breadth and providing students with multiple opportunities to grasp a concept, and more time than I would have previously allowed for.” This said, the teachers were clear they did not want their teaching responses to become “gap-filling” even though this might be needed for students to make progress. Datnow and Park (2019) pointed out that teachers need to plan for student growth by identifying student strengths. Comments such as “Celebrating successes and making sure each child has a success to celebrate” indicated that teachers also considered this was important.
In commenting on student responses to their intervention actions the teachers focused on the development of student understanding and the benefit to student confidence that came with understanding an idea that had previously been confusing. One teacher explained this impact as follows:
Children can see success—they’ve figured out one small thing but then that might be one tiny brick in the foundation, and then it snowballs. The kids are keen cos they can do it, instead of a great big concept, they can do one tiny thing then another tiny thing, and so on.
Other observations were that students now had the confidence to articulate their thinking, were happier in their work and more willing to attempt more complex problems.
A number of teachers commented on how their focused follow up actions with a small group of students had alerted them to the subtle variations in student understanding, or as one teacher explained the situation, “every student is at a different point in their journey.” Another teacher elaborated on this point saying, “If I had taught them in a bigger group, they [students] may have missed it, and I as a teacher would have missed subtleties as well.” One of the teachers summarized the overall impact of the data inquiry on her own practice and on student learning as follows:
I found I was listening more to my students, really paying attention to what their needs were as a targeted group and as individuals. The growth and increased confidence all of these students showed was tremendous.
The teachers considered their attention to the ‘So then’ aspect was particularly important in shifting student achievement as it prompted them to review the impact of their actions. One teacher summed up the implications of this focus as: “This is, in some ways, the most important step, especially if the data shows that there is still an issue.”
Although the teachers were focusing on data from their own classes, they recognized there were many areas of common concern when they shared ideas across the group. The influence of this collective sharing and recognition of common concerns is explained further in the next section.
Teachers used the Data Conversation Protocol to think about the data as individuals and in small groups then shared ideas with the whole group. During this sharing process eight of 13 teachers identified two-digit subtraction as problematic for their students. Collective analysis and sharing of student choice of answers revealed the commonly accepted answer was a deliberately designed distractor which fitted with students decomposing both numbers and subtracting the smaller ‘ones’ digit from the larger. One teacher explained: “Say if it was 52–38, they could do 50–30 but then they would just automatically swap the ones digits around because they couldn’t do 2–8. So, they just automatically went 8–2 = 6.” Important to the subsequent discussion, this pattern of choice was consistent across schools and the years for which the PAT question applied. One teacher explained the impact of sharing with the TLRI group: “Someone brought [the subtraction issue] up and it was, ‘Oh, that’s right, we have that problem as well.’ I thought it was just an issue at our school.” Another described discovering, “We all had the same issue of [students] swapping around the ones number. We’ve just looked at this because of the group, because of the coaching that we’ve been getting and noticing that in the [geographical area/Kahui Ako] that there’s an issue.” The following comment is representative of those from teachers who focused on this issue with a small group of students:
Now that I’ve seen what can happen I’d definitely go with only decomposing the second number, that has helped immensely those kids that were really struggling.
Another Explained:
… The Year 7 and 8 students I was working with, once they ‘got’ what I was telling them, they were just so AMAZED, at what they could do, because they were kids who have struggled all their way through, not achieving where they need to be, and they just looked and went “OHHHHHH! OK!”
This realisation led to another teacher asking: “Do we set students up correctly for subtraction when we teach addition?” In the context of two-digit subtraction this question related to the commonplace process of teaching two-digit addition as a process of decomposing both numbers and adding. Reflecting on this strategy the teachers’ analysis led to their recognition that students’ difficulties could be attributed to what (Ryan and Williams, 2007, 23; see also Anderson, 2009) term “intelligent overgeneralization”. Ryan and Williams describe this as the tendency to create inappropriate rules based on past experiences, that is to overgeneralize a strategy or rule of thumb. It is of note that all 13 teachers were interested in and confident in teaching mathematics but clearly many had not encountered or thought about the longer-term implications of the decomposition strategy for two-digit subtraction. The group concluded that while the decomposition teaching strategy might be helpful in the short term, they needed to reconsider its use—in the future they would teach students to decompose only the subtractend. From this example the conversation turned to wider consideration of the longer-term consequences of pedagogical strategies with one teacher asking directly: “How does what is being taught at younger year groups/lower levels impact what is taught in subsequent levels?” Suggestions for common generalizations included: comprehension of the equals symbol, where students understand the equals symbol as “find an answer” rather than “the same as”; multiplication makes bigger; division makes smaller; and longer numbers are always greater in value.
The consistency of student responses across the schools was pivotal in prompting the group to speculate that it might be their teaching strategies and not student or school attributes that were the likely reason for students’ answers. In the words of one of the teachers:
It was definitely the sharing, just bringing up that issue and then everyone going “Oh yeah, we have that issue”. You sort of just think it’s our kids …. And it was like a catalyst to think what else are we doing that might not just be them. It’s definitely something I’m going to share with the rest of the staff as well.
Teacher discussions, which pooled data and insights from teachers from different schools and school year levels, could be seen to identify and explore what Ball (1993) refers to as “horizon knowledge”. Horizon knowledge includes knowledge of how mathematical topics are related over the span of the curriculum. It includes the content and pedagogical content knowledge teachers need to understand the significance of ‘what comes before and after’ in connection to mathematical ideas. Ball argues knowledge of the mathematical horizon is important because of the role it plays in teacher decision making and because a teacher’s choices can anticipate or undermine later development, or what one teacher in our study described as “setting up misconceptions for the future”. Teachers in the TLRI project clearly came to appreciate the need to consider this possibility as a consequence of their collaborative analysis of the same standardized assessment data and the DCP.
Teacher data literacy and its development are a focus internationally, and in New Zealand which is the context for this paper. The proposition is that data use can inform and enhance teacher pedagogical decision making and action. While the focus is often on teacher formative use of data generated informally through interaction the rise in provision of commercially produced standardized assessments has opened up new and different opportunities for teacher access to data on their students’ learning. In this paper we report findings from a study exploring if and how the use of data from a commonplace assessment tool (the PAT) could be used in teacher pedagogical decision-making. PAT assessments have been in use in New Zealand classrooms for over 40 years. Generally, teachers administer the tests and the principal and school leaders’ access, analyze, reflect and act on results. Classroom teachers might take a cursory look at their class and individual student results but on the whole the data is not viewed as having direct pedagogical value.
There is considerable evidence that teachers can struggle to appreciate the pedagogical purpose of data literacy and also that, as a group, teachers can struggle to build a common understanding of the knowledge, habits of mind and language involved in data literacy (Means et al., 2011; Lai and Schildkamp, 2013; Kippers et al., 2018a; Henderson and Corry, 2020). The intervention in the study reported here began by establishing a shared understanding of the nature of and purpose for teachers working together to develop their data literacy. Teachers’ critical reflection on and refinement of their co-constructed definition for data literacy each time they met together appeared to be important in sustaining their commitment to and collective ownership of it as a process focused on informing pedagogical decision-making and action (Brown, 2008; Datnow and Park, 2019). The reiteration of its instructional purpose was important in locating their work as counter to their experience that often PAT data was only used by their principals for reporting and accountability purposes. The evolving definition provided a concrete and meaningful anchor and language for their collaborative discussion through its articulation of the process and the purpose for data use—to enhance instruction in support of student learning.
To operationalise data literacy, we employed a Data Conversation Protocol. This guided teachers in their deeper consideration of what the distribution and detail of their students’ PAT results could tell them about their students’ thinking and their own practice. Although teacher in depth interpretation of and planning for individual student learning took time, the teachers were convinced that this time was well spent—they were more than pleasantly surprised by their students’ responses. As others have found (Datnow and Park, 2019), the teachers were emphatic that they benefited from sharing their experiences with colleagues—student responses and collegial sharing and feedback validated the processes that had been undertaken as worth employing and sharing more widely (teachers are working through a process for this). Teachers using and discussing the question prompts in the Protocol focused teacher attention on the demands of particular assessment items and the patterns of student responses within and across items. Being able to consider these patterns across school years and schools appears to be particularly productive in stimulating the sharing and critical analysis of teaching approaches rather than student attributes. Through their cross school and school year level discussions teachers raised and illustrated the need to consider the possibility of unforeseen consequences of their pedagogical decisions, with the example given in this paper being the longer-term implications of a particular approach to solving addition and subtraction problems. It was the use of a standardized assessment tool (PAT) that allowed the teachers to genuinely share and discuss commonalities in student responses which then impelled them to look beyond individual student attributes and school stereotyping to consider possible implications of their pedagogical approaches. This in turn opened up different foci and options for instructional action. To us, this process has echoes of what Fors and Pink (2017) advocate in relation to the potential reconsideration of familiar data. They propose this can lead to “the constitution of new possibilities” (59), in our study this led to a critical evaluation of a commonplace teaching strategy.
In considering teachers’ action on data, Claudet (2020) argument that effective learning interventions need to address underlying “root causes” rather than surface-level “symptoms” is pertinent. Surface-level symptoms are generally more easily discernible than root causes but if root causes are not identified, then the time teachers spend on symptoms may have limited long term impact. However, identifying root causes takes time and thought. As the teachers reported, identification and action on root causes relies on teachers having in depth content and pedagogical content knowledge (Shulman, 1987). Both were needed for them to interpret the thinking that might underpin student responses. Teachers taking the time to work with a small group of students on a very specific mathematics idea alerted them to the nuances and variations in student thinking and, in some instances, challenged their assumptions about student thinking. Their comments indicated that this experience might have sensitized them to the value of careful analysis and listening going forward. In considering the efficacy of teachers’ actions it is also of note that the teachers identified that their students responded very positively to finally making sense of/understanding an idea and the confidence students gained from this success translated into their confidence in approaching other ideas/challenges. This further highlights the benefit that might be gained from such small and focused actions.
Teachers do not always consider that standardized data generated via externally produced tasks has value for pedagogical decision-making and action (Volante et al., 2020). The teachers and research team chose to focus on standardized PAT data as an opportunity to make greater formative use of data teachers were already obliged to collect. The research shows that standardized data can provide teachers with useful insights into their students’ learning, especially when they take time for careful collaborative analysis, as discussed above. Standardized tools are often online and produce a range of pre-designed reports that have the potential to inform and fast-forward teacher decision-making. Teachers in our TRLI study benefited from the range of reports that could be generated from PAT data–item, individual student, class and school. Their analysis and action on the PAT assessment data benefited from the inclusion and detailing of the distractors that were included as options in most test questions. These were based on student alternative conceptions and provided teachers with information about student ideas that they could use to inform their instructional actions (Anderson, 2009; Gierl et al., 2017). In line with the literature the teachers did raise the matter of pedagogical alignment—would their students recognize the question context and format—but they circumvented the matter of timing and curriculum alignment by focusing on particular ideas with small groups of students. In this way they were able to support targeted to students who were/were likely to be struggling with specific and important ideas. As they commented, this was both time consuming and worthwhile.
Looking forward, it is significant that through their analysis and sharing of standardized data the teachers in this study identified shifts in their focus from students or their own school as being the cause of a learning deficit to consideration of the longer-term impacts of the teaching approaches they were using. Cross school and cross school level sharing using the Data Conversation Protocol was important in this because it prompted teachers to slow down and carefully consider the patterns within and across the data they each had. This challenged rather than confirmed their assumptions (Datnow and Park, 2018) opening up space for new ways of thinking and acting as identified by Fors and Pink (2017), something that is important when then the goal is to enhance instruction for all, and not just some, students. The Data Conversation Protocol and the practices associated with it were both important because together they provided teachers with the agency and tools for better informed decision-making and action. The protocol also provided a basis from which teachers could begin to coach colleagues (a paper in preparation describes the work of teachers as coaches). The project has reported these findings to all sixteen schools in the Kāhui Ako. With principal support we are now working with the TLRI teachers to develop ways to share these insights with teachers in other Kāhui Ako schools.
The datasets presented in this article are not readily available because Data is confidential to the research team. Requests to access the datasets should be directed to bcowie@waikato.ac.nz.
The studies involving human participants were reviewed and approved by Division of Education, University of Waikato. The patients/participants provided their written informed consent to participate in this study.
We have all contributed to this manuscript.
The study that underpins this paper was funded by the New Zealand Ministry of Education Teaching and Learning Research Initiative fund which is administered by the New Zealand Council of Educational Research.
The authors declare that the research was conducted in the absence of any commercial or financial relationships that could be construed as a potential conflict of interest.
We acknowledge and thank the schools and teachers from the Pukekohe Kāhui Ako (Community of Learners schools) for their participation, in particular Nicola Gibson, Pukekohe Intermediate School who has provided active and insightful leadership to the project.
Abrams, L. M., McMillan, J. H., and Wetzel, A. P. (2015). Implementing benchmark testing for formative purposes: Teacher voices about what works. Educ. Asse Eval. Acc. 27, 347–375. doi:10.1007/s11092-015-9214-9
Alton-Lee, A. (2003). Quality teaching for diverse students in schooling: Best evidence Synthesis iteration [BES]. Report from the medium term strategy policy division. Wellington: Ministry of Education.
Anderson, J. (2009). Using NAPLAN items to develop students’ thinking skills and build confidence. Aust. Math. Teach. 65 (4), 17–23.
Athanases, S. Z., Bennett, L. H., and Wahleithner, J. M. (2013). Fostering data literacy through preservice teacher inquiry in English language arts. Teach. Educator 48 (1), 8–28. doi:10.1080/08878730.2012.740151
Ball, D. L., Sleep, L., Boerst, T. A., and Bass, H. (2009). Combining the development of practice and the practice of development in teacher education. Elem. Sch. J. 109 (5), 458–474. doi:10.1086/596996
Ball, D. L. (1993). With an eye on the mathematical horizon: Dilemmas of teaching elementary school mathematics. Elem. Sch. J. 93 (4), 373–397. doi:10.1086/461730
Barnes, N., Fives, H., and Dacey, C. M. (2015). “Teachers beliefs about assessment,” in International handbook of research on teacher beliefs. Editors H. Fives, and M. G. Gill (New York: Routledge), 284–300.
Beck, J. S., Morgan, J. J., Brown, N., Whitesides, H., and Riddle, D. R. (2020). Asking, learning, seeking out: An exploration of data literacy for teaching. Educ. Forum 84 (2), 150–165. doi:10.1080/00131725.2020.1674438
Bertrand, M., and Marsh, J. A. (2015). Teachers’ sensemaking of data and implications for equity. Am. Educ. Res. J. 52 (5), 861–893. doi:10.3102/0002831215599251
Black, P., Harrison, C., Lee, C., Marshall, B., and Wiliam, D. (2003). Assessment for learning: Putting it into practice. Buckingham: Open University.
Braun, V., and Clarke, V. (2006). Using thematic analysis in psychology. Qual. Res. Psychol. 3 (2), 77–101. doi:10.1191/1478088706qp063oa
Brookhart, S. M. (2011). Educational assessment knowledge and skills for teachers. Educ. Meas. Issues Pract. 30 (1), 3–12. doi:10.1111/j.1745-3992.2010.00195.x
Brown, G., Gebril, A., and Michaelides, M. (2019). Teachers' conceptions of assessment: A global phenomenon or a global localism. Front. Edu. 4 (16). doi:10.3389/feduc.2019.00016
Brown, G. T. L. (2008). Conceptions of assessment: understanding what assessment means to teachers and students. New York: Nova Science Publishers.
Brown, G. T. L., and Harris, L. R. (2009). Unintended consequences of using tests to improve learning: how improvement-Oriented resources heighten conceptions of assessment as school accountability. J. Multidisciplinary Eval. 6 (12), 68–91.
Bryk, A., Gomez, L., Grunow, A., and LeMahieu, P. (2015). Learning to improve: how America's schools can get better at getting better. Cambridge: Harvard University Press.
Caldwell, A., and Hawe, E. (2016). How teachers of years 4–8 students analyse, interpret and use information from the progressive achievement test: mathematics. Assess. Matters 10, 100–125. doi:10.18296/am.0019
Claudet, J. G. (2020). Using design research thinking and data-teaming processes to transform educators’ professional practice: A School Improvement Case Study. Int. J. Edu. Soc. Sci. 7 (1), 17–41.
Cobb, P., Wood, T., Yackel, E., and McNeal, B. (1992). Characteristics of classroom mathematics traditions: An interactional analysis. Am. Educ. Res. J. 29, 573–604. doi:10.3102/00028312029003573
Cowie, B., and Cooper, B. (2017). Exploring the challenge of developing student teacher data literacy. Assess. Educ. Principles Pol. Pract. 24 (2), 147–163. doi:10.1080/0969594x.2016.1225668
Crooks, T. (2011). Assessment for learning in the accountability era: New Zealand. Stud. Educ. Eval. 37 (1), 71–77. doi:10.1016/j.stueduc.2011.03.002
Dalton, J., and Anderson, D. (2016). Learning talk: Important conversations at work: Hands On Educational Consultancy Pty Limited.
Daly, A. (2012). Data, dyads, and dissemination; Exploring data use and social networks in educational improvement. Teach. Coll. Rec. 114 (11), 1–38.
Datnow, A., and Hubbard, L. (2016). Teacher capacity for and beliefs about data-driven decision making: A literature review of international research. J. Educ. Change 17 (1), 7–28. doi:10.1007/s10833-015-9264-2
Datnow, A., and Hubbard, L. (2015). Teachers’ use of assessment data to inform instruction: Lessons from the past and prospects for the future. Teach. Coll. Rec. 117 (4), 1–26.
Datnow, A., Park, V., and Kennedy-Lewis, B. (2012). High school teachers’ use of data to inform instruction. J. Edu. Students Placed Risk 17 (4), 247–265. doi:10.1080/10824669.2012.718944
Datnow, A., and Park, V. (2018). Opening or closing doors for students? Equity and data use in schools. J. Educ. Change 19, 131–152. doi:10.1007/s10833-018-9323-6
Datnow, A., and Park, V. (2019). Professional collaboration with purpose: teacher learning for equitable and excellent schools. New York, NY: Routledge.
Datnow, A. (2020). The role of teachers in educational reform: A 20-year perspective. J. Educ. Change 21, 431–441. doi:10.1007/s10833-020-09372-5
Deneen, C., and Boud, D. (2014). Patterns of resistance in managing assessment change. Assess. Eval. Higher Edu. 39 (5), 577–591. doi:10.1080/02602938.2013.859654
Deneen, C. C., and Brown, G. T. L. (2016). The impact of conceptions of assessment on assessment literacy in a teacher education program. Cogent Edu. 3, 1225380. doi:10.1080/2331186x.2016.1225380
Education Review Office ERO (2018). Evaluation at a glance: A decade of assessment in New Zealand Primary Schools - practice and trends. Available at: https://www.ero.govt.nz/publications/evaulation-at-a-glance-a-decade-of-assessment-in-new-zealand-primary-schools-practice-and-trends/section-three-ongoing-successes-and-challenges-when-collecting-and-using-assessment/.
Edwards, F., and Ogle, D. (2021). Supporting teachers’ data informed decision-making: Data informed leadership by mathematics lead teachers in New Zealand. Teach. Dev. 25 (1), 18–36. doi:10.1080/13664530.2020.1837217
Farrell, C. C., and Marsh, J. A. (2016). Contributing conditions: A qualitative comparative analysis of teachers’ instructional responses to data. Teach. Teach. Edu. 60, 398–412. doi:10.1016/j.tate.2016.07.010
Fors, V., and Pink, S. (2017). Pedagogy as possibility: health interventions as digital openness. Soc. Sci. 6, 59–71. doi:10.3390/socsci6020059
Fulmer, G. W., Lee, I. C. H., and Tan, K. H. K. (2015). Multi-level model of contextual factors and teachers’ assessment practices: An integrative review of research. Assess. Educ. Principles, Pol. Pract. 22, 475–494. doi:10.1080/0969594x.2015.1017445
Gearhart, M., and Osmundson, E. (2009). Assessment portfolios as opportunities for teacher learning. Educ. Assess. 14 (1), 1–24. doi:10.1080/10627190902816108
Gierl, M. J., Bulut, O., Guo, Q., and Zhang, X. (2017). Developing, analyzing, and using distractors for multiple-choice tests in education: A comprehensive review. Rev. Educ. Res. 87 (6), 1082–1116. doi:10.3102/0034654317726529
Gipps, C., and Murphy, P. (1994). A fair test? Assessment, achievement and equity. Buckingham, PA: Open University Press.
Gummer, E., and Mandinach, E. (2015). Building a conceptual framework for data literacy. Teach. Coll. Rec. 117 (4), 1–22.
Henderson, J., and Corry, M. (2020). Data literacy training and use for educational professionals. J. Res. Innov. Teach. Learn. [Epub ahead of print]. doi:10.1108/JRIT-11-2019-0074
Herman, J., Osmundson, E., Dai, Y., Ringstaff, C., and Timms, M. (2015). Investigating the dynamics of formative assessment: relationships between teacher knowledge, assessment practice and learning. Assess. Edu. 22 (3), 344–367. doi:10.1080/0969594x.2015.1006521
Hoover, N. R., and Abrams, L. M. (2013). Teachers’ instructional use of summative student assessment data. Appl. Meas. Edu. 26 (3), 219–231. doi:10.1080/08957347.2013.793187
Hubbard, L., Datnow, A., and Pruyn, L. (2014). Multiple initiatives, multiple challenges: The promise and pitfalls of implementing data use. Stud. Educ. Eval. 42, 54–62. doi:10.1016/j.stueduc.2013.10.003
Jimerson, J. B., Garry, V., Poortman, C. L., and Schildkamp, K. (2020). Implementation of a collaborative data use model in a United States context. Stud. Edu. Evaluat. [Epub ahead of print]. doi:10.1016/j.stueduc.2020.100866
Jimerson, J. B. (2014). Thinking about data: exploring the development of mental models for ‘‘data use’’ among teachers and school leaders. Stud. Educ. Eval. 42, 5–14. doi:10.1016/j.stueduc.2013.10.010
Kippers, W. B., Poortman, C. L., Schildkamp, K., and Visscher, A. J. (2018a). Data literacy: what do educators learn and struggle with during a data use intervention? Stud. Educ. Eval. 56, 21–31. doi:10.1016/j.stueduc.2017.11.001
Kippers, W. B., Wolterinck, C. H. D., Schildkamp, K., Poortman, C. L., and Visscher, A. J. (2018b). Teachers’ views on the use of assessment for learning and data-based decision making in classroom practice. Teach. Teach. Edu. 75, 199–213. doi:10.1016/j.tate.2018.06.015
Klenowski, V., and Wyatt-Smith, C. (2013). Assessment for education: Standards, judgement and moderation. United States: Sage.
Lai, M. K., and McNaughton, S. (2013a). “An approach for developing effective research-practice partnerships: Lessons from a decade of partnering with schools in poor urban communities,” in Research partnerships within early years education: Relational expertise and knowledges in action. Editors J. Duncan, and L. Connor (New York: Palgrave MacMillan), 49–70.
Lai, M. K., and McNaughton, S. (2013b). “Analysis and discussion of classroom and achievement data to raise student achievement,” in Data-based decision making in education: Challenges and opportunities. Editors S. Schildkamp, M. K. Lai, and L. Earl (Netherlands: Springer), 23–48.
Lai, M., and Schildkamp, K. (2013). “Data-based decision making: An overview,” in Data-based decision making in education: Challenges and opportunities. Editors K. Schildkamp, M. K. Lai, and L. Earl (Dordrecht, Netherlands: Springer), 9–21.
Love, N., Stiles, K. E., Mundry, S., and DiRanna, K. (2008). A data coach’s guide to improving learning for all students: Unleashing the power of collaborative inquiry. United States: Corwin.
Mandinach, E. B., and Gummer, E. S. (2013). A systemic view of implementing data literacy in educator preparation. Educ. Res. 42 (1), 30–37. doi:10.3102/0013189x12459803
Mandinach, E. B., and Gummer, E. S. (2016). What does it mean for teachers to be data literate: Laying out the skills, knowledge, and dispositions. Teach. Teach. Edu. 60, 366–376. doi:10.1016/j.tate.2016.07.011
Mandinach, E. B., and Jimerson, J. B. (2016). Teachers learning how to use data: a synthesis of the issues and what is known. Teach. Teach. Edu. 60, 452–457. doi:10.1016/j.tate.2016.07.009
Mandinach, E. B., and Schildkamp, K. (2020). Misconceptions about data-based decision making in education: An exploration of the literature. Stud. Edu. Evaluat. [Epub ahead of print]. doi:10.1016/j.stueduc.2020.100842
Marsh, J. A. (2012). Interventions promoting educators’ use of data: research insights and gaps. Teach. Coll. Rec. 114, 30–48.
Means, B., Chen, E., DeBarger, A., and Padilla, C. (2011). Teachers’ ability to use data to inform instruction: challenges and supports. Report prepared for the U.S. Department of EducationAvailable at: www2.ed.gov/rschstat/eval/data-to-inform-instruction/report.pdf.
Means, B., Padilla, C., and Gallagher, L. (2010). Use of education data at the local level: from accountability to instructional improvement. Report prepared for the U.S. Department of EducationAvailable at: https://www2.ed.gov/rschstat/eval/tech/use-of-education-data/use-of-education-data.pdf.
Ministry of Education (2019). Curriculum, Progress and Achievement. Available at: https://www.education.govt.nz/our-work/information-releases/issue-specific-releases/curriculum-progress-and-achievement-programme/.
Ministry of Education (2011). Ministry of Education position paper: Assessment [schooling sector]. Wellington, New Zealand: Learning Media.
Ministry of Education (2007). The New Zealand Curriculum mathematics. Wellington, New Zealand: Learning Media..
Nelson, T., and Slavit, D. (2008). Supported teacher collaborative inquiry. Teach. Edu. Q. 35 (1), 99–116.
New Zealand Council for Educational Research ZCER. Progressive achievement Tests (PATs). Available at: https://www-nzcer-org-nz.ezproxy.waikato.ac.nz/tests/pats.
Park, V., and Datnow, A. (2008). Collaborative assistance in a highly prescribed school reform model: the case of success for all. Peabody J. Edu. 83 (3), 400–422. doi:10.1080/01619560802222376
Penuel, W. R., Fishman, B. J., Haugan Cheng, B., and Sabelli, N. (2011). Organizing research and development at the intersection of learning, implementation, and design. Educ. Res. 40 (7), 331–337. doi:10.3102/0013189X11421826
Peter, M., Cowie, B., Edwards, F., Eyers, G., and Adam, A. (2017). Beyond pretty charts: From student data to pedagogical action. Available at: http://bit.ly/2q3he1R.
Pierce, R., and Chick, H. (2011). Teachers’ intentions to use national literacy and numeracy assessment data: A pilot study. Aust. Educ. Res. 38, 433–447. doi:10.1007/s13384-011-0040-x
Raffe, C., Alonzo, D., and Loughland, T. (2019). How teachers engage with student assessment data: Understanding antecedents to data-driven decision making. ACER Res. Conf. [Epub ahead of print]. doi:10.13140/RG.2.2.23741.41446
Reeves, T. D., and Honig, S. L. (2015). A classroom data literacy intervention for pre-service teachers. Teach. Teach. Edu. 50, 90–101. doi:10.1016/j.tate.2015.05.007
Ross, E. (2017). State teacher policy yearbook: national summary. Washington, DC: National Council on Teacher Quality.
Ruiz-Primo, M. A., and Furtak, E. M. (2007). Exploring teachers’ informal formative assessment practices and students’ understanding in the context of scientific inquiry. J. Res. Sci. Teach. 44 (1), 57–84. doi:10.1002/tea.20163
Ryan, J., and Williams, J. (2007). Children’s mathematics 4–15: Learning from errors and misconceptions. Maidenhead, UK: Open University Press.
Schildkamp, K., and Kuiper, W. (2010). Data-informed curriculum reform: Which data, what purposes, and promoting and hindering factors. Teach. Teach. Edu. 26 (3), 482–496. doi:10.1016/j.tate.2009.06.007
Schildkamp, K., and Poortman, C. L. (2015). Factors influencing the functioning of data teams. Teach. Coll. Rec. 117 (4), 1–42.
Schildkamp, K., Poortman, C., Luyten, H., and Ebbeler, J. (2017). Factors promoting and hindering data-based decision making in schools. Sch. Effectiveness Sch. Improvement 28 (2), 242–258. doi:10.1080/09243453.2016.1256901
Shepard, L. A. (2019). Classroom assessment to support teaching and learning. ANNALS Am. Acad. Polit. Soc. Sci. 683 (1), 183–200. doi:10.1177/0002716219843818
Shulman, L. (1987). Knowledge and teaching: Foundations of the new reform. Harv. Educ. Rev. 57 (1), 1–22. doi:10.17763/haer.57.1.j463w79r56455411
Spillane, J. P. (2012). Data in practice: conceptualizing the data-based decision-making phenomena. Am. J. Edu. 118 (2), 113–141. doi:10.1086/663283
US Department of Education (2015). Every student Succeeds act (ESSA). Available at: https://www.ed.gov/essa.
Van Gasse, R., Vanlommel, K., Vanhoof, J., and Van Petegem, P. (2020). Teacher interactions in taking action upon pupil learning outcome data: A matter of attitude and self-efficacy? Teach. Teach. Edu. 89 102989, doi:10.1016/j.tate.2019.102989
Van Gasse, R., Vanlommel, K., Vanhoof, J., and Van Petegem, P. (2017). The impact of collaboration on teachers’ individual data use. School Effectiveness and School Improvement. Int. J. Res. Pol. Pract. 28 (3), 489–504. doi:10.1080/09243453.2017.1321555
Vanlommel, K., Van Gasse, R., Vanhoof, J., and Van Petegem, P. (2017). Teachers’ decision-making: data based or intuition driven? Int. J. Educ. Res. 83, 75–83. doi:10.1016/j.ijer.2017.02.013
Visscher, A. J. (2020). On the value of data-based decision making in education: The evidence from six intervention studies. Stud. Edu. Evaluat. [Epub ahead of print]. doi:10.1016/j.stueduc.2020.100899
Volante, L., DeLuca, C., Adie, L., Baker, E., Harju‐Luukkainen, H., Heritage, M., et al. (2020). Synergy and tension between large-scale and classroom assessment: International trends. Edu. Measure. Iss. Prac. 39 (4), 21–29. 10.1111/emip.12382
Wayman, J. C., and Jimerson, J. B. (2014). Teacher needs for data-related professional learning. Stud. Educ. Eval. 42, 25–34. doi:10.1016/j.stueduc.2013.11.001
Wiener, R., and Hall, D. (2004). Accountability under no child left behind. Clear. House 78 (1), 17–21. doi:10.3200/TCHS.78.1.17-21
Keywords: data literacy, data conversation protocol, pedagogical decision making and action, standardized data, mathematics
Citation: Cowie B, Edwards F and Trask S (2021) Explicating the Value of Standardized Educational Achievement Data and a Protocol for Collaborative Analysis of This Data. Front. Educ. 6:619319. doi: 10.3389/feduc.2021.619319
Received: 20 October 2020; Accepted: 15 February 2021;
Published: 12 April 2021.
Edited by:
Dennis Alonzo, University of New South Wales, AustraliaReviewed by:
Divya Varier, George Mason University, United StatesCopyright © 2021 Cowie, Edwards and Trask. This is an open-access article distributed under the terms of the Creative Commons Attribution License (CC BY). The use, distribution or reproduction in other forums is permitted, provided the original author(s) and the copyright owner(s) are credited and that the original publication in this journal is cited, in accordance with accepted academic practice. No use, distribution or reproduction is permitted which does not comply with these terms.
*Correspondence: Bronwen Cowie, YnJvbndlbi5jb3dpZUB3YWlrYXRvLmFjLm56
Disclaimer: All claims expressed in this article are solely those of the authors and do not necessarily represent those of their affiliated organizations, or those of the publisher, the editors and the reviewers. Any product that may be evaluated in this article or claim that may be made by its manufacturer is not guaranteed or endorsed by the publisher.
Research integrity at Frontiers
Learn more about the work of our research integrity team to safeguard the quality of each article we publish.