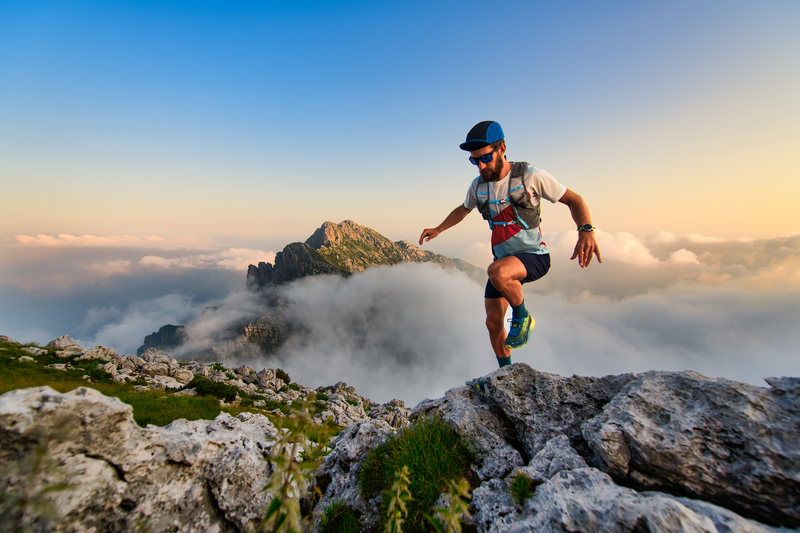
94% of researchers rate our articles as excellent or good
Learn more about the work of our research integrity team to safeguard the quality of each article we publish.
Find out more
ORIGINAL RESEARCH article
Front. Educ. , 21 October 2021
Sec. Educational Psychology
Volume 6 - 2021 | https://doi.org/10.3389/feduc.2021.529197
The study examines university students’ course preferences and their relations to learning approaches, apparently for the first time, to gain insight how different course designs are experienced by students with different learning approaches. The data includes students from two universities and fields: business (n = 467) and social sciences (n = 313). The attributes in preference measurement were selected on the basis of previous research and focus groups discussions and choice-based conjoint analysis was used. The learning approaches were measured using Experiences of Teaching and Learning Questionnaire developed by Entwistle and Ramsden. Social science students valued more interesting, challenging and time-consuming courses than business students, who valued more relevant and easier courses requiring less time. Social science students scored higher in the deep approach. The relationship between preferences and approaches was strikingly similar in the two universities: strategic students prefer relevance and deep learners challenge in both universities. An exception was that interesting courses were related to deep learning among social science students, and to the surface approach among business students. Further studies should extend our understanding of what interesting means to different kinds of students. The results give tools to design courses that enhance students’ learning and offers new insights to learning approach research.
Educational institutions function in increasingly competitive environments. Attracting and retaining motivated and skilful students is vital for universities (e.g. Soutar and Turner 2002). Recent studies (e.g. Mark, 2013) indicate that students view their educational choices in increasingly consumerist terms. How to engage students has become an important issue at universities, demonstrated for instance by different teaching strategies: for instance promoting to seek understanding instead of just passing courses (Biggs and Tang, 2007) and activating teaching methods, such as collaborative learning (Macfarlane and Tomlinson, 2017).
However, students’ perceptions on learning environment has impact on learning and academic achievement (Lizzio et al., 2002). Typically perceptions of the learning environment have been studied as course experiences, reactions to the different aspects of courses, such as appropriate assessment and workload, clear goals and standards, good tutoring and preferences for lecturers’ personalities (Entwistle et al., 2003; Richardson, 2005, Richardson, 2006; Cano et al., 2018). While previous studies have operationalized the learning context in a variety of ways, the target for evaluation has always been the students’ current experience of the learning context. We know much less about students’ course preferences - what attributes students prefer before taking the course—which are the focus of this study.
Studying general course preferences gives more insight into how students perceive the learning environment and into student engagement: what the students value in courses and what kind of preference trade-offs there exist—e.g. does raising the interest level of a course increase its total value for a student more than increasing teacher’s pedagogical abilities.
Students’ preferences for teaching and learning have been studied in particular within the online or distance learning (e.g. Marmon et al., 2014; Koper, 2015; Smith et al., 2015; Acharya and Lee, 2018; Malarkodi et al., 2018; Kuzmanovic et al., 2019). Few studies also exist within more traditional teaching showing that students prefer interesting and learning-focused courses (e.g. Sander et al., 2000; Bacon and Novotny, 2002; Wilhelm, 2004; Cunningham et al., 2006; Ting and Lee, 2012; Kuzmanovic et al., 2013; Abeysekera, 2015). Yet, it seems that there are more opportunities to utilize preference studies in student engagement than is currently acknowledged.
Several studies (e.g. Lizzio et al., 2002; Richardson 2005, 2006) have indicated the relationship of course experiences and learning approaches. Students’ learning approaches can be described as deep (aiming at understanding), surface (fragmented learning and aiming to meet formal course requirements) and strategic (organized studying and effort management to earn highest grades—also called the achieving approach; Biggs and Tang, 2007; Entwistle and McCune, 2004). Usually, the deep approach is positively linked to high academic achievement, whereas the surface approach has shown negative association (e.g. Chamorro-Premuzic and Furnham, 2008; Fenollar et al., 2007). Concerning course experiences, a heavy workload, strict time limits and intimidating situations increase the adoption of the surface approach (e.g. Entwistle and Tait, 1990; Kyndt et al., 2011) whereas relevance (Entwistle and Tait, 1990) good teaching (Lizzio et al., 2002) and a student-centred approach of teachers (Beausaert et al., 2013) increases the deep approach. There also seems to be disciplinary variation in how students experience the teaching-learning environment (Haarala-Muhonen et al., 2011), as well as the approaches to learning that they take. Students in soft disciplines (such as social science and behavioral sciences) are more prone than students in hard disciplines (such as pharmacy and science) to adopt the deep approach, and vice versa (Parpala et al., 2010; Smith and Miller, 2005). On the other hand, cultural factors play a role even within the same discipline (Byrne et al., 2009). Learning approaches are related to students’ well-being and feelings of exhaustion (Asikainen et al., 2020). It is important to examine the link between learning approaches and course preferences to better understand how students applying different approaches perceive the learning environment. This would also help instructors to design courses that support well-being and learning of students.
Besides the learning environment, individual variables such as gender may affect the learning approaches. Results concerning gender are, however, mixed. Males show either higher scores in deep learning (Sadler‐Smith, 1996; Duff, 2002) or in surface learning than females (Salamonson et al., 2013), while females have either scored higher in the strategic approach than males (Heijne-Penninga et al., 2008), or there have been no significant differences between genders (for a review see, Duff, 2002).
We make a comparative measurement of course preferences and learning orientations across two independent universities in the Helsinki metropolitan area: Aalto (Business School) and University of Helsinki (department of social sciences). These two universities also let their students take courses in the other (though this is not very common). Previous studies (Verkasalo et al., 1994; Myyry and Helkama, 2001) have indicated that these student populations differ in their value priorities measured by Schwartz (1992) value survey. Business students (BIZ) scored higher in power (societal prestige and controlling others) and achievement (personal success and competence according to social norms) than social science students (SoS), who, on the other hand, scored higher in universalism (understanding, tolerance and protection for the welfare of all people and for nature) (Verkasalo et al., 1994; Myyry and Helkama, 2001). Thus, it seems that different goals motivate students in these fields of study: individual interests for BIZ students and collective interests for SoS students. We therefore expect to find differences between BIZ and SoS students in course preferences and approaches to learning.
Proceeding from the findings outlined above, in this study we analyse the interconnection of perceived learning contexts and learning approaches. More specifically, our aim is to examine students’ course preferences and their relations to learning approaches to gain insight how course design is experienced by students. Based on the previous research it seems that at least quality of teaching, learning goals, workload and interest are important for students (Lizzio et al., 2002; Richardson, 2006; Kyndt et al., 2011; Ting and Lee, 2012; Abeysekera, 2015). We will elaborate these elements of learning environment further to study the course preferences. Because perceptions of the learning environment have an impact on the learning approaches, and, on the other hand, the learning approaches affect the perceptions of the learning environment (Richardson, 2006), it is important to examine the interaction between the two variables. Richardson (2006) even concludes that “attempts to enhance the quality of student learning in higher education need to address both students’ perceptions of their academic context and their study behavior within that context”.
Our research questions are:
What kind of course preferences do BIZ and SoS students have and how do they differ from each other?
Do BIZ and SoS students differ in their learning approaches?
Are learning approaches and course preferences associated with each other?
Do associations between learning approaches and course preferences differ between the two student groups?
Course preferences were measured by choice based conjoint analysis (CBC). That is a modern version of conjoint analysis (CA) where the preferences are elicited asking the respondent to choose the best (possibly also worst) among product/service profiles offered. The aim is to assess the total value of products/services determined by several attributes. In the profiles two or more attributes are considered simultaneously. The method has been widely adopted, but its use is still relatively scarce within education. In 2016, ISI Web of Science reported 6,248 articles published since the 1990s with “conjoint analysis” included in the topic among which 138 belonged to the behavioral sciences, 357 to psychology, and 54 to education.
Also, CA allows to assess how well its results fit the observed data. This is not possible with Likert scale questions which can also be employed for measuring preferences in the case of an additive utility function. CBC as a method of preference measurement is suitable for also educational studies related to choice—CBC is a multi-attribute method where several attributes are assessed simultaneously. The approach makes the respondent assess tradeoffs between different attributes to reveal preferences. Other approaches, like Likert-scale ratings, consider typically one attribute at a time.
Some research comparing conjoint analysis and preference elicitation using Likert scale questions (Phillips et al., 2002; Johnson et al., 2006) indicated that the methods produce different results.
In CA the total utility is assumed to be a function of the relevant attributes. The attributes included in the analysis have a number (e.g., 2–6) of alternative values called levels. Conjoint analysis techniques (e.g. Orme, 2005) use questionnaires to elicit information on the respondent’s preferences, employing hypothetical product/service profiles consisting of attribute levels that are included in the analysis (see, Figure 1). In our CBC study the respondent simply chooses the most preferred profile among those offered. CBC produces interval scaled partial utilities called part worths for every attribute level that reflect their preference. As the simple additive function is used almost without exception, the total utility of a profile is then the sum of the levels’ part worths present in the profile. The part worths can be estimated even at the level of the respondent.
The estimation of respondent part worths was carried out by Hierarchical Bayes estimation (HB, Sawtooth Software 5.2; Lenk et al., 1996). The fit measure for HB is the root likelihood (rlh) reflecting the probability that in a random task, the part worths estimated confirm the actual choice made. If the model is as good as a random model, then its rlh is 1/k, with k being the number of profiles in a task. Thus it is possible to view how well the models estimated reflects the responses given by the respondents—one advantage of CA. The validity in CA is directly related to successfully choosing correct attributes and levels.
In CBC analysis, decisions made related to the number of attributes and levels are of key importance. The greater these numbers are, the greater the number of profile choices required to reach reasonable rlh values and the more exhausting the choices are (regarding the predictive ability of HB estimation, see Halme and Kallio 2011). Thus only the most relevant attributes should be included in CBC studies. Attributes not included are assumed to have the same level. A more formal introduction to CA can be found in Appendix A.
The Bayesian approach was employed to pair-wise compare the part worths of each attribute level across university and sex, while then using that information as a covariate in HB estimation (see, Orme and Howell, 2009). For example when BIZ and SoS means are compared with respect to some attribute level, during each iteration HB generates for BIZ and SoS separately the mean of the (normal) posteriori probability distribution of that level and those pairs of means are analysed after convergence. One can examine if either the BIZ or SoS mean is larger. For example, if the BIZ mean was larger in 95 percent of the iterations, we may say that its mean is greater with a confidence rate of 95 percent, and the SoS mean is greater with a confidence rate of 5 percent.
We studied the relationship between course preferences and learning approaches by, firstly, identifying groups with different preferences using k-means cluster analysis. We then studied the differences in learning orientations across the clusters using ANOVA.
The attributes to be dealt with in the study needed to be chosen carefully. A list of potential attributes was built using existing literature (e.g., Entwistle and Tait, 1990; Bacon and Novotny, 2002; Wilhelm, 2004; Cunningham et al., 2006; Ting and Lee, 2012; Kuzmanovic et al., 2013). The attributes can be summarized as relevance and real-world orientation, grading leniency, work load and challenge level, level of interest and relevance for future career, timing of classes, group size, teaching forms, teacher abilities and existing/non-existing web-pages used for managing the course. Four focus group sessions with 17 participants were arranged in the two universities in order to generate and assess attributes. The groups first freely discussed the course selection criteria. In the second part, the members commented upon the list of attributes built from previous studies. In the last phase of the focus group sessions, the participants selected the attributes they considered as being most important. The number of attributes and levels were minimized in order to prevent respondent cognitive over-taxation and to ensure sufficient accuracy in the estimation. On the basis of the focus group results the following attributes were included in the final questionnaire: pedagogical skills (instructor), course relevance for working life and respondent’s personal interest in the topic (course content), as well as the level of challenge and work load of the course. The levels were selected in such a way that no “un-acceptables” were included—e.g., pedagogical skills were at minimum “slightly below average” and the number of levels were kept low to keep the number of questions required low. The attributes and levels are listed in Table 1.
The questionnaire was structured such that twelve sets of three course profiles were presented to the respondent for evaluation and choice. Figure 1 presents one possible set of profiles.
Software SSI Web 7.0.22 was used with the option “balanced overlap” (see, Chrzan and Orme, 2000) in the choice task generation. The questionnaire was structured such that twelve sets of three course profiles were presented to the respondent for evaluation and choice.
The learning approach scale was a modified and shortened version of the Experiences of Teaching and Learning Questionnaire (ETLQ), developed by Entwistle et al. (2003). Parpala and Lindblom-Ylänne (2012) modified a 16-item version of the ETLQ, trying to solve some of the psychometric and wording problems of the shortened version that was used previously in Finland. After piloting the 16-item version with a student sample (N = 82) we selected eleven items that loaded well on three factors: As regards one factor the absolute value of the loading exceeded 0.4, while with other loadings it was less than 0.2—with at least three items per factor required. Students responded to the questions on a 5-point Likert scale. For the final sample, the structure was confirmed by running confirmatory factor analyses for both of the universities. For SoS and BIZ, the RMSEA was 0.00 and 0.03 respectively, with the cmin/df being 3.1 for both. The factors and their Cronbach’s alphas were as follows: Deep approach (alpha 0.674), surface approach (alpha 0.671), and strategic approach (alpha 0.744). The alpha values did vary from these figures very slightly, when calculated separately according to university. In fact for the surface approach, the alpha would have increased by 0.05 had one of the items been deleted. That item, however, represented the core idea of the surface approach, while the rest of the items reflected learning difficulties and therefore were retained in the construct.
The data was gathered in May and June of 2010, from BIZ students at the AaltoUniversity and from SoS students at the University of Helsinki. The university registries provided the e-mail addresses of second year students and older. The authors sent an e-mail with a motivation vignette and a short description of the study with a link to the questionnaire. After one reminder, 467 BIZ students and 313 SoS students completed the questionnaire, comprising 20.9 and 18.3% of the students contacted from Aalto and University of Helsinki. Five respondents were excluded due to their repeating the same response.
The opening questions were demographic (Table 2). These were followed by the learning approach scale and twelve choice tasks measuring the course preference.
The sex distribution differed significatly across universities (χ2(1) = 83.83, p <.001). A risk level of 0.05 is used throughout the study.
The HB estimation of the whole sample produced the rlh 0.71 in 30,000 iterations, which means that the model exceeds by more than 100% the performance of a random model.
The part worth means were compared across university and sex. In the Bayesian pairwise comparison of two means A and B, we report cases where either A is greater than B with a confidence level of 97.5 percent or more, or vice versa. If the confidence that A is greater than B is r percent, then the confidence that B is greater than A is (100-r) percent. The observed confidence is reported in parentheses. The estimation results of HB do not allow analyses similar to two-way analyses of variance. Instead we carry out paired comparisons of sex within university and of university within sex.
The mean of “course material relevant in working life and only slightly interesting” was higher among BIZ students (100), while the mean of “course material not very relevant in working life but interesting” was higher among SoS students (100) (Table 3). Males had higher means for the attribute levels “below average pedagogical skills” (100), “relevant and only slightly interesting courses” (99), “not very relevant and slightly interesting courses” (99) and “challenge level above average and work load 25% above average” (100). Females had higher means for “teacher has ok reputation as pedagogue” (100), “course relevant and interesting” (100), “course not very relevant but interesting” (100) and “course relatively easy and work load 25% below average” (100). Viewing paired comparisons of sex within university and university within sex in Table 4 we see, e.g., that only in BIZ men value higher than females “relevant and only slightly interesting courses” and only in SoS males value higher “not very relevant and slightly interesting courses”. Only SoS females valued higher “teacher has an ok reputation as pedagog”. The challenge level and work load differences across sex seem to originate from BIZ—the females value the easy courses with low workload more, as well as the courses with average workload, and the males valued more challenging courses with high workloads. Such differences were not observed in SoS.
TABLE 4. Confidence percentages exceeding 97.5%/below 2.5% resulting from the comparison of the part worths across sex within university and university within sex. E.g. the confidence that in BIZ females value the first attribute level more than males is 0.2% meaning that males value that more with confidence 99.8%.
In a two-way analysis of variance of the learning approaches university and sex were analyzed. The interaction was significant in none of the analyses. In the analysis of the strategic approach (F(3, 773) = 2.67, p = 0.047), the sex (F(1,773) = 6.029, p = 0.014) was significant - Females scored higher in the strategic approach. In the deep approach (F(3, 773) = 17.41, p < 0.001), both sex (F(1, 773) = 5.99, p = 0.015) and university F(1, 773) = 24.128 p < 0.001) were significant. Males and SoS students scored higher in the deep approach. For the surface approach the model was not significant. The means and standard deviations of the three approaches, according to university and sex, are presented in Table 5.
TABLE 5. Means and standard deviations of three study approaches according to university (field of study) and sex, N = 775 (464 BIZ and 311 SoS).
Next we discuss the preference groups that can be found in the data, and which study approaches they associate with. The clustering task was explorative and we tried several procedures. The aim was to find a cluster centres with distinctive profiles. Moreover, we wished to exclude small clusters (less than 10 percent of the data) due to the limited size of the sample. Already for six clusters, it was almost impossible to generate a solution with no cluster size below 5%. A 5-cluster solution was chosen including interesting clusters with sufficient sizes. The two samples were also analysed separately to guarantee that all cluster types were represented.
In Table 6 we see that, for example, excellent teacher pedagogical abilities are valued highly in cluster “teacher” whereas skills even slightly below average are avoided across all of the clusters. The clusters are characterized next.
Relevance-oriented (26.9% of the respondents) BIZ: SoS 34.5%: 15.5%; BIZ percentage is larger (z = 1.81, p = 0.035). “Relevant but not very interesting” course more preferred than in other clusters. Pedagogical abilities matter less than for the other groups.
Balanced (28.3%), BIZ:SoS 25.2%: 32.9%: Challenging and more time consuming than typical courses appreciated more than in other clusters. The difference in the part worths of challenging and easy courses is the smallest which means that this cluster is the most indifferent with respect to this attribute. The part worth of “course relevant and interesting” is the highest among the clusters.
Teacher-oriented (16.7%), BIZ:SoS 18.3%: 14.3%: Teacher’s excellent pedagogical skills more appreciated than in the other clusters. Easy courses less preferred than in the other clusters.
Interest-oriented (15.4%), BIZ:SoS 8.8%: 25.2%; SoS percentage is bigger (z = 1.98, p = 0.024): This cluster emphasizes interesting course contents.
Non-challenge-oriented (12.8%), BIZ:SoS 13.2%:12.2%. Low utility in courses more challenging than the average. Less challenging courses are preferred.
Next we examine how learning approaches are linked to course preferences. We do this separately for the two universities, since we know that there are differences between them in both learning approaches as well as course preferences (see, Table 7).
We first look at the means of the learning approaches among BIZ and SoS students, separately. Using Welch’s variance-weighted Anova, we see that the learning approaches differ across the clusters among BIZ students (for all approaches p <.002; strategic F(4, 459) = 5.67, deep F(4, 459) = 4.52 and surface F(4, 459) = 5.13). Among SoS students, the difference is significant only in terms of the deep approach (F(4, 106) = 8.04, p <.001).
When looking at the means of the approaches, we see that the relevance oriented cluster gathers highly strategic oriented students in both of the universities. Among SoS students, the deep approach of members of the relevance cluster is rather low. Recalling that the SoS students’ score higher in the deep approach than BIZ, note that in the relevance cluster there is no such difference. In the balanced cluster the strategic approach mean is relatively high. Moreover among BIZ, balanced cluster members’ deep approach mean is at its highest, unlike among SoS students (whose deep approach is higher in the interest oriented cluster). The interest oriented cluster produces perhaps the most unexpected results, as the approaches of the different fields seem different. In this cluster the BIZ members’ deep approach mean is low, while their surface approach mean is the highest among any of the clusters. As for SoS members, they have the highest deep approach and lowest surface approach means. The difference in the deep approach and surface approach across universities is significant (deep t(117) = −4.11, surface t(117) = 4.42, for both p < 0.0001). However, the strategic approach mean is low in both universities, while in BIZ it has the lowest mean among any of the clusters.
It therefore seems that different attitudes towards surface and deep approaches lead to the valuation of interest in courses in the two universities. It is true that in SoS the mean of the deep approach is higher and, in turn, in BIZ the surface approach is higher. Yet the major differences seem to indicate some other explanation. As for the non-challenge cluster where members value an easy work load, in both universities they also have low strategic approach, low deep approach and high surface approach means.
The aim of this study was to investigate whether BIZ and SoS students have different course preferences, if they differ in their learning approaches, if course preferences and learning approaches are linked to each other, and if relationships between learning approaches and course preferences are different in different universities. Course preferences indicate which attributes the students prefer before taking the courses.
We found different course preferences across the universities in two attributes: SoS students preferred courses where the course material is not very relevant to working life but interesting, whereas BIZ students preferred courses with course material that is relevant in working life and only slightly interesting. In terms of preference clusters, there were more BIZ than SoS students in the relevance-oriented cluster, where relevance was emphasized over interesting content. The interest-oriented cluster members emphasized interesting contents in their course choices. A larger share of SoS than BIZ students were members of this cluster. The results are both conceptually and empirically in line with previous findings obtained from values research—BIZ students value achievement (personal success and competence according to social norms) more than SoS students, and universalism (understanding and tolerance) is valued more by SoS students than BIZ students (Verkasalo et al., 1994; Myyry and Helkama, 2001). Relevance might be more important to achieve success, and interesting content to reach understanding. The observation that BIZ students valued relevance is in conflict with Ting and Lee (2012) finding among Malaysian marketing students, who did not give much importance to future career skills. SoS students preference for interesting contents is supported by earlier results (Ting and Lee, 2012).
SoS students scored higher than BIZ students in deep learning. The result makes sense based on value differences between them, and considering that SoS represents a ‘pure-soft’ discipline, and business perhaps a mixture of ‘hard-applied’ and ‘soft-applied’ disciplines (Becher, 1994). Students in soft disciplines are more prone to adopt a deep approach than are students in hard disciplines, and vice versa (Parpala et al., 2010; Smith and Miller, 2005).
As for gender differences, we found them both in course preferences and in learning approaches. Males preferred relevance and challenging courses with a 25% larger workload and emphasized pedagogical skills less than females. Quality of teaching and perceived workload are essential elements of learning environment affecting learning (Richardson, 2006; Kyndt et al., 2011). Our results indicate, however, that they are preferred differently by males and females. In terms of learning approaches, females scored higher in the strategic approach and males scored higher in the deep approach, results of which are in line with previous studies (Heijne-Penninga et al., 2008; Sadler-Smith, 1996; Duff, 2002). The finding that males preferred challenging courses could be linked to the observation that they scored higher in the deep learning than females: The deep approach reflects intrinsic motivation and willingness to understand the course content (Biggs and Tang, 2007).
When looking at the relationships between course preferences and learning approaches, we found that the relevance-oriented cluster gathered the highly strategic students from both fields. This makes sense considering that the strategic approach refers to organized studying to achieve success (Entwistle and McCune, 2004; Biggs and Tang, 2007), and relevance of course content may then be emphasized. In the balanced cluster (appreciating challenging and time-consuming courses), the BIZ students’ deep learning mean is the highest, whereas among the SoS students it is on an average level. Deep learning aims to understanding and mastery—thus it is logical that students adopting the deep approach value challenge spending their time in studying. The most intriguing result was obtained for the interest-oriented cluster. SoS students had the highest scores in deep learning and lowest in surface learning, yet among BIZ students it was almost the opposite. They scored the highest in surface learning and nearly the lowest in deep learning. The student profiles in these two groups in the interest-oriented cluster are therefore different. Achievement values refer to seeking personal competence according to social norms (Schwartz, 1992). Thus, maybe BIZ surface learners are interested in courses which help them to reach that goal.
For the learning approaches we employed a scale based on the ETLQ, which is used globally, and made the final choice of the items by carrying out a pre-study. The reliabilities of the constructs were on satisfactory and good levels in the final questionnaire, albeit using a shortened version of the measure weakens the comparability of the results. Preceding the conjoint study, focus groups in both universities discussed freely and evaluated pre-designed lists of potential attributes that were selected from literature. From this basis, the final selection of attributes and levels was made. Thus even if only a few attributes of preferences could be studied, they were nevertheless chosen with care using both literature and focus groups. E.g., it was not reasonable to include the structure of the course among the attributes - for BIZ students the structure had very little variety whereas for SoS students there were more options. However, in the focus groups it was not gaining much attention. In CA, attributes that are excluded are assumed to have the same levels in all comparisons. The estimated fit of the individual utility functions was good, exceeding the random model by 113%. The study included only attributes that were considered to be the most important, as questions in CBC are time-consuming to answer in comparison to traditional survey questions. The courses considered were nevertheless assumed as being different only with respect to those attributes under study.
The study results are from one country and education system. The response rate was around 20% at both universities, which does not differ from the generally low rates of response for electronic surveys. Responding to a CBC questionnaire with twelve choice questions is also relatively time-consuming, from which typically low response rates can be predicted. Moreover, the data was collected in 2010. Since then, the teaching and learning environment have somewhat changed especially in one of the universities due to a big reform in how teaching is organized. However it is too early to make any detailed assumptions how the changes may have affected the preferences and learning approaches. Furthermore, personal human values have been defined as enduring beliefs (Rokeach, 13) and they are seen as fairly stable cognitive constructs (Schwartz, 1997). Value differences between social science and business students seem to be robust (Verkasalo, Daun & Niit, 1997; Myyry and Helkama, 2001). Because course preferences can be seen to reflect value priorities, it is assumed that they also are fairly stable, in spite of changes in the teaching-learning environment. Since relationships between course preferences and approaches to learning make conceptually sense, it is plausible that relationships stay approximately the same, too. Further research should be carried out with new as well as more representative samples, in different contexts (universities and fields of study) to gain more profound results. Motivation measures such as value priorities or achievement goals could also complement future studies.
In our data, SoS students’ preferences were in line with previous studies (e.g Lizzio et al., 2002; Richardson, 2006; Ting and Lee, 2012). However, BIZ students’ differed to some extent—they valued more relevance than interest in courses. Both SoS and BIZ students’ preferences reflected, however, the value differences found in these students populations (Verkasalo et al., 1996; Myyry and Helkama, 2001). Picture of engaged students, adopting deep learning, being interested and valuing good teaching, may be more accurate in soft disciplines and students with collectivist and universalistic values. This indicates that educational researchers should use more diverse student populations in their research and also more diverse motivational variables, such as preferences.
Studying course preferences also offer practical implications for teachers and instructors. When teachers know what type of courses students prefer, it is easier to design courses that raise interest. By emphasizing relevance for working-life, interesting content or understanding may attract different students. Helping students to find the meaning and relevance for understanding the contents beyond the surface level—to move the motivation from extrinsic towards intrinsic (Deci and Ryan, 1985) - could support them to apply the deep approach. Clear structure and modest work-load is often important for surface learners. Thus, avoiding high anxiety in studying, (Tait and Entwistle, 1996), may benefit students applying surface approach but also unorganized students applying deep approach who tend to have negative perceptions of the teaching-learning environment (Parpala et al., 2010). Constructive alignment, which means that course’s intended learning outcomes, teaching and assessment methods as well learning environment are aligned with each other (Biggs and Tang, 2007), has been recommended to apply in course design in order to support surface learners to apply more deep learning. Hattie (2015) extensive meta-analysis about what works best in higher education shows that about 50% of the variance in learning comes from students. Thus, more individual view for teaching and taking into account students’ different motivations, values and purposes for learning, and how these affect the selection of the field of study (Verkasalo et al., 1994; Myyry and Helkama, 2001) might enhance the learning process and mastery of the course contents.
Moreover, we observed that strategic students prefer relevance and deep learners challenge in both universities, whereas the interest cluster members coming from the two universities differed: the members were deep learners from SoS and surface learners from BIZ. This is interesting and worth examining in more detail: how students with different value preferences perceive interest, for example? The result suggests that also surface learners can be interested in the course contents to achieve their goals and not just choose easy courses, for instance. Personal value preferences are defined by Schwartz (1992) as motivational constructs, cognitive representations of abstract goals that serve to define situations, elicit more specific goals and guide action. It is possible that differently motivated students can be engaged to their studies if they can perform according to their values; goal achievement in learning may engage and motivate students. Our results have provided new perspectives to student engagement and research dealing with the teaching-learning environment, which should be explored more closely.
The datasets generated for this study are available on request to the corresponding author.
Ethical review and approval was not required for the study on human participants in accordance with the local legislation and institutional requirements. Written informed consent for participation was not required for this study in accordance with the national legislation and the institutional requirements.
MH has participated in setting the research targets and questions as well design of the questionnaire on the basis of literature. She is responsible for the quantitative analysis of the data and gathering the data in Aalto School of Business. LM is responsible for writing the introduction and the discussion of the article. A-MP-B has participated in setting the research targets and questions as well design of the questionnaire on the basis of previous research. She organized the data gathering at the University of Helsinki and has commented the article in detail.
The authors declare that the research was conducted in the absence of any commercial or financial relationships that could be construed as a potential conflict of interest.
All claims expressed in this article are solely those of the authors and do not necessarily represent those of their affiliated organizations, or those of the publisher, the editors and the reviewers. Any product that may be evaluated in this article, or claim that may be made by its manufacturer, is not guaranteed or endorsed by the publisher.
Abeysekera, I. (2015). Student Preferences for Instructional Methods in an Accounting Curriculum. Int. J. Teach. Learn. Higher Educ. 27 (3), 310–319.
Acharya, B., and Lee, J. (2018). Users' Perspective on the Adoption of E-Learning in Developing Countries: The Case of Nepal with a Conjoint-Based Discrete Choice Approach. Telematics Inform. 35, 1733–1743. doi:10.1016/j.tele.2018.05.002
Asikainen, H., Salmela-Aro, K., Parpala, A., and Katajavuori, N. (2020). Learning Profiles and Their Relation to Study-Related Burnout and Academic Achievement Among university Students. Learn. Individual Differences 78, 101781. doi:10.1016/j.lindif.2019.101781
Bacon, D. R., and Novotny, J. (2002). Exploring Achievement Striving as a Moderator of the Grading Leniency Effect. J. Marketing Educ. 24 (1), 4–14. doi:10.1177/0273475302241002
Beausaert, S. A. J., Segers, M. S. R., and Wiltink, D. P. A. (2013). The Influence of Teachers' Teaching Approaches on Students' Learning Approaches: the Student Perspective. Educ. Res. 55 (1), 1–15. doi:10.1080/00131881.2013.767022
Becher, T. (1994). The Significance of Disciplinary Differences. Stud. Higher Educ. 19 (2), 151–161. doi:10.1080/03075079412331382007
Biggs, J., and Tang, C. (2007). Teaching for Quality Learning at university. 3rd ed. Berkshire: McGraw-Hill.
Byrne, M., Flood, B., and Willis, P. (2009). An Inter-Institutional Exploration of the Learning Approaches of Students Studying Accounting. Int. J. Teach. Learn. Higher Educ. 20 (2), 155–167.
Cano, F., Martin, A. J., Ginns, P., and Berbén, A. B. G. (2018). Students' Self-Worth protection and Approaches to Learning in Higher Education: Predictors and Consequences. High Educ. 76, 163–181. doi:10.1007/s10734-017-0215-0
Chamorro-Premuzic, T., and Furnham, A. (2008). Personality, Intelligence and Approaches to Learning as Predictors of Academic Performance. Personal. Individual Differences 44 (7), 1596–1603. doi:10.1016/j.paid.2008.01.003
Chrzan, K., and Orme, B. (2000). An Overview and Comparison of Design Strategies for Choice-Based Conjoint Analysis (Software Software Research Paper Series). Available at: aHR0cHM6Ly93d3cuc2F3dG9vdGhzb2Z0d2FyZS5jb20vZG93bmxvYWQvdGVjaHBhcC9kZXNnbmNiYy5wZGY= (Accessed: July 15, 2021).
Cunningham, C. E., Deal, K., Neville, A., Rimas, H., and Lohfeld, L. (2006). Modeling the Problem-Based Learning Preferences of McMaster University Undergraduate Medical Students Using a Discrete Choice Conjoint Experiment. Adv. Health Sci. Educ. Theor. Pract 11, 245–266. doi:10.1007/s10459-006-0003-6
Deci, E. L., and Ryan, R. M. (1985). The General Causality Orientations Scale: Self-Determination in Personality. J. Res. Personal. 19, 109–134. doi:10.1016/0092-6566(85)90023-6
Duff, A. (2002). Approaches to Learning: Factor Invariance Across Gender. Personal. Individual Differences 33, 997–1010. doi:10.1016/s0191-8869(01)00208-2
Entwistle, N., and McCune, V. (2004). The Conceptual Bases of Study Strategy Inventories. Educ. Psychol. Rev. 16 (4), 325–345. doi:10.1007/s10648-004-0003-0
Entwistle, N., McCune, V., and Hounsell, J. (2003). “Approaches to Studying and Perceptions of University Teaching-Learning Environments: Concepts, Measure and Preliminary Findings,” in Powerful Learning Environments: Unravelling Basic Components. Editors E. De Corte, L. Verschaffel, N. Entwistle, and J. van Merrienboer (Oxford, UK: Elsevier), 89–108.
Entwistle, N., and Tait, H. (1990). Approaches to Learning, Evaluations of Teaching, and Preferences for Contrasting Academic Environments. High Educ. 19 (2), 169–194. doi:10.1007/bf00137106
Fenollar, P., Román, S., and Cuestas, P. J. (2007). University Students' Academic Performance: An Integrative Conceptual Framework and Empirical Analysis. Br. J. Educ. Psychol. 77 (4), 873–891. doi:10.1348/000709907X189118
Haarala-Muhonen, A., Ruohoniemi, M., Katajavuori, N., and Lindblom-Ylänne, S. (2011). Comparison of Students' Perceptions of Their Teaching-Learning Environments in Three Professional Academic Disciplines: A Valuable Tool for Quality Enhancement. Learn. Environ Res 14, 155–169. doi:10.1007/s10984-011-9087-x
Halme, M., and Kallio, M. (2011). Estimation Methods for Choice-Based Conjoint Analysis of Consumer Preferences. Eur. J. Oper. Res. 214 (1), 160–167. doi:10.1016/j.ejor.2011.03.049
Hattie, J. (2015). The Applicability of Visible Learning to Higher Education. Scholarship Teach. Learn. Psychol. 1 (1), 79–91. doi:10.1037/stl0000021
Heijne-Penninga, M., Kuks, J. B., Hofman, W. H., and Cohen-Schotanus, J. (2008). Influence of Open- and Closed-Book Tests on Medical Students' Learning Approaches. Med. Educ. 42 (10), 967–974. doi:10.1111/j.1365-2923.2008.03125.x
Johnson, F. R., Hauber, A. B., Osoba, D., Hsu, M. A., Coombs, J., and Copley-Merriman, C. (2006). Are Chemotherapy Patients' HRQoL Importance Weights Consistent with Linear Scoring Rules? A Stated-Choice Approach. Qual. Life Res. 15, 285–298. doi:10.1007/s11136-005-0581-4
Koper, R. (2015). How Do Students Want to Learn in Online Distance Education? Profiling Student Preferences. Int. Rev. Res. Open Distance Learn. 16 (1), 307–329. doi:10.19173/irrodl.v16i1.2000
Kuzmanovic, M., Andjelkovic Labrovic, J., and Nikodijevic, A. (2019). Designing E-Learning Environment Based on Student Preferences: Conjoint Analysis Approach. Int. J. Cogn. Res. Sci. Eng. Educ. 7 (3), 37–47. doi:10.5937/IJCRSEE1903037K
Kuzmanovic, M., Savic, G., Popovic, M., and Martic, M. (2013). A New Approach to Evaluation of university Teaching Considering Heterogeneity of Students' Preferences. High Educ. 66, 153–171. doi:10.1007/s10734-012-9596-2
Kyndt, E., Dochy, F., Struyven, K., and Cascallar, E. (2011). The Direct and Indirect Effect of Motivation for Learning on Students' Approaches to Learning through the Perceptions of Workload and Task Complexity. Higher Educ. Res. Develop. 30 (2), 135–150. doi:10.1080/07294360.2010.501329
Lenk, P. J., DeSarbo, W. S., Green, P. E., and Young, M. R. (1996). Hierarchical Bayes Conjoint Analysis: Recovery of Partworth Heterogeneity from Reduced Experimental Designs. Marketing Sci. 15, 173–191. doi:10.1287/mksc.15.2.173
Lizzio, A., Wilson, K., and Simons, R. (2002). University Students' Perceptions of the Learning Environment and Academic Outcomes: Implications for Theory and Practice. Stud. Higher Educ. 27 (1), 27–52. doi:10.1080/03075070120099359
Macfarlane, B., and Tomlinson, M. (2017). Critiques of Student Engagement. High Educ. Pol. 30 (1), 5–21. doi:10.1057/s41307-016-0027-3
Malarkodi, M., Indumathi, V. M., Indumathi, V. M., and Praveena, S. (2018). Preference Towards Online Mode of Distance Education Courses-Conjoint Analysis. IJBSM 9 (1), 178–182. doi:10.23910/ijbsm/2018.9.1.1858
Mark, E. (2013). Student Satisfaction and the Customer Focus in Higher Education. J. Higher Educ. Pol. Manage. 35 (1), 2–10. doi:10.1080/1360080x.2012.727703
Marmon, M., Vanscoder, J., and Gordensky, J. (2014). Online Student Satisfaction: an Examination of Preference, Asynchronous Course Elements and Collaboration Among Online Students. Curr. Issues Educ. 17 (3), 1–12.
McFadden, D. (1974). “Conditional Logit Analysis of Qualitative Choice Behaviour,” in Frontiers in Econometrics. Editor P. Zarembka (New York: Academic Press), 105–142.
Myyry, L., and Helkama, K. (2001). University Students' Value Priorities and Emotional Empathy. Educ. Psychol. 21 (1), 25–40. doi:10.1080/01443410123128
Orme, B., and Howell, J. (2009). Application of Covariates within Sawtooth Software’s CBC/HB Program, Theory and PracticalExample. Sequim, WA: Sawtooth Software Research Paper Series.
Parpala, A., Lindblom-Ylänne, S., Komulainen, E., Litmanen, T., and Hirsto, L. (2010). Students' Approaches to Learning and Their Experiences of the Teaching-Learning Environment in Different Disciplines. Br. J. Educ. Psychol. 80, 269–282. doi:10.1348/000709909X476946
Parpala, A., and Lindblom-Ylänne, S. (2012). Using a Research Instrument for Developing Quality at the university. Qual. Higher Educ. 18 (3), 313–328. doi:10.1080/13538322.2012.733493
Phillips, K. A., Johnson, F. R., and Maddala, T. (2002). Measuring What People Value: A Comparison of "Attitude" and "Preference" Surveys. Health Serv. Res. 37 (6), 1659–1679. doi:10.1111/1475-6773.01116
Richardson, J. T. (2006). Investigating the Relationship between Variations in Students' Perceptions of Their Academic Environment and Variations in Study Behaviour in Distance Education. Br. J. Educ. Psychol. 76, 867–893. doi:10.1348/000709905X69690
Richardson, J. T. E. (2005). Students' Perceptions of Academic Quality and Approaches to Studying in Distance Education. Br. Educ. Res. J. 31 (1), 7–27. doi:10.1080/0141192052000310001
Sadler‐Smith, E. (1996). Approaches to Studying: Age, Sex and Academic Performance. Educ. Stud. 22 (3), 367–379.
Salamonson, Y., Weaver, R., Chang, S., Koch, J., Bhathal, R., Khoo, C., et al. (2013). Learning Approaches as Predictors of Academic Performance in First Year Health and Science Students. Nurse Educ. Today 33, 729–733. doi:10.1016/j.nedt.2013.01.013
Sander, P., Stevenson, K., King, M., and Coates, D. (2000). University Students' Expectations of Teaching. Stud. Higher Educ. 25 (3), 309–323. doi:10.1080/03075070050193433
Schwartz, S. H. (1992). “Universals in the Content and Structure of Values: Theoretical Advances and Empirical Tests in 20 Countries,” in Advances in Experimental Social Psychology. Editor M.P. Zanna (San Diego: Academic Press), 25, 1–65. doi:10.1016/s0065-2601(08)60281-6
Schwartz, S. H. (1997). “Values and Culture,” in Motivation and Culture. Editors D. Munro, S. Carr, and J. Schumaker (New York: Routledge), 69–84.
Smith, A. A., Synowka, D. P., and Smith, A. D. (2015). Online Education and its Operational Attractions to Traditional and Non-Traditional College Students. Int. J. Process Manage. Benchmarking 5 (1), 37–73. doi:10.1504/ijpmb.2015.066026
Smith, S. N., and Miller, R. J. (2005). Learning Approaches: Examination Type, Discipline of Study, and Gender. Educ. Psychol. 25 (1), 43–53. doi:10.1080/0144341042000294886
Soutar, G. N., and Turner, J. P. (2002). Students' Preferences for university: A Conjoint Analysis. Intl Jnl Educ. Mgt 16 (1), 40–45. doi:10.1108/09513540210415523
Tait, H., and Entwistle, N. (1996). Identifying Students at Risk through Ineffective Study Strategies. High Educ. 31 (1), 97–116. doi:10.1007/bf00129109
Ting, D. H., and Lee, C. K. C. (2012). Understanding Students' Choice of Electives and its Implications. Stud. Higher Educ. 37 (3), 309–325. doi:10.1080/03075079.2010.512383
Verkasalo, M., Daun, A., and Niit, T. (1994). Universal Values in Estonia, Finland and Sweden. Ethnologia Europaea 24, 101–117.
Wilhelm, W. B. (2004). The Relative Influence of Published Teaching Evaluations and Other Instructor Attributes on Course Choice. J. Marketing Educ. 26 (1), 17–30. doi:10.1177/0273475303258276
In the analysis the total utility U is assumed to be a sum of the total value V and a random error term
Total value V is generally considered to be an additive function of product attributes. Each attribute is assumed to have only a finite set of possible values called levels. If there are n attributes a1, a2, …, an the total utility U is
where ui, i = 1,…, n is a utility function and ε is the random error term with expected value zero and—in the case that the utility is estimated on the segment level for example, the ε elements are independent and identically distributed. The estimated values of ui (ai) are often called partial utilities or part worths of the attribute levels. The additive function includes only main effects of the attributes, but also two-way interactions can be included. In CBC, the error term ε has the Gumbel distribution which leads to the multinomial logit choice model (McFadden, 1974). With multinomial logit if k product profiles with total values V1, …, Vk are compared—employing suitable scaling—the probability that profile l is chosen is pl
In the text when we speak about utility function or total utility, we are referring to the value function and total value V. This is because there is variety in research practices and terminology, and the word value can mean a number of things.
Keywords: Conjoint Analysis, social science students, learning approaches, course preferences, business students
Citation: Halme M, Myyry L and Pirttilä-Backman A-M (2021) Business and Social Science Students’ Course Preferences and Learning Approaches. Front. Educ. 6:529197. doi: 10.3389/feduc.2021.529197
Received: 23 January 2020; Accepted: 27 September 2021;
Published: 21 October 2021.
Edited by:
Jesus de la Fuente, University of Navarra, SpainReviewed by:
Marija Kuzmanovic, University of Belgrade, SerbiaCopyright © 2021 Halme, Myyry and Pirttilä-Backman. This is an open-access article distributed under the terms of the Creative Commons Attribution License (CC BY). The use, distribution or reproduction in other forums is permitted, provided the original author(s) and the copyright owner(s) are credited and that the original publication in this journal is cited, in accordance with accepted academic practice. No use, distribution or reproduction is permitted which does not comply with these terms.
*Correspondence: Merja Halme, bWVyamEuaGFsbWVAYWFsdG8uZmk=
Disclaimer: All claims expressed in this article are solely those of the authors and do not necessarily represent those of their affiliated organizations, or those of the publisher, the editors and the reviewers. Any product that may be evaluated in this article or claim that may be made by its manufacturer is not guaranteed or endorsed by the publisher.
Research integrity at Frontiers
Learn more about the work of our research integrity team to safeguard the quality of each article we publish.