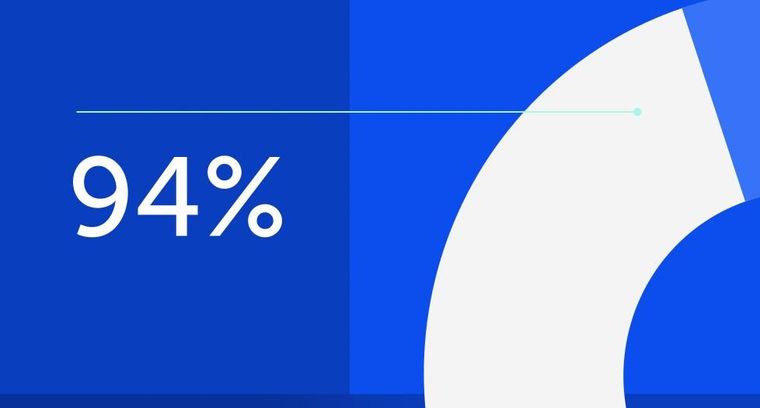
94% of researchers rate our articles as excellent or good
Learn more about the work of our research integrity team to safeguard the quality of each article we publish.
Find out more
ORIGINAL RESEARCH article
Front. Educ., 04 October 2019
Sec. Assessment, Testing and Applied Measurement
Volume 4 - 2019 | https://doi.org/10.3389/feduc.2019.00103
The regular formative assessment of students' abilities across multiple school grades requires a reliable and valid vertical scale. A vertical scale is a precondition not only for comparing assessment results and measuring progress over time, but also for identifying the most informative items for each individual student within a large item bank independent of the student's grade to increase measurement efficiency. However, the practical implementation of a vertical scale is psychometrically challenging. Several extant studies point to the complex interactions between the practical context in which the scale is used and the scaling decisions that researchers need to make during the development of a vertical scale. As a consequence, clear general recommendations are missing for most scaling decisions. In this study, we described the development of a vertical scale for the formative assessment of third- through ninth-grade students' mathematics abilities based on item response theory methods. We evaluated the content-related validity of this new vertical scale by contrasting the calibration procedure's empirical outcomes (i.e., the item difficulty estimates) with the theoretical, content-related item difficulties reflected by the underlying competence levels of the curriculum, which served as a content framework for developing the scale. Besides analyzing the general match between empirical and content-related item difficulty, we also explored, by means of correlation and multiple regression analyses, whether the match differed for items related to different curriculum cycles (i.e., primary vs. secondary school), domains, or competencies within mathematics. The results showed strong correlations between the empirical and content-related item difficulties, which emphasized the scale's content-related validity. Further analysis showed a higher correlation between empirical and content-related item difficulty at the primary compared with the secondary school level. Across the different curriculum domains and most of the curriculum competencies, we found comparable correlations, implying that the scale is a good indicator of the math ability stated in the curriculum.
Modern computer technology can be used as a tool for providing formative feedback in classrooms on a regular basis (e.g., Hattie and Brown, 2007; Brown, 2013). It allows for implementing complex measurement models and item-selection algorithms that support teachers in providing objective, reliable, and valid feedback (e.g., Glas and Geerlings, 2009; Wauters et al., 2010; Tomasik et al., 2018). In Northwestern Switzerland, four cantons—Aargau, Basel-Landschaft, Basel-Stadt, and Solothurn—joined forces to develop a computer-based, formative feedback system for classrooms (Tomasik et al., 2018; see also https://www.mindsteps.ch/) to serve teachers and their nearly 100,000 third- through ninth-grade students, as an instrument for data-based decision making (Schildkamp et al., 2013; van der Kleij et al., 2015). The data-based decision making approach to formative assessments (van der Kleij et al., 2015) originates from the No Child Left Behind Act in the United States and places a strong emphasis on monitoring the attainment of specific learning targets through objective data.
The specific purpose of the computer-based, formative feedback system is to support teachers and students in collecting objective information about students' current abilities (i.e., strengths and weaknesses) as well as learning progress within the domains and competencies stated in the curriculum for four school subjects: German, the schools' language; English and French, the two foreign languages taught; and mathematics (Tomasik et al., 2018). To provide targeted feedback (i.e., objective data targeted on students and teachers' specific needs), the system is conceptualized as an item bank with several thousand assessment items that teachers and students can select based on curriculum-related, as well as empirical, criteria, such as curriculum-related competence levels or empirical item difficulty estimates. Depending on their assessment specifications, teachers and students receive reports about the students' current ability in particular domains, or their mastery of particular competence levels or topics. In line with the data-based decision making approach to formative assessments, teachers and students can use the assessment outcomes to define appropriate learning goals, evaluate progress in realizing these goals over time, and adjust teaching, learning environments, or goals, if necessary (Hattie and Timperley, 2007; van der Kleij et al., 2015).
Two basic prerequisites for implementing such a computer-based system for data-based decision making are clear content specifications, which guide the assessment items' development for the item bank (Webb, 2006), and a vertical measurement scale, which allows for monitoring and comparing students' abilities over several school grades (Young, 2006). For Northwestern Switzerland, the competence-based curriculum Lehrplan 21, which was made available to German-speaking cantons in Switzerland in autumn 2014 (D-EDK, 2014), is an obvious choice as a basis for the content specifications. However, it is a more challenging endeavor to develop a vertical measurement scale to represent students' competence levels over seven school grades (i.e., from the third to the ninth grades) as stated in the curriculum.
As a basis for representing student abilities on a metrical vertical scale, the system uses item response theory (IRT, Lord, 1980; de Ayala, 2009), or rather the Rasch model (Rasch, 1960), as an underlying measurement model. One advantage of this measurement approach is that it allows for directly comparing assessment results related to different item sets. Thus, each student can work on a targeted selection of items within the item bank, but still compare results with those of other students, as well as with their own results from earlier assessments. In addition, a vertical IRT scale does not only allow for representing student ability across multiple school grades but it also allows for representing item difficulty across a broad difficulty range. Thanks to its feature of representing student ability and item difficulty on the same scale, the IRT approach supports targeted item selection through teachers and students as well as algorithms for computer-adaptive testing (CAT), which use preliminary ability estimates during assessment for selecting the most appropriate and informative items for each individual student (Wainer, 2000; van der Linden and Glas, 2010).
However, the development of such a vertical IRT scale is rather complex. To establish the scale, items representative of the underlying content specifications need to be calibrated based on response data from students from the target grade groups. This procedure involves various scaling decisions such as choice of the test specifications, data collection design, number of linking items, or linking calibration procedure. Results from previous studies on vertical scaling indicated that the interpretation of growth or progress on a vertical IRT scale might depend on the concrete combination of such scaling decisions (see Harris, 2007; Briggs and Weeks, 2009; Kolen and Brennan, 2014, for a general overview). However, the results from these studies are mixed due to various interactions between the different scaling decisions (Hanson and Béguin, 2002; Pomplun et al., 2004; Tong and Kolen, 2007; Briggs and Weeks, 2009; Lei and Zhao, 2012; see also Harris, 2007), and do not provide clear guidance for the practical implementation of vertical scales based on IRT methods. Simulation studies might help investigate selected combinations of scaling decisions systematically. However, in practice, the best combination of decisions might depend largely on the practical context such as the vertical scale's specific measurement objectives, changes in ability distribution across grades (e.g., Keller and Hambleton, 2013), or the extent to which the data meet strict assumptions of unidimensionality and parameter invariance in the underlying IRT model (Béguin et al., 2000; Tong and Kolen, 2007; Pohl et al., 2015). We are not aware of any studies that considered all these factors in order to investigate potential interaction effects and provide guidance for particular practical contexts. Furthermore, such an amount of different factors goes way beyond the scope of a single simulation study.
Against this backdrop and in contrast to previous studies on vertical scaling, in this paper, we suggest validating a new vertical IRT scale and the related scaling decisions by contrasting the calibrated items' empirical difficulties with the items' theoretical content-related difficulties. Thus, we extend previous research on vertical IRT scaling by using an external criterion for validating the vertical scale; specifically, a criterion that allows for justifying the decisions made during test development and item calibration, while considering the concrete, latent construct or ability to be measured, and for verifying the item difficulty parameters and the related growth pattern as the true ones (Harris, 2007; Tong and Kolen, 2007; Ito et al., 2008; Briggs and Weeks, 2009; Dadey and Briggs, 2012). We illustrate this procedure by validating the vertical math scale for formative assessment for third- through ninth-grade students in Northwestern Switzerland by means of cross-sectional data from a pretest calibration sample. In particular, we investigate the extent to which the empirical item difficulty parameters resulting from the Rasch calibration reflect the items' content-related difficulties based on their assignment to specific competence levels of the curriculum Lehrplan 21. Such a content-related validation criterion was missing from previous studies on vertical scaling.
To monitor students' abilities over time, a vertical measurement scale is required, which refers to “an extended score scale that spans a series of grades and allows the estimation of student growth along a continuum” (Young, 2006, p. 469; see also Harris, 2007; Briggs, 2013; Kolen and Brennan, 2014). Such a scale is the basis for comparing the outcomes of various consecutive measurement occasions and, thus, for measuring progress, analyzing students' growth in relation to vertically moderated standards, and computer-adaptive testing across grades (e.g., Cizek, 2005; Ferrara et al., 2005; Dadey and Briggs, 2012). A precondition for justifying a vertical scale is the assumption that the measured abilities or competencies are continuously stimulated and that they increase over time (Young, 2006). In contrast to horizontal scales, which represent one specific age or grade group's abilities, vertical scales combine test forms or item sets that vary in their mean difficulty, reflecting the broad ability range that must be covered when assessing ability over several school grades. However, even though the different assessment forms refer to different difficulty levels, the underlying latent construct needs to remain constant from a content perspective. Otherwise, a unidimensional vertical scale cannot be justified.
In line with the requirements for a vertical scale, the curriculum Lehrplan 21 states a continuous development of students' math competencies throughout compulsory school (D-EDK, 2016a). It describes the competencies that students should acquire from kindergarten until the end of compulsory school, providing teachers and schools with a basis for planning their teaching and evaluating students' progress [(D-EDK, 2014, 2016b); see also www.lehrplan.ch]. Within the subject of mathematics, the curriculum is structured hierarchically into 3 domains (i.e., “number and variable,” “form and space,” and “measures, functions, data, and probability”), 26 competencies, and various competence levels (D-EDK, 2016a). For each competency, the curriculum calls for a continuous development of it over the school years, and mastery of lower competence levels is a precondition for mastering more advanced competence levels [see also (BIFIE, 2011; Reusser, 2014)]. Furthermore, the curriculum delineates three different cycles—kindergarten to second grade, the third to sixth grades, and the seventh to ninth grades (i.e., secondary school); defines basic requirements for each cycle (i.e., the minimal competence levels that students need to have mastered at the end of each cycle); and states two points of orientation at the end of the fourth and eighth grades. The cycles, basic requirements, and points of orientation anchor the competence levels and, thus, the development of competencies, across the 11 compulsory school years. However, the curriculum focuses much more on the development of students' competence levels across grades than on the specific competencies within a particular school grade, thereby following a domain definition of growth as defined by Kolen and Brennan (2014, pp. 429–431).
Figure 1 provides an example of a mathematics competency, namely MA.1.A.2, which covers “counting, ordering, estimating” and is part of the domain “number and variable” (D-EDK, 2016a). Within this competency, the mathematics curriculum distinguishes between 10 different competence levels of increasing difficulty (i.e., levels a to j). For each competence level, it provides detailed descriptions of what students should know to master the level. For example, level c is described as following: “Students can count forward up to 100 in steps of 1, 2, 5, or 10. They can order numbers up to 100 (e.g., on a number ray or a table)” (D-EDK, 2016a). The first three levels, a to c, belong to the curriculum's first cycle, whereas the gray, highlighted level c refers to this cycle's basic requirements. Levels d to g refer to the curriculum's second cycle, with level g including this cycle's basic requirements. Levels h to j belong to the third cycle, with level j providing the basic requirements. In addition, two red, dotted lines serve as orientation for students' competence levels at the end of grades 4 (i.e., level f) and 8 (i.e., level j, which simultaneously represents the basic requirements of cycle 3). Thus, the curriculum contains detailed descriptions for distinct competencies and levels, as well as references to minimal standards for certain school grades. As such, it serves as an ideal basis (i.e., the content framework) for developing items to assess students' ability formatively at various points in time during their compulsory school years.
Figure 1. Extract from the curriculum Lehrplan 21: Example of a mathematics competency and a description of its competence levels. Orange, blue, and green frames indicate the curriculum's three cycles: cycle 1 = kindergarten through second grade; cycle 2 = third through sixth grades; cycle 3 = seventh through ninth grades. Gray levels refer to the basic requirements within a cycle, and red, dotted lines serve as orientations at the ends of fourth and eighth grades. From D-EDK (2016a) Copyright 2016 by Deutschschweizer Erziehungsdirektoren-Konferenz. Reprinted with permission.
To establish a vertical measurement scale (i.e., a vertical IRT scale), items reflecting the content framework need to be calibrated based on response data from students from the target grade groups. Kolen and Brennan (2014, pp. 431–434) distinguish between three different test designs for collecting these data: (1) equivalent group designs, (2) common item designs, and (3) scaling test designs. In equivalent group designs, groups with equivalent ability distribution within a school grade are randomly assigned to answer items related to their own, adjacent lower, or adjacent higher grade. The linking within each grade is based on the assumption that all groups have comparable ability distributions. The linking between grades is based on items administered to two adjacent grades. Administering identical items to students from adjacent grades is the basic idea of common item designs, the second design type. The advantage of this design type is that it does not require equivalent groups, only common items (i.e., linking items), to link several item blocks. Linking items serves not only to establish a link between two grades, but also to align overlapping item blocks within one grade. Scaling test designs, the third design type, is similar to common item designs to the extent that students from different school grades solve identical items. However, when applying a scaling test design, common items are shared not only between adjacent grades. Instead, one block of items, namely the scaling test, is administered to all involved grades. Besides the scaling test, students from each grade answer items related to their specific grade.
Of these three designs, scaling test designs are the most consistent with a domain definition of growth and are the first choice in such a context from a theoretical perspective (Kolen and Brennan, 2014). In contrast, common item designs are the easiest to implement in practice under the condition that it is reasonable to administer the same items to students from adjacent grades from a content perspective (Kolen and Brennan, 2014). Equivalent group designs require more complex administration procedures within one school grade to ensure samples with equivalent ability distributions. Scaling test design requires that identical items be administered to students from even more school grades, which is difficult to justify from a content perspective if the target population spans seven school years, as in our case.
After administering the items to students by means of the data collection designs described above, the items need to be calibrated to establish a vertical measurement scale. Within the context of the Rasch model, item calibration refers to establishing model fit and estimating the difficulty parameter, βi, of an item i based on response data by means of maximum likelihood estimation procedures (Vale and Gialluca, 1988; Eggen and Verhelst, 2011). Generally, two different procedures are used to link IRT-based item difficulty parameters to a common vertical scale across multiple grades: concurrent and grade-by-grade calibration (Briggs and Weeks, 2009; Kolen and Brennan, 2014). Under the concurrent procedure (Wingersky and Lord, 1983), all item parameters are estimated in one single calibration run, whereby different underlying population-ability distributions need to be specified for each grade group (DeMars, 2002; Eggen and Verhelst, 2011). By factoring in the groups' differences in ability, this procedure directly maps all item parameters onto one common scale by means of linking items shared by two adjacent grades.
In contrast, item parameters are estimated separately for each grade under the grade-by-grade calibration procedure. These parameters are then transformed onto one common scale by means of linear transformations. Different methods allow for determining the linking constants, of which one of the most accurate and popular methods is the Stocking and Lord method (Stocking and Lord, 1983; e.g., Briggs and Weeks, 2009; Kolen and Brennan, 2014), which determines linking constants by minimizing differences between the linking items' item characteristic curves between two grades. To link parameters over more than two grades, several transformation steps are required for grades that are placed further away from the base grade level.
Previous research comparing concurrent and grade-by-grade calibration procedures yielded mixed results and did not provide clear guidance for practical implementation of vertical scales based on IRT methods. Some studies identified concurrent calibration as superior to grade-by-grade calibration (e.g., Kim and Cohen, 1998; Hanson and Béguin, 1999), whereas others reported the opposite (e.g., Béguin et al., 2000; Ito et al., 2008). In addition, several studies point out diverse interactions between the calibration procedure and various other decisions during the development of the vertical scale, such as the choice of IRT model, data collection design, number of linking items, or test specifications (Hanson and Béguin, 2002; Pomplun et al., 2004; Tong and Kolen, 2007; Briggs and Weeks, 2009; Lei and Zhao, 2012; see also Harris, 2007).
From a theoretical perspective, concurrent calibration might be superior to grade-by-grade calibration. According to Kolen and Brennan (2014, p. 444), it “is expected to produce more stable results because it makes use of all of the available information for parameter estimation.” Furthermore, the concurrent procedure is less prone to errors because it does not require the estimation of linking constants, which can elicit additional estimation errors, and it is more efficient because it requires only one calibration run (Briggs and Weeks, 2009). However, Kolen and Brennan (2014) also listed several arguments that support the use of grade-by-grade calibration in a practical context (e.g., Hanson and Béguin, 2002). First, grade-by-grade calibration has the advantage of allowing for the direct comparison of item parameter estimates between two adjacent grades and, thus, for investigating potential deficiencies in parameter invariance across school grades. Investigating parameter invariance is also possible under the concurrent calibration procedure, but it requires—depending on the calibration software—additional calibration runs for subsamples of data. Second, separate calibrations for each grade group are based on smaller and simpler data matrices than in concurrent calibration, which includes all available data at once. As a result, the estimation procedure converges faster, so convergence problems are less likely. Last but not least, grade-by-grade calibration might be more robust against the violation of the unidimensionality assumption because it only considers data from two school grades (Béguin et al., 2000; Béguin and Hanson, 2001; Hanson and Béguin, 2002). Thus, separate calibration might be the first choice if the empirical data cannot be perfectly described through the IRT model.
Against this backdrop, Hanson and Béguin (2002), as well as Kolen and Brennan (2014), recommended applying both procedures when developing a vertical scale. Differences between the two procedures' outcomes might help detect serious problems in the calibration process, such as multidimensionality and, thus, model misfit (Hanson and Béguin, 2002). However, in the case of differences between the outcomes of the two procedures, a decision needs to be made about which procedure to use for the final scale. Making this decision is very difficult in practice and requires additional information about the purpose of the scale or the assessment system (Kolen and Brennan, 2014). Previous literature on vertical scaling provides limited information on how to make this decision.
The aim of this study is to validate a vertical Rasch scale and its underlying scaling decisions for the formative assessment of students' ability in mathematics from the third through ninth grades by contrasting the calibrated items' empirical difficulties with the items' theoretical content-related difficulties derived from the content framework. In particular, we investigated the scale's validity for mapping competency development from the third through ninth grades, as stated in the curriculum Lehrplan 21. To this end, we aim to answer the following two research questions:
I. Do the item calibration's empirical outcomes (i.e., item difficulty estimates) reflect the increasing complexity of the competence levels represented by the items and, thus, match the items' theoretical, content-related difficulties?
II. Does the match between the empirical item difficulty estimates and the theoretical, content-related item difficulties differ for items related to different curriculum cycles, domains, or competencies?
The research questions were addressed by means of correlation and multiple regression analysis based on data from a cross-sectional calibration study with third- through ninth-grade students from Northwestern Switzerland. We assumed a strong positive relationship between the empirical item difficulty estimates and content-related item difficulties. Furthermore, we hypothesized that the relationship strength would be similar across different curriculum cycles, domains, and competencies.
Our content experts identified 18 out of the 26 different mathematics competencies from the curriculum Lehrplan 21 (D-EDK, 2016a) as assessable through computer-based items with clear answer formats (e.g., multiple choice, short text, drag-and-drop items). Within these competencies, they identified competence levels ranging from the basic requirements of cycle 1 to the penultimate or ultimate competence level of cycle 3 as relevant for students in the third through ninth grades. The number of competence levels within this target range varies between 5 and 10, resulting in some competence levels that span a broader part of primary and secondary school than others. To compare the levels' relative difficulty over the different competencies, we aligned the competence levels according to the basic requirements for cycles 1, 2, and 3 and based on the orientation lines for the end of grades 4 and 8 (cf. Figure 1). This procedure's outcome is a matrix presented in Table 1. On one hand, this matrix served as a basis for the item calibration test design, which we describe in more detail in the next section, and for item development. Item developers were instructed to construct items that could be clearly assigned to one competence level each (e.g., MA.1.A.2.f) and, therefore, were related to one single competency (MA.1.A.2), domain (MA.1.A), and curriculum cycle (C2). On the other hand, we used the matrix to translate each competence level into a score on a scale from 1 to 11, which served as a measure of content-related difficulty (CRD) for our analyses (see Table 1, last row). Competence levels spanning more than one scale unit were represented by the underlying scale units' mean (e.g., CRD = 4.5 for level f of competency MA.1.A.2).
Table 1. Overview of 18 mathematics competencies from curriculum Lehrplan 21 and the alignment of their competence levels with the scale for CRD.
To establish a vertical scale for measuring students' competence levels in mathematics from the third through ninth grades, we developed a common item design, which included 520 mathematics items representing the 18 competencies described in Table 1. Table 2 provides a macro-level design overview. Generally, we administered a combination of grade-specific and linking items to each grade, with 240 grade-specific items administered to one grade only (highlighted in light blue in Table 2) and 280 linking items administered to two adjacent grades (highlighted in dark blue in Table 2) to link the various grades. The design contained one exception to the general structure. Due to the broad, overlapping ability range within the eighth and ninth grades, the design disregarded grade-specific items for the eighth grade for the benefit of a larger amount of linking items dedicated to both grades.
To reduce the workload for individual students, we further divided the items dedicated to each grade over five booklets. Most of the items were included in two booklets, whereas grade-specific items were included in two booklets within one grade group, and linking items were included in two booklets of adjacent grade groups. To balance the design regarding the number of items per competency, 40 out of the 80 linking items between the eighth and ninth grades were included in 4 different booklets (see Table 2 for an overview of the number of observations per item per grade and in total). An extract from the resulting design is displayed in Table 3. In total, the design comprised 35 linked booklets with 32 items in each booklet.
Four practical requirements guided content distribution within the design. First, we aimed to include approximately 29 items from each of the 18 competencies in the design (i.e., 520 divided by 18 competencies). Second, the number of items per competence level should correspond to the width of the competence levels as displayed in Table 1. Third, the booklets should comprise pairs of items related to the same competency to prevent constant switching between topics. Last but not least, each booklet should include as many different competencies as possible, given the other three requirements. As a result, the 35 booklets comprised, on average, M = 12.457 items of the domain “number and variable” (SD = 1.379), M = 8.8 items of the domain “form and space” (SD = 1.828), and M = 10.743 items related to the domain “measures, functions, data, and probability” (SD = 1.686). Each booklet included, on average, items related to M = 12.829 different competencies (SD = 1.361), and each competency was represented by M = 28.889 items (SD = 4.351).
In spring 2017 and 2018, we administered the 35 mathematics booklets to a sample of 2,733 students from schools in Northwestern Switzerland. The mathematics booklets were available for teachers besides other assessment templates in a first version of the computer-based assessment system MINDSTEPS (Tomasik et al., 2018). Teachers were invited to administer the booklets to their students during school lessons to support the calibration of the system's item pool. In line with the American Psychological Association's Ethical Principles and Code of Conduct, as well as with the Swiss Psychological Society's Ethical Guidelines, written informed consent from students and their parents was not required because this study was based on the assessment of normal educational practices and curricula in educational settings (i.e., solving a computer-based mathematics assessment at school; Swiss Psychological Society, 2003; American Psychological Association, 2017). Given that the assessments were very low-stakes for students and teachers, we excluded students with a high percentage of missing responses (i.e., students who did not answer one-third or more of the items presented to them). This yielded a calibration sample of N = 2,436 students. Table 4 displays the original sample size per grade, the percentage of students excluded prior to the calibration due to too many missing responses, as well as the size of the final calibration sample per grade in total, for grade-specific items and for linking items.
Table 4. Overview of the calibration sample per grade: total sample, percentage of excluded students, and final calibration sample in total, for grade-specific items and for linking items.
The computer-based assessment system automatically scored students' responses, with wrong or omitted responses scored as 0, and correct responses scored as 1. To investigate parameter invariance across school grades and to detect possible calibration problems (Hanson and Béguin, 2002; Kolen and Brennan, 2014), we used both concurrent and grade-by-grade calibration procedures to generate marginal maximum likelihood (MML) estimates of the item parameters from the Rasch model (Rasch, 1960). For both procedures, we used the software package TAM (Kiefer et al., 2016) within the development environment R (R Core Team, 2016). First, the dichotomized response data were calibrated concurrently over all school grades. Separate ability distributions were estimated for each school grade to ensure unbiased parameter estimation (DeMars, 2002; Eggen and Verhelst, 2011). The mean of the sixth-grade population (i.e., the center of the vertical scale) was constrained to 0. Second, the response data were calibrated separately for each school grade. All item parameters from this grade-by-grade calibration were subsequently transformed onto the sixth-grade scale using the characteristic curve transformation method by Stocking and Lord (1983, see also Kolen and Brennan, 2014; González and Wiberg, 2017). The transformation resulted in two item difficulty parameters for each linking item. By means of the unit-weighted average method (McKinley, 1988), we combined the two difficulty estimates for each linking item into one single parameter.
Outcome differences from the two calibration procedures would indicate multidimensionality, thereby suggesting scale instability (e.g., Béguin and Hanson, 2001). However, in our study, the two procedures' results were very similar and did not indicate any technical problems regarding item calibration. The item difficulty parameters resulting from the final calibration correlated with r = 0.999 over the entire item pool. Furthermore, Figure 2 shows the population means, estimated by means of the weighted maximum likelihood (WML) method proposed by Warm (1989), and the related 95% confidence interval based on the final calibration run for the concurrent and grade-by-grade calibration for each of the seven school grades. Both procedures resulted in similar trends for the development of the mean ability from the third to the ninth grades with a steep increase in mathematics ability throughout primary school, followed by a decreased ability progression throughout secondary school. Differences in the groups' mean ability between the two procedures were largest for the third grade (i.e., Mconc = −3.439; SEconc = 0.060, Mgrade = −3.557, SEgrade = 0.059), decreased from the fourth to the sixth grade (i.e., both calibrations' reference grade), and were very small for all secondary school grades (i.e., the seventh to ninth grades). None of the differences was statistically significant. We concluded from these findings that the established unidimensional vertical Rasch scale was a stable scale from a psychometric perspective.
Figure 2. Estimated population means based on the final calibration run for the concurrent and grade-by-grade calibrations, including the 95% confidence interval.
To investigate whether the items fit a unidimensional vertical Rasch scale, we analyzed, for each item, the number of observations and response patterns, item discrimination, item fit to the overall Rasch model, and parameter invariance across school grades. We defined four criteria for identifying problematic items, which we excluded from the final vertical scale. First, items with equal to or less than three correct or incorrect responses were excluded because such a low variation within the response pattern would result in large standard errors in the particular item's difficulty estimate. Moreover, the low variation might indicate a large mismatch between the item's target difficulty as estimated by the content experts during test development and the item's true difficulty. Second, we excluded items with a discrimination of rit ≤ 0.10. Third, item fit was analyzed based on the concurrent calibration by means of the root mean square deviation (RMSD), a standardized index of the difference between the expected and observed item characteristic curve (Oliveri and von Davier, 2011). The RMSD was calculated separately for each school grade. For linking items assigned to two school grades, we additionally computed the weighted root mean square deviation (WRMSD; von Davier et al., 2016; Yamamoto et al., 2016). An RMSD value of 0 indicates a perfect fit of the item with the model, whereas higher values indicate a poorer fit. In this study, we rejected items with a MaxRMSD > 0.20 (i.e., with RMSD > 0.20 in at least one school grade), as well as linking items with a WRMSD > 0.20. Fourth, we investigated parameter invariance (e.g., Rupp and Zumbo, 2016) over school grades by comparing linking items' grade-specific item difficulty estimates with grade-by-grade calibration. For each linking item set (e.g., linking items between the third and fourth grades), we plotted a 99% confidence interval based on each item pair's mean difficulty, each pair's mean standard error, and the item set's overall mean for each of the two grades (cf. Luppescu, 1991). Items that fell outside the 99% confidence band were excluded. In addition, we computed the Pearson correlation coefficient between the difficulty estimates related to the two grades. After excluding all misfit items based on these four criteria, we recalibrated the remaining items. This procedure was repeated until all the remaining items fulfilled our evaluation criteria.
In total, we excluded 48 of the 520 items from the final calibration (i.e., 9.2% of the original item pool), with 18 of these excluded because of low variation in the response data (i.e., number of correct or incorrect responses ≤ 3). These items were either too easy or too difficult for the target population and, thus, could not be estimated accurately. Furthermore, 10 items were excluded because they discriminated very badly between low-ability and high-ability students (i.e., rit ≤ 0.10), nine indicated large item misfit (i.e., WRMSD ≥ 0.20 or MaxRMSD ≥ 0.20) in the first calibration run, and two indicated large item misfit after recalibration in the second and third calibration runs. The item parameters for adjacent grade groups were generally very highly correlated, indicating parameter invariance across grades. The lowest correlation was found between the fourth and fifth grades (r = 0.816), and the highest correlation was found between the eighth and ninth grades (r = 0.962). Nine linking items were excluded due to a large difference between the two grade-specific difficulty parameters.
Four calibration runs were required before all remaining items showed satisfying values in the four evaluation criteria. Table 5 provides an overview of selected descriptive statistics from the final item pool. As shown in Table 5's third column, item exclusion was rather evenly distributed over the different curriculum cycles and domains, whereas the percentage of excluded items varied between 7% for the domain MA.1 (i.e., “number and variable”) and 11% for the domain MA.2 (i.e., “form and space”). As for competence levels, we excluded between 0 and 25% of the original items. The largest number of items (i.e., eight) had to be excluded for competency MA.3.C.2 (i.e., “mathematization of situations and verification of results”). As indicated in Table 5, the items related to competency MA.3.C.2 also had the highest mean difficulty (Mβ = −0.022), the largest variation in difficulty (SDβ = 3.564), and the largest mean standard error of item difficulty [MSE(β) = 0.320] of all 18 competencies. Furthermore, the excluded items were related to all seven target grades, so they varied substantially in their CRD (MCRD = 5.083, SDCRD = 2.396, MinCRD = 1, MaxCRD = 11). The remaining 472 items covered 117 of the 119 competence levels across all 18 competencies. Only two competence levels, which were represented by three items in total in the original item pool, were no longer covered by the final item pool (i.e., MA.2.A.3.f and MA.3.C.1.c). We argue that the exclusion of these two competence levels is acceptable given the large number of competencies covered in relation to the limited size of the item pool.
In sum, a satisfying number of items (i.e., 472) fit well into the established vertical Rasch scale, and the scale covered all relevant domains, competencies, and difficulty levels within the mathematics curriculum's two target cycles.
Given that the two calibration procedures' outcomes were very similar, we used only the parameters from the concurrent calibration in our analyses for validating the vertical scale from a content perspective. First, we investigated the relationship between the difficulty estimates from the final scale and the CRD by means of Pearson product-moment correlation coefficients to explore the scale's validity from a content perspective. Because of our study's relatively small sample sizes, we used a simulation-based approach to factor in the estimated difficulty parameters' error when calculating the correlations. Namely, we followed five steps:
1. We specified a normal distribution Ni(, SD[]) for each item i, in which refers to the difficulty parameter of item i from the calibration, and SD() refers to the standard deviation calculated based on the standard error of .
2. We randomly drew k = 10,000 samples Sik of size ni from Ni, in which ni was equal to the number of observed responses for item i.
3. For each Sik, we calculated the estimate as the mean of Sik, and we stored these estimates in a matrix with i × k elements.
4. For each of the estimates' k samples, we computed the correlation rk between the estimated difficulty parameters and the CRD, as well as the related p value pk.
5. Finally, we calculated the estimates r* and p* as the mean and related standard errors SE(r*) and SE(p*) as the standard deviation of rk and pk over the k samples; r* and p* were computed not only over the total item pool, but also separately for each curriculum cycle, domain, and competency.
With this approach, we were able to reproduce and SE(). For each item, (i.e., the mean over ) corresponded to , and SE() (i.e., the standard deviation of ) corresponded to SE(), resulting in correlations of r = 1.00. To compare the resulting correlation coefficients between cycles1, domains, and competencies, we performed omnibus tests (Paul, 1989), as well as subsequent range tests (Levy, 1976), by means of the computer program INCOR (Silver et al., 2008).
Second, the variation in item difficulty within each CRD category was investigated by means of boxplots and scatterplots. A boxplot was used to illustrate the general variation in item difficulty, and several scatterplots were used to visualize the relationship between item difficulty and CRD at different curriculum levels (i.e., per cycle, per domain, and per competency).
Finally, we performed three multiple linear regression analyses to investigate possible interaction effects between CRD and (1) curriculum cycles1, (2) domains, and (3) competencies on the prediction of the empirical item difficulty parameters. Similarly, for estimating correlation coefficients, we also used a simulation-based approach to factor in the estimated difficulty parameter errors for the regression analyses. Steps 1 to 3 were identical to the procedure described above. In step 4, we ran the multiple linear regressions for each of the 10,000 samples of and computed the regression parameters (i.e., adjusted , Fk and the related p value pFk, Bk and the related p value pBk). In step 5, we then calculated the adjusted R2*, F*, , B*, and as the mean and related standard errors as the regression parameters' standard deviation over the k samples.
To validate the vertical math scale from a content perspective and to address our first research question, we investigated the correlation between the empirical item difficulty estimates from the concurrent calibration and the CRD, as defined based on the matrix in Table 1, at different curriculum levels. Table 6 summarizes the number of items N per analysis, the estimates for the Pearson correlation coefficients r*, and the related standard errors SE(r*) based on the simulation, as well as the estimated p values p* for the correlation coefficients and their standard errors SE(p*).
Table 6. Estimated pearson correlation coefficients between CRD and difficulty estimates from the concurrent calibration over all items, per cycle, per domain, and per competency.
In line with our hypothesis, we found a significantly strong positive correlation of = 0.672 with p* < 0.001 between the CRD and the difficulty parameters from the concurrent calibration over the whole item pool [SE(r*) = 0.004; SE(p*) = 0.000]. Overall, items related to more advanced competence levels of the curriculum had higher difficulty estimates than items related to more basic competence levels. This finding is also supported by Figure 3, which illustrates the difficulty parameters' distribution within each CRD category over all competencies through boxplots. At the top of Figure 3, the different colors represent the three curriculum cycles. The boxplots show a considerable overlap of difficulties between the different CRD categories. Nevertheless, the boxplots for low CRD categories are generally located lower on the difficulty scale than those for higher ones, reflecting the positive correlation between the empirical and theoretical difficulties reported in Table 6.
Figure 3. Boxplots illustrating the distribution of difficulty estimates from the concurrent calibration for each CRD category. C1, C2, and C3 refer to cycles 1, 2, and 3, respectively.
Besides general correlation, we were also interested in the correlation between item difficulty and CRD within the curriculum's different cycles, domains, and competencies (see the second research question). Contrary to our hypothesis, Figure 3 indicates that the correlation between the difficulty estimates and CRD was significantly stronger for cycles 1 and 2 (i.e., primary school) than for cycle 3 (i.e., secondary school). The estimated correlation coefficients per curriculum cycle reported in the top part of Table 6 confirmed this assumption: The estimated correlation coefficients were r*(304) = 0.622 (p* < 0.001) and r*(168) = 0.220 (p* < 0.01) for primary school and for secondary school, respectively. The standard errors of r* and p*, estimated based on the simulations, were small and, thus, did not affect the interpretation of the results (see Table 6). Further analysis indicated that the correlation between the difficulty estimates and CRD within primary school was significantly stronger than that within secondary school [ = 26.157, p > 0.001; cf. (Paul, 1989)]. In line with this finding, the regression lines of the difficulty estimates and each cycle's CRD indicate an interaction effect from CRD and the curriculum cycle on the estimated item difficulty parameters (see the left graph of Figure 4). The multiple regression analysis results presented in Table 7 also strengthened this finding. We found a significant regression equation [adjusted R2* = 0.461, F*(3, 468) = 135.064, p* < 0.001], which included a significant negative interaction effect from CRD and the curriculum cycle on item difficulty (B* = −0.357, p* < 0.01). In addition, we found significant positive main effects from CRD (B* = 0.697, p* < 0.001) and the curriculum cycle2 ( = 2.204, p* < 0.05). Taken together, we concluded from these results that the vertical scale better represented the competencies stated in the curriculum for cycles 1 and 2 (i.e., primary school) than for cycle 3 (i.e., secondary school).
Figure 4. Relationship between difficulty estimates and CRD and related regression lines per cycle (left) and per domain (right).
Table 7. Results from multiple linear regression analyses for predicting empirical item difficulty by CRD and by the curriculum levels cycle, domain, and competencies.
The middle part of Table 6 includes the correlation between CRD and item difficulty for each of the three curriculum domains. All three correlations were statistically significant (p* < 0.001), and the related estimated standard errors, SE(r*) and SE(p*), were negligibly small (see Table 6). The correlation was slightly weaker for the domain MA.2 (i.e., “form and space”) with r*(126) = 0.603 than for the other two domains, MA.1 (i.e., “number and variable”) and MA.3 (i.e., “measures, functions, data, and probability”), with r*(188) = 0.706 and r*(158) = 0.707, respectively. However, in line with our hypothesis, the differences between the correlation coefficients were not statistically significant ( = 3.040, p = 0.219). Similarly, the graph on the right in Figure 4 shows that the three domains' regression lines are rather parallel and, thus, do not indicate any interaction effect from CRD and the domain on item difficulty. Moreover, a significant regression equation was found for the multiple regression analysis of CRD and the domain on item difficulty [adjusted R2* = 0.462, F*(5, 466) = 82.059, p* < 0.001], which indicated that neither the interaction effects of CRD and the domains3 ( = −0.079, p* = 0.283; = 0.043, p* = 0.521) nor the domains' main effects were statistically significant ( = 0.823, p* = 0.061; = 0.367, p* = 0.362; see Table 7). CRD was the only significant predictor of item difficulty in this model (B* = 0.581, p* < 0.01). Thus, we concluded from these findings that the vertical scale represented all three domains of the curriculum equally well.
Finally, as a third aspect within our second research question, we investigated the correlation between CRD and item difficulty for each of the 18 competencies represented by the vertical scale. The correlation coefficients, which we estimated based on our simulations, are displayed at the bottom of Table 6. The correlations varied between r*(22) = 0.179 (p* = 0.430) for competency MA.2.C.3, referring to “geometric figures and objects in different positions,” and r*(24) = 0.894 (p* < 0.001) for competency MA.3.C.2, referring to “mathematization of situations and verification of results.” In total, 15 of the 18 correlation coefficients were statistically significant at the 5% level when considering the standard errors of the p values SE(p*), which we estimated based on our simulations [i.e., p* + 1.96 × SE(p*) < 0.05]. Besides competency MA.2.C.3, we found insignificant correlations for competencies MA.2.B.1, referring to “exploration of lengths, surfaces, and volumes” [r*(19) = 0.433, p* = 0.066], and MA.3.B.2, referring to “statistics, combinatorics, and probability” [r*(29) = 0.402, p* = 0.033, SE(p*) = 0.014]. The omnibus test for correlations' equality indicated that significant differences existed between the different competencies' correlation coefficients [ = 31.261, p < 0.05]. Subsequent range tests (Levy, 1976) showed that the correlation within competency MA.2.C.3 was significantly lower than all the 15 significant correlations (p < 0.05). The other two insignificant correlations of competencies MA.2.B.1 and MA.3.B.2 were significantly lower than all correlations corresponding to r > 0.701 and r > 0.670, respectively (p < 0.05; i.e., 10 and 11 competencies, respectively, of the 18 competencies). On the other hand, the correlation of competency MA.3.C.2 (i.e., the highest of all the correlations) was significantly higher than correlations corresponding to r < 0.746 (p < 0.05), which applied to 13 of the 18 competencies. Furthermore, the range tests showed that the correlation of competency MA.1.B.1 (i.e., “numbers and operations”), which showed an average correlation between item difficulty and CRD [r*(24) = 0.670, p* < 0.001], only significantly differed from the correlations of competencies MA.2.C.3 and MA.3.C.2 (i.e., the competencies with the lowest and highest correlations; p < 0.05).
Furthermore, we regressed CRD and the competencies on item difficulty. To facilitate the interpretation of the results from this multiple regression analysis, we specified competency MA.1.B.1 (i.e., the competency with an average correlation coefficient) as the base level for the competency dummy coding. This specification allowed us to detect competencies that deviated from the general pattern. As reported in Table 7, the regression equation was significant [adjusted R2* = 0.528, F*(35, 436) = 16.033, p* < 0.001], including significant negative main effects, as well as significant positive interaction effects with CRD on item difficulty for competencies MA.2.A.3, referring to “computation of lengths, surfaces, and volumes” [ = −2.508, p* < 0.05, SE(p*) = 0.007; = 0.412, p* < 0.05, SE(p*) = 0.005] and MA.3.C.2, referring to “mathematization of situations and verification of results” [i.e., the competency with the strongest correlation between item difficulty and CRD; = −2.517, p* < 0.05, SE(p*) = 0.005; = 0.743, p* < 0.001]. In addition, we found a significant main effect from CRD (B* = 0.510, p* < 0.001). All other competencies' main and interaction effects were not significant.
Figure 5 visualizes the relationship between item difficulty and CRD within the different competencies of the curriculum. As a reference, each of the 18 scatterplots includes the regression line of the “average” competency MA.1.B.1 (gray line) beside the competency-specific regression line (blue line). The three competencies' scatterplots with insignificant correlations (i.e., MA.2.B.1, MA.2.C.3, and MA.3.B.2) are highlighted with purely white backgrounds. All three plots showed considerable overlap in item difficulty estimates between the different CRD categories, and the related regression lines had lower slopes than the regression lines of the other 15 competencies. The plots related to competencies MA.2.B.1 and MA.2.C.3 indicate, in addition, that these two competencies were poorly represented within cycle 3. In the curriculum (D-EDK, 2016a), these two competencies' level descriptions—and especially those related to cycle 3—are very abstract and complex (e.g., “students can formulate hypotheses while exploring geometrical relationships”), and partly refer to additional tools, such as dedicated computer software (e.g., “students can use dynamic geometry software to explore geometrical relationships”). Therefore, content experts only could develop a very limited number of items representing these levels. Furthermore, the curriculum only states five very broad competence levels for MA.2.C.3 and only seven competence levels, including one very broad one within cycle 2, for competency MA.3.B.2 (see Table 1). The equally low number of CRD categories might not map the variation in item difficulty sufficiently and, thus, probably does not reflect the development of students' competence levels within these categories either.
Figure 5. Relationship between difficulty estimates and CRD and related regression lines by competency. Bold blue lines = regression lines, gray lines = regression line of competency MA.1.B.1, white background = competencies with insignificant correlations, and gray background = competencies with significant interaction effects.
The scatterplots of the two competencies with significant main and interaction effects according to the regression analysis (i.e., MA.2.A.3 and MA.3.C.2) are highlighted in gray in Figure 5. The regression lines of these two competencies differed from the general pattern in their steep slopes. On one hand, these two competencies showed the highest variation in item difficulty among all the competencies, as indicated by the standard deviations of β in Table 5. On the other hand, compared with most of the other competencies, higher CRD and, thus, more advanced competence levels were related to clearly higher empirical item difficulties than lower CRD within these two competencies. This was especially true for competency MA.3.C.2, in which all items related to the highest competence level in the item pool (i.e., level f) had higher item difficulty parameters than the remaining items. At the same time, the items related to level MA.3.C.2.f were among the most difficult items of the whole item pool, which, in turn, explains the high mean and large standard deviation of item difficulty within this competency compared with the other competencies (see Table 5).
The slopes of the regression lines of the remaining 13 competencies were comparable with the slope of the regression line of the reference competency MA.1.B.1. In conclusion, our findings indicate that 15 of the 18 mathematics competencies were well-reflected by our vertical scale, whereas we found a particularly strong connection between theoretical and empirical item difficulty for 2 of these 15 competencies.
To assess students' abilities over the course of compulsory schooling and to evaluate their progress over time, a vertical measurement scale is essential, which allows for comparing scores from different measurement occasions (Young, 2006; Harris, 2007; Briggs, 2013; Kolen and Brennan, 2014). At the same time, a vertical scale is an important feature for identifying the most informative items from an item bank to assess students over a broad ability range (Dadey and Briggs, 2012; Tomasik et al., 2018), independent of whether the items are selected by a CAT algorithm or manually filtered based on difficulty by teachers or students. However, the development of a vertical scale is psychometrically challenging. Several extant studies point to the complex interactions between the practical context in which the scale is used and the decisions that researchers need to make during the development of a vertical scale (e.g., the selection of the calibration procedure, data collection design, or linking items; Béguin et al., 2000; Harris, 2007; Tong and Kolen, 2007; Briggs and Weeks, 2009; Dadey and Briggs, 2012; Keller and Hambleton, 2013). Given these complex interactions, no clear general recommendations exist for most scaling decisions (e.g., Harris, 2007; Tong and Kolen, 2007; Briggs and Weeks, 2009).
In this study, we described the development of a vertical scale for the formative assessment of students' mathematics abilities from the third through ninth grades based on IRT methods, and we evaluated this scale from a content-related perspective regarding the underlying content framework (i.e., the curriculum Lehrplan 21; (D-EDK, 2014, 2016a,b)). Hence, compared with most previous studies investigating the development of vertical scales, we not only looked at the scale's psychometric properties, but also considered its validity for measuring the target construct (i.e., students' ability in mathematics as described by the curriculum). For this purpose, we compared the empirical item difficulties as estimated on the vertical scale with content-related item difficulty estimates based on content experts' knowledge. Thanks to our theory-based external validation criterion (i.e., the items' content-related difficulty regarding the curriculum), we were able to validate the specific combination of scaling decisions that we took during the development of the vertical scale within our specific application and context.
Results from the item analysis indicated a satisfactory fit for more than 90% of the items from the initial item pool with the final unidimensional vertical Rasch scale. Given the Rasch model's strong assumptions, it is not surprising that we had to exclude some items during the calibration process for establishing the scale. Nevertheless, we did not find any systematic patterns of item exclusion across curriculum cycles or domains, and the final scale included items from all relevant competencies. Furthermore, we found strong correlations between item difficulty parameters from adjacent school grades, as well as between the item difficulty parameters from concurrent and grade-by-grade calibration procedures. These findings confirm the unidimensionality and, thus, the scale's stability across grades from a psychometric perspective (Hanson and Béguin, 2002; Kolen and Brennan, 2014).
From a content perspective, we found a strong positive correlation between the empirical item difficulty parameters on our vertical Rasch scale and the content-related item difficulties based on the mapping of the items to the curriculum's competence levels by the content experts who developed the items. As intended, items related to more advanced competence levels were generally represented by higher item difficulties on the vertical scale. However, we also found large variations in item difficulty within each content-related item difficulty category and, therefore, a strong overlap in item difficulty variation across the different content-related difficulty categories. At the same time, the large variation in item difficulty within the content-related item difficulty categories corresponds to the large variation in student ability within grades. In their longitudinal study investigating the development of mathematics and German abilities during compulsory school, Angelone et al. (2013, p. 35) found that the standard deviation of student ability in mathematics within one grade corresponded to more than twice the average learning progress per school year (see also Stevens et al., 2015). Item developers might have mirrored this wide variation in abilities within a grade by creating items of different difficulty for single competence levels. However, further studies are needed to get to the bottom of this assumption.
Further analyses demonstrated a stronger correlation between empirical and content-related item difficulty for primary school (i.e., the third through sixth grades, covered in the curriculum by the end of cycle 1 and the whole of cycle 2) than for secondary school (i.e., cycle 3), which contradicted our hypothesis and the vertical scale's objective. The significant difference in the strength of the two correlation coefficients implies that the vertical scale is more representative of the competencies described for primary school than those related to secondary school. However, we did not find any indication of multidimensionality in the empirical vertical scale, nor in our item analysis, nor in the comparison of the two calibration procedures, which were both in favor of a unidimensional stable vertical scale. One possible conclusion could be that the competence levels stated for cycle 3 differ less in difficulty than those stated for cycles 1 and 2. This assumption is in line with our finding of a steeper increase in mathematics ability throughout primary school, followed by a stagnation in ability progression throughout secondary school. Extant studies have reported similar learning trajectories (e.g., Bloom et al., 2008; Angelone et al., 2013; Stevens et al., 2015; Moser et al., 2017). In particular, competence levels from cycles 1 and 2 within one competency might reflect competence development, strictly speaking, whereas competence levels of cycle 3 might differ in terms of content and complexity, rather than in pure difficulty (Yen, 1985; Angelone et al., 2013; Moser et al., 2017). Apart from that, it might also be more difficult to describe the competencies' more complex development on secondary school levels by means of concrete competence descriptions and to differentiate clearly between distinct levels. Furthermore, it might be more challenging to create items targeted at higher competence levels and to predict their true difficulty (cf. Sydorenko, 2011). Further studies with a stronger focus on mathematics didactics and competence development are required to evaluate these hypotheses and investigate whether the competence levels described for secondary school fulfill the basic precondition for a unidimensional vertical scale of a continuous increase in the target competence over time (Young, 2006).
More detailed analyses related to the curriculum domains showed no significant differences in the correlation between empirical and content-related item difficulty across the three domains. The correlations within the domains were similar and corresponded to the general overall correlation. We concluded from these results that the vertical scale satisfactorily represented all three domains. Similar conclusions could also be drawn for most of the 18 competencies in our study, which revealed comparable correlations between empirical and content-related item difficulty. On the other hand, some findings provided interesting input for further research. Three selected, rather abstract and complex curriculum competencies were represented poorly on the general vertical mathematics scale. At the same time, 2 of the 18 competencies showed remarkably strong relationships between empirical and content-related item difficulty. For one thing, these results might be related to the various competencies' content-specific characteristics. However, they also could be related to our finding that the vertical scale was less representative in measuring mathematics ability in secondary- than primary-level students. Creating difficult items related to higher-level competencies might be especially difficult for selected competencies, whereas other competencies might provide better foundations. Unfortunately, we are unable to investigate these interactions further based on our data set, which certainly included a large item pool, but still contained only a limited number of items representing each competency and, in particular, each of the numerous competence levels. Therefore, further studies are needed to replicate our results with a larger item pool, including larger samples of items for each competence level, thereby allowing for drawing final conclusions about the causes of the differences between competencies.
Besides the limited size of the sample of items, other limitations in the present study should be noted. First, our study's focus lay in the validation of the empirical, vertical Rasch scale, whereas we did not question the validity of the competence levels stated in the curriculum or the representativeness of the items for their assigned competence levels. Curricula or content standards, which serve as a theoretical basis for test-content specifications, often lack empirical validation of the stated domains, competencies, and competence levels and, especially, of their development over time (Fleischer et al., 2013). Thus, studies with a design like ours could also contribute to the validation of theoretical assumptions about competence development and the quality of assessment items. For example, the comparison of empirical and content-related item difficulty could be used to detect competence descriptions that do not follow a continuous increase in difficulty over time, or test items that do not reach the expected empirical difficulty (i.e., items deviating from the general regression line between empirical and content-related difficulty) because they do not fit the underlying content specifications or suffer from technical problems. Even though it limits interpretation of the results to some extent that neither the theoretical framework nor the empirical vertical scale is completely bias-free, it also refers to a huge opportunity to connect the two dimensions for reciprocal validation and for gradually improving both dimensions.
A second limitation of our study is that we only used limited item formats (i.e., simple dichotomous items suitable for automated scoring). More advanced item formats, allowing for the assessment of cognitive processes or interactions, might contribute to better representing more complex competencies or competence levels. However, the question arises of whether such complex competencies are still representable by a unidimensional vertical scale or whether they would require more complex measurement models. Further studies are required to answer these questions.
Finally, it is also important to note that we developed a unidimensional vertical scale for assessing general mathematics ability. By excluding items with large drift between two age groups, we also might have excluded selected content areas that develop differently from the average competencies over the school years (Taherbhai and Seo, 2013). Therefore, in practice, it is important to complement formative assessments based on such a general scale with additional diagnostic assessments that allow for the detection of growth in very specific competencies, as well as differences in growth between competencies (e.g., Betebenner, 2009).
In sum, our study emphasizes the benefits of complementing psychometric and, thus, technical verification with content-related and, thus, theoretical validation when developing a vertical scale for measuring students' abilities across multiple school grades. Studies related to other subjects might result in different correlation patterns across different curriculum levels (i.e., cycles, domains, and competencies). However, the procedure that we suggested for validating the vertical scale can be transferred to all subjects for which the curriculum states a continuous increase in ability or content difficulty. Technical procedures, such as item analysis and the comparison of different calibration procedures, helped us identify items with misfit and underpin the scale's stability. However, only contrasting the empirical item difficulties with content-related ones provided information about the adequacy of our decisions during the scaling process for a particular practical context and the scale's validity in representing the underlying content framework (i.e., the curriculum). Therefore, our study points out the importance of close collaboration and discussion between psychometricians and content experts to develop valid and, thus, meaningful vertical scales.
This study's datasets will not be made publicly available because Northwestern Switzerland's (i.e., the contracting authorities) four cantonal authorities own them. Requests to access the datasets should be directed to Martin Brändli, TWFydGluLkJyYWVuZGxpQGRiay5zby5jaA==.
The four cantonal authorities of Northwestern Switzerland (Bildungsraum Nordwestschweiz) mandated that the Institute for Educational Evaluation and Cito develop vertical scales for educational assessment and collect related data. The data used for this study were collected by means of computer-based assessments, which were available for teachers besides other assessment templates in a first version of the computer-based assessment system MINDSTEPS (Tomasik et al., 2018). Teachers were invited to administer the booklets to their students during school lessons to support the calibration of the system's item pool. In line with the American Psychological Association's Ethical Principles and Code of Conduct, as well as with the Swiss Psychological Society's Ethical Guidelines, written informed consent from students and their parents was not required because this study was based on the assessment of normal educational practices and curricula in educational settings (i.e., solving a computer-based mathematics assessment at school; American Psychological Association, 2017, Paragraph 8.05; Swiss Psychological Society, 2003, Paragraph D19). As the contractors, the Institute for Educational Evaluation and Cito signed and committed to obeying the laws of the four cantons from Northwestern Switzerland to ensure strict data confidentiality. In line with the laws of the four cantons of Northwestern Switzerland and the contract between the cantons and the two institutes, approval from an Ethics Committee was not required for this study.
AV conceptualized the common item design. SB assembled the specific assessments, organized the data collection, analyzed, and interpreted the data supervised by TE and AV, and drafted the manuscript. UM, SB, and AV contributed to the development of the computer-based assessment system MINDSTEPS, which was used for data collection. All authors contributed to the manuscript revision, read and approved the submitted version, and contributed to the study's conception and design.
Northwestern Switzerland's (Bildungsraum Nordwestschweiz) cantonal authorities commissioned and funded the Institute for Educational Evaluation and Cito to develop and operate the computer-based formative feedback system, for which we developed and validated the vertical scale.
1. ^For the correlation and multiple regression analyses, we combined cycles 1 and 2 that represented primary school, whereas cycle 3 alone represented secondary school. Cycle 1 was only represented by one competence level per competency in our study.
2. ^The combination of cycles 1 and 2, representing primary school, served as a base level for the dummy coding.
3. ^MA.1 served as a base level for the dummy coding.
American Psychological Association (2017). Ethical Principles of Psychologists and Code of Conduct. Washington, DC: American Psychological Association. Retrieved from American Psychological Association website: https://www.apa.org/ethics/code
Angelone, D., Keller, F., and Moser, U. (2013). Entwicklung Schulischer Leistungen Während der Obligatorischen schulzeit: Bericht zur Vierten Zürcher Lernstandserhebung Zuhanden der Bildungsdirektion des Kantons Zürich [Development of School Performance During Compulsory School: Report on the fourth assessment for the attention of the Zurich department of education]. Zürich: Institut für Bildungsevaluation (IBE). Retrieved from Institut für Bildungsevaluation (IBE) website: http://www.ibe.uzh.ch/projekte/lezh/Lernstandserhebung_9KlasseZH_Bericht.pdf
Béguin, A. A., and Hanson, B. A. (2001). “Effect of noncompensatory multidimensionality on separate and concurrent estimation in IRT observed score equating,” in Paper presented at the Annual Meeting of the National Council on Measurement in Education (Seattle, WA).
Béguin, A. A., Hanson, B. A., and Glas, C. A. W. (2000). “Effect of multidimensionality on separate and concurrent estimation in IRT equating,” in Paper presented at the 2000 annual meeting of the National Council of Measurement in Education (New Orleans, IL).
Betebenner, D. (2009). Norm- and criterion-referenced student growth. Educ. Meas. Issues Pract. 28, 42–51. doi: 10.1111/j.1745-3992.2009.00161.x
BIFIE (Bundesinstitut für Bildungsforschung Innovation Entwicklung) (Ed.). (2011). Kompetenzorientierter Unterricht in Theorie und Praxis [Competence Orientation in Theory and Practice]. Graz: Leykam. Retrieved from: https://www.bifie.at/wp-content/uploads/2017/06/bist_vs_sek1_kompetenzorientierter_unterricht_2011-03-23.pdf
Bloom, H. S., Hill, C. J., Black, A. R., and Lipsey, M. W. (2008). Performance trajectories and performance gaps as achievement effect-size benchmarks for educational interventions. J. Res. Educ. Effect. 1, 289–328. doi: 10.1080/19345740802400072
Briggs, D. C. (2013). Measuring growth with vertical scales. J. Educ. Meas. 50, 204–226. doi: 10.1111/jedm.12011
Briggs, D. C., and Weeks, J. P. (2009). The impact of vertical scaling decisions on growth interpretations. Educ. Meas. Issues Pract. 28, 3–14. doi: 10.1111/j.1745-3992.2009.00158.x
Brown, G. T. L. (2013). “AsTTle – a national testing system for formative assessment: how the national testing policy ended up helping schools and teachers,” in Advances in Program Evaluation: Volume 14. A national developmental and negotiated approach to school self-evaluation, Vol. 14, eds S. Kushner, M. Lei, and M. Lai (Bradford: Emerald Group Publishing Limited), 39–56.
Cizek, G. J. (2005). Adapting testing technology to serve accountability aims: the case of vertically moderated standard setting. Appl. Meas. Educ. 18, 1–9. doi: 10.1207/s15324818ame1801_1
Dadey, N., and Briggs, D. C. (2012). A meta-analysis of growth trends from vertically scaled assessments. Pract. Assess. Res. Eval. 17, 1–13.
de Ayala, R. J. (Ed.). (2009). “The theory and practice of item response theory,” in Methodology in the Social Sciences (New York, NY: Guilford Press).
D-EDK (Deutschschweizer Erziehungsdirektoren-Konferenz) (2014). Lehrplan 21: Rahmeninformationen. Luzern. Retrieved from: http://www.lehrplan.ch/sites/default/files/lp21_rahmeninformation_%202014-11-06.pdf
D-EDK (Deutschschweizer Erziehungsdirektoren-Konferenz) (2016a). Lehrplan 21: Mathematik. Luzern. Retrieved from: https://v-fe.lehrplan.ch/container/V_FE_DE_Fachbereich_MA.pdf
D-EDK (Deutschschweizer Erziehungsdirektoren-Konferenz) (2016b). Lehrplan 21: Überblick. Luzern. Retrieved from https://v-fe.lehrplan.ch/container/V_FE_Ueberblick.pdf
DeMars, C. (2002). Incomplete data and item parameter estimates under JMLE and MML estimation. Appl. Meas. Educ. 15, 15–31. doi: 10.1207/S15324818AME1501_02
Eggen, T. J. H. M., and Verhelst, N. D. (2011). Item calibration in incomplete testing designs. Psicológica 32, 107–132.
Ferrara, S., Johnson, E., and Chen, W.-H. (2005). Vertically articulated performance standards: logic, procedures, and likely classification accuracy. Appl. Meas. Educ. 18, 35–59. doi: 10.1207/s15324818ame1801_3
Fleischer, J., Koeppen, K., Kenk, M., Klieme, E., and Leutner, D. (2013). Kompetenzmodellierung: struktur, konzepte und forschungszugänge des DFG-schwerpunktprogramms. Zeitschr. Erziehungswissenschaft 16, 5–22. doi: 10.1007/s11618-013-0379-z
Glas, C. A. W., and Geerlings, H. (2009). Psychometric aspects of pupil monitoring systems. Stud. Educ. Eval. 35, 83–88. doi: 10.1016/j.stueduc.2009.10.006
González, J., and Wiberg, M. (2017). Applying Test Equating Methods. Cham: Springer International Publishing.
Hanson, B. A., and Béguin, A. A. (1999). Separate Versus Concurrent Estimation of IRT Parameters in the Common Item Equating Design. ACT Research Report Series No. 99-8. Iowa City, IA: ACT.
Hanson, B. A., and Béguin, A. A. (2002). Obtaining a common scale for item response theory item parameters using separate versus concurrent estimation in the common-item equating design. Appl. Psychol. Meas. 26, 3–24. doi: 10.1177/0146621602026001001
Harris, D. J. (2007). “Practical issues in vertical scaling,” in Linking and Aligning Scores and Scales, eds N. J. Dorans, M. Pommerich, and P. W. Holland (New York: Springer), 233–251.
Hattie, J. A. C., and Brown, G. T. L. (2007). Technology for school-based assessment and assessment for learning: development principles from New Zealand. J. Educ. Technol. Syst. 36, 189–201. doi: 10.2190/ET.36.2.g
Hattie, J. A. C., and Timperley, H. (2007). The power of feedback. Rev. Educ. Res. 77, 81–112. doi: 10.3102/003465430298487
Ito, K., Sykes, R. C., and Yao, L. (2008). Concurrent and separate grade-groups linking procedures for vertical scaling. Appl. Meas. Educ. 21, 187–206. doi: 10.1080/08957340802161741
Keller, L. A., and Hambleton, R. K. (2013). The long-term sustainability of IRT scaling methods in mixed-format tests. J. Educ. Meas. 50, 390–407. doi: 10.1111/jedm.12025
Kiefer, T., Robitzsch, A., and Wu, M. L. (2016). TAM: Test Analysis Modules. Available online at: cran.R-project.org/package=TAM
Kim, S. H., and Cohen, A. S. (1998). A comparison of linking and concurrent calibration under item response theory. Appl. Psychol. Measure. 22, 131–143. doi: 10.1177/01466216980222003
Kolen, M. J., and Brennan, R. L. (2014). Test Equating, Scaling, and Linking: Methods and Practices, 3rd Edn. New York, NY: Springer.
Lei, P.-W., and Zhao, Y. (2012). Effects of vertical scaling methods on linear growth estimation. Appl. Psychol. Meas. 36, 21–39. doi: 10.1177/0146621611425171
Levy, K. J. (1976). A multiple range procedure for independent correlations. Educ. Psychol. Meas. 36, 27–31. doi: 10.1177/001316447603600103
Lord, F. M. (1980). Applications of Item Response Theory to Practical Testing Problems. New York, NY: Routledge.
McKinley, R. L. (1988). A comparison of six methods for combining multiple IRT item parameter estimates. J. Educ. Meas. 25, 233–246. doi: 10.1111/j.1745-3984.1988.tb00305.x
Moser, U., Oostlander, J., and Tomasik, M. J. (2017). “Soziale Ungleichheiten im leistungszuwachs und bei bildungsübergängen [Social disparities in performance gains and transitions probabilities],” in Bildungsverläufe von der Einschulung bis in den ersten Arbeitsmarkt. Theoretische Ansätze, Empirische Befunde und Beispiele, eds M. P. Neuschwander and C. Nägele (Wiesbaden: Springer VS), 59–77.
Oliveri, M. E., and von Davier, M. (2011). Investigation of model fit and score scale comparability in international assessments. Psychol. Test Assess. Model. 53, 315–333.
Paul, R. S. (1989). Test for the equality of several correlation coefficients. Can. J. Stat. 17, 217–227. doi: 10.2307/3314850
Pohl, S., Haberkorn, K., and Carstensen, C. H. (2015). “Measuring competencies across the lifespan: challenges of linking test scores,” in Springer Proceedings in Mathematics & Statistics: Volume 145. Dependent Data in Social Sciences Research. Forms, Issues, and Methods of Analysis. eds M. Stemmler, A. von Eye, and W. Wiedermann (Cham: Springer), 281–308.
Pomplun, M., Omar, M. H., and Custer, M. (2004). A comparison of Winsteps and Bilog-Mg for vertical scaling with the Rasch model. Educ. Psychol. Meas. 64, 600–616. doi: 10.1177/0013164403261761
R Core Team (2016). R: A Language and Environment for Statistical Computing. Vienna: R Foundation for Statistical Computing.
Rasch, G. (1960). Probabilistic Models for Some Intelligence and Attainment Tests. Copenhagen: Danmarks Paedagogiske Institut.
Reusser, K. (2014). Kompetenzorientierung als Leitbegriff der Didaktik [Competence orientation as a key concept of teaching]. Beiträge zur Lehrerinnen Lehrerbildung 32, 325–339.
Rupp, A. A., and Zumbo, B. D. (2016). Understanding parameter invariance in unidimensional IRT models. Educ. Psychol. Meas. 66, 63–84. doi: 10.1177/0013164404273942
Schildkamp, K., Lai, M. K., and Earl, L. (2013). Data-Based Decision Making in Education: Challenges and Opportunities. Dordrecht: Springer.
Silver, N. C., Zaikina, H., Hittner, J. B., and May, K. (2008). incor: a computer program for testing differences among independent correlations. Mol. Ecol. Resour. 8, 763–764. doi: 10.1111/j.1755-0998.2008.02107.x
Stevens, J. J., Schulte, A. C., Elliott, S. N., Nese, J. F. T., and Tindal, G. (2015). Growth and gaps in mathematics achievement of students with and without disabilities on a statewide achievement test. J. Sch. Psychol. 53, 45–62. doi: 10.1016/j.jsp.2014.11.001
Stocking, M. L., and Lord, F. M. (1983). Developing a common metric in item response theory. Appl. Psychol. Meas. 7, 201–210. doi: 10.1177/014662168300700208
Swiss Psychological Society (2003). Ethische Richtlinien für Psychologinnen und Psychologen der Schweizerischen Gesellschaft für Psychologie [Ethical Guidelines for Psychologists of the Swiss Psychological Society]. Bern: Swiss Psychological Society. Retrieved from Swiss Psychological Society website: https://www.swisspsychologicalsociety.ch/sites/default/files/ethische_richlinien18.dt_.pdf
Sydorenko, T. (2011). Item writer judgments of item difficulty versus actual item difficulty: a case study. Lang. Assess. Q. 8, 34–52. doi: 10.1080/15434303.2010.536924
Taherbhai, H., and Seo, D. (2013). The philosophical aspects of IRT equating: modeling erift to evaluate cohort growth in large-scale assessments. Educ. Meas. Issues Pract. 32, 2–14. doi: 10.1111/emip.12000
Tomasik, M. J., Berger, S., and Moser, U. (2018). On the development of a computer-based tool for formative student assessment: epistemological, methodological, and practical issues. Front. Psychol. 9:2245. doi: 10.3389/fpsyg.2018.02245
Tong, Y., and Kolen, M. J. (2007). Comparisons of methodologies and results in vertical scaling for educational achievement tests. Appl. Meas. Educ. 20, 227–253. doi: 10.1080/08957340701301207
Vale, C. D., and Gialluca, K. A. (1988). Evaluation of the efficiency of item calibration. Appl. Psychol. Meas. 12, 53–67. doi: 10.1177/014662168801200106
van der Kleij, F. M., Vermeulen, J. A., Schildkamp, K., and Eggen, T. J. H. M. (2015). Integrating data-based decision making, assessment for Learning and diagnostic testing in formative assessment. Assess. Educ. Princip. Policy Pract. 22, 324–343. doi: 10.1080/0969594X.2014.999024
van der Linden, W. J., and Glas, C. A. W. (eds.). (2010). Elements of Adaptive Testing. New York, NY: Springer.
von Davier, M., Weeks, J. P., Chen, H., Allen, J., and van der Velden, R. (2016). Creating Simple and Complex Derived Variables and Validation of Background Questionnaire Data. Technical report of the survey of adult skills (PIAAC) (Ch. 20). Paris: OECD.
Wainer, H. (Ed.). (2000). Computerized adaptive testing: A Primer, 2nd Edn. Mahwah, NJ: Lawrence Erlbaum Associates.
Warm, T. A. (1989). Weighted likelihood estimation of ability in item response theory. Psychometrika 54, 427–450. doi: 10.1007/BF02294627
Wauters, K., Desmet, P., and Noortgate, W., van den. (2010). Adaptive item-based learning environments based on the item response theory: possibilities and challenges. J. Comput. Assist. Learn. 26, 549–562. doi: 10.1111/j.1365-2729.2010.00368.x
Webb, N. L. (2006). “Identifying content for student achievement tests,” in Handbook of Test Development, eds S. M. Downing and T. M. Haladyna (Mahwah, NJ: Lawrence Erlbaum Associates), 155–180.
Wingersky, M. S., and Lord, F. M. (1983). An Investigation of Methods for Reducing Sampling Error in Certain IRT Procedures (ETS Research Reports Series No. RR-83-28-ONR). Princeton, NJ: Educational Testing Service.
Yamamoto, K., Khorramdel, L., and von Davier, M. (2016). Scaling PIAAC Cognitive Datam. Technical report of the survey of adult skills (PIAAC) (Ch. 17). Paris: OECD.
Yen, W. M. (1985). Increasing item complexity: A possible cause of scale shrinkage for unidimensional item response theory. Psychometrika 50, 399–410. doi: 10.1007/BF02296259
Keywords: vertical scaling, item calibration, item response theory, curriculum, validation
Citation: Berger S, Verschoor AJ, Eggen TJHM and Moser U (2019) Development and Validation of a Vertical Scale for Formative Assessment in Mathematics. Front. Educ. 4:103. doi: 10.3389/feduc.2019.00103
Received: 04 March 2019; Accepted: 10 September 2019;
Published: 04 October 2019.
Edited by:
Zi Yan, The Education University of Hong Kong, Hong KongReviewed by:
Giray Berberoglu, Başkent University, TurkeyCopyright © 2019 Berger, Verschoor, Eggen and Moser. This is an open-access article distributed under the terms of the Creative Commons Attribution License (CC BY). The use, distribution or reproduction in other forums is permitted, provided the original author(s) and the copyright owner(s) are credited and that the original publication in this journal is cited, in accordance with accepted academic practice. No use, distribution or reproduction is permitted which does not comply with these terms.
*Correspondence: Stéphanie Berger, U3RlcGhhbmllLkJlcmdlckBpYmUudXpoLmNo
Disclaimer: All claims expressed in this article are solely those of the authors and do not necessarily represent those of their affiliated organizations, or those of the publisher, the editors and the reviewers. Any product that may be evaluated in this article or claim that may be made by its manufacturer is not guaranteed or endorsed by the publisher.
Research integrity at Frontiers
Learn more about the work of our research integrity team to safeguard the quality of each article we publish.