- 1Research and Education Center for the Learning Sciences, Shizuoka University, Shizuoka-shi, Japan
- 2Graduate School of Integrated Science and Technology, Shizuoka University, Hamamatsu-shi, Japan
Idea emergence is critical in learning as knowledge creation. Although recent advancements make it possible to detect emergent ideas and evaluate how students engage in knowledge creation in collaborative learning contexts, the relationship between the learning processes and final conceptual understanding has not been well-studied. One confounding factor is how much students engage in their study topic during collaboration. In this study, therefore, we propose a new procedure for evaluating idea emergence in the context of jigsaw instruction by combining a socio-semantic network analysis of discourse and a text-mining algorithm, “the term-frequency.” This procedure was used to evaluate how high-school learners engaged in their social process of knowledge creation as well as how much they discuss their study topics, the human immune system. Results showed that the weight of priority on a study topic did not significantly differ in both high and low conceptual understanding groups, but high conceptual understanding groups were more engaged in sharing and discussing ideas in the early stage of their collaboration. It is suggested that students' recognition of the study topic was not different depending on the levels of their conceptual understanding in a well-structured collaborative learning context such as jigsaw instruction. However, what matters is how students discuss their ideas through collaborative discourse.
Introduction
Learning as Knowledge Creation
In the perspective of learning as knowledge creation (Paavola and Hakkarainen, 2005), the objective of learners is to create new knowledge through collaborative work (Bereiter, 2002). Paavola et al. (2004) identified the following unique aspects of learning as knowledge creation. First, learning is defined as the pursuit of newness. Learners are not only expected to acquire knowledge and skills but also create something new based on their learned knowledge. The main goal of learning as knowledge creation is to develop the expertise to engage in creating knowledge. Second, knowledge creation practice is a social process. Learners do not have to make newness alone. Instead, they have to collaborate with others to share their ideas and improve them. Third, even in such a social process of knowledge creation, individual competence to contribute to the process should also be a goal of instruction. When learners are engaged in knowledge-creation practices, Scardamalia (2002) discusses how students need to have collective cognitive responsibility to contribute ideas to collective knowledge advancement in the community. She defined intentional engagement in the knowledge-creation practice as the epistemic agency and proposed this agency as a new goal for instruction in the knowledge age (Scardamalia et al., 2012). For evaluating students' learning as knowledge creation, therefore, we need to assess how students engage in their social process of knowledge creation, and how each student could contribute to the social process.
For facilitating students' learning as knowledge creation, many studies have been conducted to identify adequate collaborative learning arrangements (Sawyer, 2014). One such instructional method is jigsaw instruction (Miyake and Kirschner, 2014). In jigsaw instruction, learners collaboratively work on the same materials in the expert group activity. Their collaboration facilitates constructive interaction (Miyake, 1986) among learners who meet their individual goals to become experts in their assigned material. Then, learners join the jigsaw group activity, where those having studied different materials collaborate to integrate the different sources of knowledge. In the jigsaw group activity, students engage in social interaction within multiple zones of proximal development (e.g., Brown and Campione, 1996). One student is an expert concerning one component and teaches the other group members. In this study, collaborative learning in the jigsaw instruction was analyzed by using an analytic technique described in section Evaluation of Learning as Knowledge Creation.
Evaluation of Learning as Knowledge Creation
While most studies on learning as knowledge creation have been conducted by applying qualitative approaches, it is needed to develop a new quantitative analytic framework for evaluating learning as a knowledge creation practice to handle larger and richer datasets (Martinez et al., 2003; Scardamalia et al., 2012). Both qualitative and quantitative analyses are expected to provide us with deeper insights into student learning in the mixed-methods approach (Johnson and Onwuegbuzi, 2006; Oshima et al., 2018). A promising quantitative approach that recent studies have discussed is socio-semantic network analysis (SSNA). Educational research, Computer-Supported Collaborative Learning, in particular, started the utilization of social network analysis (SNA) for analyzing an essential social interaction such as who was communicating with whom. This type of SNA provides researchers with a picture of a community from the perspective of social interaction. It has been argued, however, that the ordinary SNA is not sufficient to examine how learners engage in the social process of knowledge creation, how they exchange their ideas through their collaborative discourse (e.g., Oshima et al., 2012; Shaffer, 2017). For solving the problem, researchers thought the use of a new procedure similar to SNA, but a socio-semantic network analysis to examine different types of networks. It is based on the vocabulary that learners use in their discourse (Oshima et al., 2012), the categorized codes representing cultural practices they engage in (Shaffer, 2017), and so on. The socio-semantic network analysis (SSNA) approach is based on the co-occurrence of words or categorized codes used in discourse rather than action logs, such as who commented on whom. The basic assumption behind the algorithm is that students' ideas are represented as clusters of words used in explaining their ideas. When students discuss their ideas in a deeper way by using a variety of vocabulary, the structure of the word network becomes robustly structured. Through visualizing the network structure of words or codes used in discourse, researchers could represent how a group of learners engage in their knowledge creation. The SSNA approach has been adopted in educational studies to analyze rotation of leadership among students in the knowledge-building community (e.g., Ma et al., 2016) and to detect productive interaction patterns in the knowledge-creation practice, such as in the jigsaw instruction (e.g., Oshima et al., 2018).
Although the SSNA approach has given educational researchers a new window to evaluate students' social process of knowledge creation, it has not been sufficiently examined yet concerning other essential variables such as students' knowledge related to the study topic, and their final conceptual understanding. For instance, even though we find a different social process in high conceptual understanding groups from that in low conceptual understanding groups, it may be because high conceptual understanding groups discussed more their study topic in discourse. Thus, to effectively use SSNA approach for evaluating the classroom practice where students engage in learning as knowledge creation, we have to conduct studies further to examine the relationship among their social process of knowledge creation, their study topic related knowledge used in their discourse, and their final conceptual understanding.
Structure-Behavior-Function Framework for Evaluating Students' Understanding of a Complex Scientific Concept
In knowledge-creation practices, learners have to take on complex tasks and comprehension of phenomena. Wilensky and Jacobson (2014) define complex systems as multiple levels of organizations locally interacting with one another. Such systems include financial economies and weather systems. It is a big challenge for many learners to sufficiently understand such complex systems, despite their importance. There are several reasons behind the difficulty. One main reason is that the understanding of the complex systems often conflicts with learners' prior experience. Learners have a “centralized” mindset by which they provide explanations by assuming central control and simple causality never seen in the complex systems (Jacobson, 2001).
For appropriately assessing learners' understanding of complex systems, Hmelo-Silver and Pfeffer (2004) proposed the structure–behavior–function (SBF) framework. The framework can provide researchers with accurate information about how each learner understands multiple interrelations and the dynamic nature of complex systems. Hmelo-Silver and Pfeffer (2004) used the SBF framework for assessing conceptual understanding of the aquarium by novices and experts as follows: Structures represent elements of a system such as fish, plants, and a filter. Behaviors mean how system structures achieve their purpose. The behavior of filters is to remove waste by trapping large particles, absorbing chemicals, and converting ammonia into harmless chemicals. Finally, functions represent purposes of elements within the system, why the elements exist within a given system. The filter should exist to remove aquarium byproducts. Hmelo-Silver and Pfeffer studied verbal responses and pictorial representations created by middle school students, preservice teachers, and experts. They found that novices focused on perceptually available, static system components. Experts, on the other hand, focused more on interrelation among structures, functions, and behaviors. The results suggested that the SBF framework could be a useful formalism for understanding complex systems. In this study, the authors used the SBF framework for evaluating conceptual understanding based on their explanations of the human immune system, a complex system in biology.
Research Design and Questions
In this study, for examining how the social process of knowledge creation by high-school students is interrelated to their knowledge used in discourse and their final conceptual understanding, we analyzed their collaborative discourse in a lesson unit of the human immune system in the form of the jigsaw instruction. Their conceptual understanding was evaluated by the pre- and post-test paradigm. The social process of knowledge creation was analyzed by using SSNA, and their knowledge used in discourse related to the study topic was analyzed by calculating term-frequency, a measure of the importance of words in discourse. With the data analyses, we attempted to answer the following two research questions:
(1) Is there any significant difference in students' use of their study topic related words between high and low conceptual understanding groups in the jigsaw instruction? This research question was examined by comparing means of term-frequencies of study topic related words in discourse across high and low conceptual understanding groups. The term-frequency is a measure to evaluate how important each word is in group discourse. The measure has been used to identify the uniqueness of a document in the text-mining research (Feldman and Sanger, 2007). We utilized the procedure to examine whether study topic related words were essential to identify high or low conceptual understanding groups.
(2) Is there any difference in the pattern of social processes in the discourse between high and low conceptual understanding groups? We applied SSNA to their discourse data for examining how they exchanged their ideas related to the study topic by constructing a network of the study topic words. The difference was discussed in conjunction with the results in the first research questions.
Method
Data Contexts
Student Sample
Thirty-nine tenth-grade (15–16 years old) students (19 females and 20 males) of a high school in Japan participated in this study as part of their regular curriculum. The school is well-known and highly ranked in its district as a college prep school. Most graduating students from this school go on to universities. A science teacher with more than 10 years of teaching experience taught the students.
Lesson Unit
The lesson unit we targeted our analysis was the human immune system, a study topic in the biology class. The authors collaborated with the classroom teacher to design the lesson unit within three class hours. For representing the human immune system as a complex system, the teacher and the authors created its SBF framework based on the contents of the textbook the students used. The teacher then decided to divide the lesson unit contents into three local subsystems interacting with one another: (1) humoral immunity, (2) primary and secondary responses, and (3) cell-mediated immunity. The three subsystems were documented in three separate materials that were used in students' expert group activity.
The lesson unit was designed by the teacher and the authors in the form of jigsaw instruction (e.g., Miyake and Kirschner, 2014). Students were divided into 12 groups of three or four members. They were given a challenge in their class, such as “Can you explain how vaccinations protect us from infections?” and then provided with three study documents, each of which was necessary for solving the challenge. The documents were on (1) humoral immunity, (2) primary and secondary responses, and (3) cell-mediated immunity. In the first phase, one or two students from each group gathered to form an expert group (of three or four members) and worked on their allocated materials over 1.5 class hours (each class hour was 50 min). After the expert group activity, students returned to their original group (the jigsaw group), where the other members had different pieces of information. They were encouraged to share and integrate their knowledge to solve the challenge. The jigsaw activity took another 1.5 class hours (see Figure 1). The teacher designed group composition for both group activities. The analysis in this study was focused on student discourse in the jigsaw group activity because the task requirement in the jigsaw group activity made them share and discuss their ideas through discourse.
Study Design
Before and after the lessons, each student was asked to individually explain her/his thoughts on how vaccination protects one from infections by writing and drawing on a paper. Their discussion during the group activities was video-recorded.
Data Analysis
Evaluation of Students' Conceptual Understanding
Each student's writing and drawing for explaining how vaccination protects one from infections at the pre- and post-test was evaluated by creating the SBF framework and comparing it with the teacher-made framework. The comparison was conducted by the first author and another collaborator who was familiar with the SBF framework. When a student explanation appropriately covered more than two subsystems and their interrelations, the student was evaluated as holding the integrated understanding. When a student showed an understanding of only one subsystem but did not refer to its interrelation to other subsystems, the student was evaluated as holding a single understanding. Others who made no understanding were categorized as no understanding. After each student conceptual understanding was identified, we categorized 12 jigsaw groups into high and low conceptual understanding groups. The process-oriented measures, such as term-frequency and the total value of the degree centrality were compared between the different levels of conceptual understanding. More details are described in the results section.
Term-Frequencies of Study Topic Related Words
For evaluating how importantly students recognized the study topic related words in their discourse, we calculated the term-frequencies of the study topic related words. In the field of natural language processing (NLP) or text-mining, several algorithms are used to calculate the unique contribution of words to a discourse. The most typical metric is the term-frequency. We calculated the term-frequencies of words representing the study topic by using the formula
where t, g, and ft mean a word, a group, and frequency of the word, respectively.
We selected words representing the structure and function components of human immunity SBF as vocabularies and calculated their term-frequencies for comparing high and low conceptual understanding groups. From the formula above, it is clear that term-frequency is not a metric of an amount of time, but is an indicator that helps us examine how uniquely words contribute to a discourse. We used the metric for comparing the quantum of study-topic related talk the students engaged in during their group activities.
Socio-Semantic Network Analysis
This study aimed to evaluate students' knowledge creation practice from the perspective of how they discussed their ideas in discourse. For doing so, we conducted SSNA of vocabulary. We used the same vocabularies selected in the term-frequency analysis. A unique process in our SSNA was that we repeatedly project the vocabulary network whenever a new conversation turn appeared so that we could examine the temporal change in the vocabulary network structure. The total value of the degree centrality of a vocabulary network served as the primary network indicator. This value was recalculated based on aggregative discourse segments whenever a new conversation turn was added. The degree centrality is a measure of how strongly related to each word is within the network of vocabulary, and the centrality has been used as a measure to indicate how robust and cohesive the network structure is in previous studies (e.g., Oshima et al., 2012; Ma et al., 2016; Lee and Tan, 2017). In this study, the same measure was used for evaluating how high school students engaged in their discourse around their ideas of the vaccination mechanism. The increase in the value meant that students' discourse about their ideas became more fluent and robust. The mathematical formula for calculating the degree centrality coefficient is as follows:
For a network with n nodes, the normalized degree centrality, C'd(i), of node i is
where aij is the degree between node i and another j (1 or 0 in the algorithm of this study). The total value of degree centrality is calculated by
at any point in time during an episode of discourse. When a new conversation turn is added, the network structure may be changed by the addition of new nodes or links between new or existing nodes. A temporal change in the centrality was depicted visually as a graph with conversation turns plotted along the horizontal axis and the indicator on the vertical axis.
Results
Students' Conceptual Understanding and Group Differences
Students were categorized as having (1) no understanding, (2) single understanding, and (3) integrated understanding. When a student responded using appropriate connections among the three components (Structure, Behavior, and Function) across the sub-mechanisms, s/he was categorized into the group with integrated understanding. If a student demonstrated his/her understanding by using appropriate connections among the components within a single sub-mechanism, s/he was categorized into the group with a single understanding. Referring to the SBF framework of the human immune system, the first author and another researcher independently evaluated 10 randomly selected students' SBF frameworks based on their explanatory discourse and pictures in each of the pre- and post-tests. Cohen's Kappa coefficient for the agreement between the two raters was 0.92. Disagreements were resolved through discussion. The first author evaluated the remaining data. Because no students demonstrated proper conceptual understanding (single or integrated) of the human immune system in the pre-test, we focused on their conceptual understanding in the post-test for our analysis. Based on the SBF framework evaluation of student conceptual understanding, we categorized the 12 groups as high conceptual understanding groups (n = 3) or low conceptual understanding groups (n = 9). Groups were categorized as high conceptual understanding when every group member was evaluated as having an integrated conceptual understanding at the post-test.
Group Differences in Term-Frequencies
We selected 19 words as vocabularies representing students' ideas from the teacher-made SBF of the human immune system. Each word's term-frequency was calculated, and the means of their term-frequencies among groups were compared by using one-way ANOVA with repeated measures. As a result, we found no significant differences in the term-frequency means across the 12 groups, F(11, 198) = 2.35, p > 0.05.
SSNA of Student Discourse
Figures 2, 3 show the temporal changes in the total values of degree centrality of vocabulary networks across conversation turns. Although we could not conduct statistical analysis for examining the difference between the high and the low conceptual understanding groups because of the small sample size, our visual inspection of the graphs revealed the following. The values show a quick increase and then exceed 10.0 in all the high conceptual understanding groups (see Figure 2), whereas the values stay low and slowly increase across discourse exchanges in the low conceptual understanding groups (see Figure 3). These results may suggest that the high conceptual understanding groups engaged in sharing and discussing their ideas more quickly and sustainably.
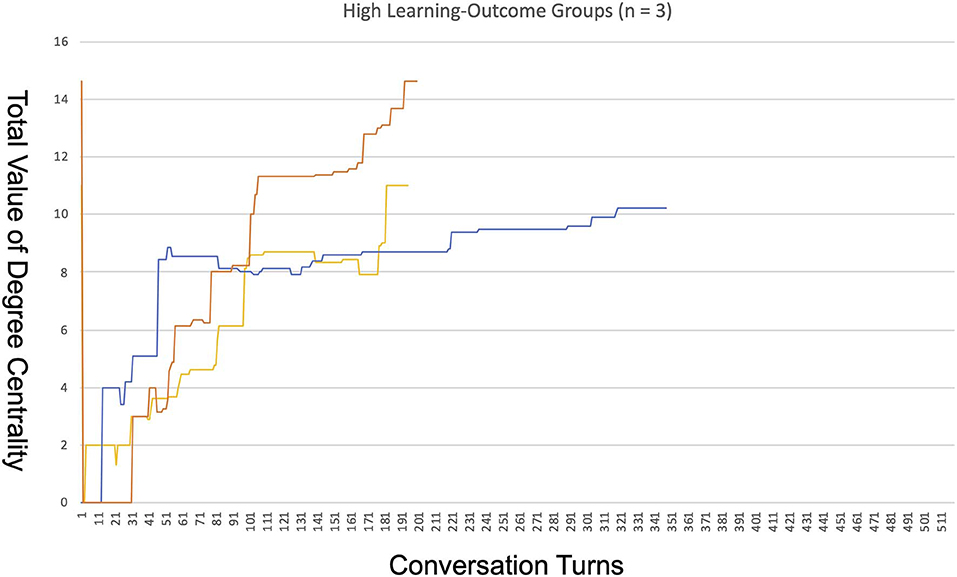
Figure 2. Temporal change in the total value of degree centrality of the vocabulary network in high conceptual understanding groups.
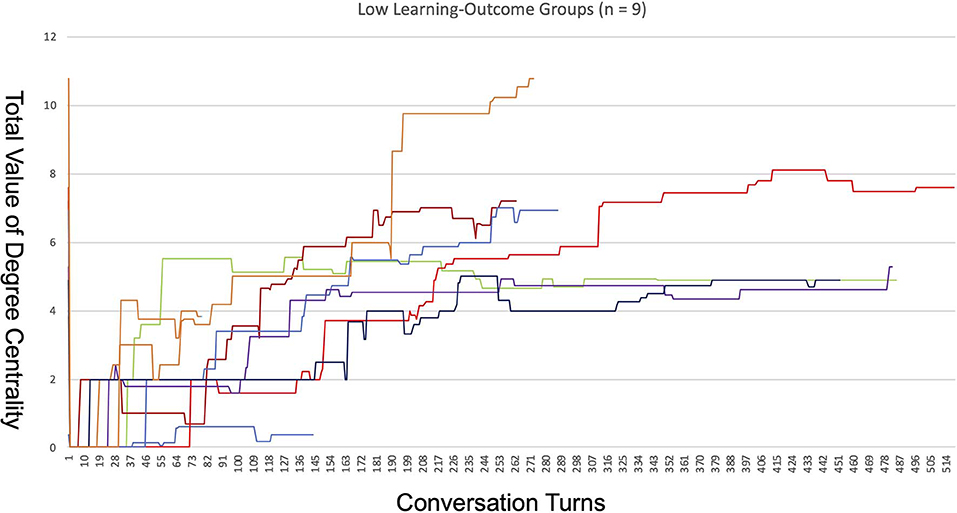
Figure 3. Temporal change in the total value of degree centrality of the vocabulary network in low conceptual understanding groups.
For examining our interpretations of the SSNA results, we further conducted discourse analysis. Students in a high conceptual understanding group engaged in their discourse as follows (The original discourse was in Japanese and translated into English by the first author. SSNA vocabulary is in bold.):
Students engaged in creating shared understanding of how the human immune system works. Student A was a key person to externalize shared understanding through monitoring confirmation by others (B and C). Student B played the role of recording ideas on the paper, and so frequently revoiced student A's externalizations to support the fluency of the group and check for a shared understanding (turn #159, #161, #163, #167, #169, #171, and #173). In contrast, student C went beyond just creating shared understanding to generative collaborative actions by up-taking student A's talk (turn #165). Student C is considered to have attempted to improve student A's idea based on self-understanding by asking important questions from a different perspective (e.g., “Wait a minute. How about memory T-cells?”). Within the discourse segment, students A and C were more engaged in generative collaborative actions.
Students in a low conceptual understanding group, on the contrary, could not sustain their engagement in their ideas. They were collaboratively constructing sentences for their explanatory discourse. Their discourse, however, was digressed from their engagement in ideas by a student's turn (“Why do not we just follow this [picture in their studied document]?”). In the conversation turn, the student proposed the transformation of their learning goal into the performance goal, and the other students quickly accepted this proposal.
Discussion
Our first research question was, Is there any significant difference in students' use of their study topic words between high and low conceptual understanding groups in the jigsaw instruction? If groups of students in the jigsaw instruction engage in discourse around their study topic with different weights of priority, how would it influence the conceptual understanding? The question was examined by calculating term-frequencies, a measure of a unique contribution of words related to the study topic in their discourse. Our results revealed no significant differences in the means of term-frequencies across groups. It suggests that students in the context of the jigsaw instruction engage in discourse with a similar recognition of importance of their study topic. If significant differences were seen in the conceptual understanding, therefore, the group differences should not be attributed to what students talk about in their jigsaw group activity.
Our second research question was, Is there any difference in the pattern of social process in the discourse between high and low conceptual understanding groups? That is, how differently students engage in their collaborative discourse in high and low conceptual understanding groups. The second question was examined by analyzing student discourse using the SSNA of vocabularies and complementary discourse analysis. Results revealed that students in high conceptual understanding groups were more engaged in collective knowledge advancement by producing more ideas in the early stage. This finding suggests that how a collaborative discourse is initiated and sustainably continued may be the key to a deeper conceptual understanding in the jigsaw instruction. Our results here replicate the findings from recent studies on the regulation of collaboration. When students are successfully involved in a collaboration, they regulate the collaboration in a socially shared way (Hadwin et al., 2018). In the metacognitive process of collaboration, the most crucial aspect is planning. In the early stage of their collaboration, students need to establish their goals and plans on how to discuss their ideas by socially sharing the metacognitive knowledge of what good collaboration should be like for them. Our results suggesting the high conceptual understanding groups' engagement with more ideas in their early stage lead the authors to future studies to examine a hypothesis that the high conceptual understanding groups may be successful in establishing their agreement of how to proceed collaboration.
Taken results together, it is concluded in this study that early agreement seemed to enable more integration of concepts in high conceptual understanding groups, while low conceptual understanding groups took more time to reach high levels of the degree centrality.
Based on our discussion above, we further consider the directions of future research. First, our proposed evaluation procedure should be further developed so that we can assess students' learning processes in a formative way. If we can identify the pattern of discourse leading to unsuccessful conceptual understanding in the middle of students' learning, we can implement further appropriate instructional supports to improve their discourse toward more successful conceptual understanding. In this study, we started how to identify the differences in students' discourse processes in their knowledge-creation practices after they had finished their learning. Further research should be conducted to examine if we can appropriately predict the differences in their learning processes.
Second, another direction of future research should be to adapt existing instructional supports in a way that we can use to support learning as knowledge-creation. One promising instructional support is collaboration scripts (Fischer et al., 2013). A variety of external scripts have been developed for supporting contexts of collaboration but not for learning as knowledge-creation yet. Further, in-depth discourse analyses are needed to examine what scripts successful groups have used in the jigsaw instruction. Our proposed evaluation procedure could help researchers to identify the segments of discourse that they have to examine for the purpose.
We finally state several limitations of this study for future works. First, we have mainly focused on the quantitative analyses but not sufficiently conducted the mixed-methods approach. The quantitative analyses could let researchers pay attention to significant segments of discourse. They cannot provide them with sufficient information without complementary qualitative analyses. Second, the algorithm to evaluate the knowledge creation practices could be further improved. The current algorithm is partially temporal but not powerful enough to demonstrate the dynamic change of ideas discussed in discourse. It can evaluate how new ideas might appear but not how they might disappear in discourse. The algorithm should be improved to calculate how long the linkage between nodes is activated.
Data Availability Statement
The datasets generated for this study will not be made publicly available for the privacy policy for the subjects.
Ethics Statement
The studies involving human participants were reviewed and approved by Shizuoka University. Written informed consent to participate in this study was provided by the participants' legal guardian/next of kin.
Author Contributions
JO as the corresponding author designed the instruction and collected data with RO. JO also conducted the SSNA part of the data analysis. TT contributed to the article by conducting the Term-Frequency analysis as an expert in the natural language processing field.
Funding
This work was supported by JSPS KAKENHI Grant Number 16H0187.
Conflict of Interest
The authors declare that the research was conducted in the absence of any commercial or financial relationships that could be construed as a potential conflict of interest.
Acknowledgments
An earlier version of this article was presented at EARLI SIG 1 conference in 2018 and CSCL 2017. Constructive feedback from the audience is acknowledged for modifying the manuscript.
References
Brown, A. L., and Campione, J. C. (1996). “Psychological theory and the design of innovative learning environments: on procedures, principles, and systems,” in Innovations in Learning: New Environments for Education, eds L. Schauble and R. Glaser (Mahwah, NJ: Lawrence Erlbaum Associates), 289–325.
Feldman, R., and Sanger, J. (2007). The Text Mining Handbook: Advanced Approaches in Analyzing Unstructured Data. Cambridge: Cambridge University Press. doi: 10.1017/CBO9780511546914
Fischer, F., Kollar, I., Stegmann, K., and Wecker, C. (2013). Toward a script theory of guidance in computer-supported collaborative learning. Educ. Psychol. 48, 56–66. doi: 10.1080/00461520.2012.748005
Hadwin, A. F., Järvelä, S., and Miller, M. (2018). “Self-regulation, co-regulation, and shared regulation in collaborative learning environments,” in Handbook of Self-Regulation of Learning and Performance, 2nd Edn, eds. D. H. Schunk and J. A. Greene (New York, NY: Routledge), 83–106. doi: 10.4324/9781315697048-6
Hmelo-Silver, C., and Pfeffer, M. G. (2004). Comparing expert and novice understanding of a complex system from the perspective of structures, behaviors, and functions. Cogn. Sci. 28, 127–138. doi: 10.1207/s15516709cog2801_7
Jacobson, M. J. (2001). Problem solving, cognition, and complex systems: differences between experts and novices. Complexity 6, 1–9. doi: 10.1002/cplx.1027
Johnson, R. B., and Onwuegbuzi, A. J. (2006). Mixed methods research: a research paradigm whose time has come. Educ. Res. 33, 14–26. doi: 10.3102/0013189X033007014
Lee, A. V. Y., and Tan, S. C. (2017). Promising ideas for collective advancement of communal knowledge using temporal analytics and cluster analysis. J. Learn. Anal. 4, 76–101. doi: 10.18608/jla.2017.43.5
Ma, L., Matsuzawa, Y., Chen, B., and Scardamalia, M. (2016). “Community knowledge, collective responsibility: the emergence of rotating leadership in three knowledge building communities,” in Transforming Learning, Empowering Learners: The International Conference of the Learning Sciences (ICLS) 2016, Vol. 1, eds C. K. Looi, J. L. Polman, U. Cress, and P. Reimann (Singapore: International Society of the Learning Sciences), 615–622.
Martinez, A., Dimitriadis, Y., Rubia, B., Gomez, E., and de la Fuente, P. (2003). Combining qualitative evaluation and social network analysis for the study of classroom social interactions. Comput. Educ. 41, 353–368. doi: 10.1016/j.compedu.2003.06.001
Miyake, N. (1986). Constructive interaction and the iterative process of understanding. Cogn. Sci. 10, 151–177. doi: 10.1207/s15516709cog1002_2
Miyake, N., and Kirschner, P. A. (2014). “The social and interactive dimensions of collaborative learning,” in The Cambridge handbook of the learning sciences, 2nd Edn, ed K. Sawyer (New York, NY: Cambridge University Press), 418–438. doi: 10.1017/CBO9781139519526.026
Oshima, J., Oshima, R., and Fujita, W. (2018). A mixed-methods approach to analyze shared epistemic agency in jigsaw instruction at multiple scales of temporality. J. Learn Anal. 5, 10–24. doi: 10.18608/jla.2018.51.2
Oshima, J., Oshima, R., and Matsuzawa, Y. (2012). Knowledge building discourse explorer: a social network analysis application for knowledge building discourse. Educ. Tech. Res. Dev. 60, 903–921. doi: 10.1007/s11423-012-9265-2
Paavola, S., and Hakkarainen, K. (2005). The knowledge creation metaphor—an emergent epistemological approach to learning. Sci. Educ. 14, 535–557. doi: 10.1007/s11191-004-5157-0
Paavola, S., Lipponen, L., and Hakkarainen, K. (2004). Models of innovative knowledge communities and three metaphors of learning. Rev. Educ. Res. 74, 557–576. doi: 10.3102/00346543074004557
Sawyer, K., and (ed.). (2014). The Cambridge Handbook of the Learning Sciences, 2nd Edn. New York, NY: Cambridge University Press. doi: 10.1017/CBO9781139519526
Scardamalia, M. (2002). “Collective cognitive responsibility for the advancement of knowledge,” in Liberal Education in a Knowledge Society, ed B. Smith (Chicago, IL: Open Court), 67–98.
Scardamalia, M., Bransford, J., Kozma, B., and Quellmalz, E. (2012). “New assessments and environments for knowledge building,” in Assessment and Teaching of 21st Century Skills, eds P. Griffin, B. McGraw, and E. Care (New York, NY: Springer Science+Business Media), 231–300. doi: 10.1007/978-94-007-2324-5_5
Keywords: assessment, idea emergence, subject-matter, collaborative learning, socio-semantic network analysis, text-mining algorithm
Citation: Oshima J, Tsunakawa T and Oshima R (2020) An Assessment of Idea Emergence in Subject-Matter Collaborative Learning. Front. Educ. 5:21. doi: 10.3389/feduc.2020.00021
Received: 24 August 2019; Accepted: 25 February 2020;
Published: 10 March 2020.
Edited by:
Carmen Tomas, University of Nottingham, United KingdomReviewed by:
Abelardo Pardo, University of South Australia, AustraliaJill Willis, Queensland University of Technology, Australia
Copyright © 2020 Oshima, Tsunakawa and Oshima. This is an open-access article distributed under the terms of the Creative Commons Attribution License (CC BY). The use, distribution or reproduction in other forums is permitted, provided the original author(s) and the copyright owner(s) are credited and that the original publication in this journal is cited, in accordance with accepted academic practice. No use, distribution or reproduction is permitted which does not comply with these terms.
*Correspondence: Jun Oshima, joshima@inf.shizuoka.ac.jp