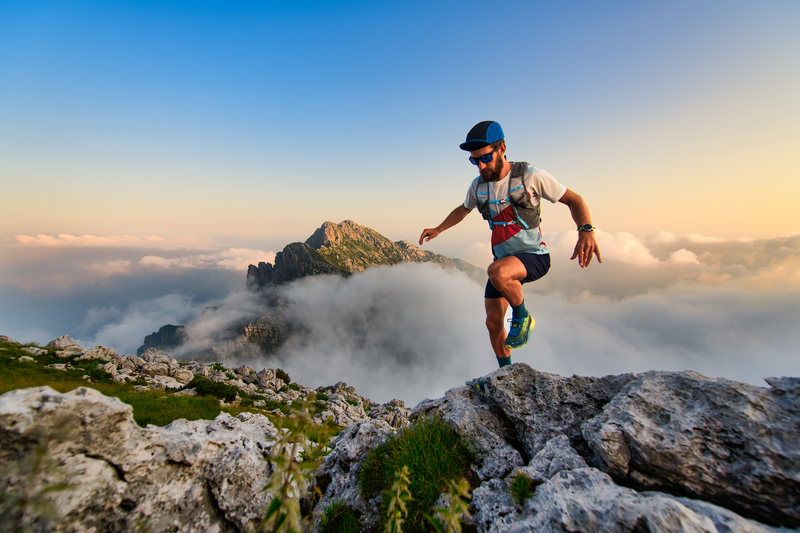
94% of researchers rate our articles as excellent or good
Learn more about the work of our research integrity team to safeguard the quality of each article we publish.
Find out more
ORIGINAL RESEARCH article
Front. Educ. , 29 June 2018
Sec. Digital Education
Volume 3 - 2018 | https://doi.org/10.3389/feduc.2018.00044
One of the persistent problems of online and distance learning is how to effectively support students' successful learning outcomes. A promising approach to this issue is to investigate the extent that learning is determined by learner characteristics and skills. This study explores the effects of domain-specific prior knowledge, intrinsic motivation, computer attitude, computer anxiety, and learning management skills on learning in a distance-training course on media pedagogy for teachers. The data were collected from 127 trainee teachers who responded to various questionnaires and completed knowledge tests. We investigated whether the selected characteristics of learners could be used to model five cognitive load assessments that included ratings of content difficulty, learning ease, experienced pressure/tension, effort/importance while learning, and performance in tests. Computer anxiety and computer attitude were significant predictors of content difficulty, learning ease, and experienced pressure/tension. In addition, higher learning-strategy skills reduced ratings of content difficulty. Effort/Importance ratings were best modeled by intrinsic motivation and learning strategy usage. Performance was best predicted by prior knowledge, intrinsic motivation, and computer attitude.
Distance and online learning have become common and reputable educational methods in vocational training and higher education (e.g., Allen and Seaman, 2016). Numerous studies investigating theoretical and applied aspects of distance and online learning have been published. However, the problem of effectively supporting successful student learning still persists (Jo et al., 2016). To gain insight into this problem, one line of research has focused on the characteristics and skills of distance and online learners and the extent to which they predict learning processes and outcomes. To advance this research, we investigated the extent to which a selected set of learner characteristics affect cognitive load of trainee teachers while learning and performing in a script-based distance-training course about media pedagogy. As characteristics, domain-specific prior knowledge, intrinsic motivation, computer attitude, computer anxiety, and learning strategies were selected, because all of them have shown to be relevant for distance and online learning in empirical studies, and they represent cognitive, motivational, affective, and skill aspects. As theoretical background of the current study, the cognitive load theory (CLT) was chosen (Sweller, 2010).
The CLT highlights the role of cognitive capacity in working memory for successful learning outcomes (Sweller, 2010). Working memory is limited in capacity. If memory items cannot be rehearsed, they decline within seconds. If the material to be learned is complex, that is, it comprises numerous information elements that are highly connected to each other, then learning will be difficult because of the limited capacity of working memory. Correspondingly, learners must keep these elements in working memory while concurrently relating them to understand the material. This cognitive burden often results in exceeding working-memory capacity (i.e., the working memory is overloaded). Consequently, successful learning is forced to occur within the limits of working memory. Accordingly, learning success is determined by cognitive processes while learning, which places cognitive loads on working-memory capacity. Sweller (2010) characterized cognitive load in terms of whether it is useful (intrinsic, germane) or unnecessary (extraneous). Intrinsic load reflects the difficulty of the learning task, resulting from the number of information elements and their interrelations that must be learned (Sweller, 2010). In other words, it is the extent that working-memory capacity is occupied by the basic processing necessary to understand the subject of the presented material. Germane cognitive load mounts on the intrinsic load, occupying additional working-memory capacity by the processes needed to construct schemas and store them in long-term memory (Sweller, 2010). Finally, extraneous cognitive load occupies working-memory capacity by the processes unrelated to subject understanding, schema construction, and automation (Sweller, 2010). Extraneous load is suggested to stem mainly from the manner in which content is presented. Hence, a bad presentation design can lead to high extraneous cognitive load, which can hinder learning. Based on the conceptions of cognitive load types, the first precondition for successful learning is that all three types of cognitive load should not overburden working-memory capacity. In general, a working memory overload often results from too much extraneous and intrinsic load (Sweller, 2010). A second precondition for optimum learning outcomes is that sufficient working-memory capacity should be occupied by germane load reflecting schema acquisition, schema automation, and schema storage.
Martin (2014) classified measuring methods for cognitive load by focusing on the causal relationship to cognitive load and the objectivity of the measure. A direct causal relationship exists when the measures directly reflect the effects of cognitive load. An indirect relationship exists when the measures are indirectly affected by cognitive load via further processes. Methods are subjective when measures reflect self-reported data, and objective when non-subjective assessments of behavior, physiological conditions, or performance are applied. Direct subjective ratings using single items have been often used to assess intrinsic cognitive load of the learning material and overall cognitive load while learning. Intrinsic load is measured by asking learners to rate the difficulty of the material. Overall cognitive load is measured by asking learners to rate the difficulty of studying. Subjective ratings of invested mental effort and stress level have been proposed for measuring overall cognitive load indirectly and directly. Accordingly, performance assessments are indirect, objective measures. Performance depends on storage and retrieval processes, which may be influenced by cognitive load. A discussion of cognitive load measures can be found in de Jong (2010).
Against the background of CLT, the concept of the expertise reversal effect comprises instructional design effects that depend on prior knowledge. It proposes that what is effective for novice learners may be ineffective for more experienced and expert learners (Kalyuga, 2014). Effects have been described in a variety of instructional designs. The focus has been often set on learning in less complex and relatively short learning situations compared to distance training. Expertise reversal effects are not expected to occur in typical distance learning courses, which mostly allow self-paced and self-sequenced learning, an adequate learning environment for experienced learners (Kalyuga, 2014). With reference to CLT, experienced learners (i.e., those with greater prior knowledge) are assumed to engage more adequately in processing the learning content than less experienced learners (i.e., those with lower prior knowledge). Prior knowledge is assumed to influence intrinsic load. The higher the level of prior knowledge, the less intrinsic load might be experienced while learning, because prior knowledge should reduce the complexity of the content. Accordingly, learners might experience less working memory load and consequently, less threat of cognitive overload. Viewed from this perspective, the level of prior knowledge can be connected to learning success. In the context of distance and online learning, which are more complex learning environments than has been investigated against the background of CLT, studies have reported that domain-specific prior knowledge is positively related to various measures of performance (e.g., McDonald and Stevenson, 1998; Stiller, 2003, 2009, in press; Amadieu et al., 2009).
Intrinsic motivation refers to performing an act because it is inherently interesting or enjoyable, and it is connected to high-quality learning (Ryan and Deci, 2000; Schunk et al., 2008). Analogously, intrinsic motivation is also assumed to play a key part in successful distance and online learning (Jones and Issroff, 2007). In general, positive correlations between motivation and performance have been reported (Fredericksen et al., 2000; Ali and Franklin, 2001; Aragon et al., 2001; Sankaran and Bui, 2001; Delialioglu, 2005; Waschull, 2005; Yukselturk and Bulut, 2007; Artino, 2008). A higher level of intrinsic motivation might make learners invest more resources in learning and process information more deeply, thus contributing to successfully passing tests (van Merriënboer and Ayres, 2005; Moreno, 2006; Moreno and Mayer, 2007; Orvis et al., 2008). With reference to CLT, motivation might influence load levels and how students manage load, especially when it is high. Generally, higher motivation is assumed to lead to higher germane load (which can be seen as high-quality learning) and consequently, to higher overall load (Vollmeyer and Rheinberg, 2006; Schnotz et al., 2009).
Self-regulated learning is considered a central factor for successful distance and online learning (Barnard et al., 2009). According to Pintrich (1999), self-regulated learning includes the use of cognitive and metacognitive learning strategies and resource management strategies. In particular, metacognitive strategies, time management, and strategies for arranging a supporting environment for learning are relevant for distance and online learning (Lee et al., 2013). Metacognitive strategies include planning, monitoring, and the regulation of cognitive processes, and resource management strategies (including time management and adapting to the study environment) are used to manage and control the study environment (Pintrich, 1999). Higher abilities to engage in effective strategies might reduce cognitive load while learning and especially avoid overload. Strategies might reduce extraneous load (being able to assign time to learning without disturbances) and foster learning (being able to focus cognitive resources on information processing more efficiently). Thus, higher ability levels in employing effective strategies might facilitate learning success. Management skills have been shown to be significant predictors of learning achievement (e.g., Yukselturk and Bulut, 2007), but the significance of management skills has been reported more often to be relevant for dropout decisions (e.g., Lee and Choi, 2011; Hart, 2012).
Attitudes are described by cognitive, affective, and conative components (Richter et al., 2010). Computer attitude might affect working memory load and thus, influence learning success. Negative computer attitudes are assumed to increase extraneous load while learning. This happens because additional task-irrelevant thoughts about the computer malfunctioning or crashing might occur, for instance. Learners with positive attitudes are assumed to have less disturbing thoughts and task-foreign cognitions and are, therefore, more likely to experience a lower level of extraneous load. Such learners are assumed to process information and construct knowledge adequately. The effects of computer attitude on learning performance have been rarely investigated. Stiller (2009, in press) found that negative effects on performance were related to negative attitudes and positive effects with positive attitudes. The effects also depended on information presentation and navigation. Stiller found that a more interactive access to textual information by clicking on parts of pictures was superior to a simpler linear page-turning access when attitudes were negative. Positive effects on course usage and persistence have also been reported (e.g., Stiller and Köster, 2016).
Computer anxiety is assumed to be a trait (Richter et al., 2010), consisting of cognitive and affective components. Similar to the effects of computer attitudes, worrisome thoughts and feelings of anxiety could increase extraneous load because of additional task-irrelevant negative thoughts about the computer and additional task-irrelevant processes of coping with negative emotions. Conversely, learners free of computer anxiety should experience less working memory load and thus be able to adequately process information and construct knowledge. In the literature, computer anxiety has been reported to directly affect computer self-efficacy, which influences the efficient use of a learning system and learning outcomes (Desai, 2001; Sam et al., 2005; Saadé and Kira, 2009; Hauser et al., 2012). Further evidence also exists that demonstrates the direct effects of computer anxiety on learning, communication, and performance (e.g., Fuller et al., 2006).
In this study, we explored the extent that learner characteristics, cognitive loads, and learning outcome are related using a script-based distance-training course in media pedagogy for trainee teachers. The research question leading the study was as follows: “To what extent do the selected motivational, affective, cognitive, and skill characteristics of learners account for cognitive load and performance?”
We assumed that a higher level of prior knowledge makes the content more comprehensible (i.e., reduces intrinsic load) and thus makes it easier to study the modules (i.e., reduces overall experienced load while learning). We expected that learners with higher levels of prior knowledge would put less effort in processing the course content and feel less pressure and tension while learning. Consequently, learning performance would be higher with higher levels of prior knowledge, and higher levels of prior knowledge would facilitate the integration of new information.
A higher level of intrinsic motivation might make learners put more effort into studying, and learners are likely to feel less pressured, because learning is less dependent on external contingencies. Allocating more resources for learning should normally increase the experienced overall load (difficulty of learning), but higher motivation might lead to an experienced ease of studying. Being more motivated might also result in higher learning performance, and because of the effort that goes into studying, intrinsic load (difficulty of content) might be rated lower.
Metacognitive learning strategies, strategies of time management, and arranging one's learning environment are three factors that have been shown to influence distance and online learning (Yukselturk and Bulut, 2007; Lee and Choi, 2011; Hart, 2012). In general, the assumptions follow that a higher level of strategy skills contributes to the preparation for adequate learning. Given that an online course is more directly managed by strategy usage, extraneous load is likely to be reduced. For example, students enrolled in an online course have the advantage of less unexpected interruptions, and they can assign periods of the day to focus on studying without any further need to manage studying while learning and focus on their learning goals without any further unnecessary goal setting and corrections. Consequently, overall cognitive load (difficulty of learning) will also decrease. Under these conditions, the effort put into learning is expected to increase with strategy usage, whereas the experienced pressure while learning should decrease. When learning occurs with minimum interruptions of any kind, performance should be higher.
Finally, negative computer attitudes and higher computer anxiety should increase the level of extraneous load while learning because of task irrelevant cognitions and regulation processes of negative emotions triggered by online learning. When high extraneous load compromises working memory, the experienced overall load should increase and knowledge construction should be disrupted, leading to lower levels of learning performance.
The data was collected from trainee teachers who had enrolled in a distance-training course about media pedagogy offered in the German federal state of Bavaria. The training addressed teachers and was promoted via flyers at all primary schools, secondary general schools, intermediate schools, and grammar schools in Bavaria (see Federal Ministry of Education and Research, 2016, pp. 95–97, for details on German school classification). The training was part of the governmental continuing vocational education and training offering for teachers. Through an online portal, 575 trainee teachers registered for the training (female: n = 414, 72%; male: n = 161, 28%; mean age M = 27.25 years, SD = 4.00, range from 20 to 49 years, n = 573). Most trainees worked in intermediate (33%) and grammar (30%) schools, followed by primary (20%) and secondary general (15%) schools. Only nine trainees worked in other school types.
Of the 575 registered trainee teachers, the data were not used from 196 students (34.1%) who completed none of the final module tests, 184 students (32.0%) who only worked on one to seven modules, and 68 students (11.8%) who completed at least one of the eight studied modules very quickly (indicating that they had not spent sufficient time with studying). The data from the remaining 127 trainee teachers (22%) who studied all eight training modules were used to analyze learning success. These best students (n = 127) were chosen, because they also formed the largest group regarding the number of studied modules (the second largest group completed one module, n = 79, and the third largest group two modules, n = 49). Furthermore, by keeping the number of studied modules constant, we excluded the impact of this variable from analyses, which might be confounded with motivation and other variables that might be correlated with the number of studied modules. Study time might also be confounded with motivation and other variables relevant for learning. Thus, we introduced a criterion for minimum study time per module (25 min of the estimated 60–90 min workload per module) to exclude learners from analyses that were suspected of not engaging seriously in learning.
The descriptive statistics analysis of the 127 trainee teachers revealed that more female (74.0%) than male (26.0%) trainees studied all eight of the training modules. The mean age of trainees was 26.12 years (SD = 2.34, range from 23 to 35 years). Most trainees worked in intermediate (45.7%) and grammar (27.6%) schools, followed by primary (18.1%), and secondary (7.9%) general schools. Only one trainee (0.8%) worked in a different school type. Almost all of the 127 trainee teachers successfully completed all eight modules (n = 126); only one student failed one of the module tests.
The starting point of the distance training was a Moodle course portal. The training consisted of an introductory module and eight modules about media pedagogy (e.g., Web 2.0—the participatory web, Risks and dangers for children and adolescents in the web, WebQuests—a method of pupil-centered learning by using the web). After enrollment, the first login of the students placed them at the beginning of the introductory module, which could be worked through voluntarily and at any time during training. The introductory module comprised information about content, technical requirements, training organization, and self-management skills needed for successful online and distance learning. Then, the students could freely decide which of the eight modules they wanted to study, the time to study them, and in which sequence they preferred to study the modules. Six screen pages linearly structured each module. The first page was the module profile, which provided an overview of the module content and the teaching goals. The second page presented an exemplary authentic real-life problem, which emphasized the relevance of the module content. The purpose of this page was to stimulate the curiosity of the students. The third page provided the module test of domain-specific prior knowledge. The test was used for activating prior knowledge and for providing feedback about students' current knowledge level before the instruction began. No time limit was set for the test. The fourth page was the instructional unit that gave access to the instructional content and supporting material, which could be used for further elaboration. The training was based on obligatory instructional module texts. The optional supporting material included, for example, links to videos, audios, webpages, and literature. The fifth page gave access to the module questionnaire that assessed study experience. The sixth page provided the final module test measuring learning success and feedback about it. A workload of 60–90 min was calculated for studying a module, thus 8–12 h were calculated for completing the entire training. Students were supported when asked via email, chat, or phone. Replies to email inquiries were normally sent within a few hours. Chat and phone consulting was possible during assigned office hours. Office hours were announced at least 4 weeks in advance.
The training was offered during a regular German school year from October to July. Any trainee teacher could enroll as a student in the training. After logging in for the first time, the students were directed to the introductory module. Then, before the students could access their first module, they completed the first online questionnaire that gathered demographic information and assessed various learner characteristics. After completing the first questionnaire, the trainee teachers could access the eight course modules. At the beginning of each module, students were required to complete a prior-knowledge test. After studying a module, students were questioned about the module before advancing to the next module by taking the final module test. Tables S1–S6 of the Supplementary File list the items of the used measurement scales (see Supplementary Material section).
The trainee teachers' motivation to study the modules was assessed with the interest/enjoyment scale of the intrinsic motivation inventory (IMI; Ryan, 1982; Leone, 2011). Then, their attitude toward computers was measured using the scale “Personal experience/learning and working/autonomous entity” from the Questionnaire of the Content-specific Measurement of Attitudes toward the Computer (QCAAC; Richter et al., 2010). This scale assesses the experience of individuals with using the computer as a means for learning and working and focusses on the negative aspects of its use, that is, regarding the computer as an uncontrollable machine. Later, computer anxiety was measured by using the scale “Confidence in dealing with computers and computer applications” of the QCAAC that assesses the cognitive and affective components. Additionally, self-regulated learning competence was measured by subjective ratings of learning strategy usage. Three strategies were assessed: metacognitive learning strategies (including planning, monitoring, and regulating), time management, and creating an adequate learning environment (see Questionnaire for Measuring Learning Strategies of Students developed by Wild and Schiefele, 1994).
The perceived cognitive load of participants while learning was assessed with four subjective instruments. Cognitive load was measured indirectly by the effort/importance scale and directly by the pressure/tension scale of the IMI (Ryan, 1982; Leone, 2011; 5-item scales). In addition, intrinsic load (rating of content difficulty) and overall load (rating of difficulty of studying) were each measured directly with one item.
In each module, a 5-item multiple-choice test was used to assess prior knowledge and a 15-item multiple-choice test to assess performance. Each performance test included the five items of the corresponding prior knowledge test (the items were presented again after learning), 10 items unknown to the learners were added for measuring performance. Each item listed four answers of which, at least one was correct. The aim of the training was to provide factual knowledge. Thus, multiple-choice tests were an appropriate instrument for measuring learning success.
All learner characteristics were measured with the first questionnaire before training began, which included intrinsic motivation, computer attitude, computer anxiety, and the three types of learning strategies, assessed with multiple-item scales. The means of items were calculated as scores and used for further analyses.
Per module, the scores of the multiple-item scales (i.e., domain-specific prior knowledge, experienced effort/importance and pressure/tension, and performance) were calculated as the mean of items. The one-item measures of intrinsic and overall load were not transformed. Then, a mean was calculated across the eight module scores (i.e., domain-specific prior knowledge, load assessments, and performance). Prior knowledge and performance scores were calculated as percent correct. The means of the eight module scores were used in the analyses.
Overall, higher scores express higher level of features except for computer attitude of which higher scores indicate less negative attitudes (for simplicity, higher scores could be thought of as “positive” attitudes). Scale features are presented in Table 1, based on all available data of the total sample (n = 575).
The correlation matrix of all measured variables is shown in Table 2. A total of 66 correlations were computed. Applying the cumulative alpha error control according to the Bonferroni-Holm correction resulted in 15 significant correlations (absolute value of r ≥ 0.29, see Table 2). Ignoring correlations smaller than the absolute value of 0.29 and assuming instead null correlations might not support the interpretation of regression results, all significant correlations were considered using a non-corrected alpha level of 0.05 (see Table 2).
The correlations between the learner characteristics show a clear pattern. Computer attitude and computer anxiety solely correlated with each other, showing a very high correlation of −0.83. The more positive the attitude toward computers, the lower the level of computer anxiety. Intrinsic motivation correlated positively with prior knowledge, the use of metacognitive learning strategies, and time management strategies. The higher the intrinsic motivation, the higher the level of prior knowledge and strategy usage. In addition, usage of the three learning strategies correlated positively with each other. Overall, correlations were in the small to medium range.
Large, positive, significant correlations were found among the various subjective direct assessments of cognitive load (i.e., intrinsic load, overall cognitive load, and pressure/tension). The higher that learners rated content difficulty (intrinsic load), the higher their experience of overall cognitive load (difficulty of studying) and their experience of pressure and tension (also an overall cognitive load measure). The indirect measure of overall cognitive load via the scale effort/importance did not correlate significantly with any of the other measures. In addition, for performance, some weak correlations with direct overall load assessments were found but not with intrinsic load and effort/importance.
Overall, the correlations between the learner characteristics and direct measures of cognitive load were medium sized, and a clear pattern also emerged. Computer attitude and computer anxiety mainly correlated significantly with these load ratings. Having a more positive computer attitude negatively correlated with intrinsic load, overall cognitive load, and experienced pressure and tension. The reverse pattern was found with computer anxiety scores. Arranging an adequate learning environment also appeared to correlate with intrinsic load. The better the learning environment, the lower the ratings of intrinsic load. The indirect measure of overall cognitive load (i.e., the experience of pressure and tension) generally correlated significantly with all learning strategies and intrinsic motivation. The higher the strategy usage and motivation scores, the higher the experience of effort and importance. Finally, prior knowledge, intrinsic motivation and computer attitude was positive related with performance. The more the students knew about the content before learning, the more they were motivated, and the more positive attitudes they had about the computer, the higher their scores in the final module tests.
All cognitive load measures could be modeled with multiple linear regression, entering the learner characteristics as predictors (see Tables 3, 4). Backward elimination models consistently resulted in a multiple correlation between 0.33 and 0.45 and an adjusted explained variance between 10% and 18%.
Intrinsic load could be modeled by computer anxiety and all learning strategies. Students who scored higher on computer anxiety and time management, reported higher intrinsic load, and students who scored higher in metacognitive strategies and the strategy of arranging an adequate learning environment, reported lower intrinsic load. The strongest predictor was computer anxiety, followed by learning environment, and the contributions of these two predictors to intrinsic load were about equally high. Time management can be interpreted as a suppression variable, because the correlation with intrinsic load was almost zero, but the beta was positive. We propose that time management suppressed the variance of the two other strategies, which correlated moderately with time management.
Overall load could best be modeled by computer anxiety and pressure/tension by computer attitude. Given that computer attitude and anxiety are highly correlated, the two regression models produced similar results. Students who scored higher on computer attitude, reported higher pressure and tension, and correspondingly, the higher the computer anxiety scores, the higher the overall load ratings.
Effort/Importance could be modeled by intrinsic motivation, time management, and strategies focusing on the learning environment. Intrinsic motivation accounted for the largest proportion of variance in effort/importance, which was slightly higher than the contribution of strategies focusing on the learning environment. Time management accounted for the smallest proportion of variance. Students who had higher intrinsic motivation and time management and learning environment strategies reported greater effort and importance.
Performance could be modeled by prior knowledge, motivation, strategies focusing on the learning environment, and computer attitude. The strongest contribution was found with prior knowledge. Higher prior knowledge and intrinsic motivation scores and more positive computer attitudes resulted in higher student performance on the final module tests. We interpreted learning environment strategies as traditional suppression variable because of the positive correlation with performance and the negative beta. We suppose that learning environment suppressed the variance of intrinsic motivation, which correlated 0.25 with learning environment strategies.
The self-correlation of residuals from regression models was tested with the Durbin-Watson test, which calculates values in the range of 0–4. Intervals of 1.5–2.5 are commonly accepted as indicating the absence of self-correlations of residuals. Inspecting the Durbin-Watson test values for all calculated models in Table 3 revealed that all values fell within the interval and thus no self-correlations of residuals per model were assumed.
Homoscedasticity was tested by the Koenker test (Koenker, 1981). Homoscedasticity was not violated for intrinsic load (λ2 = 2.04, df = 4, p = 0.73), overall load (λ2 = 0.68, df = 1, p = 0.41), pressure/tension (λ2 = 0.31, df = 1, p = 0.58), effort/importance (λ2 = 1.21, df = 3, p = 0.75), and performance (λ2 = 7.90, df = 4, p = 0.10).
Collinearity analysis computes tolerance values for correlations between predictors. Values less than 0.10 indicate collinearity of predictors, and values near 1 are unproblematic. The tolerance index of the predictors for all regression models were over 0.74 and mostly approached 1, thus indicating non-collinearity of predictors (see Table 4).
In the literature, researchers often suggest to investigate the characteristics of successful distance and online learners to inform educators about designing high-quality courses and programs that fit the needs of distance and online students (Yukselturk and Bulut, 2007). Therefore, we focused on the relationship between student characteristics and learning success and conducted analyses with data gathered from trainee teachers in a script-based distance course about media pedagogy. This study explored the extent that important learner characteristics help students succeed and to propose how to prepare distance courses based on the results.
The correlation and regression analyses revealed that domain-specific prior knowledge, intrinsic motivation, and computer attitude positively correlated with performance, with prior knowledge showing the highest predictive value. No other student characteristics significantly correlated with performance. These findings are consistent with previous research results on prior knowledge (McDonald and Stevenson, 1998; Stiller, 2003, 2009, in press; Amadieu et al., 2009), intrinsic motivation (Fredericksen et al., 2000; Ali and Franklin, 2001; Aragon et al., 2001; Sankaran and Bui, 2001; Delialioglu, 2005; Waschull, 2005; Yukselturk and Bulut, 2007; Artino, 2008), computer attitude (Stiller, 2009, in press), and computer anxiety (Desai, 2001; Sam et al., 2005; Saadé and Kira, 2009; Hauser et al., 2012). The analyses of attitude toward computers and computer anxiety produced equivalent results, which reflects the redundancy of the measures as shown by the very high correlation between them. Thus, results according to one characteristic are not separable from the other. Overall, the results can be interpreted as hypothesized. Higher levels of prior knowledge facilitate integrating new information, higher levels of intrinsic motivation will likely result in more effort when studying and thus result in higher test performance, negative computer attitudes and higher levels of computer anxiety increase extraneous load while learning, disrupting knowledge construction and leading to lower levels of test performance.
Self-regulation skills are proposed to influence successful distance learning, but learning strategies in this study had little direct effect on performance. This result appears to contradict previous research (Yukselturk and Bulut, 2007). However, after scrutinizing the correlations between the self-regulation skills and the load measures, the skills appeared to influence intrinsic load and effort. The skill to arrange an adequate learning environment mainly correlated significantly and negatively with intrinsic load but positively with effort. Moreover, according to the backward regression analyses, the skill was a significant predictor in the regression models in which intrinsic load and effort were the outcome measures. The metacognitive strategy skill was also a significant predictor in the intrinsic load model and time management in the effort model. These results indicate that self-regulation skills nevertheless are important for arranging and organizing learning and thus contribute to successful learning. In other words, self-regulation skills help to create learning environments such that an adequate control of the time and space facilitates the effort and the controlling, monitoring and regulation of learning, and thus it keeps cognitive load more easily manageable.
Why was performance not influenced by self-regulation skills in this study? It should be noted that in this study only factual knowledge was required to be learned and the module tests accordingly only assessed factual knowledge. Against the background of CLT, factual knowledge is already acquirable when using surface learning strategies (e.g., information rehearsal, rote learning strategies), whereas higher knowledge (e.g., knowledge about systems and their functioning) requires deeper learning strategies, such as elaboration, critical thinking, and organizing knowledge, and a corresponding assessment (e.g., practical and transfer tasks). Thus, only higher-level, self-regulation skills might contribute to deeper learning, whereas lower-level skills might already enable surface learning. In other words, lower levels of self-regulation might support the learning of less difficult content to the same extent as higher levels of self-regulation. Studying complex information might then only benefit from higher-level, self-regulation skills.
Intrinsic motivation not only influenced test performance, it correlated positively with invested effort. Furthermore, intrinsic motivation was a significant predictor of invested effort and performance. These results indicate that intrinsic motivation contributes to learning success by managing effort while learning. This result is consistent with the reviewed literature on learning success.
Overall, the results appear to contradict prior research on learning, but when the focus on performance also includes cognitive-load indices, the results are consistent with the literature. We conclude from the results that students' self-regulation and motivation should be supported, and prior knowledge should be considered in designing training. In addition, distance training should also be designed in a way that reduces computer anxiety and to support more positive computer attitudes.
To support self-regulation, “learning strategies could be provided to enhance students' achievement of intended learning outcomes” (Yukselturk and Bulut, 2007, p. 80). In our study, this support was provided in the introductory module of the training (students were told how to learn best), which might have also contributed to the reduced effects of self-regulation skills. In general, support could be given in smaller, continuous steps while studying when learners have skill deficits; otherwise, guidance would not be necessary. Recently, Nikolaki et al. (2017) emphasized that the supporting teachers (e.g., providing feedback and reflections on learning), the provided educational materials (e.g., stating the aims and objectives of the material and the expected outcomes), and the educational tools (e.g., interactive learning tools and collaborative tools) influence the development and use of self-regulated learning strategies in distance learning.
Motivation should be kept at a high level through instructional activities during the distance training (Yukselturk and Bulut, 2007). We developed the training with the objective of providing a stimulating learning environment that motivates students (Keller and Kopp, 1987; Keller, 2010), which might have contributed to the reduction of intrinsic motivation effects. In general, in any distance course, increasing the appeal of a course will not eliminate motivational effects. Similar to self-regulation skills, a more individualized, adaptive course design might be better to support learners with lower intrinsic motivation, for example, by increasing the relevance of the material through more individualized case examples (Keller, 2010).
Finally, training should be more tailored to student's prior knowledge (Kalyuga, 2012). This task can be accomplished by initial diagnostics and subsequent didactic decisions about which adaptive instruction to provide (Kalyuga, 2012). For less knowledgeable students, providing smaller steps of knowledge acquisition (e.g., splitting modules into smaller modules that build on one another) to support the construction of useful schemas could promote better outcomes. Kalyuga (2012), for example, summarized appropriate methods for learners with inadequate prior knowledge and for learners who are more advanced.
Kalyuga (2012) suggested “developing cognitively efficient adaptive IDE [interactive distance education] … as an important trend in this area of educational research and practice” (p. 183). Accordingly, adaptive methods are the means to tailor an instruction to the various individual characteristics of students, which could be cognitive, affective, or motivational characteristics. Adaptive methods are used to dynamically accommodate content, methods, and procedures to learner characteristics and are considered to support more efficient learning (Kalyuga, 2012).
The results of this study should be interpreted carefully. The method was a non-experimental field study with a special type of script-based distance training. Results should be replicated under more controlled conditions. The sample was also a special group of trainee teachers who studied and completed all eight modules of the training. Hence, the analyses were computed for the most successful learners. The analyses, which were computed in part on means calculated across the eight modules, could have led to underestimated or undetected effects. When learners are studying over a longer period, they might change in their characteristics, for example, in intrinsic motivation, computer anxiety, and computer attitudes. Online training courses can be regarded as interventions that might change computer anxiety and computer attitude (Dupin-Bryant, 2002; Woszczynski et al., 2004). If computer anxiety decreases and computer attitudes become more positive, the overall effects will be undetected.
When a modularized distance training is provided, further research should investigate learning experiences and outcomes while learners study a sequence of single modules. This method would allow extending the investigation to less successful learners who fail to complete all of the modules. A prerequisite for analyzing the course of learning and its preceding learner characteristics is aimed to implement a repeated measures design, which is closer to the actual process of learning. For example, the regulation of learning through strategies is proposed to be a more valid indicator of learning quality than a trait-based measure of strategy usage skills. In addition, the promising methods of learning analytics and data mining could complement such a research strategy with objective data gathered by log systems catching online traces (Johnson et al., 2014; Cohen, 2017). Such modern approaches could help to identify learners that have learning problems (e.g., Cohen, 2017).
An ethics approval was not required as per the guidelines of the Department of Educational Science, University of Regensburg, and national regulations. For the study, there were no ethics-relevant problems. Distance students completed questionnaires, and data about their usage of the online learning environment were collected. No manipulation was applied and no misleading information about the goal of the study were given. The materials and procedures were also not invasive. In accordance with the guidelines of the Ethical Committee of the German Psychological Society, anonymity of data was established and all subjects gave written informed consent for data collection and analyses and data usage for scientific purposes.
KS has analyzed, interpreted the data, and written the paper. RB has developed the conception and design of the study including the distance training and collected the data.
The authors declare that the research was conducted in the absence of any commercial or financial relationships that could be construed as a potential conflict of interest.
This work was supported by the German Research Foundation (DFG) within the funding programme Open Access Publishing.
The Supplementary Material for this article can be found online at: https://www.frontiersin.org/articles/10.3389/feduc.2018.00044/full#supplementary-material
Ali, A., and Franklin, T. (2001). Internet use in the classroom: potentials and pitfalls for student learning and teacher-student relationships. Educ. Technol. 41, 57–59. Available online at: http://www.jstor.org/stable/44428684
Allen, I. E., and Seaman, J. (2016). Online Report Card: Tracking Online Education in the United States. Babson Park, MA: Babson Research Group.
Amadieu, F., Tricot, A., and Mariné, C. (2009). Exploratory study of relations between prior knowledge, comprehension, disorientation and on-line processes in hypertext. Ergonom. Open J. 2, 49–57. doi: 10.2174/1875934300902010049
Aragon, S. R., Johnson, S. D., and Shaik, N. (2001). “A preliminary analysis of learning style influence on student success in online vs. face-to-face environments,” in Learning for the Future, eds B. Cope and M. Kalantzis (Melbourne, VIC: Common Ground Publishing), 3–17.
Artino, A. R. (2008). Motivational beliefs and perceptions of instructional quality: predicting satisfaction with online training. J. Comput. Assist. Lear. 24, 260–270. doi: 10.1111/j.1365-2729.2007.00258.x
Barnard, L., Lan, W. Y., To, Y. M., Osland Paton, V., and Lai, S.-L. (2009). Measuring self-regulation in online and blended learning environments. Int. High Educ. 12, 1–6. doi: 10.1016/j.iheduc.2008.10.005
Cohen, A. (2017). Analysis of student activity in web-supported courses as a tool for predicting dropout. Educ. Tech. Res. Dev. 65, 1285–1304. doi: 10.1007/s11423-017-9524-3
de Jong, T. (2010). Cognitive load theory, educational research, and instructional design: some food for thought. Instr. Sci. 38, 105–134. doi: 10.1007/s11251-009-9110-0
Delialioglu, O. (2005). “Investigation of source of motivation in a hybrid course,” in Presented at Association for Educational Communications and Technology Annual Meeting 2005. Available online at: https://eric.ed.gov/?id=ED485032
Desai, M. S. (2001). Computer anxiety and performance: an application of a change model in a pedagogical setting. J. Instr. Psychol. 28, 141–154.
Dupin-Bryant, P. (2002). Reducing computer anxiety in adult microcomputer training. J. Exten. 40. Available online at: https://www.joe.org/joe/2002october/tt3.php
Federal Ministry of Education Research (2016). Education and Research in Figures 2016. Available online at: https://www.bmbf.de/pub/Education_and_Research_in_Figures_2016.pdf
Fredericksen, E., Pickett, A., Shea, P., Pelz, W., and Swan, K. (2000). Student satisfaction and perceived learning with on-line courses: principles and examples from the SUNY learning network. Online Learn. 4, 7–38. Available online at: https://onlinelearningconsortium.org/jaln_article/student-satisfaction-and-perceived-learning-with-on-line-courses-principles-and-examples-from-the-suny-learning-network-2/
Fuller, R. M., Vician, C., and Brown, S. A. (2006). E-learning and individual characteristics: the role of computer anxiety and communication apprehension. J. Comput. Inform. Syst. 46, 103–115. doi: 10.1080/08874417.2006.11645917
Hart, C. (2012). Factors associated with student persistence in an online program of study: a review of the literature. J. Interact. Online Learn. 11, 19–42. Available online at: http://www.ncolr.org/issues/jiol/v11/n1/factors-associated-with-student-persistence-in-an-online-program-of-study-a-review-of-the-literature
Hauser, R., Paul, R., and Bradley, J. (2012). Computer self-efficacy, anxiety, and learning in online versus face to face medium. J. Inform. Technol. Educ. Res. 11, 141–154. doi: 10.28945/1633
Jo, I.-H., Park, Y., Yoon, M., and Sung, H. (2016). Evaluation of online log variables that estimate learners' time management in a Korean online learning context. Int. Rev. Res. Open Dis. 17, 195–213. doi: 10.19173/irrodl.v17i1.2176
Johnson, L., Adams Becker, S., Estrada, V., and Freeman, A. (2014). NMC Horizon Report: 2014 Higher Education Edition. Austin, TX: The New Media Consortium. Available online at: https://www.nmc.org/publication/nmc-horizon-report-2014-higher-education-edition/
Jones, A., and Issroff, K. (2007). “Learning technologies: affective and social issues,” in Contemporary Perspectives in e-learning Research: Themes, Methods and Impact on Practice, eds G. Conole and M. Oliver (London: Routledge), 190–202.
Kalyuga, S. (2012). Interactive distance education: a cognitive load perspective. J. Comput. High. Educ. 24, 182–208. doi: 10.1007/s12528-012-9060-4
Kalyuga, S. (2014). “The expertise reversal principle in multimedia learning,” in The Cambridge Handbook of Multimedia Learning, ed R. E. Mayer (New York, NY: Cambridge University Press), 576–597.
Keller, J. M. (2010). Motivational Design for Learning and Performance: The ARCS Model Approach. New York, NY: Springer.
Keller, J. M., and Kopp, T. W. (1987). “An application of the ARCS model of motivational design,” in Instructional Theories in Action: Lessons Illustrating Selected Theories and Models, ed C. M. Reigeluth (Hillsdale, MI: Erlbaum), 289–320.
Koenker, R. (1981). A note on studentizing a test for heteroskedascity. J. Econom. 17, 107–112. doi: 10.1016/0304-4076(81)90062-2
Lee, Y., and Choi, J. (2011). A review of online course dropout research: implications for practice and future research. Educ. Technol. Res. Dev. 59, 593–618. doi: 10.1007/s11423-010-9177-y
Lee, Y., Choi, J., and Kim, T. (2013). Discriminating factors between completers of and dropouts from online learning courses. Br. J. Educ. Technol. 44, 328–337. doi: 10.1111/j.1467-8535.2012.01306.x
Leone, J. (2011). Intrinsic Motivation Inventory (IMI). Available online at: http://selfdeterminationtheory.org/intrinsic-motivation-inventory/
Martin, S. (2014). Measuring cognitive load and cognition: metrics for technology-enhanced learning. Educ. Res. Eval. 20, 592–621. doi: 10.1080/13803611.2014.997140
McDonald, S., and Stevenson, R. J. (1998). Effects of text structure and prior knowledge of the learner on navigation in hypertext. Hum. Factors 40, 18–27. doi: 10.1518/001872098779480541
Moreno, R. (2006). Does the modality principle hold for different media? A test of the method-affects-learning hypothesis. J. Comput. Assist. Lear. 22, 149–158. doi: 10.1111/j.1365-2729.2006.00170.x
Moreno, R., and Mayer, R. (2007). Interactive multimodal learning environments: special issue on interactive learning environments: contemporary issues and trends. Educ. Psychol. Rev. 19, 309–326. doi: 10.1007/s10648-007-9047-2
Nikolaki, E., Koutsoumpa, M., Lykesas, G., Venetsanou, F., and Savidou, D. (2017). The support and promotion of self-regulated learning in distance education. Eur. J. Open Dist. E-learn. Available online at: http://www.eurodl.org/materials/briefs/2017/Nikolaki_et_al.pdf
Orvis, K. A., Horn, D. B., and Belanich, J. (2008). The roles of task difficulty and prior videogame experience on performance and motivation in instructional videogames. Comput. Hum. Behav. 24, 2415–2433. doi: 10.1016/j.chb.2008.02.016
Pintrich, P. R. (1999). The role of motivation in promoting and sustaining self-regulated learning. Int. J. Educ. Res. 31, 459–470. doi: 10.1016/S0883-0355(99)00015-4
Richter, T., Naumann, J., and Horz, H. (2010). Eine revidierte Fassung des Inventars zur Computerbildung (INCOBI-R) [A revised version of the Computer Literacy Inventory]. Z Padagog. Psychol. 24, 23–37. doi: 10.1024/1010-0652/a000002
Ryan, R. M. (1982). Control and information in the intrapersonal sphere: an extension of cognitive evaluation theory. J. Pers. Soc. Psychol. 43, 450–461. doi: 10.1037/0022-3514.43.3.450
Ryan, R. M., and Deci, E. L. (2000). Intrinsic and extrinsic motivations: classic definitions and new directions. Contemp. Educ. Psychol. 25, 54–67. doi: 10.1006/ceps.1999.1020
Saadé, R. G., and Kira, D. (2009). Computer anxiety in e-learning: the effect of computer self-efficacy. J. Info. Tech. Edu. Res. 8, 177–191. doi: 10.28945/166
Sam, H. K., Othman, A. E. A., and Nordin, Z. S. (2005). Computer self-efficacy, computer anxiety, and attitudes toward the internet: a study among undergraduates in Unimas. J. Educ. Technol. Soc. 8, 205–219. Available online at: http://ir.unimas.my/id/eprint/17413
Sankaran, S. R., and Bui, T. (2001). Impact of learning strategies and motivation on performance: a study in web-based instruction. J. Instr. Psychol. 28, 191–198. Available online at: https://www.learntechlib.org/p/95445/
Schnotz, W., Fries, S., and Horz, H. (2009). “Some motivational aspects of cognitive load theory,” in Contemporary Motivation Research: From Global to Local Perspectives, eds S. Wosnitza, S. A. Karabenick, A. Efklides, and P. Nenniger (Göttingen: Hogrefe), 86–113.
Schunk, D. H., Pintrich, P. R., and Meece, J. L. (2008). Motivation in Education. Upper Saddle River, NJ: Pearson Merrill Prentice Hall.
Stiller, K. (2003). Lernstrategien und Lernerfolg beim computerbasierten Wissenserwerb [Learning strategies and achievement in computer-based knowledge acquisition]. Psychol. Erz. Unterr. 50, 258–269.
Stiller, K. D. (2009). Mono- und bimodale Textpräsentationen zu Bildern in Hypermedia-Systemen [Mono- and bimodal text presentations accompanying pictures in hypermedia systems]. Psychol. Erz. Unterr. 56, 49–63. Available online at: https://www.reinhardt-journals.de/index.php/peu/article/view/686
Stiller, K. D. (in press). Fostering learning via pictorial access to on-screen text. J Educ Multimed Hypermedia. 27.
Stiller, K. D., and Köster, A. (2016). Learner attrition in an advanced vocational online training: the role of e-learning experience, computer attitude, and computer anxiety. Eur. J. Open Dist. E-Learn. 19, 1–14. doi: 10.1515/eurodl-2016-0004
Sweller, J. (2010). Element interactivity and intrinsic, extraneous, and germane cognitive load. Educ. Psychol. Rev. 22, 123–138. doi: 10.1007/s10648-010-9128-5
van Merriënboer, J. J. G., and Ayres, P. (2005). Research on cognitive load theory and its design implications for e-learning. Educ. Tech. Res. 53, 5–13. doi: 10.1007/BF02504793
Vollmeyer, R., and Rheinberg, F. (2006). Motivational effects on self-regulated learning with different tasks. Educ. Psychol. Rev. 18, 239–253. doi: 10.1007/s10648-006-9017-0
Waschull, S. B. (2005). Predicting success in online psychology courses: self-discipline and motivation. Teach. Psychol. 32, 190–192. doi: 10.1207/s15328023top3203_11
Wild, K.-P., and Schiefele, U. (1994). Lernstrategien im Studium: Ergebnisse zur Faktorenstruktur und Reliabilität eines neuen Fragebogens [Learning strategies of university students: Factor structure and reliability of a new questionnaire]. Zeitschr. Differentielle Diagnostische Psychol. 15, 185–200.
Woszczynski, A. B., Lazar, L. D., and Walker, J. M. (2004). “Does training reduce computer anxiety?” in SAIS 2004 Proceedings. Available online at: http://aisel.aisnet.org/sais2004/20
Keywords: distance training, teacher training, higher education, self-regulated learning, computer attitude, computer anxiety, cognitive load theory
Citation: Stiller KD and Bachmaier R (2018) Cognitive Loads in a Distance Training for Trainee Teachers. Front. Educ. 3:44. doi: 10.3389/feduc.2018.00044
Received: 07 February 2018; Accepted: 28 May 2018;
Published: 29 June 2018.
Edited by:
Fabrizio Consorti, Sapienza Università di Roma, ItalyReviewed by:
Gabriella Agrusti, Libera Università Maria SS. Assunta, ItalyCopyright © 2018 Stiller and Bachmaier. This is an open-access article distributed under the terms of the Creative Commons Attribution License (CC BY). The use, distribution or reproduction in other forums is permitted, provided the original author(s) and the copyright owner(s) are credited and that the original publication in this journal is cited, in accordance with accepted academic practice. No use, distribution or reproduction is permitted which does not comply with these terms.
*Correspondence: Klaus D. Stiller, a2xhdXMuc3RpbGxlckB1ci5kZQ==
Disclaimer: All claims expressed in this article are solely those of the authors and do not necessarily represent those of their affiliated organizations, or those of the publisher, the editors and the reviewers. Any product that may be evaluated in this article or claim that may be made by its manufacturer is not guaranteed or endorsed by the publisher.
Research integrity at Frontiers
Learn more about the work of our research integrity team to safeguard the quality of each article we publish.