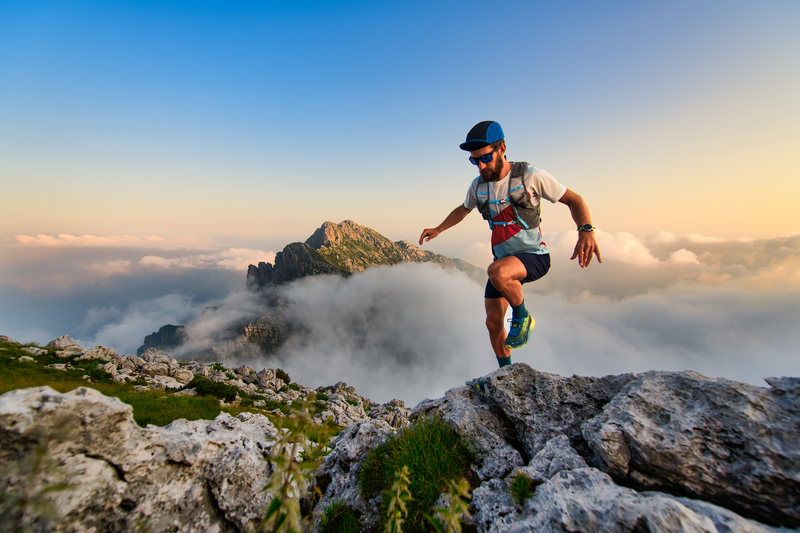
95% of researchers rate our articles as excellent or good
Learn more about the work of our research integrity team to safeguard the quality of each article we publish.
Find out more
ORIGINAL RESEARCH article
Front. Educ. , 19 February 2021
Sec. Teacher Education
Volume 6 - 2021 | https://doi.org/10.3389/feduc.2021.570229
This article is part of the Research Topic Fostering Self-Regulated Learning Classrooms Through Intentional Links Between Conceptual Frameworks, Empirical-Based Models and Evidence-Based Practices. View all 6 articles
Teachers’ ability to self-regulate their own learning is closely related to their competency to enhance self-regulated learning (SRL) in their students. Accordingly, there is emerging research for the design of teacher dashboards that empower instructors by providing access to quantifiable evidence of student performance and SRL processes. Typically, they capture evidence of student learning and performance to be visualized through activity traces (e.g., bar charts showing correct and incorrect response rates, etc.) and SRL data (e.g., eye-tracking on content, log files capturing feature selection, etc.) in order to provide teachers with monitoring and instructional tools. Critics of the current research on dashboards used in conjunction with advanced learning technologies (ALTs) such as simulations, intelligent tutoring systems, and serious games, argue that the state of the field is immature and has 1) focused only on exploratory or proof-of-concept projects, 2) investigated data visualizations of performance metrics or simplistic learning behaviors, and 3) neglected most theoretical aspects of SRL including teachers’ general lack of understanding their’s students’ SRL. Additionally, the work is mostly anecdotal, lacks methodological rigor, and does not collect critical process data (e.g. frequency, duration, timing, or fluctuations of cognitive, affective, metacognitive, and motivational (CAMM) SRL processes) during learning with ALTs used in the classroom. No known research in the areas of learning analytics, teacher dashboards, or teachers’ perceptions of students’ SRL and CAMM engagement has systematically and simultaneously examined the deployment, temporal unfolding, regulation, and impact of all these key processes during complex learning. In this manuscript, we 1) review the current state of ALTs designed using SRL theoretical frameworks and the current state of teacher dashboard design and research, 2) report the important design features and elements within intelligent dashboards that provide teachers with real-time data visualizations of their students’ SRL processes and engagement while using ALTs in classrooms, as revealed from the analysis of surveys and focus groups with teachers, and 3) propose a conceptual system design for integrating reinforcement learning into a teacher dashboard to help guide the utilization of multimodal data collected on students’ and teachers’ CAMM SRL processes during complex learning.
Self-regulated lerning (SRL) necessitates lerners actively and dynamically monitor and regulte their cognitive, affective, metacognitive, and motivational (CAMM) processes to accomplish learning objectives (Azevedo et al., 2018; Winne, 2018). Research has consistently shown that effectively employing SRL processes (e.g., judgments of learning) and strategies (e.g., note taking), improves academic performance, particularly when learning about complex topics and problem-solving tasks (e.g., Azevedo and Cromley, 2004; Dignath and Büttner, 2008; Bannert et al., 2009; de Boer et al., 2012; Azevedo, 2014; Kramarski, 2018; Michalsky and Schechter, 2018; Moos, 2018; Jansen et al., 2019). Teachers’ role in this process is thus to act as active agents in their students’ learning to help introduce and then further reinforce SRL experiences (Kramarski, 2018). This requires that teachers are aware of when these experiences occur and to what capacity (e.g., a teacher recognizing when a student tries to assess their learning in a judgement of learning to determine their next steps vs. a student who fails to and gets “stuck” in their learning). Teacher dashboards could provide a unique opportunity to positively influence teacher decision making in order to foster student’s’ SRL by providing rich and nuanced learning data for a holistic and robust capture of a student’s current state captured through behavioral data (e.g., performance measures, skill practice, etc.), online trace data (e.g., log files capturing navigational trails, metacognitive judgements, strategy use, etc.), and psychophysiological signatures (e.g., eye-tracking, skin conductance, etc.). In this paper, we propose the conceptual system design of MetaDash, a new teacher dashboard that will aid teachers’ instructional decision making by providing process data, behavioral information, and visualizations of their class’ SRL CAMM processes collected through audio and video recordings of the classroom, log files, and psychophysiological sensors. We begin by describing the current successes of grounding advanced learning technologies in theoretical SRL frameworks to highlight the need for a strong theoretical foundation for dashboards. While many cognitive tutors and game-based learning environments have leveraged the theoretical models of SRL to support their development, analytical dashboards have not done the same. We briefly review these gaps to outline the specific elements that should be the focus of the next generation of dashboards. Next we report on findings from an analysis of surveys and focus groups with teachers that point towards some of the important design features and elements within intelligent dashboards that provide teachers with real-time data visualizations of their students’ SRL processes and engagement while using ALTs in classrooms. These perceptions of data visualizations are used in conjunction with our review of dashboard literature to propose the conceptual design of MetaDash integrating reinforcement learning, whose specific features and elements are described.
We begin the conceptual system design of MetaDash with a review and understanding of the current state of ALTs and the collection of multimodal SRL trace data. Advanced learning technologies (ALTs) such as intelligent tutoring systems, game-based learning environments, and extended-reality systems can potentially support and augment learning to help students self-regulate as they monitor and regulate their CAMM processes during academic achievement activities involving learning, problem solving, reasoning, and understanding. The affordances of artificial intelligence (AI) and machine learning allow more complex data streams (e.g., eye movements, natural language, physiological data, etc.) to model learners’ SRL processes and inform ALTs to support and foster CAMM processes. For example, where previous research has used human coding to map students’ SRL processes (e.g., Greene and Azevedo, 2007), natural language processing captured through concurrent think-alouds could be used to inform the system of a student’s current state and possibly suggest help or hints to scaffold their learning, while facial expression recognition could be used to detect frustration or confusion, allowing for intervention prior to disengagement (D’Mello et al., 2018).
The design of ALTs has seen a shift from the traditional pretest-posttest paradigm to include process data and self-report data (Azevedo et al., 2018; Azevedo et al., 2019). Process data are time stamped behavioral traces of an individual’s actions during a learning session, and can be collected from different data channels, such as eye tracking, face videos, log files, and electrodermal activity. It can be beneficial to collect process data because they can be used as indicators of students engaging in different phases of SRL (planning, monitoring, strategy-use, making adaptations) during a learning session, which has been shown to be useful for developing teacher dashboards (Matcha et al., 2019). For example, sequences of short eye-movement durations on different areas of a system’s interface can be indicative of scanning behaviors, and demonstrates planning. If a student re-reads text after displaying facial expressions of confusion, this can demonstrate engaging in metacognitive monitoring and regulating their confusion by adapting their reading behavior and rereading the text. These data can then serve as inputs for teacher dashboards and support the teacher by providing real-time scaffolding to either the student or class (depending on the granularity of the data), or even themselves. For example, while using an e-texbook in a biology class, a teacher would only be able to deduce that a student is reading. However, utilizing eye-tracking or other process data, the teacher could be alerted that a student is viewing a page that is not relevant to their current learning goal, and can have the teacher guide the student to a relevant page, demonstrate how to effectively monitor the relevancy of the page, scan and search content, and then finally suggest a strategy to learn the material. Or in another context, students could demonstrate the use of learning strategies by typing into a virtual notebook where log files document the amount of time spent taking notes, as well as the written content, which can then be scored for different components, such as accuracy. If a student returns to a previously-viewed page, as demonstrated by the log-file data, this can indicate they are making adaptations to their plans by revisiting a content page. Thus, with process data, we would be able to obtain detailed behavioral traces of a student’s actions during a learning session along with contextual information with which to ground those behaviors (Azevedo and Gašević, 2019; Winne, 2019).
Additionally, it is imperative that teachers become strong and proactive self-regulated learners themselves so that they may help their students achieve similar skills and abilities (Kramarski, 2018; Callan and Shim, 2019). Teacher dashboards can provide a platform and analytical tool that would foster the development of SRL within teachers and their students. It would allow them to reflect and monitor data and behaviors otherwise unavailable to them in a traditional classroom Michalsky and Schechter (2018). In sum, while ALTs have been used widely to study SRL in lab and classroom settings, the focus has been on enhancing learners’ CAMM SRL processes. Furthermore, there is a necessity to provide real-time dynamic temporal fluctuations of SRL processes that occur throughout the learning session. This can be achieved with AI-based techniques to provide teachers with the same data these systems use for adaptive scaffolding and to fostering both students’ and teachers’ SRL.
Dashboards can provide analytical data, usually through aggregated visualizations, to provide important information about one’s performances at a glance. One of the defining design elements of these systems that will affect future features is the classification of the end user (i.e., students vs. teachers vs. academic advisors/administration). Previous research on both student and teacher facing dashboards provide valuable insight for the future development of the next generation of theoretically-based dashboards that provide more than just behavioral data (e.g. time on tasks, objectives completed, embedded assessment scores, etc.) but also analytical data on important online trace data (e.g., navigation trails, event recordings, log files, etc.) and psychophysiological behaviors (e.g., facial expression, skin conductance, etc.). To begin our review, we conducted a literature search in eight databases for peer-reviewed empirical articles and conference proceedings about teacher dashboards and how they are being designed and utilized. These databases included the University of Central Florida’s Library, IEEE Xplore, Science Direct, LearnTechLib, ACM Digital Library, Springer LINK, ProQuest Social Sciences, PsycInfo, and Web of Science. Our search consisted of a variety of synonyms for “teacher dashboard” including learner analytics dashboard, instructional panel, teacher interactive dashboard, educational dashboard, learning dashboard, data visualization dashboard, and learner progress monitoring. Our search resulted in 5,537 peer-reviewed articles in the past ten years. Out of those results, our team found that 53 of them were highly relevant to our project. Additional literature was collected informally to help supplement specific queries. The learning analytics community has focused most efforts around teacher dashboards using online trace data on Massive Open Online Courses (MOOCS; Verbert et al., 2013) and Intelligent Tutoring Systems (Bodily and Verbert, 2017; Holstein et al., 2017), but have neglected augmenting and supporting learning management systems (i.e., virtual environments that support e-Learning) utilizing these data streams. Learning management systems have been transforming from early detections systems, or alert systems, designed for academic advisors to categorize at risk students (Dawson et al., 2010; Arnold and Pistilli, 2012; Krumm et al., 2014; Schwendimann et al., 2018)into student-facing systems that provide students with their performance data directly (Teasley, 2017, e.g., Blackboard, Desire2learn, Canvas, etc.). In the same way that many intelligent tutoring systems have used trace data to provide personalized feedback (Azevedo et al., 2019), we posit that teacher facing dashboards should leverage the same type of data streams to provide a more informative set of analytical visualizations and alerts of student performance. That is, more focus should be on collecting, sharing, and providing teachers’ with more complex CAMM SRL learner process data that go beyond than sharing easily accessible behavioral data (e.g., time on tasks, objectives completed, embedded assessment scores) that fail to illustrate the complexities of SRL that contribute to learning.
Within student facing dashboards and learning management systems, students can be provided autonomy over their learning by having the systems encourage intrinsic motivation through progress self-monitoring (e.g., Scheu and Zinn, 2007; Santos et al., 2013; Dodge et al., 2015; Beheshitha et al., 2016; Gros and López, 2016) Bodily and Verbert (2017) conducted a systematic review of student-facing dashboards and found that the most prevalent systems were enhanced data visualizations (included a class comparison feature or an interactivity feature) or data mining recommender systems (recommended resources to a student). The review recommended that future research should be focused on systems that target the design and development of reporting systems and not the final products.
Not all research, however, has supported the effects of student-facing dashboards. For example, a systematic review by Jivet et al. (2018) found that the current designs of dashboards employ a biased comparison frame of reference to promote competition between learners rather than content mastery. These features included performance comparison to peers and gamification elements (e.g., badges, leaderboards, etc.). Specifically, these types of features) support the “reflection and self-evaluation” phase of self-regulated learning (SRL; e.g., Schunk and Zimmerman, 1994) while neglecting the other aspects. Furthermore, it raises the question if supporting self-evaluation that focuses on extrinsic motivation and performance goal orientations really supports effective SRL? Additionally, Jivet et al. (2018) highlight that current dashboard evaluation is based on the systems as software instead of focusing on their pedagogical impacts and suggest future research should start to evaluate how the systems affect student goals, affect, motivation, and usability. Other researchers have echoed this concern, arguing that too much of the current research is exploratory and proof-of-concept based (Schwendimann et al., 2018).
Bodily et al. (2018) conducted a systematic review, focusing on analytic dashboards in open learner models (OLMs), which, like student facing dashboards, can provide students with their learning analytics. They reported that 60% of these new models are based on a single type of data, only 33% use behavioral metrics, 39% allow for user input, and just 6% encompass multiple data streams. Their research suggests that OLMs are likely to be interactive but are less likely to utilize behavioral metrics or multiple channels of data. Given that OLM’s have high levels of interactivity and larger solution spaces than closed problems, OLMs, similar to simulations, have unique challenges in providing data. Only recently has research begun to explore the use of simulations to help provide low-risk practice for teachers or to augment student learning (López-Tavares et al., 2018). These systems provide valuable insight on data visualizations, while failing to capture and report on multiple aspects of student learning.
Prior research on teacher dashboards has focused on the data visualizations of student performance data, addressing the state and federal mandates for data use in the classroom (e.g. the Every Student Succeeds Act of 2017, 20 USC 7112). Research and design on teacher dashboards that give access to learner analytics on both the individual student and aggregate class level has been growing in popularity to empower teachers with quantifiable evidence of student learning and performance (Matcha et al., 2019). While the majority of teacher dashboards do not include SRL-type process data (e.g., strategy choice, metacognitive judgements, motivation orientations, etc.), there is emerging evidence that some are starting to capture and create data visualizations of learning through activity and behavioral data, with the goal of promoting SRL for students and monitoring tools for instructors (e.g., Matcha et al., 2019; Molenaar et al., 2019). Teaching analytics have been used to support student decision making, reflecting, and drawing awareness to student issues (e.g., misconceptions, disengagement, etc.; Calvo-Morata et al., 2019; López-Tavares et al., 2018; Martinez-Maldonado et al., 2013; McLaren et al., 2010; Tissenbaum et al., 2016; Vatrapu et al., 2011) but less on how to use this data to inform teacher instructional decisions. That is, many of the existing systems have been designed to support students without involving teachers (Baker and Yacef, 2009; Ferguson, 2012; Koedinger et al., 2013; Martinez-Maldonado et al., 2015) despite the fact that teachers play a critical role in the classroom as orchestrators of learning activities and feedback providers (Hattie and Timperley, 2007; Dillenbourg et al., 2013; Roschelle et al., 2013; Martinez-Maldonado et al., 2015). These systems help augment (and in many cases become the primary source of) feedback (Verbert et al., 2013), but fail to support teachers’ instructional decision making about what, how, and when to introduce certain material or revisit previously introduced material. However, there are still many systems that have sought to directly address instructional decision making. For example, Martinez-Maldonado et al. (2015) provided support to teacher decision making about the timing and focus of feedback delivery and argued that systems should not look to replace teachers, but rather support them. However, their work did not explore other types of support for instructional decision making (e.g., activity introductions, misconception alerts, strategy suggestions, etc.) outside of feedback. Wise and Jung (2019) identified four major approaches in the current work on how teachers utilize learning analytics in their decision making- 1) teacher inquiry for self-design of professional growth; 2) learning activity design and development considering student engagement; 3) analytic use in real-time; and 4) institutional support for understanding and using analytics. The authors highlight a need to organize and align analytics with pedagogical perspectives. We argue this gap can be addressed by grounding systems within learning theories (e.g., SRL) which will position analytics less in the abstract and more reflective of student learning.
Previous research has provided invaluable information about the effectiveness of various design features for student and teacher facing dashboards. However, in the next generation of these systems, we highlight key elements that should be addressed and studied with the ultimate goal of providing a more holistic and complete indication of learning. First, these systems and their respective design features need to be theoretically grounded within learning theories (e.g., self-regulated learning). Many of the current systems have neglected the role that motivation, engagement, and metacognition have on SRL. Furthermore, empirical evidence suggests that many current design features (e.g., gamification or competition driven elements) are inadvertently negatively impacting these key facets of SRL. Systems have also been heavily reliant on performance and less on process. Second, the information provided to both students and teachers should be informed by more than just behavioral data (e.g., mouse clicks, time on task, etc.). Online trace data (e.g., self-reported metacognitive judgments, attention and rereading as captured through eye-tracking, etc.) and psychophysiological behaviors and responses (skin conductance as evidence of arousal, pupil dilation as evidence of interest, etc.) should help provide a more robust and complete picture of student learning. Online trace data has proven to be important data streams for other ALTs and should also be used to inform analytical dashboards. Finally, as many researchers have already suggested (e.g., Bodily and Verbert, 2017; Schwendimann et al., 2018), we echo the sentiment that research conducted on dashboards should shift a focus from proof-of-concept and exploratory towards the theoretical implication and design as well as the empirical evaluation of these systems. As such, we propose the conceptual development of MetaDash to begin to address these gaps in conjunction with additional features and design choices that will be further supported by a brief synthesis of findings from a survey study and focus groups meetings with teachers.
In parallel with the conceptual system design considerations (see MetaDash: A Teacher Dashboard for Fostering Learners’ SRL), we have begun analyzing the needs and perceptions of teachers to help guide the feature design of a future dashboard that is based on learners’ real-time CAMM SRL processes and could be utilized for in-the-moment instructional decision making and post-lesson reflection. Teachers’ perceptions were important for us to collect and analyze so that the conceptual design would be aligned with real-world constraints and needs in order to be useful and accessible in real classrooms (Simonsen and Robertson, 2012; Bonsignore et al., 2017; Holstein et al., 2017; Prieto-Alvarez et al., 2018). As we move into future iterations of MetaDash, it will be vital that teachers continue to be co-designers of the system to avoid common problems of providing complex learning analytics to individual educators with various levels of data literacy (e.g., misrepresentations of learners’ and teachers’ needs, steep learning curves, etc.; Gašević et al., 2016; Prieto-Alvarez et al., 2018) Teachers’ perceptions of dashboard designs, student engagement, and role of student emotions during learning were collected via a survey distributed to 1,001 secondary science teachers in a Southeastern state (Kite et al., 2020). One hundred four (N = 104) completed surveys were received and the responses were analyzed using both qualitative content analysis (Mayring, 2015) and the constant comparative method (Corbin, 1998). We also conducted two 2-h focus groups with a guidance counselor and seven middle school teachers from multiple disciplines (i.e., remedial math, civics, biology, chemistry, social studies, and English) at a Southeastern school.
The survey provided teachers with two different data visualizations (See Figures 1 and 2) of student emotions and asked them to write about the ways that each data visualization might impact their instruction or lesson planning. Given the critical role of emotions in academic achievement (Linnenbrink-Garcia et al., 2016) and ALTs such as game-based learning environments (Loderer et al., 2018), the first data visualization depicts real-time data of six different emotions (i.e., joy, frustration, anger, contempt, surprise, and confusion) for a single student engaged in a learning task (see Figure 1). The second data visualization depicts the emotional progressions of the class drawn from aggregated student data for the six emotions over the course of the class period (see Figure 2).
FIGURE 1. Data visualization depicting real-time data of six different emotions (i.e., joy, frustration, anger, contempt, surprise, and confusion) for a single student engaged in a learning task.
FIGURE 2. Data visualization depicting the emotional progression of the class drawn from aggregated student data for the six emotions over the course of the class period.
Two themes emerged from the analysis of the survey: 1) Teachers viewed both data visualizations of student emotions as being potentially valuable tools for lesson design, evaluation, and revision, 2) Teachers viewed real-time data visualizations of individual students’ emotional data as potentially valuable tools to prompt teachers to use specific strategies to reduce negative student emotions.
With regards to the first theme, teachers highlighted lesson planning, evaluation, and revision as potentially useful applications of both the real-time emotional data visualization for individual students and the aggregated emotional data for the class over the course of the task. More than 70% of teachers’ responses relating to the class-level data visualization fall under this theme. Specifically, teachers believed that the class-level data would be most valuable for 1) lesson planning/design (29.7% of quotations) and 2) lesson evaluation (19% of quotations). Commenting on the class-level data visualization, one teacher remarked that “knowing when a lesson is frustrating or confusing and ‘when’ exactly it happened would be crucial information to have to alter that lesson for the future.” While another respondent stated that “Over the course of a class period (1.5 h long) it is hard to see what is working and what is not. It would be great to see how my students [sic] emotions change throughout a lesson and be able to adjust and grow as a professional.” Many teachers’ reactions to the real-time data visualization of an individual student’s emotions centered on the data visualizations as tools to provide information to shift their pedagogy or data to personalize instruction, as indicated in the following excerpt, “Movement of the lesson and timing would be impacted. The level of confusion and frustration would guide whether to move the student on to higher level activities, or to review or come up with a completely different lesson.”
In addition to viewing student emotional data visualizations as tools for lesson planning and revision, many teachers reported in the survey that they believed the data visualizations of individual students’ emotional data could be highly valuable for prompting in-the-moment interventions to reduce negative student emotions. For example, one teacher stated that data visualizations of individual student’s emotions would “help me make changes ‘on the fly’ when the distribution of emotions of students skew to the negative side.” While another reflected that “If my students are confused or frustrated during a lesson, I will stop what I am doing and reassess how I am teaching and start over with a new approach.” Many teachers echoed the sentiments that these real-time data visualizations could be particularly helpful for “identifying students who quietly sit and never ask questions” and to “better target which students need additional support.” While most teachers discussed the utility of these tools with regards to “typical” students, one teacher mentioned that data visualizations of individual student’s emotions could be particularly beneficial for teachers of English Language Learners and another noted that this type of data could be useful for teachers of students with Autism Spectrum Disorder (ASD). Describing specific strategies to counter negative student emotions, teachers proposed ideas such as implementing more hands-on experiences, better connecting the material to students’ lives, implementing more robust learning scaffolds, and initiating emotional education.
Similar opinions were echoed by a group of eight middle school teachers from a southeastern school who participated in a 2-h focus group about their perceptions of student engagement and teacher dashboard design elements. One sixth grade civics teacher remarked that they would use individual data on students’ emotions as a reflective tool for future lesson planning but would rely on the aggregate class-level emotional data for real-time intervention and instructional decision making. Conversely, a remedial math teacher with a small classes (around five students per class) commented that individual level data would “…be better for in the moment. Like what is happening with that kid that is keeping them from learning? Keeping kids around them from learning?” This suggests that high flexibility in the aggregation and personalization of the dashboard is necessary to address the various disciplines, teacher needs, and class compositions. Just as learning should not be approached with a “one-size-fits-all” mentality, teacher dashboards and tools should not either.
Further supporting this idea, we found that the three science teachers who participated did not particularly want or trust emotional student data. They all agreed that they felt competent in identifying their classroom’s emotions, however having “objective data such as heart-rate would be useful for evidence during reflection”. Interestingly, the non-STEM teachers and counselor all showed reluctance to use any data visualizations more complex than a simple bar-chart or pie-chart of aggregated data while STEM teachers wanted the raw, or nearly-raw, data during reflection. Going forward, it is important to further explore data literacy among teachers and common misconceptions that may occur in the interpretation of data provided to teachers from a dashboard.
Based on both the survey and focus group data, it is vital we consider the end-user (i.e., teachers) needs and desires for a dashboard during the design of the features and elements for a new dashboard. There are general considerations from both a practical use standpoint (as indicated from teachers) and a theoretical standpoint (as indicated from prior research) that need to be taken into account: 1) dashboard audience (i.e., student vs. teacher facing), 2) dashboard modality (i.e., mobile vs. desktop), 3) aggregation levels (i.e., student vs. small group vs. class levels), 4) analytical and data sources (e.g., performance measures, CAMM processes, etc.), and 5) types of data visualizations (e.g., static bar/line graphs vs. dynamic time-series plots). Our initial analysis from these two data sources suggest that high levels of personalization is needed across most of these items. These data were used to design our initial prototype teacher dashboard, MetaDash, and are described in the next section.
While multiple frameworks have been proposed for the design and development of teacher dashboards [e.g., the LATUX framework (Martinez-Maldonado et al., 2015), causal chain frameworks (Xhakaj et al., 2016), etc.], none of them have used theoretical models and empirical evidence of SRL to guide feature development. Below, we propose the development of MetaDash, a teacher dashboard that will aid teachers’ instructional decision making by providing process data, behavioral information, and visualizations of their class’ SRL CAMM processes collected through audio and video recordings of the classroom, log files, and psychophysiological sensors. In this section, we explicitly describe MetaDash’s design elements and their grounding in Winne (2018)’s Information Processing Theory (IPT). This conceptual design helps address many of the gaps raised within the literature (i.e., 1) need for theoretical grounding; 2) use of event-level online trace data in conjunction with behavioral performance data; and 3) evaluation beyond proof of concept) as well as the design elements from the themes raised in results of our survey and focus groups (i.e., 1) use of data visualizations of student emotions as tools for lesson design, evaluation, and revision; 2) real time student emotion data as tools to address negative emotion on the fly; and 3) need for high personalization across dashboard audience, modality, aggregation levels, analytical and data sources, and visualization type).
According to the IPT model of SRL, learning occurs within four cyclical and recursive phases: 1) defining the task, 2) goal setting and planning, 3) enacting study tactics and strategies, and 4) metacognitively adapting studying. It is important to highlight that SRL does not necessarily unfold temporally from phase 1 to phase 4 before returning to phase 1 in a set sequential pattern, but rather information generated during each phase could prompt any other phase or jump back into the same phase. Additionally, occurring throughout these four phases are five different components of tasks which can be used to define and provide context. These include 1) Conditions, 2) Operations, 3) Products, 4) Evaluations, and 5) Standards (COPES).
Our conceptual design begins with the theoretical grounding of the system within Winne’s model of SRL. This model includes several assumptions that are aligned with the design of MetaDash. These assumptions include the 1) view that CAMM SRL processes unfold over time as learners monitor and regulate their CAMM processes while using ALTs to learn and that these processes can be captured in real-time using several trace methodologies, techniques, and sensors; 2) these processes can be tracked, modeled, and inferred using statistical and other AI-based, machine learning techniques e.g., reinforcement learning) and can be fed back to learners and teachers in ways that are meaningful to each group of users; 3) these data can be fed back to learners by providing adaptive scaffolding and feedback using a variety of instructional methods (e.g., prompting by pedagogical agent to monitor one’s learning) to foster and support their SRL; and 4) these data can be fed back to teachers (as in the use of MetaDash) to provide actionable data visualizations of learners’ CAMM SRL data at various levels of granularity to enhance teachers’ instructional decision making.
Below, we describe the features of MetaDash using two examples of vastly different classrooms, based on the four phases of Winne’s model described above. The first, in a traditional lecture style physics classroom in which students are first introduced to and learn about simple free body diagrams. This classroom has well-structured problems and face-to-face instruction with traditional lecturing. The second is a blended classroom (i.e., online and in-person instruction) tackling American history, where students are specifically being asked to find and use primary documents and resources to discover how state and local governments used Jim Crow laws to restrict newly gained freedoms after the Civil War. Unlike the physics classroom, the American history class has more ill-defined problems and exploratory lessons.
During phase 1, defining the task, students identify and outline their understanding of the tasks and goals to be performed. Within the MetaDash dashboard, these tasks could be explicitly defined for both the student and the teacher based on lesson plans or the local curriculum. While this particular lesson might be a component of a larger semester goal, there are still levels of task definitions that need to be defined (i.e., task conditions) such as the amount of time dedicated to the lesson and instructional resources available for the lesson. Most educational programs already require teachers to document their lesson plans, and curricula are often set statewide. This proposed dashboard, therefore, would not require any additional work, just a new destination where teachers are asked to report their plans. Within our physics classrooms, the dashboard might say that the curriculum goal is to “Solve basic force problems” while the current lesson goal is to “Label the parts of a free body diagram”. The first goal is a larger component that multiple lessons will cover while the lesson goal has a clear and defined product that students can do within a single lesson. Task definition, goal setting, and planning would belong to the preparatory phases of SRL and are the most critical components of SRL (Panadero, 2017). Also, depending on the task and type of instruction (e.g., student-centered or teacher-centered), defining the task may involve substantial teacher-student interactions that will be critical for the next phase of goal setting and planning (Butler and Cartier, 2004). It is also important to highlight that task definitions may need to be altered or modified at any point during learning and teaching. A dashboard, such as MetaDash, will need to be capable of tracking these changes (e.g., when, what, how, and potentially why was the definition of the changed or altered?). Similar to the work of Martinez-Maldonado et al. (2015), feedback about the current state of one’s tasks should be provided through teacher intervention in conjunction with system production rules. Allowing teachers to set these goals and plans ensures that static production rules do not limit the flow of learning activities or constrict teachers’ ability to orchestrate student learning but instead supports their ability to unobtrusively generate explicit tasks both preemptively and on the fly. Furthermore, by allowing direct input from teachers, this dashboard does not lock teachers into a set lesson developed outside of the classroom. Instead, it allows for high personalization, a theme that was highlighted in our survey and focus groups from teachers.
During Phase 2, goal setting and planning, students generate specific goals, sub goals, and plans to achieve these goals. For example, in our physics classroom, students might first want to learn what are the components to be labeled within the free body diagrams (i.e., what do the arrows represent, what are the planes, what do features of the arrows such as their length mean) while in the blended history classroom, goals might be less defined and exploratory such as collecting relevant looking documents, identifying key challenges, or researching specific laws.
Research suggests that systems that offer students the ability to control their instructional options and goals based on various types of choices and preferences might not be effective because students lack effective metacognitive skills that would support successful choices (Scheiter and Gerjets, 2007; Clark and Mayer, 2008; Wickens et al., 2013; Chen et al., 2019). Therefore, MetaDash will have an explicit goal and task tracker that will serve as a roadmap for learning. By making these goals explicit and easily available, students and teachers will be better able to orient their learning and center it around a specific task. This feature is directly supportive of multiple principles of Mayer, (2014, in Press) cognitive theory of multimedia learning (CTML). Specifically, it is supported by the (a) guided discovery, (b) navigational, and (c) site map principles. These state that learning is improved and most effective when (a) there is some guidance during self-discovery, (b) appropriate navigational aids improve learning, and (c) there is a map of where one is within a lesson (Mayer, 2014, in Press).
This would be a key phase at which disengagement or maladaptive learning could be alerted to the teacher. For example, if a student fails to set any goals or identify the current task, the dashboard might alert the teacher which students are off task or fail to set goals. This would allow for intervention by the teacher in a more directed manner and would avoid the unnecessary additional work of manually checking each student’s goals and plans; adhering to the signaling principle (i.e., learning is improved when cues highlight essential material; Mayer, 2014, in press). Additionally, this would also allow for different levels of goals to be set to help individualize learning for each student’s capabilities. While one student might be able to identify relevant primary sources immediately, others might first want to revisit and define what makes a source primary vs. secondary. Furthermore, individualization of goals would support students with various goal-orientations while providing this insight to teachers. That is, one student is motivated to learn to gain mastery over a specific subject while another student is driven by competition and to perform well comparatively to their peers (Elliot and Murayama, 2008). Armed with this information, teachers would be able to directly address motivational issues or provide opportunities to help students set different types of goals and shape their goal-orientations.
The underlying mechanism that will dictate when the system should ideally alert the teacher to support a student’s goal setting and planning will be established through data-driven approaches and the application of Reinforcement Learning (RL) techniques. RL offers one of the most promising approaches to data-driven pedagogical decision making for improving student learning, and a number of researchers have studied applying RL to improve the effectiveness of pedagogical agents (Chi et al., 2011; Mandel et al., 2014; Rowe and Lester, 2015; Doroudi et al., 2016; Doroudi et al., 2018; Shen and Chi, 2016; Holstein et al., 2017). In this prior research, we formulates student learning as a sequential decision process in which RL would provide constant guidance to determine the best action for the students to take in any given situation so as to maximize a cumulative reward (often the student learning gains). Herein, to learn the optimal strategy of goal setting by RL, we model the states as the learning environment features, the rewards as the students’ learning gain, and the actions as the setting of different goals. Under a certain state, if a student did not follow the optimal goal indicated by RL, the dashboard will give alerts so that the teachers can take proper actions to assist students in planning. Martinez-Maldonado et al. (2015) have shown that teachers who take advantage of notifications about group activities were able to make informed decisions about who to attend to, which in turn resulted in higher performance and misconception reduction. Extending this work, our alert systems would allow for more than just feedback about performance to be addressed, but rather attempt to categorize goal setting and planning before students engage in inefficient study. Additionally, by creating an alert system that notifies the teacher about their students’ goals and planning, they still have the autonomy to either further investigate the context to assess if they believe intervention is needed, or if they should continue on as normal. The need for this type of flexibility was brought up in our surveys and focus groups by teachers discussing the potential use of dashboards in real time.
During phase 3, enacting study tactics and strategies, students employ their chosen strategies to attempt to reach their goals. This can range from simple processes such as reading and taking notes to more cognitively demanding strategies such as self-quizzing or creating self-models of new and prior knowledge. For example, within our physics classroom, students might begin by annotating the simple diagram with notes from the teacher’s own expert model or their textbook. They might then cover up specific labels and see if they can remember details about them to explain to a peer. Within our blended classroom, students might begin by conducting literature searches using lists of key phrases or words. Other students might choose to watch videos and take notes of key pieces of information.
Mapping and capturing strategies in classroom settings is difficult due to the myriad strategies available to students, especially across multiple disciplines and domains. Therefore, a main feature of MetaDash would be a “strategy toolbox” for teachers and students to have as an explicit model of their metacognition. Essentially, learners would have a set of strategies, examples of those strategies being modeled, and expert and self-descriptions of those strategies. These would be developed from a base set of strategies that students could add self-examples or notes about in addition to “building their toolbox” by adding new strategies and tactics as they develop within the classroom. For example, within the base toolbox, a strategy might be “Take Notes from Lecture”. A student could choose this as a tactic and be offered hints about how to organize those notes (e.g., Cambridge style), resources to consult for notes (e.g., textbook or videos provided by the teacher), or annotations they added from the last time they took notes (e.g., “use color to differentiate topics”). More complex and specific tactics could be added in directly, such as the steps for approaching a force problem in physics as defined by the teacher (e.g., first draw a free body diagram). This toolbox would be carried from classroom to classroom and grow as students face different types of problems. It serves as an explicit and dynamic representation of the metacognitive processes they have that helps eliminate the need to implicitly search for the appropriate strategy. The different metacognitive judgments are being made with different inputs from various monitoring processes as they are made at different times during the acquisition, rehearsal, and retrieval phases of learning (Nelson and Narens, 1990).
Strategies and resources could be directly tracked and monitored from the dashboard to help alert the teacher to optimal strategy use, suboptimal strategy use, as well as strategy misuse or disuse. In other words, the dashboard could be used to help identify if a student has been spending too long copying notes verbatim without elaboration or idea construction. Furthermore, it could make suggestions directly to the students and teacher in order to prompt for deeper strategies such as content evaluation of specific sources being used, or self-quizzing opportunities. This is both feedback on their current tactics as well as a set of standards to which the student can compare themselves objectively to. The dashboard would also provide an in-depth report of strategy use on a class level to help the teacher identify what strategies students tend to use, or which strategies prove to be more successful than others for specific tasks. Over time and use of MetaDash, the system will also become less reliant on the direct reporting of strategies.
Reinforcement learning (RL) used in computer science can be employed to help students identify the more successful strategies while alerting teachers to the students’ suboptimal strategies. Specifically, our RL agent will determine what is the optimal action for a student to take; then by monitoring the students’ actual behavior and matching it to the optimal action, the suboptimal behavior can be identified and reflected on the dashboard. Herein, to learn the optimal strategy by RL, we model the states as the learning environment features, the rewards as the students’ learning gain, and the actions as the simple processes during learning, such as reading or notes taking. The goal is to utilize RL to advise MetaDash and the underlying system to support teachers to make effective instructional decisions based on student engagement or maladaptive behaviors. For example, within the physics classroom, if the optimal student action would be to label the diagram, but the student immediately begins reading, the system might alert the teacher to prompt a reflection of previous approaches to similar problems, or ask for a metacognitive judgment to be made about what the student believes they need to learn.
While each instructional decision may affect the students’ learning, our prior work showed that some decisions might be more important and impactful than others and thus we can design our dashboard so that teachers will not be alerted unless the student makes a suboptimal choice on a critical decision. The critical decisions can be identified based on the Q-values (Ju, 2019; Ju et al., 2020). The intuition is that, when applying RL to induce the optimal policy, we generate the optimal value function Q*(s,a). For a given state s, a large difference between the optimal state-action maxa Q(s,a) and the remaining Q-value functions indicates that it is more important for the agent to follow the best action in the state s. Simply, if a state-action pair (s,a) is identified as critical while the student does not follow the action in that state, the system should alert the teacher to consider making instructional choices to guide the student towards that action therefore improving learning; otherwise, the alert will not pop up. This method can avoid frequent teacher distractions by unimportant messages on the dashboard and enable them to only focus on the most critical guidance.
In addition, to identify students’ strategies as a means of providing personalized interventions, we will apply Inverse Reinforcement Learning (IRL). IRL can automatically learn a policy directly from learning trajectories, which is more suitable for modeling the students’ learning process, especially their self-regulated learning strategies. Specifically, IRL learns students’ strategies by first inferring the reward function from their learning trajectories. Once the reward functions are inferred, IRL will induce the students’ strategies following the normal RL process. Rafferty et al. (2016) applied IRL to assess learners’ mastery of some skills in solving algebraic equations. Based on the inferred reward function, some skills misunderstood by the learner were detected and then personalized feedback for improving those skills were rendered accordingly (Rafferty et al., 2016). However, most IRL methods are designed to model the data by assuming that all trajectories share a single pattern or strategy. In our work, we will take student heterogeneity into consideration by assuming students’ strategies vary during complex self-regulated learning, given that the decisions are generally made based on a trade-off among multiple factors (e.g., time, emotions, difficulty of content, etc.). To capture the different self-regulated strategies among students, Yang et al. (2020) employed an expectation-maximization IRL to model the heterogeneity among student subtypes by assuming that different student subtypes have different pedagogical strategies and students within each subtype share the same strategy. Results indicated the potential of more customized interventions for different subtypes of students. By leveraging the rewards inferred from IRL to model the students’ decision-making process, we can recognize the subgroups of students who follow suboptimal or misused strategies and alert teachers to the need for an intervention. Similar to the goal setting alerts, this feature (strategy alerts) is supported by the signaling principle Mayer (2014) and a body of scaffolding and modeling literature that suggests optimal strategy use during SRL leads to increased performance (e.g., Azevedo and Cromley, 2004; Dignath and Büttner, 2008; Bannert et al., 2009; de Boer et al., 2012; Azevedo, 2014; Kramarski, 2018; Michalsky and Schechter, 2018; Moos, 2018; Jansen et al., 2019). Additionally, it supports the idea that systems should augment and support teachers, not replace them (Martinez-Maldonado et al., 2015). Teachers will be provided information to make more informed decisions in the moment without having to solely rely on performance data after the fact.
Finally, in phase 4, adaptation, students make adaptations to their approach based on newly acquired information or triggered conditional knowledge. For example, the students studying the physics free body diagrams might recognize the familiar X–Y coordinate system from their geometry and algebra classes and begin to label those axes prior to looking at their textbook. Other students learning about Jim Crow laws might realize the article they are reading is about modern slavery in the early 2000s and conclude it is not relevant to their current task. This would lead them to discarding that source and searching out more relevant articles. MetaDash, therefore, would help teachers and students identify places where standards of learning could be assessed. Self-reports could be directly administered and pushed out to students for feedback about their interest levels and to help alert teachers to growing levels of disengagement. Facial recognition of emotions detecting higher than normal levels of frustration or confusion might also help suggest new strategies to students, which would move them from phase 3 into phase 4 and ideally back into a more optimal phase 3 with newly defined tactics and strategies or phase 2 for more effective goals and plans to enact those strategies. This evaluation tool would allow for explicit reflection on their progress towards their goal without relying purely on the performance-based measures that are the center of contemporary dashboard design.
As students transverse the four phases, MetaDash would help monitor and quantify students’ task conditions, cognitive conditions as measured through self-reports, performance measures, and behaviors, student and expert standards, and the products that, during traditional learning, are hidden from teachers. In other words, unlike previous dashboards, this proposed system will consider students’ COPES (Winne, 2018) and illustrate them with dynamic and adaptable visualizations and the evidence of these aspects. First, task conditions will be contextualized through audio and video recordings of the lesson. This is essential for the teacher to be able to self-reflect on a particular lesson or return to in case there is a need to revisit why a particular lesson was successful or not. For example, if the dashboard alerts the teacher that a student has become disengaged during a lesson, they would be able to watch what that student was doing just prior and might see high levels of frustration and confusion about a specific topic. This would allow them to mitigate negative emotions on the fly, which is an important tool that teachers revealed would be useful in our survey.
Additionally, the system will have a marking system that is directly tied to the video and audio recordings for the dashboard. Given the myriad demands for teachers’ attention, teachers are unlikely to be able to stop their lesson to make a note of something occurring within the classroom. By allowing teachers to quickly mark important events with predefined keys or buttons, the dashboard will allow them to revisit that moment for further reflection or tagging. For example, if during a student presentation on their primary sources the teacher notices other students perking up at the mention of voting restrictions, they might quickly press a button they have assigned to mean interest. The system will then be able to point them back to that moment when they are reviewing their lesson for future instructional planning. This helps reduce the cognitive load of trying to remember everything that happens within a single lesson, while also highlighting specific cues of moments within a lesson (i.e., the signaling principle; Mayer, 2014).
Dashboards can provide both a platform and an analytical tool to support instructional decision making through data analytics and visualizations. Specifically, this information could be leveraged to help teachers self-regulate their own learning to help their students develop and practice the same skills and processes. However, the current state of dashboards for a variety of learning contexts (e.g., MOOCs, ITSs, learning management systems, etc.) has primarily focused on exploratory or proof-of-concept projects, relied heavily or entirely on data analytics and visualizations of behavioral traces or performance metrics, and neglected most theoretical aspects of SRL. This paper has addressed these gaps by grounding the development of MetaDash within Winne and colleagues’ (2018) Information Processing Theory of SRL and empirical evidence collected from surveys and focus groups. Our work suggests that teacher dashboards, such as MetaDash, should take in several considerations about the features and data visualizations that are available for teachers. These include dashboard audiences, modality, aggregation levels, analytical data sources, and types of data visualizations. Importantly, personalization and flexibility across these specific dimensions is vital for the development of a dashboard that teachers will be able to effectively use in multiple types of classrooms. Additionally, these features should be able to capture, view, and subsequently model CAMM SRL processes in real time. This information is not only to be fed back to the students and teachers to help enhance teachers’ instructional decision making, but also into the system to help create adaptive and intelligent alerts and scaffolding. In other words, the system will be helping to regulate student CAMM SRL processes alongside teachers within the classroom by drawing attention to specific traces and data in real time.
Our ongoing work will focus on the technical challenge of the physical development of MetaDash that will help serve as the tool to empirically examine the effects of teacher dashboards and the support of teacher CAMM SRL processes on their students’ own processes within a classroom. This will include exploring various types of classrooms (STEM, social sciences, blended, etc.) and how teachers use data analytics, data visualizations, and suggestions from MetaDash to augment or support their instructional decision making in both real-time and prospectively. Further research will explore how teacher dashboards can help students’ learning and achievement within the classroom.
The raw data supporting the conclusions of this article will be made available by the authors, without undue reservation.
The studies involving human participants were reviewed and approved by the Ethics Committees of both the University of Central Florida and North Carolina State University. The participants provided their written informed consent to participate in this study. Written informed consent was obtained from the individual(s) for the publication of any potentially identifiable images or data included in this article.
MW took the lead in writing the majority of the manuscript under the supervision of RA. MW and VK collected the data, analyzed, and discussed the data in relation to the survey results and focus groups, theory, and framework for the design of the system. XY analyzed the data and contributed to the writing of the reinforcement learning framework. SP, MC, MT, and RA provided extensive feedback and they each contributed to different sections of the manuscript.
This manuscript was supported by funding from the National Science Foundation (DRL#1916417). Any opinions, findings, conclusions, or recommendations expressed in this material are those of the author and do not necessarily reflect the views of the National Science Foundation.
The authors declare that the research was conducted in the absence of any commercial or financial relationships that could be construed as a potential conflict of interest.
The authors would like to thank members of the SAMRT Lab at the University of Central Florida. We also thank the teachers in North Carolina and Florida that participated in our project.
Arnold, K. E., and Pistilli, M. D. (2012). “Course signals at purdue: using learning analytics to increase student success”, in Proceedings of the 2nd international conference on learning analytics and knowledge, 267–270.
Azevedo, R., and Cromley, J. G. (2004). Does training on self-regulated learning facilitate students' learning with hypermedia? J. Educ. Psychol. 96, 523. doi:10.1037/0022-0663.96.3.523
Azevedo, R., and Gašević, D. (2019). Analyzing multimodal multichannel data about self-regulated learning with advanced learning technologies: issues and challenges. Comput. Hum. Behav. 96, 207–210. doi:10.1016/j.chb.2019.03.025
Azevedo, R. (2014). Issues in dealing with sequential and temporal characteristics of self- and socially-regulated learning. Metacognit. Learn. 9, 217–228. doi:10.1007/s11409-014-9123-1
Azevedo, R., Mudrick, N. V., Taub, M., and Bradbury, A. (2019). “Self-regulation in computer-assisted learning systems,” in The Cambridge handbook of cognition and education. Editors J. Dunlosky, and K. A. Rawson (Cambridge: Cambridge University Press), 597–618.
Azevedo, R., Taub, M., and Mudrick, N. V. (2018). “Understanding and reasoning about real-time cognitive, affective, and metacognitive processes to foster self-regulation with advanced learning technologies”. in Handbook of self-regulation of learning and performance, Editos. J. A. Greene, and D. H. Schunk (New York, NY: Routledge), 254–270.
Baker, R. S., and Yacef, K. (2009). The state of educational data mining in 2009: a review and future visions. JEDM J. Educ. Data Min. 1, 3–17. doi:10.1016/j.sbspro.2013.10.240
Bannert, M., Hildebrand, M., and Mengelkamp, C. (2009). Effects of a metacognitive support device in learning environments. Comput. Hum. Behav. 25, 829–835. doi:10.1016/j.chb.2008.07.002
Beheshitha, S. S., Hatala, M., Gašević, D., and Joksimović, S. (2016). “The role of achievement goal orientations when studying effect of learning analytics visualizations”, in Proceedings of the sixth international conference on learning analytics & knowledge, 54–63.
Bodily, R., Kay, J., Aleven, V., Jivet, I., Davis, D., Xhakaj, F., et al. (2018). “Open learner models and learning analytics dashboards: a systematic review”, in Proceedings of the 8th international conference on learning analytics and knowledge, 41–50.
Bodily, R., and Verbert, K. (2017). “Trends and issues in student-facing learning analytics reporting systems research”, in Proceedings of the seventh international learning analytics & knowledge conference, 309–318.
Bonsignore, E., DiSalvo, B., DiSalvo, C., and Yip, J. (2017). “Introduction to participatory design in the learning sciences”, in Participatory Design for Learning, 28, 15–18.
Butler, D. L., and Cartier, S. C. (2004). Promoting effective task interpretation as an important work habit: a key to successful teaching and learning. Teach. Coll. Rec. 106, 1729–1758. doi:10.1111/j.1467-9620.2004.00403.x
Callan, G. L., and Shim, S. S. (2019). How teachers define and identify self-regulated learning. Teach. Educ. 54, 295–312. doi:10.1080/08878730.2019.1609640
Calvo-Morata, A., Alonso-Fernández, C., Pérez-Colado, I. J., Freire, M., and Martínez-Ortiz, I. (2019). Improving teacher game learning analytics dashboards through ad-hoc development. J. Univ. Comput. Sci. 25, 1507–1530. doi:10.1109/ACCESS.2019.2938365
Chen, Z.-H., Lu, H.-D., and Chou, C.-Y. (2019). Using game-based negotiation mechanism to enhance students' goal setting and regulation. Comput. Educ. 129, 71–81. doi:10.1016/j.compedu.2018.10.011
Chi, M., VanLehn, K., Litman, D., and Jordan, P. (2011). Empirically evaluating the application of reinforcement learning to the induction of effective and adaptive pedagogical strategies. User Model. User Adapted Interact. 21, 137–180. doi:10.1007/s11257-010-9093-1
Clark, R. C., and Mayer, R. E. (2008). Learning by viewing versus learning by doing: evidence-based guidelines for principled learning environments. Perform. Improv. 47, 5–13. doi:10.1002/pfi.20028
Corbin, J. M. (1998). The corbin and strauss chronic illness trajectory model: an update. Res. Theor. Nurs. Pract. 12, 33.
Dawson, S., Bakharia, A., and Heathcote, E. (2010). Snapp: realising the affordances of real-time sna within networked learning environments
de Boer, H., Bergstra, A., and Kostons, D. (2012). Effective Strategies for self-regulated learning : a meta-analysis. Ph.D. thesis, gronings Instituut voor Onderzoek van Onderwijs
Dignath, C., and Büttner, G. (2008). Components of fostering self-regulated learning among students. A meta-analysis on intervention studies at primary and secondary school level, Metacognit. Learn. 3, 231–264. doi:10.1007/s11409-008-9029-x
Dillenbourg, P., Nussbaum, M., Dimitriadis, Y., and Roschelle, J. (2013). Design for classroom orchestration. Comput. Educ. 69, 485–492. doi:10.1016/j.compedu.2013.04.013
Dodge, B., Whitmer, J., and Frazee, J. P. (2015). “Improving undergraduate student achievement in large blended courses through data-driven interventions”, in Proceedings of the fifth international conference on learning analytics and knowledge, 412–413.
Doroudi, S., Aleven, V., Brunskill, E., Kimball, S., Long, J. J., Ludovise, S., et al. (2018). Where’s the reward? a review of reinforcement learning for instructional sequencing. ITS Worksh. 147, 427.
Doroudi, S., Holstein, K., Aleven, V., and Brunskill, E. (2016). Sequence matters but how exactly? a method for evaluating activity sequences from data. Grantee Submission 28, 268. doi:10.1145/2858036.2858268
D’Mello, S., Kappas, A., and Gratch, J. (2018). The affective computing approach to affect measurement. Emot. Rev. 10, 174–183. doi:10.1371/journal.pone.0130293
Elliot, A. J., and Murayama, K. (2008). On the measurement of achievement goals: Critique, illustration, and application. J. Educ. Psychol. 100, 613. doi:10.1037/0022-0663.100.3.613
Ferguson, R. (2012). Learning analytics: drivers, developments and challenges. Ijtel 4, 304–317. doi:10.1504/ijtel.2012.051816
Gašević, D., Dawson, S., and Pardo, A. (2016). How do we start? state directions of learning analytics adoption. Oslo, Norway: International Council for Open and Distance Education.
Greene, J. A., and Azevedo, R. (2007). A theoretical review of Winne and Hadwin's model of self-regulated learning: new perspectives and directions. Rev. Educ. Res. 77, 334–372. doi:10.3102/003465430303953
Gros, B., and López, M. (2016). Students as co-creators of technology-rich learning activities in higher education. Int. J. Educ. Technol. Higher Educ. 13, 28. doi:10.1186/s41239-016-0026-x
Hattie, J., and Timperley, H. (2007). The power of feedback. Rev. Educ. Res. 77, 81–112. doi:10.3102/003465430298487
Holstein, K., McLaren, B. M., and Aleven, V. (2017). “Intelligent tutors as teachers’ aides: exploring teacher needs for real-time analytics in blended classrooms”, in Proceedings of the seventh international learning analytics & knowledge conference, 257–266.
Jansen, R. S., Leeuwen, A. V., Janssen, J., and Jak, S. (2019). Self-regulated learning partially mediates the effect of self- regulated learning interventions on achievement in higher education: a meta-analysis. Educ. Res. Rev. 28, 1–20. doi:10.1016/j.edurev.2019.100292
Jivet, I., Scheffel, M., Specht, M., and Drachsler, H. (2018). “License to evaluate: preparing learning analytics dashboards for educational practice”, in Proceedings of the 8th international conference on learning analytics and knowledge, 31–40.
Ju, S. (2019). “Identify critical pedagogical decisions through adversarial deep reinforcement learning”, in Proceedings of the 12th international conference on Educational Data Mining (EDM 2019).
Ju, S., Zhou, G., Barnes, T., and Chi, M. (2020). “Pick the moment: identifying critical pedagogical decisions using long-short term rewards”, in Proceedings of the 13th international conference on Educational Data Mining.
Kite, V., Nugent, M., Park, S., Azevedo, R., Chi, M., and Taub, M. (2020). in What does engagement look like? secondary science teachers’ reported evidence of student engagement (Portland, OR: interactive poster presented at the international conference of National Association for Research in Science Teaching (NARST))
Koedinger, K. R., Brunskill, E., Baker, R. S., McLaughlin, E. A., and Stamper, J. (2013). New potentials for data-driven intelligent tutoring system development and optimization. AI Mag. 34, 27–41. doi:10.1609/aimag.v34i3.2484
Kramarski, B. (2018). “Teachers as agents in promoting students’ srl and performance: applications for teachers’ dual-role training program,” in Handbook of self-regulation and of learning and performance. Editors J. Green, and D. Schunk (New York, NY: Routledge), 223–239.
Krumm, A. E., Waddington, R. J., Teasley, S. D., and Lonn, S. (2014). “A learning management system-based early warning system for academic advising in undergraduate engineering”, in Learning analytics, New York: Springer, 103–119.
Linnenbrink-Garcia, L., Patall, E. A., and Pekrun, R. (2016). Adaptive motivation and emotion in education. Policy Insights Behav. Brain Sci. 3, 228–236. doi:10.1177/2372732216644450
Loderer, K., Pekrun, R., and Plass, J. L. (2018). “Emotional foundations of game-based learning,” in MIT handbook of game-based learning. Editors J. L. Plass, R. E. Mayer, and H. D. Bruce (Boston, MA: MIT Press).
López-Tavares, D., Perkins, K., Reid, S., Kauzmann, M., and Aguirre-Vélez, C. (2018). “Dashboard to evaluate student engagement with interactive simulations”, in Physics Education Research Conference (PERC).
Mandel, T., Liu, Y.-E., Levine, S., Brunskill, E., and Popovic, Z. (2014). Offline policy evaluation across representations with applications to educational games. AAMAS 1077–1084.
Martinez-Maldonado, R., Dimitriadis, Y., Martinez-Monés, A., Kay, J., and Yacef, K. (2013). Capturing and analyzing verbal and physical collaborative learning interactions at an enriched interactive tabletop. Int. J. Comput. Support. Collab. Learn. 8, 455–485. doi:10.1007/s11412-013-9184-1
Martinez-Maldonado, R., Pardo, A., Mirriahi, N., Yacef, K., Kay, J., and Clayphan, A. (2015). Latux: an iterative workflow for designing, validating and deploying learning analytics visualisations. J. Learn. Anal. 2, 9–39.
Matcha, W., Gasevic, D., and Pardo, A. (2019). A systematic review of empirical studies on learning analytics dashboards: a self-regulated learning perspective. IEEE Trans. Learn. Technol. 37, 787. doi:10.1145/3303772.3303787
Mayer, R. E. (2014). Incorporating motivation into multimedia learning. Learn. InStruct. 29, 171–173. doi:10.1016/j.learninstruc.2013.04.003
Mayer, R. (2020). The Cambridge handbook of multimedia. 3 edn. Cambridge: Cambridge University Press.(in Press).
Mayring, P. (2015). “Qualitative content analysis: theoretical background and procedures”, in Approaches to qualitative research in mathematics education (New York: Springer), 365–380.
McLaren, B. M., Scheuer, O., and Mikšátko, J. (2010). Supporting collaborative learning and e-discussions using artificial intelligence techniques. Int. J. Artif. Intell. Educ. 20, 1–46. doi:10.1007/s40593-013-0001-9
Michalsky, T., and Schechter, C. (2018). Teachers' self-regulated learning lesson design: integrating learning from problems and successes. Teach. Educat. 53, 101–123. doi:10.1080/08878730.2017.1399187
Molenaar, I., Horvers, A., Dijkstra, R., and Baker, R. (2019). “Designing dashboards to support learners’ self-regulated learning”, in Companion proceedings of the 9th international learning analytics & knowledge conference, 1–12.
Moos, D. (2018). “Emerging classroom technology: using self-regulation principles as a guide for effective implementation,” in Handbook of self-regulation of learning and performance. Editors J. Green, and D. Schunk (New York, NY: Routledge), 243–253.
Nelson, T. O., and Narens, L. (1990). Metamemory: a theoretical framework and new findings. Psychol. Learn. Motiv. 26, 125–173. doi:10.1016/s0079-7421(08)60053-5
Panadero, E. (2017). A review of self-regulated learning: six models and four directions for research. Front. Psychol. 8, 422. doi:10.3389/fpsyg.2017.00422
Prieto-Alvarez, C. G., Martinez-Maldonado, R., and Anderson, T. D. (2018). Co-designing learning analytics tools with learners. Learn. Analyt. Classroom Transl. Learn. Anal. Res. Teach. 91, 93–110. doi:10.4324/9781351113038-7
Rafferty, A. N., Jansen, R., and Griffiths, T. L. (2016). Using inverse planning for personalized feedback. EDM 16, 472–477. doi:10.1021/acs.jchemed.8b00048
Roschelle, J., Dimitriadis, Y., and Hoppe, U. (2013). Classroom orchestration: synthesis. Comput. Educ. 69, 523–526. doi:10.1016/j.compedu.2013.04.010
Rowe, J. P., and Lester, J. C. (2015). “Improving student problem solving in narrative-centered learning environments: a modular reinforcement learning framework”, in International conference on artificial intelligence in education (New York: Springer), 419–428.
Santos, J. L., Verbert, K., Govaerts, S., and Duval, E. (2013). Addressing learner issues with stepup! an evaluation. In Proceedings of the third international conference on learning analytics and knowledge, 14–22.
Santos, O. C., Boticario, J. G., and Pérez-Marín, D. (2014). Extending web-based educational systems with personalised support through user centred designed recommendations along the e-learning life cycle. Sci. Comput. Program. 88, 92–109. doi:10.1016/j.scico.2013.12.004
Scheiter, K., and Gerjets, P. (2007). Learner control in hypermedia environments. Educ. Psychol. Rev. 19, 285–307. doi:10.1007/s10648-007-9046-3
Scheu, O., and Zinn, C. (2007). “How did the e-learning session go? the student inspector”, in 13th international conference on artificial Intelligence and Education (AIED 2007) (Amsterdam: IOS Press).
Schunk, D. H., and Zimmerman, B. J. (1994). Self-regulation of learning and performance: issues and educational applications (Mahwah, NJ: Lawrence Erlbaum Associates, Inc).
Schwendimann, B. A., Kappeler, G., Mauroux, L., and Gurtner, J.-L. (2018). What makes an online learning journal powerful for vet? distinguishing productive usage patterns and effective learning strategies. Empir. Res. Voc. Educ. Train. 10, 9. doi:10.1186/s40461-018-0070-y
Shen, S., and Chi, M. (2016). “Reinforcement learning: the sooner the better, or the later the better?”, in Proceedings of the 2016 conference on user modeling adaptation and personalization, 37–44.
Simonsen, J., and Robertson, T. (2012). Routledge international handbook of participatory design. (London, UK: Routledge).
Teasley, S. D. (2017). Student facing dashboards: one size fits all?. Technol. Knowl. Learn. 22, 377–384. doi:10.1007/s10758-017-9314-3
Tissenbaum, M., Matuk, C., Berland, M., Lyons, L., Cocco, F., Linn, M., et al. (2016). “Real-time visualization of student activities to support classroom orchestration”, in Proceedings of international society of the learning sciences.
Vatrapu, R., Teplovs, C., Fujita, N., and Bull, S. (2011). “Towards visual analytics for teachers’ dynamic diagnostic pedagogical decision-making”, in Proceedings of the 1st international conference on learning analytics and knowledge, 93–98.
Verbert, K., Duval, E., Klerkx, J., Govaerts, S., and Santos, J. L. (2013). Learning analytics dashboard applications. Am. Behav. Sci. 57, 1500–1509. doi:10.1177/0002764213479363
Wickens, C. D., Hutchins, S., Carolan, T., and Cumming, J. (2013). Effectiveness of part-task training and increasing-difficulty training strategies: a meta-analysis approach. Hum. Factors 55, 461–470. doi:10.1177/0018720812451994
Winne, P. H. (2018). “Cognition and metacognition within self-regulated learning,” in Handbook of self-regulation of learning and performance, Editors J. A. Greene, and D. H. Schunk (New York, NY: Routledge), 52–64.
Winne, P. H. (2019). “Enhancing self-regulated learning for information problem solving with ambient big data gathered by nstudy”, in Contemporary technologies in education. (New York, NY: Springer), 145–162.
Wise, A. F., and Jung, Y. (2019). Teaching with analytics: towards a situated model of instructional decision-making. J. Learn. Anal. 6, 53–69. doi:10.18608/jla.2019.62.4
Xhakaj, F., Aleven, V., and McLaren, B. M. (2016). “How teachers use data to help students learn: contextual inquiry for the design of a dashboard”, in European conference on technology enhanced learning, (New York, NY: Springer), 340–354.
Keywords: self-regulated learning (SRL), teacher decision making, learning, multimodal data, teacher dashboards
Citation: Wiedbusch MD, Kite V, Yang X, Park S, Chi M, Taub M and Azevedo R (2021) A Theoretical and Evidence-Based Conceptual Design of MetaDash: An Intelligent Teacher Dashboard to Support Teachers' Decision Making and Students’ Self-Regulated Learning. Front. Educ. 6:570229. doi: 10.3389/feduc.2021.570229
Received: 06 June 2020; Accepted: 11 January 2021;
Published: 19 February 2021.
Edited by:
Tova Michalsky, Bar-Ilan University, IsraelReviewed by:
Kenneth J Holstein, Carnegie Mellon University, United StatesCopyright © 2021 Wiedbusch, Kite, Yang, Park, Chi, Taub and Azevedo. This is an open-access article distributed under the terms of the Creative Commons Attribution License (CC BY). The use, distribution or reproduction in other forums is permitted, provided the original author(s) and the copyright owner(s) are credited and that the original publication in this journal is cited, in accordance with accepted academic practice. No use, distribution or reproduction is permitted which does not comply with these terms.
*Correspondence: Megan D. Wiedbusch, TWVnYW5XaWVkYnVzY2hAa25pZ2h0cy51Y2YuZWR1
Disclaimer: All claims expressed in this article are solely those of the authors and do not necessarily represent those of their affiliated organizations, or those of the publisher, the editors and the reviewers. Any product that may be evaluated in this article or claim that may be made by its manufacturer is not guaranteed or endorsed by the publisher.
Research integrity at Frontiers
Learn more about the work of our research integrity team to safeguard the quality of each article we publish.