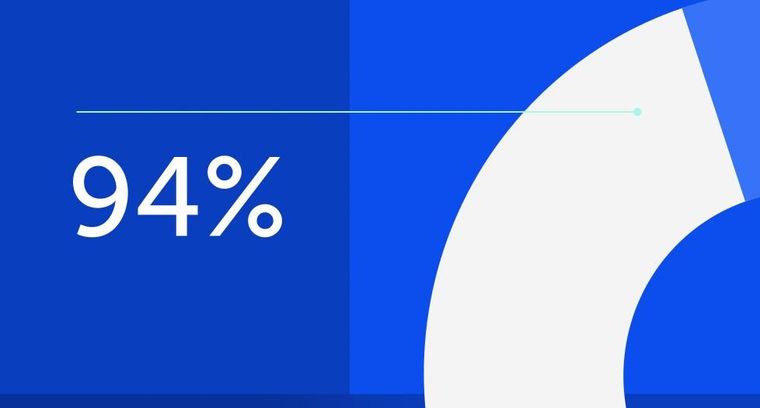
94% of researchers rate our articles as excellent or good
Learn more about the work of our research integrity team to safeguard the quality of each article we publish.
Find out more
ORIGINAL RESEARCH article
Front. Educ., 22 February 2019
Sec. Special Educational Needs
Volume 4 - 2019 | https://doi.org/10.3389/feduc.2019.00012
This article is part of the Research TopicHow Can Education Better Support the Mental Health & Wellbeing of Young People? Contributions from Developmental Psychopathology & Educational Effectiveness ResearchView all 13 articles
This study explored (1) whether growth mixture modeling (GMM) could identify different trajectories of learning efficiency during a working memory (WM) training programme for young children diagnosed with Attention Deficit Hyperactivity Disorder (ADHD), compared with a typically developing (TD) control group, and (2) if learning trajectories and outcomes were different for simple and complex training tasks. Children completed simple visuospatial short-term memory (VSSTM) and complex visuospatial WM (VSWM) tasks for 15 min a day, 5 days a week, and for 8 weeks. Parent-reported executive functioning, and children's WM and attention control, educational achievement, and IQ were measured prior to (T1), immediately following (T2) and 3 months after training (T3). GMM analysis showed that WM training was represented as one learning curve, and there was no difference for the trajectories of the ADHD and TD groups. The learning trajectory for the VSSTM tasks across groups was represented as one learning curve and for the VSWM tasks there were three learning curves. Learning for the VSSTM tasks and for most children in the VSWM tasks was characterized by an inverted-U shape, indicating that training was effective for up to 15 sessions, was stable and declined thereafter, highlighting an optimal training timeframe. For the VSWM tasks, the two remaining groups showed either a U-shaped or a high inverted U-shaped trajectory, with the latter group achieving the highest T1T2 change score (i.e., children showed a lower starting point and the most gain in terms of learning and post-training performance). There were no broader benefits of training at post-test or follow-up. Further research should explore who would benefit most from intensive cognitive training, as well as the potential benefits for mental health and well-being.
Developmental research has highlighted that attentional control skills, including working memory (WM), are positively linked to several indices of adjustment in childhood and adolescence, such as emotion regulation (reviews by Hadwin et al., 2016; Moran, 2016). In addition, research has shown that performance in WM tasks in children and adolescents is positively associated with educational achievement in core subjects, including text comprehension and reading (e.g., Daneman and Carpenter, 1980; Perfetti, 1985; Baddeley, 1986) and mathematical problem solving (e.g., Bull and Scerif, 2001; Swanson and Beebe-Frankenberger, 2004). These associations are argued to reflect cognitive potential that is separate from current knowledge (e.g., Gathercole et al., 2004; Phye and Pickering, 2006; Halford et al., 2007; Cowan, 2014).
Several studies have aimed to improve attentional control via WM training in clinical populations who experience difficulty with inattention. Training typically focuses on increasing short-term memory and WM skills using adaptive procedures that are delivered across multiple 30–40 min sessions each week (Klingberg et al., 2002, 2005). Task difficulty is typically adjusted automatically to performance across sessions to maximize learning at the boundaries of an individual's competence (Cortese et al., 2015). Several studies have found that improvements in WM associated with training is underpinned by neural change (Vinogradov et al., 2012) and that some degree of adaptability is a necessary precondition for its continuity (Poldrack and Gabrieli, 2001; Lewis et al., 2009).
Evidence further suggests that WM training represents one potential avenue for reducing symptoms of inattention and hyperactivity in Attention Deficit Hyperactivity Disorder (ADHD) (Klingberg et al., 2002, 2005). Building on evidence of brain plasticity from rehabilitation science and contemporary developmental neuroscience, WM training is premised on the notion that it impacts key brain networks implicated in ADHD (Vinogradov et al., 2012). Additionally, researchers have suggested that neuropsychological deficits mediate the pathways between originating causes and ADHD symptoms. It is argued, therefore, that improvement in neuropsychological functioning, including WM, may be a prerequisite for ADHD symptom reduction (Coghill et al., 2005).
Several recent reviews of research in WM training have questioned the viability of existing training programs to impact core symptoms and improve daily functioning in individuals diagnosed with ADHD (Chacko et al., 2014). These have highlighted the lack of scientific rigor in existing studies. In addition, they have raised concerns around goodness of fit that reflects the heterogeneous symptom profile in ADHD. For example, researchers have concluded that the results of WM training studies are inconsistent because of inadequate controls and ineffective measures to understand change in core cognitive functioning (e.g., Shipstead et al., 2010). Moreover, a systematic meta-analytic review of WM training studies with children and adult populations with ADHD used stringent criteria for inclusion to ensure that all (N = 23) studies were either randomized controlled trials or quasi-experiments (Melby-Lervåg and Hulme, 2013). The review found collective short-term improvements in practiced skills in clinical populations diagnosed with ADHD, however, there was no clear evidence for transfer to broader cognitive functioning or generalization (i.e., a reduction of ADHD symptoms in daily life).
A further meta-analysis considered the effect of cognitive training on ADHD symptoms, comparing reported outcomes across individuals who were blind or not blind to the intervention group (Sonuga-Barke et al., 2013). The analysis showed significant positive change in parent and teacher reported ADHD symptoms, however, this effect was lost when blinded assessments were analyzed. Moreover, a further meta-analysis showed that reported improvements dropped substantially and became statistically non-significant when only blinded measures were considered (Cortese et al., 2015).
Reviews of this evidence highlight that WM training for individuals diagnosed with ADHD leads to short-term improvements in WM functions that are directly taught. Increasingly, however, the proposed effectiveness of WM training to improve inattention and the quality of daily life more broadly for children and young people diagnosed with ADHD has been met with skepticism. Given that ADHD is a neuropsychological heterogeneous disorder (Nigg et al., 2005; Willcutt et al., 2005; Noreika et al., 2013), then the promise of the effectiveness of WM training may only apply to a subgroup of individuals who show specific processing impairments (Cortese et al., 2015).
The current study extended previous work to explore the possibility that training outcomes were moderated by individual characteristics. Specifically, we used growth mixture modeling (GMM) to investigate individual differences in training efficiency by analyzing trajectories of learning performance across an extended series of training sessions (Muthén and Muthén, 1998; Muthén and Shedden, 1999). GMM is a widely applied data analysis technique used to identify unobserved heterogeneity in a population and to describe and examine longitudinal change within these sub-populations (Nylund et al., 2007; Ram and Grimm, 2009). The results will have significant implications for understanding the development of key cognitive skills known to impact emotion regulation and learning and that can be delivered in an educational context for the benefit of children and young people.
We used an intensive computer WM training protocol that was developed in-house and delivered over 40 sessions and across 8 weeks to young typically developing (TD) children and those given a diagnosis of ADHD. In addition, we measured learning post-training and 3 months later to assess its impact on taught (near transfer) and novel (far transfer) tasks. We investigated three research questions to consider (1) whether trajectories of learning in performance during WM training (i.e., an index of training efficiency) were different between individuals diagnosed with ADHD vs. TD controls; (2) whether GMM would allow us to identify sub-groups with different trajectories of training efficiency for simple and complex training tasks and (3) if different trajectories were associated with near and far transfer in non-trained outcomes. Near transfer tasks included WM tasks completed by the children. Far transfer tasks included constructs that are known to be associated with performance in WM training (i.e., educational achievement and IQ) (Holmes et al., 2009; Jaeggi et al., 2010; Dahlin, 2011; Rudebeck et al., 2012). In addition, we also explored parent reported general behavior and cognitive ability in offspring.
The study was registered via the ISRCTN registry as a retrospective trial (https://doi.org/10.1186/ISRCTN15153056).
We recruited one-hundred-and-twenty-six children from pre-school and primary schools located in the Warsaw area of Poland. Sixty-one TD children were free of developmental disability and any other emotional or behavioral disorders (mean age = 6.70, SD = 0.39, range = 5.90–7.50). Sixty-five children (mean age = 6.70, SD = 0.39, range = 5.90–7.50) met diagnostic criteria for ADHD (American Psychiatric Association, 2000). In addition, five children in this group had a comorbid disorder including oppositional defiant disorder (N = 2) or autism spectrum disorder (N = 3). No one in the group was using psychotropic medication (e.g., methylphenidate) for ADHD disorder. The WM training group was made up of N = 126 children (N = 65 children diagnosed with ADHD and N = 61 typically developing children; see Figure 1).
Figure 1. shows the flow of participants through each stage of the study. Blue frames display participants through the intervention described in the article. *To deal with missing data we used Expectation Maximization (EM).
The computerized WM training was developed by the first author and was based on a published WM training program (Thorell et al., 2009). Because children younger than 7 years typically rely on visuospatial STM and WM (e.g., Hitch and Halliday, 1983; Hitch et al., 1988), training included only visuospatial training tasks. Five visuospatial short-term memory (VSSTM) tasks based on a similar theme were included. In two tasks, participants were shown the position of a target (presented for 1,000 ms) in a series of 4 × 4 matrices and they were asked to recall its location by using the mouse to tap the squares on the computer screen. With progression multiple targets appeared at the same time and were displayed for 2,000 ms. The third VSSTM task required the participant to tap the squares on the computer screen to identify the position of a previous target in a series of 2 × 5 matrices. In the last two VSSTM tasks the child was shown the position of a target in an irregular place and had to recall all positions by tapping the mouse in each position on the computer screen.
Three visuospatial WM (VSWM) tasks used moving stimuli that switched into targets to change color one by one and the target (presented for 1,000 ms) was held in a different color. When the last target was shown, the stimuli stopped moving, the child was asked to point to the targets in the order of appearance. In the first two tasks all stimuli moved in regular way around circle and then around an irregular figure. In the last task the movement was irregular.
Each task had six levels of difficulty, and each of these had three sub-levels. Task performance was recorded as the highest score obtained in each session and where each level corresponded to the number of items that the child had to remember. There were 2 items at level 1 and 7 items at level 6. Learning (training efficiency) was calculated across eight blocks each with five training sessions (the possible score range for each block was 1–18).
The training interface provided children with feedback for correct and incorrect performance (a smiley or sad face). The task was continuously adapted across training so that the difficulty level reflected current performance (i.e., three correct trials were required in order to advance to the next level, and for each incorrect trial, difficulty decreased by three sublevels). Children were asked to complete three tasks per session. The training was scheduled so that children worked on each task equally across 40 training sessions.
Our primary measure of memory improvement was derived from a tablet-based test of Working Memory Capabilities (Test pamiȩci roboczej- TPR, Educational Research Institute) (Kaczan et al., 2014), designed for children aged 6 and 7 years. It measures three aspects of STM/WM (Oberauer et al., 2003, 2008), including a counting span task, a set switching task and a task that measured memory for spatial locations.
With respect to the counting span task, children counted balls that were the same color as a target box (located at the bottom in the left corner of the screen on each trial) and ignored balls of a different color. The number of balls to be counted on each screen varied from one to five. On each trial children were asked to count the number of balls displayed and to remember and repeat them in the order of appearance. Children completed two practice trials followed by two sets of two, three, four and five screens (eight sets in total, presented in a quasi-random order, so that the difference in number of items to be remembered between adjacent screens was not bigger than two). Scores represent the proportion of correctly recalled balls.
In the set switching task, children were presented with a series of drawings of boys' or girls' emotional faces (happy or sad) presented in a square divided into four quarters (Rogers and Monsell, 1995). The task was to switch between decision making criterions that included a YES/NO response; “YES” to a BOY'S face (if the face appeared in the upper quarter of the screen and irrespective of emotion) or a HAPPY face (if the face appeared in the lower quarter and irrespective of gender), and “NO” otherwise. The task had 6 practice trials and five sets of 12 trials, where the criterion (between gender and emotion) switched after two trials and child response triggered the next trial. On each trial, accuracy was recorded and scores reflected the proportion of correct trials.
In the spatial location memory task children were asked to remember the position of 2–5 ladybirds that appeared sequentially on a matrix (6 × 6 squares) and across 12 trials (there were three trials at each level of difficulty). The test starts with a training trial with two objects (ladybirds). Performance reflects the mean accuracy of remembered sequences between the presented elements and where higher scores (expressed as a proportion of correct responses) reflected increased accuracy.
Attentional control was measured using a version of the Erikson Flanker task (Eriksen and Schultz, 1979). Participants were asked to identify a centrally presented target (happy face vs. unhappy) and to ignore flanker faces that were the same (congruent trials) or different (incongruent trials) to the central face. All children completed 6 practice trials (3 congruent and 3 incongruent) and 2 blocks of test trials. Each block included 32 randomly presented trials; 22 congruent (11 happy and 11 unhappy faces) and 10 incongruent trials. The main outcome measure in this task is a conflict score, calculated by subtracting the mean RT of the incongruent items from the mean RT of the congruent items on correct trials and where higher scores indicate increased distractor interference from flanker stimuli.
We used a Polish version of the Behavior Rating Inventory of Executive Function (BRIEF) (Gioia et al., 2000a). The parent report BRIEF estimates executive function abilities in children ranging from 5 to 18-years-old. It includes two domains (i) behavioral regulation (inhibition, shifting and emotional control) and (ii) metacognition (initiation, WM, planning, organization of materials and monitoring). The questionnaire has 86 items and 72 are used to compute the final score (Gioia et al., 2000a). Answers are given on a 3-point Likert scale (0-never to 2-often, making a total possible global score from 0 to 144 (and sub-scale scores for behavioral regulation and metacognition; maximum scores of 56 and 88 respectively). Parents are asked to rate behavior in relation to the context of their child's everyday home setting (Gioia et al., 2000b). This questionnaire was adapted from an existing measure that was independently translated into Polish by two researchers (both fluent in English). The final version was based on these two translations and a back translation and was evaluated by a group of experts (child psychiatrists and psychologists specializing in ADHD). Questionnaire items were accepted as final when the original and back-translated versions were identical or very similar.
We used the tablet based Test of Knowledge and Competences (Test UmiejȩtnościnaStarcieSzkolnym–TUNSS; Educational Research Institute) (Kaczan et al., 2014), designed to measure knowledge and skill in mathematics, reading and writing in 6- and 7-year-olds. (1) The numeracy skills scale includes numbers, measurement, space and shape, relationships and interconnections. (2) The writing skills scale measures visuomotor, visuospatial and audiolingual skills, penmanship and writing. (3) The reading skills scale measures audiolingual, audiovisual and reading skills. The test is completed individually and it includes an introductory phase (aimed at building a relationship with children and to familiarize them with the tablet and program) and a test phase. In the test phase the child is asked to complete a maximum of 10 tasks from each scale (reading, writing and numeracy skills) and the TUNSS score provides an estimate of a child's skill levels in each area. The score was computed as a variable theta (normal distribution, scale with M = 0 and SD = 1) for each scale (reading, writing and numeracy).
We used the Raven's CPM (Raven et al., 1986) to measure general intelligence. The test is designed for 4–10-year-olds and consists of 36 items in three sets that test a child's ability to complete continuing patterns, to perceive spatial relations and for abstract thinking. The items are presented in the form of incomplete patterns or matrices and children are asked to choose the missing element from a given set of possible answers. Each item was scored 1 for correct answer or 0 for incorrect answer. The maximum score is 36. Figure 2 presents a summary of all pre- and post-tests, follow up measures and WM training tasks.
This study was approved by the Institutional Research Ethics of Empirical Research with the Participation of People as Persons Researched. Parents and children were invited to take part in the study via a letter and each provided informed written consent. The children were asked to practice on the training task for 15 min a day on their personal computers at home, 5 days a week for 8 weeks. There were two inclusion criteria for both groups: participants were required to have an IQ score of ≥85, and we asked for an overview of the child's educational and social functioning, as reported by their teacher.
Participants were assessed individually in the University of Social Sciences and Humanities laboratory at three different time points; prior to training (pre-test/T1), post-test-no more than 1 week after training was completed (T2) and follow up-3 months after completing training (T3). All assessments were conducted by research staff in a laboratory, where children also completed the working memory, attention control measures, educational achievement, and IQ test. Additionally, parents of children completed the BRIEF scale.
We contrasted the training trajectories for the ADHD and the control (typical develop children–TD) groups by using latent growth curve modeling (LGCM) for time series data from the eight training blocks (one block made up 5 training sessions) (Duncan et al., 2011). First, an intercept-only model (a model with an assumption of no change in time) and then models with linear and quadratic slopes were estimated. The fit of individual models and comparisons between models were based on the chi-square value (χ2), the normed chi-square (χ2/df), the non-normed fit index (also known as Tucker–Lewis Index, TLI), the Comparative Fit Index (CFI), the Root Mean Square Error of Approximation (RMSEA), the Standardized Root Mean Square Residual (SRMR), and the Information Criteria Indexes: the Bayesian Information Criterion (BIC), and the Akaike Information Criterion (AIC).
A non-significant χ2 test indicates a good model fit, although its use is not free from limitations, especially for small sample sizes. Thus, the ratio of χ2 to degrees of freedom was also used, with values lower than 2 assumed to be satisfactory (Tabachnick and Fidell, 2007). The TLI and CFI threshold values of 0.90 indicate satisfactory fit, whereas values above 0.95 indicate good fit (Hooper et al., 2008). For a well-fitting model, the RMSEA should be close to 0: values below 0.05 indicate a good model fit and a value of 0.08 represents reasonable errors of approximation, whereas the SRMR should be below 0.10. The model with the lower information criterion values are preferable as they indicate better fit. The group comparison (ADHD vs. TD) was based on χ2 Wald test. The maximum likelihood (ML) as estimator was applied (Muthén and Muthén, 1998). Factor loadings corresponded directly to the blocks timing.
We then used growth mixture modeling (GMM) to identify sub-groups of individuals across groups with distinct training trajectories using data from the eight training tasks. GMM was performed with Mplus Version 7.3 (Muthén and Muthén, 1998). GMM allows the existence of latent homogeneous sub-populations of individuals within heterogeneous samples to be identified (i.e., the latent classes of individuals characterized by different learning curves). The maximum likelihood with robust standard errors (MLR) as estimator was applied (Muthén and Muthén, 1998). Factor loadings corresponded directly to the blocks timing. Both linear and quadratic slopes were estimated. Analyses were performed separately for (1) all training tasks combined, and for (2) VSSTM training tasks and (3) VSWM training tasks separately, and models for 1–4 classes were computed.
The models were compared using the Bayesian Information Criterion (BIC), the Akaike Information Criterion (AIC), the Bootstrap Likelihood Ratio Test (BLRT), the Vuong-Lo-Mendell-Rubin Likelihood Ratio Test (VLMRLRT), the entropy value, the subsample size, and practical usefulness of the latent training efficiency curve classes (Jung and Wickrama, 2008; Duncan et al., 2011). The model with the lower BIC and AIC values, greater entropy value (>0.80) indicated good fit (Jung and Wickrama, 2008; Duncan et al., 2011). The BLRT and VLMRLRT tests compare two models with different parameters. Significant p-values of tests indicated that the estimated model is preferable over a model with one fewer latent class (Nylund et al., 2007). The results were replicated to avoid local solutions (Jung and Wickrama, 2008).
Finally, we compared the subgroups identified using GMM in terms of pre-, post-intervention changes in our transfer of training measures. In order to compare training effects for sub-groups of children with different trajectories using one-way ANOVA analyses (SPSS Version 22) with GMM sub-group membership as baseline (pretest outcome measures) and change indicators including (1) pretest—posttest (T1T2) and (2) pretest—follow-up (T1T3) as the dependent (created by standardized regression residuals) (Duncan et al., 2011). Increasingly positive scores indicated positive change, scores that tend to zero reflect no change, and negative scores indicate negative change.
Missing training efficiency data varied between 2.2 and 28% per participant across training blocks. In order to maintain the full time series missing data was modeled using Full Information Maximum Likelihood (FILM) estimation with robust standard errors (Asparouhov and Muthen, 2010). This approach uses all observations in the dataset to produce the maximum likelihood estimation parameters without imputing data and is reported to be one of the best approaches currently available to handle missing time series data (Graham, 2009). Missing data in non-trained outcome measures (working memory, executive attention, educational achievement and IQ) varied from 1.1 to 28.9% across all measures. We used Expectation Maximization (EM) (Dong and Peng, 2013) to deal with missing pre- and post-intervention data, that were imputed with maximum likelihood values (Acock, 2005; Schlomer et al., 2010; Dong and Peng, 2013). Additionally Missing Value Analysis (Little's MCAR test) was consistent with a “missing at random” assumption for training tasks and non-trained outcome measures (Chen and Little, 1988).
Ninety participants (71% overall), 41 TD (67%) and 49 ADHD (75%), completed 15 or more training session within 8 weeks (our minimum compliance criteria). Fifteen children did not initiate training, 21 children withdrew before finishing 15 sessions. Overall, compliance to the intervention was good, in the context of a long and intensive home-based intervention parent-supported intervention delivered via the Internet (40 sessions over 8 weeks). Across 90 participants, 94% (N = 85) finished 20 sessions (1 block), 91% (N = 82) finished 25 (2 blocks), 88% (N = 79) finished 30 (3 blocks), 72% (N = 65) finished 35 (4 blocks), and 62% (N = 56) finished 40 sessions (5 blocks).
Compliance was defined as completing ≥15 of the 40 training sessions within a 8-week period. Using this algorithm, each child was categorized as compliant or noncompliant to treatment.
Table 1 shows the fit indicators for the unconditional growth models (stability, linear and quadratic change) based on all training (VSSTM and VSWM) tasks performed over 8 blocks of training. For all outcomes the quadratic slope model fitted better than the intercept-only and linear models, thus, the assumption of quadratic change over training in the whole sample is preferred, over the assumption of no change or linear change.
Table 1. Fit indicates for latent growth curve modeling (LGCM) models based on all memory training tasks (WMT) combined, and for visuospatial short-term training tasks (VSSTM) and visuospatial working memory training tasks (VSWM) over 8 learning sessions and across the whole sample (N = 90).
Means and variance of intercept and slopes in each task were significantly different from zero (see Table 2). When group was considered as a whole there was a significant increase and then decrease in latent tasks scores across time (i.e., the quadratic slope has a negative sign). There was also a significant slope factor variance. That means that children differed, not only in their initial latent task scores, but also in their training trajectories over time. However, the training trajectories of ADHD and TD groups did not differ significantly across all tasks (χ2 Wald test = 0.155, df = 1, p = 0.694), for the VSSTM (χ2 Wald test = 0.895, df = 1, p = 0.344), and the VSWM (χ2 Wald test = 3.706, df = 1, p = 0.054) tasks.
Table 3 presents the descriptive statistics for performance across training sessions for all tasks, and for the VSSTM and VSWM tasks separately and for the participant group as a whole. Models were generated for the total group of participants and across 8 blocks of training efficiency (see Table 4). With respect to all training tasks, the entropy value and the AIC value supported a two-class model, whereas the BIC value supported the one-class solution. VLMRLRT and the BLRT tests indicated that two- or more-class models were not superior to the one-class solution. Based on these indices, the one-class model was considered as the best fit model. With respect to the VSSTM, the AIC, the BIC values, as well as the VLMRLRT test, supported the one-class model. In contrast, the p-values of the BLRT test indicated that the two-class model was superior ion. Based on these indicators, but primarily the meaningfulness of the identified curves of training efficiency and class sizes, the one-class model was chosen. With respect to the heterogeneity of the learning curve of VSWM, the AIC and the BIC values supported the four- or one-class model respectively. The remaining indices supported the three-class solution. Based on the meaningful curves of training efficiency the three-class model was considered as the best fitting model.
Table 3. Performance for working memory tasks for the typically developing and children with ADHD over 8 blocks (each with 5 sessions) for all working memory training tasks (WMT) combined, and for visuospatial short-term training tasks (VSSTM) and visuospatial working memory training tasks (VSWM) separately (N = 90).
Table 4. Fit indices for GMM models based performance for WM tasks for the typically developing and clinical intervention groups over 8 blocks (with including 5 sessions) for all tasks combined (WMT) and for visuospatial short term memory (VSSTM) and visuospatial working memory tasks (VSWM) separately (N = 90).
According to GMM analyses, the whole sample (N = 90) across all WMT tasks represented an inverted U-shaped category (intercept = 9.22, SE = 0.17, slope = 0.87, SE = 0.09, quadratic = −1.13, SE = 0.01, p < 0.001), indicating gradual increase to Block 3 (15 sessions), followed by stability and then a decrease thereafter (see Figure 3A). Similar results were found for VSSTM tasks performance. Here training efficiency patterns followed a curvilinear trajectory, increasing to Block 3, stability and followed by a decrease (intercept = 9.71, SE = 0.18, slope = 0.86, SE = 0.09, quadratic = −0.11, SE = 0.01, p < 0.001), see Figure 3B. The analysis for the VSWM produced a three-class model. In this case, more than half of the sample (N = 69; 76.7%) was characterized by an inverted U-shaped (see class 2; intercept = 8.41, SE = 0.23, slope = 0.47, SE = 0.08, quadratic = −0.06, SE = 0.01, p < 0.001), with performance, increasing to block 3, stability and subsequently decrease. The second group (class 1, N = 14; 15.5%) was characterized by a U-shaped curve, with the highest starting scores then deterioration of performance up to block 5 and then slight increase (intercept = 10.05, SE = 0.83, slope = −0.94, SE = 0.25, quadratic = 0.13, SE = 0.03, p < 0.001). The smallest group (class 3; N = 7; 7.8%) was characterized by a high inverted U-shaped curve (intercept = 7.48, SE = 0.99, slope = 2.15, SE = 0.26, quadratic = −0.24, SE = 0.03, p < 0.001), highlighting the lowest starting scores and the highest improvement in performance across training (see Figure 3C).
Figure 3. latent learning curve over 8 blocks for all tasks in all working memory training tasks (WMT; A), visuospatial short-term training tasks (VSSTM; B) and visuospatial working memory training tasks (VSWM; C) separately (N = 90).
Table 5 presents the descriptive statistics for outcome measures for the three trajectory groups (from GMM in VSWM training tasks). Considering associations between learning and change between pretest-posttest (T1T2) outcome measures, the results showed a statistical trend toward significance for group for the spatial location memory task [F(2, 89) = 2.60, p = 0.080, η2 = 0.06]. Here, the class 3 achieved the highest change T1T2 score in spatial location memory task (M = 0.39, SD = 0.18). Furthermore, class 1 had a trend toward a significant greater improvement in T1T2 spatial location memory task (M = 0.32, SD = 0.13), compared with sub-group class 2. All other results were not significant.
Table 5. Performance for pre-test outcome measures for three trajectory groups (from GMM in VSWM training tasks).
The current study extended previous research to utilize GMM to explore learning/training efficiency during an extended WM training protocol for TD children and children given a diagnosis of ADHD. We examined performance curves using GMM across 40 training sessions (represented in 8 blocks) to explore performance between groups and across the sample. In addition, we investigated whether child characteristics were important in understanding individual differences in learning. Furthermore, we considered learning for simple and complex training tasks on training efficiencyand transfer to tasks that were conceptually similar to trained tasks (near transfer), as well as those not associated with training (far transfer).
Considering group differences in training efficiency, the results showed that young children diagnosed with ADHD did not differ from the TD group in their learning trajectories, and where this result was evident for all training tasks combined and when considering training on learning simple VSSTM, as well as more complex VSWM tasks. Specifically, the results showed that across groups learning during WM training (across all WM and for simple short term memory tasks) was characterized by an inverted-U highlighting initial learning (increased performance to block 3; around 15 training sessions), stability and then gradual decrease in performance. When learning more complex tasks (VSWM), three independent learning curves were identified; most children followed the same inverted-U trajectory described above, and two smaller groups were characterized by a U-shaped curve (a high starting point, followed by initial deterioration and then increase) or a sharp inverted-U, that showed the largest increase in learning over the first three blocks. Further analysis highlighted that this latter group showed the lowest pre-training WM scores and the highest T1T2 change.
The results provide a novel set of findings associated with training WM in young children. They highlight that across training tasks learning was most evident in the first 15 training sessions, it then remained stable and showed some deterioration thereafter. The results indicate that WM training is most effective in the short term, and that young children (at least in this sample) did not benefit from an extended approach to training. These results contradict previous findings from adult samples, where a greater number of training sessions was linked to more effective outcomes (Jaeggi et al., 2008; Schmiedek et al., 2010). This difference raises the possibility that the motivation for intense and sustained intellectual effort across weeks in young children is different compared with adults. It is possible that adults have increased metacognitve skills in learning and therefore may be more aware of the potential benefits of their efforts. Alternatively, children may have a limited ability to acquire new mnemonic strategies, and while existing techniques allow them to learn quickly at the beginning of training, this limitation may prevent them from developing further as the training progresses. This explanation is consistent with the development of short term memory, which highlights that it is not until middle childhood (around 7 years of age) that children will typically start to utilize mnemonic strategies to enhance performance in memory tasks (Cowan, 1997). Further studies into the potential moderators of outcome, for example, an exploration of the function of mnemonic techniques and metacognitive awareness in learning would allow for further delineation of children who might benefit most from training.
The results also highlighted training in the most complex tasks revealed a different pattern of learning efficiency. Specifically, they indicated that a small subgroup of children who performed less well at pre-test benefitted most from training and achieved the highest change score (T1T2) in complex spatial WM tasks (showing a high inverted U-shaped training curve). This finding highlights most benefit for this group of children on near transfer tasks, i.e., those that tap working memory function (vs. attentional control more broadly; see also Oberauer et al., 2008). The current study did not reveal benefits to executive functioning more broadly or to parent reports of daily behavior and educational achievement, see also Chacko et al. (2014) for similar results. In addition, one further group of children in the current study did not show any benefit of training. While the lack of transfer to distant, non-trained tasks in the current study might reflect the decline in learning over the course of the program, this result is consistent with recent reviews of WM training which suggest that WM training has most impact on tasks that are most similar to those that are trained (Shipstead et al., 2010). In the current paper, the results suggest that children who show difficulties with WM benefitted most from training. Future research should be focused more carefully on identifying further sub-groups that might benefit from WM training.
The current study revealed that most children were able to show improvements in WM tasks during training and where this increase was most effective for 15 sessions of training (with stability and decrease thereafter). In addition, it highlighted that children who experienced the lowest scores on WM tasks showed most learning and broader benefits post-training in similar non-trained WM tasks. There was no evidence of broader benefits in attention tasks or on parent-reported daily functioning and educational achievement. Future research should focus on possible enhancement of the capacity working memory through training and the possibility of far transfer (e.g., academic skills). Further studies into the potential moderators of outcome, for example, an exploration of the function of mnemonic techniques and metacognitive awareness in learning would allow for further delineation of children who might benefit from training. The results indicate that those young children who show difficulties with tasks that require attentional control in an educational context might benefit from WM training, and further research should focus on understanding whether there are longer term benefits of enhancing working memory skills in the short term. In addition, the research field would benefit from the use of standardized and reliable and valid tests to assess executive functions and evaluate the effectiveness of training tasks. This could facilitate a more accurate analysis of cognitive training effectiveness and the stability of its effects over time.
The present study had several limitations. In order to understand potential change associated with time or the active nature of this intervention, the study would have benefitted from a placebo intervention group, as well as a passive control group. In addition, WM training was conducted via the Internet and the children participated under parental supervision, consequently, we were not able to monitor treatment integrity across the intervention. Moreover, a diagnostic approach that generated a continuous measure of ADHD would have enabled a comprehensive profile of symptoms across the entire sample.
Please see registration: ISRCTN15153056 https://doi.org/10.1186/ISRCTN15153056 working memory training effects on young children's functioning.
This study was carried out in accordance with the recommendations of Study Protocol Approved by Ethics Committee, SWPS University of Social Sciences and Humanities, Warsaw, Poland “Training, motivation and maturation influences on the development of executive functions at early childhood,” [Komisja ds. Etyki Badań Empirycznych z Udziałem Ludzi jako Osób Badanych, Warszawa, Polska “Wpływ treningu motywacji, dojrzewania na rozwój funkcji wykonawczych u dzieci we wczesnym wieku rozwojowym”] with written informed consent from all subjects. All subjects gave written informed consent in accordance with the Declaration of Helsinki. The protocol was approved by the Ethics Committee, SWPS University of Social Sciences and Humanities, Warsaw, Poland; number 2/V/11-12 [Komisja ds. Etyki Badań Empirycznych z Udziałem Ludzi jako Osób Badanych, Warszawa, Polska; nr 2/V/11-12].
AO, JH, AK, and ES-B: contributed to the conception and design of the study; AO: organized the database, the project administration and was the research leader; AO and AK: performed the statistical analysis; AO, AK, and JH: wrote the first draft of the manuscript; AO, JH, AK, and ES-B: wrote sections of the manuscript. All authors contributed to manuscript revision, read and approved the submitted version.
This research and preparation of the paper were supported by grants from the National Science Centre, Poland (UMO-2011/03/N/HS6/04849 and UMO-2014/12/T/HS6/00216).
The authors declare that the research was conducted in the absence of any commercial or financial relationships that could be construed as a potential conflict of interest.
Acock, A. C. (2005). Working with missing values. J. Marriage Family 67, 1012–1028. doi: 10.1111/j.1741-3737.2005.00191.x
American Psychiatric Association (2000). Diagnostic and Statistical Manual of Mental Disorders (DSM-IV-TR). Washington DC, American Psychiatric Association.
Asparouhov, T., and Muthen, B. (2010). Multiple Imputation with Mplus. Mplus technical report. Available online at: https://www.statmodel.com
Bull, R., and Scerif, G. (2001). Executive functioning as a predictor of children's mathematics ability: Shifting, inhibition, and working memory. Develop. Neuropsychol. 19, 273–293. doi: 10.1207/S15326942DN1903_3
Chacko, A., Bedard, A. C., Marks, D. J., Feirsen, N., Uderman, J. Z., Chimiklis, A., et al. (2014). A randomized clinical trial of cogmed working memory training in schoolage children with ADHD: a replication in a diverse sample using a control condition. J. Child Psychol. Psychiatry 55, 247–255. doi: 10.1111/jcpp.12146
Chen, H. Y., and Little, R. (1988). A test of missing completely at random for generalised estimating equations with missing data. J. Am. Stat. Assoc. 86, 1198–1202.
Coghill, D., Nigg, J., Rothenberger, A., Sonuga-Barke, E., and Tannock, R. (2005). Whither causal models in the neuroscience of ADHD? Develop. Sci. 8, 105–114. doi: 10.1111/j.1467-7687.2005.00397.x
Cortese, S., Ferrin, M., Brandeis, D., Buitelaar, J., Daley, D., Dittmann, R. W., et al. (2015). Cognitive training for attention-deficit/hyperactivity disorder: metaanalysis of clinical and neuropsychological outcomes from randomized controlled trials. J. Am. Acad. Child Adolescent Psychiatry 54, 164–174. doi: 10.1016/j.jaac.2014.12.010
Cowan, N. (1997). “The development of working memory,” in The Development of Memory in Childhood, eds N. Cowan and C. Hulme (New York, NY: Psychology Press Ltd), 163–200.
Cowan, N. (2014). Working memory underpins cognitive development, learning, and education. Educ. Psychol. Rev. 26, 197–223. doi: 10.1007/s10648-013-9246-y
Dahlin, K. I. E. (2011). Effects of working memory training on reading in children with special needs. Read. Writing 24, 479–491. doi: 10.1007/s11145-010-9238-y
Daneman, M., and Carpenter, P. A. (1980). Individual differences in working memory and reading. J. Verbal Learn. Verbal Behav. 19, 450–466. doi: 10.1016/S0022-5371(80)90312-6
Dong, Y., and Peng, C. J. (2013). Principled missing data methods for researchers. SpringerPlus 222, 1–17. doi: 10.1186/2193-1801-2-222
Duncan, T. E., Duncan, S. C., and Strycker, L. A. (2011). An Introduction to Latent Variable Growth Curve Modeling: Concepts, Issues, and Application, 2nd edn. Mahwah, NJ: Lawrence Erlbaum Associates.
Eriksen, C. W., and Schultz, D. W. (1979). Information processing in visual search: a continuous flow conception and experimental results. Percept. Psychophys. 25, 249–263. doi: 10.3758/BF03198804
Gathercole, S. E., Pickering, S. J., Ambridge, B., and Wearing, H. (2004). The structure of working memory from 4 to 15 Years of Age. Develop. Psychol. 40, 177–190. doi: 10.1037/0012-1649.40.2.177
Gioia, G. A., Isquith, P. K., Guy, S. C., and Kenworthy, L. (2000a). Behavior Rating Inventory of Executive Function. Professional Manual, 3th edn. Lutz: PAR.
Gioia, G. A., Isquith, P. K., Guy, S. C., and Kenworthy, L. (2000b). Behavior rating inventory of executive function. Child Neuropsychol. A J. Normal Abnormal Develop. Childhood Adolescence 6, 235–238. doi: 10.1076/chin.6.3.235.3152
Graham, J. W. (2009). Missing data analysis: making it work in the real world. Ann. Rev. Psychol. 60, 549–576. doi: 10.1146/annurev.psych.58.110405.085530
Hadwin, J. A., Visu-Petra, L., Muris, P., Derakshan, N., and Macleod, C. (2016). Introduction to the special issue: understanding the role of attentional control in the development of anxiety in childhood, adolescence, and across lifespan. J. Exp. Psychopathol. 7, 277–295. doi: 10.1177/204380871600700301
Halford, G. S., Cowan, N., and Andrews, G. (2007). Separating cognitive capacity from knowledge: a new hypothesis. Trends Cogn. Sci. 11, 236–242. doi: 10.1016/j.tics.2007.04.001
Hitch, G. J., and Halliday, M. S. (1983). Working memory in children. Philos. Trans. R. Soc. London Series B 302, 324–340. doi: 10.1098/rstb.1983.0058
Hitch, G. J., Halliday, S., Schaafstal, A. M., and Schraagen, J. M. C. (1988). Visual working memory in young children. Memory Cogn. 16, 120–132. doi: 10.3758/BF03213479
Holmes, J., Gathercole, S. E., and Dunning, D. L. (2009). Adaptive training leads to sustained enhancement of poor working memory in children. Develop. Sci. 12, 9–15. doi: 10.1111/j.1467-7687.2009.00848.x
Hooper, D., Coughlan, J., and Mullen, M. (2008). Structural Equation Modelling: Guidelines for Determining Model Fit. Dublin Institute of Technology. 53–60. Available online at: http://arrow.dit.ie/libart/4
Jaeggi, S. M., Buschkuehl, M., Jonides, J., and Perrig, W. J. (2008). Improving fluid intelligence with training on working memory. Proc. Natl. Acad. Sci. U.S.A. 105, 6829–6833. doi: 10.1073/pnas.0801268105
Jaeggi, S. M., Studer-Luethi, B., Buschkuehl, M., Su, Y. F., Jonides, J., and Perrig, W. J. (2010). The relationship between n-back performance and matrix reasoning - implications for training and transfer. Intelligence 38, 625–635. doi: 10.1016/j.intell.2010.09.001
Jung, T., and Wickrama, K. A. S. (2008). An introduction to latent class growth analysis and growth mixture modeling. Social Personal. Psychol. Compass 2, 302–317. doi: 10.1111/j.1751-9004.2007.00054.x
Kaczan, R., Rycielski, P., Rzenca, K., and Sijko, K. (2014). Metody Diagnozy na Pierwszym Etapie Edukacyjnym Podrȩcznik Narzȩdzi Diagnostycznych. Warszawa: Instytutu Badań Edukacyjnych.
Klingberg, T., Fernell, E., Olesen, P., Johnson, M., Gustafsson, P., Dahlstrom, K., et al. (2005). Computerized training of working memory in children with ADHDFa randomized, controlled trial. J. Am. Acad. Child Adolescent Psychiatry 44, 177–186. doi: 10.1097/00004583-200502000-00010
Klingberg, T., Forssberg, H., and Westerberg, H. (2002). Training of working memory in children with ADHD. J. Clin. Exp. Neuropsychol. 24, 781–791. doi: 10.1076/jcen.24.6.781.8395
Lewis, C. M., Baldassarre, A., Committeri, G., Romani, G. L., and Corbetta, M. (2009). Learning sculpts the spontaneous activity of the resting human brain. Proc. Natl. Acad. Sci. U.S.A. 106, 17558–17563. doi: 10.1073/pnas.0902455106
Melby-Lervåg, M., and Hulme, C. (2013). Is working memory training effective? A meta-analytic review. Develop. Psychol. 49, 270–291. doi: 10.1037/a0028228
Moran, T. P. (2016). Anxiety and working memory capacity: a meta-analysis and narrative review. Psychol. Bull. 142, 831–864. doi: 10.1037/bul0000051
Muthén, B., and Shedden, K. (1999). Finite mixture modeling with mixture outcomes using the EM algorithm. Biometrics 55, 463–469. doi: 10.1111/j.0006-341X.1999.00463.x
Muthén, L. K., and Muthén, B. O. (1998). Mplus User's Guide. 7th Edn. Los Angeles, CA: Muthén & Muthén.
Nigg, J. T., Willcutt, E. G., Doyle, A. E., and Sonuga-Barke, E. J. S. (2005). Causal heterogeneity in attention-deficit hyperactivity disorder: do we need neuropsychologically impaired subtypes? Biol. Psychiatry 57, 1224– 1230. doi: 10.1016/j.biopsych.2004.08.025
Noreika, V., Falter, C. M., and Rubia, K. (2013). Timing deficits in attention-deficit/hyperactivity disorder (ADHD): evidence from neurocognitive and neuroimaging studies. Neuropsychologia 51, 235–266. doi: 10.1016/j.neuropsychologia.2012.09.036
Nylund, K. L., Asparouhov, T., and Muthen, B. O. (2007). Deciding on the number of classes in latent class analysis and growth mixture modeling: a monte carlo simulation study. Struc. Equ. Model. 14, 535–569. doi: 10.1080/10705510701575396
Oberauer, K., Süß, H. M., Wilhelm, O., and Wittman, W. W. (2003). The multiple faces of working memory: storage, processing, supervision, and coordination. Intelligence 31, 167–193. doi: 10.1016/S0160-2896(02)00115-0
Oberauer, K., Süß, H. M., Wilhelm, O., and Wittman, W. W. (2008). Which working memory functions predict intelligence? Intelligence 36, 641–652. doi: 10.1016/j.intell.2008.01.007
Phye, G. D., and Pickering, S. J. (2006). Working Memory and Education. New York, NY: Academic Press.
Poldrack, R. A., and Gabrieli, J. D. (2001). Characterizing the neural mechanisms of skill learning and repetition priming: evidence from mirror reading. Brain 124, 67–82. doi: 10.1093/brain/124.1.67
Ram, M., and Grimm, K. J. (2009). Growth mixture modeling: a method for identifying differences in longitudinal change among unobserved groups. Int. J. Behav. Dev. 33, 565–576. doi: 10.1177/0165025409343765
Raven, J. C., Court, J. H., and Raven, J. (1986). Manual for Raven's Progressive Matrices and Vocabulary Scales, Research Supplement No. 3. London: Lewis.
Rogers, R. D., and Monsell, S. (1995). Costs of a predictible switch between simple cognitive tasks. J. Exp. Psychol. General 124, 207–231. doi: 10.1037/0096-3445.124.2.207
Rudebeck, S. R., Bor, D., Ormond, A., O'Reilly, J. X., and Lee, A. C. (2012). A potential spatial working memory training task to improve both episodic memory and fluid intelligence. PLoS One 7:e50431. doi: 10.1371/journal.pone.0050431
Schlomer, G. L., Bauman, S., and Card, N. A. (2010). Best practices for missing data management in counseling psychology. J. Counsel. Psychol. 57, 1–10. doi: 10.1037/a0018082
Schmiedek, F., Lövdén, M., and Lindenberger, U. (2010). Hundred days of cognitive training enhance broad cognitive abilities in adulthood: findings from the COGITO study. Front. Aging Neurosci. 2:27. doi: 10.3389/fnagi.2010.00027
Shipstead, Z., Redick, T. S., and Engle, R. W. (2010). Does working memory training generalize? Psychol. Belgica 50, 245–276. doi: 10.5334/pb-50-3-4-245
Sonuga-Barke, E. J., Brandeis, D., Cortese, S., Daley, D., Ferrin, M., Holtmann, M., et al. (2013). Nonpharmacological interventions for ADHD: systematic review and meta-analyses of randomized controlled trials of dietary and psychological treatments. Am. J. Psychiatry 170, 275–289. doi: 10.1176/appi.ajp.2012.12070991
Swanson, H. L., and Beebe-Frankenberger, M. (2004). The relationship between working memory and mathematical problem solving in children at risk and not at risk for math disabilities. J. Educ. Psychol. 96, 471–491. doi: 10.1037/0022-0663.96.3.471
Tabachnick, B. G., and Fidell, L. S. (2007). Using Multivariate Statistics. 6th edn. Boston: Pearson.
Thorell, L. B., Lindqvist, S., Bergman, N., Bohlin, G., and Klingberg, T. (2009). Training and transfer effects of executive functions in preschool children. Develop. Sci. 12, 106–113. doi: 10.1111/j.1467-7687.2008.00745.x
Vinogradov, S., Fisher, M., and de Villers-Sidani, E. (2012). Cognitive training for impaired neural systems in neuropsychiatric illness. Neuropsychopharmacology 37, 43–76. doi: 10.1038/npp.2011.251
Keywords: WM training, learning trajectories, young children, attention-deficit/hyperactivity disorder (ADHD), growth mixture modeling (GMM)
Citation: Orylska A, Hadwin JA, Kroemeke A and Sonuga-Barke E (2019) A Growth Mixture Modeling Study of Learning Trajectories in an Extended Computerized Working Memory Training Programme Developed for Young Children Diagnosed With Attention-Deficit/Hyperactivity Disorder. Front. Educ. 4:12. doi: 10.3389/feduc.2019.00012
Received: 23 July 2018; Accepted: 04 February 2019;
Published: 22 February 2019.
Edited by:
Vasiliki Totsika, University College London, United KingdomReviewed by:
Mats Granlund, Jönköping University, SwedenCopyright © 2019 Orylska, Hadwin, Kroemeke and Sonuga-Barke. This is an open-access article distributed under the terms of the Creative Commons Attribution License (CC BY). The use, distribution or reproduction in other forums is permitted, provided the original author(s) and the copyright owner(s) are credited and that the original publication in this journal is cited, in accordance with accepted academic practice. No use, distribution or reproduction is permitted which does not comply with these terms.
*Correspondence: Anna Orylska, YW9yeWxza2FAc3dwcy5lZHUucGw=
Disclaimer: All claims expressed in this article are solely those of the authors and do not necessarily represent those of their affiliated organizations, or those of the publisher, the editors and the reviewers. Any product that may be evaluated in this article or claim that may be made by its manufacturer is not guaranteed or endorsed by the publisher.
Research integrity at Frontiers
Learn more about the work of our research integrity team to safeguard the quality of each article we publish.