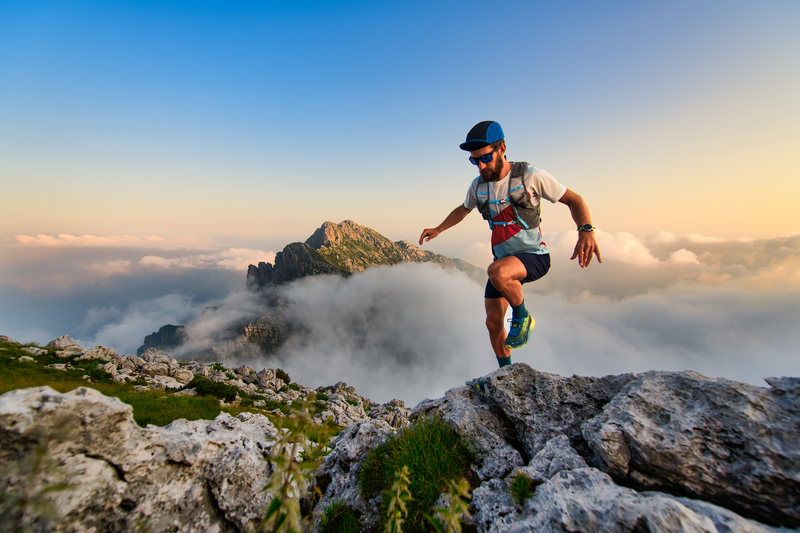
94% of researchers rate our articles as excellent or good
Learn more about the work of our research integrity team to safeguard the quality of each article we publish.
Find out more
ORIGINAL RESEARCH article
Front. Educ. , 01 May 2018
Sec. Assessment, Testing and Applied Measurement
Volume 3 - 2018 | https://doi.org/10.3389/feduc.2018.00030
Using multiple admission tools in university admission procedures is common practice. This is particularly useful if different admission tools uniquely select different subgroups of students who will be successful in university programs. A signal-detection approach was used to investigate the accuracy of Secondary School grade point average (SSGPA), an admission test score (ACS), and a non-cognitive score (NCS) in uniquely selecting successful students. This was done for three consecutive first year cohorts of a broad psychology program. Each applicant's score on SSGPA, ACS, or NCS alone—and on seven combinations of these scores, all considered separate “admission tools”—was compared at two different (medium and high) cut-off scores (criterion levels). Each of the tools selected successful students who were not selected by any of the other tools. Both sensitivity and specificity were enhanced by implementing multiple tools. The signal-detection approach distinctively provided useful information for decisions on admission instruments and cut-off scores.
Admission committees of selective university programs need information on the accuracy of admission tools and on the selection outcomes that result from them. The literature on admission and selection at universities focuses primarily on predicting academic achievement in terms of grade point averages, retention and study length. Whereas in North America standardized admission tests, such as the SAT (Scholastic Aptitude Test) or ACT (American College Testing), are available and across the world standardized tests are being developed and fine-tuned, these are to date not employed in Europe. European university programs use program-specific admission procedures and tools, partly depending on how access to university programs is regulated for these programs, or in specific countries (De Witte and Cabus, 2013; Schripsema et al., 2014; Makransky et al., 2016). Research into predictors other than secondary school grade point average (SSGPA)—which is far from accurate—is still work in progress, and a “gold standard” has not yet been conceived (Richardson et al., 2012; Schripsema et al., 2014, 2017; Shulruf and Shaw, 2015; Makransky et al., 2016; Patterson et al., 2016; Pau et al., 2016; Sladek et al., 2016; Yhnell et al., 2016; Wouters et al., 2017). Program- and institution specific work samples appear promising valuable predictors of academic achievement in addition to past academic achievement (Niessen et al., 2016; Stegers-Jager, 2017; van Ooijen-van der Linden et al., 2017).
Currently, “best practices” in the implementation of admission tools appear to include clearly cognitive measures, such as past academic achievement (secondary school grade point average, SSGPA) and current academic achievement (cognitive admission tests), but also non-cognitive, non-intellectual measures (Robbins et al., 2004; Richardson et al., 2012; Sternberg et al., 2012; Cortes, 2013; Steenman et al., 2016). These latter measures are intended to capture motivation, community commitment or leadership skills that may be predictive of future academic achievement, and clearly differ from testing gained knowledge and competencies, such as writing, reasoning and abstract thinking (Urlings-Strop et al., 2017). To date, the available knowledge about the characteristics of admission tools is primarily based on analyses at group level (Schmitt, 2012; Stegers-Jager et al., 2015). Yet, the importance of more information on differences between institutions, year levels and admission instruments has become clear by studies like those of Edwards et al. (2013), Schripsema et al. (2017), and Stegers-Jager et al. (2015), in which the existence of such differences was established.
Signal Detection Theory (Green and Swets, 1966; Stanislaw and Todorov, 1999; Macmillan and Creelman, 2005) provides the framework and analyses to study the effects of admission tools and specific cut-off scores (criteria) to use both at the group and at the individual level. As is depicted in Figure 1, regression analyses and Signal Detection Theory complement each other. Regressions provide information on the relative contributions of specific predictors in specific models and thus provide an evidence-base for designing the best possible program-specific model explaining academic success. The performance of the model as informed by regressions, is best monitored using a Signal Detection approach. This is, as far as we know, currently not common practice at selective programs, but selection of capable students is a signal detection problem. For each applicant, the question at the time of application is whether she or he will be successful and graduate. The decision to admit an individual applicant or to advise to reconsider the application is made in uncertainty. Of course, prior academic achievement and admission tests are informative, but not all who performed well before will continue to do so and not all who barely made it to application will continue to struggle and fail. In Signal Detection Theory, two possible incorrect decisions as well as two possible correct decisions together describe the total outcome. Admitted applicants who later fail are deemed “false alarms” and rejected applicants who would have been successful are deemed “misses.” Admitted applicants who become successful are deemed “hits” and rejected applicants who would have failed are deemed “correct rejections.” The accuracy of an admission tool is calculated based on the hit-rate (the proportion of admitted students of the total number of successful students) and the false-alarm-rate (the proportion of admitted students of the total number of unsuccessful students). Signal Detection Theory allows monitoring of admission outcomes: after having awaited the academic achievement of freshman in their first courses or first year (or other forms of academic achievement), a hypothetical, retrospective selection can be performed and used to inform future admission decisions. Different admission tools and different cut-off scores can be compared in terms of the resulting proportions correct and incorrect decisions and on the resulting proportions of two types of incorrect decisions. In addition to the information of the general effects of different criteria, Signal Detection Theory offers information at the individual level. In short, each applicant turns out to be either a successful or an unsuccessful student and the—retrospective, hypothetical admission decision based on a specific admission tool and a specific criterion—after having awaited actual academic success, is correct or incorrect for that specific individual (see Figures 1, 2).
Figure 1. Scheme of how regression analyses and Signal Detection Theory can—in general—be used to design admission tools and to monitor their accuracy across cohorts. a—The literature provides information on the validity of certain predictors for certain types of academic success. b—The findings from the literature inform decisions on program-specific admission tools, procedure, and data collection. c—Regression analyses show which model best explains academic success. d—Signal Detection Theory informs whether the model performs as expected from the regression analyses, adding information on sensitivity, specificity and selection outcomes depending on the application of specific criteria. The Signal Detection outcomes can be used in quality assurance of admission: it provides information on group level, the individual level and on the effects of specific criteria. It thus allows evaluation of the operationalization of academic success and the admission tools used. This, in turn, informs future data collection.
Figure 2. Graphical representation of how we used Signal Detection Theory to investigate validity and reliability of admission instruments by monitoring accuracy of instruments across three cohorts. Successfulness is defined as having passed at least seven of eight first year courses. We used SSGPA, ACS, NCS, and all combinations of those (seven admission tools) as predictors and ≥6.5 and ≥7 as criteria. The blue numbers in this figure correspond to the blue numbers in Figure 1. (1) Defining and operationalizing academic success: Y1 = successfulness = having passed at least seven of eight first year courses. (2) Choosing predictors of academic success: SSGPA = secondary school grade point average, ACS = cognitive admission test score, NCS = non-cognitive admission test score. These were the “single” tools. Another three “double” tools were constructed to determine whether students had scored at or above criterion on both-SSGPA-and-ACS-but-not-NCS, both-SSGPA-and-NCS-but-not-ACS and both-ACS-and-NCS-but-not-SSGPA. From the three single admission tools, we constructed “SSGPA/ACS/NCS” which determined whether students scored at or above criterion on all three single predictors (SSGPA and ACS and NCS) and not on only one or two of the three. (3) Choosing which criteria applicants scoring at or above are hypothetically admitted, will be applied: ≥6.5 and ≥7. (4) Calculating the outcomes of the hypothetical selection. Each individual student was hypothetically classified a hit, miss, false alarm, or correct rejection based on their score on each of the seven admission tools and their academic success. Hypothetical rejection of an unsuccessful student is a correct rejection, hypothetical rejection of successful student is a miss, hypothetical admission of an unsuccessful student is a false alarm and hypothetical admission of a successful student is a hit. AUC = area under the curve (see Figure 3).
Signal Detection Theory allows admission committees to acquire knowledge not only on the validity of admission tools but also on the effects of applying less or more selective criteria: more selective criteria might lead to a larger proportion of misses, for instance, while more liberal criteria may lead to an increase in the proportion of false alarms. Furthermore, the pay-off matrix—in which costs and benefits are assigned to both types of incorrect and correct decisions—can be used to prescribe a criterion—to apply in admission of future cohorts—that suits the goals of the admission committee. Since both sensitivity and specificity are used in a signal detection approach to calculate the accuracy of admission tools in discerning successful students from unsuccessful students, and because it offers description and prescription of criteria, it provides more information than the conventional regression analyses (Green and Swets, 1966; Kiernan et al., 2001; Macmillan and Creelman, 2005; van Ooijen-van der Linden et al., 2017). If, for example, an admission committee is inclined to prevent rejecting applicants that would have been successful students and/or if the goal is to convincingly advise applicants that are likely to fail to reconsider their choice, the committee needs to know which instruments and which criteria allow this with sufficient accuracy.
Equal accuracy, or even equal sensitivity (hit-rate) and specificity (1—false alarm-rate), of different admission tools does not necessarily mean these tools admit the same individuals. An individual applicant might be a “hit” according to their SSGPA, but a “miss” according to an admission test score. For another applicant, this might be the other way around. Even if their overall accuracy is comparable, causing the tools to appear interchangeable, each admission tool could yield unique hits and false alarms. If so, a set of such tools is more useful than each of the single tools alone. After awaiting actual academic achievement and thus the proportions of successful and unsuccessful students in a cohort, admission tools can be compared on the proportions of unique hits and unique false alarms each tool would have yielded at a certain criterion. Unless the set of admission tools is either completely non-discriminative or perfectly accurate, there will be successful students that would not have been admitted by any of the tools at a given criterion: these are “Total Misses.” There will also be unsuccessful students that would not have been admitted by any of the tools at the set criteria: these are “Total Correct Rejections.” The more selective admission to a program is, the more relevant a detailed comparison of accuracy of tools, of their ability to uniquely successfully select students and of the effects of certain criteria becomes.
The data presented in the current study include not only the hits and false alarms, but also the misses and correct rejections—not normally available after selection of students—because none of the applicants were rejected due to the characteristics of the selection procedure used here. This provides a unique opportunity to investigate the prediction of academic achievement of applicants that would have been rejected in a more competitive setting. The accuracy of SSGPA, a cognitive pre-admission test score (ACS) and a non-cognitive pre-admission test score (NCS) are compared for three consecutive cohorts of first year students of a psychology bachelor program. ACS is the average grade for a multiple-choice exam and an essay in a study sample in which applicants “studied for a week.” NCS is a self-reported measure of community commitment and motivation calculated from data from the application form. This set of predictors was chosen based on empirical findings on prediction of academic achievement (e.g., Richardson et al., 2012; Cortes, 2013; Patterson et al., 2016).
Provided accuracy of admission tools is found to be stable across cohorts, analyses of which tool uniquely successfully selects or rejects which number of individuals allow program-specific informed decisions on the admission tools and criteria used. The main question we investigate here, is to what extent different admission tools uniquely predict academic success for individual cases.
In 2013 we piloted our newly developed matching program (for the psychology bachelor program at Utrecht University in The Netherlands) in which applicants “studied for a week”; they followed lectures and a class, studied at home, wrote an essay and took a multiple-choice exam. The matching program was designed to provide applicants with a work sample of their first year. Students report the matching program to be representative and informative in written and oral evaluations. In 2013 the matching program was optional and 53.2% of the 524 students that started their first year in September had participated. In 2014 80% of a maximum of 550 to be enrolled applicants were admitted based on their total admission scores (based on their SSGPA, ACS, and NCS which were rank numbered). The other 20% were admitted through a weighted lottery. In 2015 all applicants were obliged to participate and were again rank numbered on a total admission score based on their SSGPA, ACS, and NCS to a maximum of 520 to be enrolled. In both 2014 and 2015, the number of applicants did not exceed the numerus clausus and therefore all applicants were admitted.
In 2013 279 enrolled students had fully participated in the matching program (229 females with a mean age of 19.1 years, SD = 1.1, and 50 males with mean age 19.9 years, SD = 1.9), in 2014 347 students (273 females with a mean age of 19.3 years, SD = 1.9, and 74 males with mean age 19.9 years, SD = 1.9), and in 2015 377 students (304 females with a mean age of 19.1 years, SD = 1.1, and 73 males with mean age 20.3 years, SD = 1.6). The gender distributions in these samples are representative for the gender distribution in the program. Informed consent was not needed for publication of the data we report here, as per our institution's guidelines and national regulations. This project is part of larger project, which was approved by the Ethics Committee of the Faculty of Social and Behavioral Sciences of Utrecht University.
After the students completed their first year, we determined whether they passed at least seven of eight first year courses as a measure of academic success. Those who did were considered successful, students who did not were considered unsuccessful (Y1).
The application form and the matching program provided three admission tools, see Tables S1 and S2 in Online Resource 1: Secondary School Grade Point Average (SSGPA), a cognitive admission test score (ACS), and a non-cognitive admission score (NCS). SSGPA and NCS were obtained from the application form. Applicants reported their grades for the four obligatory topics in secondary education in the Netherlands—mathematics, Dutch, English and social sciences—and biology if applicable, as available at the time of application. For most applicants, these are their pre-exam year grades as they apply to university in their exam year, usually at the age of 17 or 18. SSGPA consisted of the average of these four or five grades. In the application form of later cohorts, the grades of all topics were included to capture secondary school academic achievement more completely. NCS is a measure of motivation based on self-report and behavioral measures: it was calculated from questions regarding grade goal (whether applicants are willing to work harder for grades higher than necessary to pass courses), community commitment (self-report of community service activities in the past years), whether they took an extra final exam topic and whether they reported interest in the honors programs available in the psychology curriculum. The raw score was a seven-point scale to which grade goal contributed up to four points. Multiplying the raw score with 1.43 resulted in a grade on a ten-point scale allowing more clear-cut comparisons and interpretations, since SSGPA and ACS were both graded on a ten-point scale as well, yet NCS still had a lower resolution. ACS is the average grade for the multiple-choice exam and the essay with which applicants concluded the matching program.
The predictive validity of SSGPA, ACS, and NCS was, as expected, confirmed by a comparison of Signal Detection analyses and correlational analyses in a previous study (van Ooijen-van der Linden et al., 2017) and the regression analyses matching the Signal Detection analyses for the data in this study are presented in Online Resource 1. A summary of the data collection and Signal Detection approach used in this study, a specification of b and d in Figure 1 for this study, is depicted in Figure 2.
In the current paper, only data of applicants with complete data—a fully completed application form and full participation in the matching program—were included in the analyses to be able to make an unconfounded comparison of the capacity of admission tools to uniquely select individual cases.
For each student who fully participated in the matching program, her/his score on SSGPA, ACS, and NCS was compared with all relevant criteria; grades 6–8 in steps of 0.1 for SSGPA, grades 4–8 in steps of 0.1 for ACS, and 2.9, 4.3, 5.7, 7.2, and 8.6 for NCS. For each student, his/her unweighted mean of SSGPA, ACS, and NCS (SSGPA/ACS/NCS) was also compared with grades/criteria 4–8 in steps of 0.1. The resulting hit-rates and false alarm-rates were plotted in a Receiver Operating Characteristic curve (ROC-curve) visualizing each tool's accuracy (see Figure 3). The area under the curve (AUC) was calculated as a numerical measure of accuracy for each admission tool, ranging from 0.5, chance level, to 1, perfect accuracy. The AUC (instead of, for example, d′) was chosen because this model best suits our data: ACS and NCS are not normally distributed and our data allowed full, empirical ROC-curves. Note that accuracy expressed as AUC can have identical values for different admission tools, but these might result from a different pattern of hit-rates and false alarm-rates across criteria. In addition, a certain combination of hit-rate and false alarm-rate can occur at one criterion for one tool and at another criterion for a different tool.
Figure 3. The Receiver Operating Characteristic curve (ROC-curve) visualizes the accuracy of a predictor of academic success. The area under the curve (AUC), ranging from 0.5 to 1, quantifies the accuracy. Each applicant's score on the predictor is compared to each possible criterion, in this case grades 4–8 in steps of 0.1 for SSGPA/ACS/NCS. Each criterion yields a false alarm rate and a hit rate for the sample of students, a data point in de the ROC-curve.
Logistic regressions and odds ratios based on the Signal Detection outcomes are reported in Online Resource 1 to allow comparison of the Signal Detection outcomes with more commonly reported outcomes. By design, regression analyses provide information on which predictors explain variance in what degree, but they do not provide information on cut-off scores. Individual differences in the predictive validity of different predictors results in low explained variance and its source can only be found if a relevant covariate is tested and found to be significant. Signal Detection Theory allows the combination of a more person-centered approach of investigating differences in predictive validity with a more instrument-centered approach. Signal Detection Theory allows detection of individual differences in the predictive validity of specific predictors without first choosing and testing specific possible covariates (Kiernan et al., 2001), and also provides information on the sensitivity and specificity of instruments and combinations of instruments at group level.
The primary goal of the present study is to determine whether different tools uniquely select (successful or unsuccessful) students. The three single admission tools were combined to form a set of seven admission tools and a Signal Detection approach was used to determine whether students had scored at or above criterion on which, or none, of these seven tools. For all individuals in the group of successful students and in the group of unsuccessful students it could thus be determined which of the seven tools would have selected them or whether they would not have been selected by any of these tools. The set of seven consists of the three single tools, three “double” tools and a seventh tool called “SSGPA/ACS/NCS.” The single tools are SSGPA-only, ACS-only, and NCS-only. The double tools are SSGPA-and-ACS-but-not-NCS, SSGPA-and-NCS-but-not-ACS and ACS-and-NCS-but-not-SSGPA. The seventh tool determined whether students scored at or above criterion on all three single predictors (SSGPA and ACS and NCS). A previous analysis showed that tools constructed by weighing SSGPA, ACS, and NCS differently in a total score did not outperform the unweighted average of SSGPA, ACS, and NCS (SSGPA/ACS/NCS) (van Ooijen-van der Linden et al., 2017, see also Wainer, 1976).
Given the proportions of successful and unsuccessful students in a cohort and carrying out a hypothetical, retrospective selection, a set of admission tools can be compared on the proportions of unique hits and unique false alarms each tool within the set yields. A student is a unique hit, within the set, if she or he would have been selected by only that tool and not the others within the set and turns out to be a successful student. Successful students who were hypothetically rejected by all seven tools are deemed “Total Misses.” Unsuccessful students who were rejected by all seven tools are deemed “Total Correct Rejections.” In other words, the Signal Detection approach was used to determine which of seven tools would have selected an individual applicant or whether the applicant would have been rejected. For the successful students, this resulted in proportions of students selected by each of seven tools (“Hits”) and a proportion of unselected students (“Total Misses”), totaling 1. For the unsuccessful students, this resulted in proportions of students selected by each of seven tools (“False alarms”) and a proportion of unselected students (“Total Correct Rejections”), totaling 1. Two criteria were compared: a medium score criterion (score ≥6.5 out of 10) and a high(er) criterion (score ≥7 out of 10).
The means and standard deviations of SSGPA, ACS, and NCS of the students that fully participated in the matching program are given in Table 1.
Table 1. Means and standard deviations of Secondary School Grade Point Average (SSGPA), Admission test Cognitive Score (ACS), Non-Cognitive Score (NCS) for each cohort.
Given the uniformity of the ROC-curves in Figure 4 and the comparable AUCs for cohort 2014–2015 and 2015–2016, SSGPA and ACS appear equally accurate in selecting students. NCS was more accurate than ACS in 2013–2014, but whereas ACS appeared to stabilize in the next two years, NCS's accuracy dropped. See Tables S3–S5 for the logistic regressions and Table S6 for the odds rations based on the Signal Detection outcomes, if the reader wishes to compare the two analyses.
Figure 4. Receiver Operating Characteristic Curves (ROC-curves) for three consecutive cohorts for Secondary School Grade Point Average (SSGPA), Admission test Cognitive Score (ACS), Non-Cognitive Score (NCS), and the unweighted mean of SSGPA, ACS, and NCS (SSGPA/ACS/NCS). The diagonal from the upper left corner to the middle of each ROC-space represents a neutral criterion and thus equal proportions of misses and false alarms. The solid arrows point to the enlarged data point for each cohort corresponding to criterion ≥6.5 and the dashed arrows point to the enlarged data point for each cohort corresponding to criterion ≥7.
If we had actually applied SSGPA ≥6.5 to select applicants, we would have admitted 80% of the applicants in 2013, 77% in 2014 and 74% in 2015. Criterion ≥7 for SSGPA would have resulted in the admission of 43% of the applicants in 2013, 41% in 2014 and 40% in 2015. If we had actually applied SSGPA/ACS/NCS ≥6.5 it would have resulted in 42, 53, and 34% admissions for the three consecutive cohorts. Application of criterion ≥7 to SSGPA/ACS/NCS would have admitted 24, 24, and 17% of the applicants. The hit-rates and false alarm-rates of SSGPA for criterion ≥6.5 and criterion ≥7—and thus the sensitivity (hit-rate) and specificity (1—false alarm-rate), see the enlarged data points in Figure 4—were more similar across cohorts for SSGPA than for ACS, NCS, and SSGPA/ACS/NCS. A comparison of the upper left (SSGPA) and lower right (SSGPA/ACS/NCS) panel in Figure 4 shows that for SSGPA criteria ≥6.5 to ≥7 covered the whole middle range of the ROC-curve whereas for SSGPA/ACS/NCS they only covered the lower, left part of the ROC-curve, where all criteria indicate a bias to reject applicants. The upper, right part of the ROC-curve of SSGPA/ACS/NCS, is occupied by criteria ≤6 for 2013–2014 and 2015–2016 and criteria ≤6.5 for 2014–2015. These criteria, right of the diagonal from the upper left corner to the middle of the ROC-space indicating a neutral criterion, all represent an increasing bias toward admitting more applicants as the criterion lowers (see also Figure 3). Whereas, in secondary education pupils can only enter the pre-exam year with sufficiently high grades for all topics (resulting in a narrowed range for SSGPA), in the matching program applicants can have low(er) scores for both ACS and NCS. Therefore, the range of possible scores is wider for ACS than for SSGPA and in our sample the distributions of SSGPA are clearly smaller than those of ACS (Table 1). If the distributions of the scores on SSGPA and ACS differ enough, a combined score will have an even wider range than ACS alone. As follows from a comparison of the ROC-curves for ACS (upper, right panel in Figure 4) and for SSGPA/ACS/NCS (lower, right panel in Figure 4), the admission decision space—the range of the cut-off scores from which to choose a specific criterion to match a certain desirable bias toward rejection or admission—indeed increases when these scores are combined. Also, note the relatively low percentages, 53% at most, of admitted applicants even with the lower criterion ≥6.5 for SSGPA/ACS/NCS; these appear comparable to the percentages admissions with SSGPA ≥7. Adding ACS and NCS to the admission toolbox, compared to SSGPA alone, clearly enlarges the decision space.
The Signal Detection approach allowed analyses of the proportions of students uniquely selected or rejected by each of the seven admission tools as presented in Figure 5 (see also van Ooijen-van der Linden et al., 2017). SSGPA-only (i.e., excluding applicants that scored at or above criterion for SSGPA and one of the other measures) selected a remarkably high percentage of unsuccessful students, especially with criterion ≥6.5, as is depicted by the gray bars at the left side of Figure 5. Note that the average SSGPA was 6.8 with a standard deviation of 0.5 in these cohorts. Figure 5 shows that all seven tools uniquely selected both successful and unsuccessful students, both with the criterion set at ≥6.5 and at ≥7. SSGPA/ACS/NCS (GAN, the purple bars in Figure 5) was the tool with the highest sensitivity—specificity ratio (and thus accuracy) for all three consecutive cohorts when criterion ≥6.5 was applied; for this criterion, as apparent from the hits-bars and false alarms-bars, the GAN-tool admitted the largest number of applicants in all three cohorts and this tool had the best ratio between the proportion hits and the proportion false alarms. With criterion ≥7 there were two exceptions to this pattern of SSGPS/ACS/NCS showing the highest accuracy: SSGPA-and-NCS in 2013–2014 (the lacking green bar of false alarms in Figure 5 for cohort 2013–2014 and criterion ≥7) and ACS-and-NCS in 2015–2016 (the lacking blue bar of false alarms for cohort 2015–2016 and criterion ≥7) yielded zero false alarms. Note that these exceptions occur with double and not single tools. In addition, for the applicants who were not selected by any of the tools (the white “Rejected” bars in Figure 5), the proportions of correct decisions for unsuccessful students, Total Correct Rejections (at the right end of the false alarms-bars), are remarkably larger than the proportions of incorrect decisions for successful students, Total Misses (at the right end of the hits-bars), across three cohorts. Note that removing a tool from the toolbox would render the hits of that tool misses, which would add to the number of Total Misses. The false alarms from the removed tool would become correct rejections and add to the number of Total Correct Rejections.
Figure 5. Proportions of unique hits and false alarms, Total Misses and Total Correct Rejections for a set of seven admission tools, with criteria ≥6.5 and ≥7 applied. For each applicant, we determined whether they scored at or above criterion on SSGPA-only, ACS-only, and NCS-only. These were the “single” tools. Another three “double” tools were constructed to determine whether students had scored at or above criterion on both-SSGPA-and-ACS-but-not-NCS, both-SSGPA-and-NCS-but-not-ACS and both-ACS-and-NCS-but-not-SSGPA. From the three single admission tools, we constructed “SSGPA/ACS/NCS” which determined whether students scored at or above criterion on all three single predictors (SSGPA and ACS and NCS) and not on only one or two of the three. “Hits” are successful students who would have been selected with the given tool and criterion and “false alarms” are unsuccessful students who would have been selected with the given tool and criterion. “Total Misses” are successful students who are not selected by any admission tool in this set with the given criterion and “Total Correct Rejections” are unsuccessful students who are not selected by any admission tool in this set with the given criterion. Each student contributes to only one proportion for each criterion (≥6.5 and ≥7) in their cohort: each student in each cohort, and for each of both criteria, is a hit or false alarm for only one of the seven tools OR a total miss or total correct rejection. The proportions of unique hits (of total successful students) and unique false alarms (of total unsuccessful students) were calculated for each of the tools in the set of seven tools as described above. These seven proportions of unique hits plus the Total Misses sum up to 1 (100% successful students). The seven proportions of unique false alarms plus the Total Correct Rejections sum up to 1 (100% unsuccessful students).
Applicants to the investigated program do not score particularly high on SSGPA and ACS, but the majority does successfully pass at least seven of the eight first year courses. By design, ACS is a snapshot measurement of academic achievement compared to SSGPA—ACS being based on one week of program-specific “studying for a week” and SSGPA on 5 years of secondary education. Nonetheless, their accuracy appears comparable and stable across cohorts. Such work samples in admission of psychology students have been found to be predictive of academic achievement in the program (Visser et al., 2012; Niessen et al., 2016). Psychology students admitted through an admission procedure including a cognitive admission tests and Multiple Mini Interviews have been shown to outperform psychology students admitted based on their SSGPA (Makransky et al., 2016). Although it is also consistent with most other available information, most studies investigating predictors of academic success other than SSGPA focus on medical programs, and those are currently undecided on the “best practices” in the implementation of admission tools other than SSGPA (Richardson et al., 2012; Schripsema et al., 2014, 2017; Shulruf and Shaw, 2015; Patterson et al., 2016; Pau et al., 2016; Sladek et al., 2016; Wouters et al., 2017). Note that NCS is quite susceptible to social desirability and that the literature does not allow firm conclusions on the validity of these and other non-cognitive factors (Patterson et al., 2016). In addition, in 2013–2014 participation in the matching program was optional, whereas in 2014–2015 and 2015–2016 it was obligatory and students were selected. This implicates the lesser accuracy of NCS in 2014 and 2015 might also be due to the combination of these characteristics of the admission procedure across cohorts and the instruments used (Niessen and Meijer, 2017; Niessen et al., 2017). However, the accuracy and stability of ACS across three cohorts allowed to take the interpretation one step further.
Although SSGPA/ACS/NCS had comparable accuracy to SSGPA alone, adding ACS and NCS did expand the decision space. Combining secondary school grades with admission test grades and non-cognitive scores increases the range in admission scores and thus the space to set the criterion to minimize bias toward one of the two type of mistakes that will inevitably be made, or to prefer admitting applicants who will fail over rejection applicants who will be successful or vice versa. A closer look at the distinct contribution of each tool to successful selection at the individual level revealed nuances that were not provided by the ROC-curves and that are not provided by approaches other than Signal Detection.
Given the differences in whether SSGPA, ACS, and NCS were used to determine whether students from the studied cohorts were being admitted or not—no selection in 2013, 80% selection in 2014 and 100% selection in 2015—differences between the cohorts were to be expected. It was already mentioned that due to the number of applicants not exceeding the numerus clausus all applicants were admitted, but the applicants were unaware of this at the time of application and during participating in the matching program. Yet, the pattern in the proportions of unique correct and incorrect decisions across three consecutive cohorts stably and clearly showed that an admission toolbox consisting of multiple tools outperformed single tools in both sensitivity and specificity, in this multiple cohort sample. In addition, the patterns in the proportions of both types of correct and incorrect decisions across cohorts (Figure 5) shows the imperfection of the instruments in more detail than the regression analyses do. Of course, instruments with relative low predictive validity can be useful to select-in applicants on grounds other than the expectation of retention and high grades (Wainer, 1976; Sedlacek, 2003; Schmitt, 2012; Sternberg et al., 2012). We showed that using multiple instruments can increase the proportion of correct decisions without having to make assumptions on how explained variance at group level translates to predictive validity at the individual level: each instrument, with both criteria and across three cohorts, selected individual applicants that would not have been selected by any of the other instruments and that turn out to be successful. Signal Detection offers information on the effects of specific criteria on both the proportion of incorrect decisions and the type of incorrect decisions that result from specific criteria applied to specific instruments. It does so at group level and the individual level. These details allow fine tuning of both the choice of instruments and of the applied criterion.
Data of previous cohorts can thus provide an evidence-based admission tool and criterion for a future cohort and each next cohort updates the available dataset. The chosen criterion for a future cohort can aim at equal proportions of false alarms and misses, at a rejection bias or at an admission bias. The relative desirability of one or another is to be determined by policy makers based on the costs and benefits of hits, misses, false alarms and correct rejections for that specific program. For institutions, the admission procedure resulting in the highest possible proportion of admitted successful students can be argued to be the best possible procedure. Yet, incorrect decisions are inevitable. From a societal viewpoint, the admission procedure resulting in the lowest proportion of rejected potentially successful students would contribute best to the development and use of human potential. To date, we have not encountered other methods that provide information on the proportions of both types of mistakes resulting from different tools and criteria, though methods complementary to regressions have been reported (e.g., Shulruf et al., 2018). In medicine, Signal Detection Theory is commonly used in investigating the accuracy of diagnostic tests. It not only matters how well the diagnostic instrument performs in detecting disease in the sick (sensitivity), but it also matters how well it performs in detecting people not having the disease as such (specificity). Likewise, in admission of students to university, the highest proportion correct decisions or the highest proportion of successful admitted students is only half the information. Signal Detection Theory also provides the other half.
Note that the matching program, the application of specific admission tools and criteria and the academic achievement of the selected students can interact. If the selection outcomes apparently do not sufficiently match the goals of the admission committee as students progress (or not) through their first, second and third year, the admission procedure will be adjusted. We argue that individual differences in which tool accurately predicts future academic success might be worth further investigation in the light of actual and desired levels of diversity in student populations (Stegers-Jager et al., 2015; Pau et al., 2016) in relation to both open admission and selection. It depends on the goals of the admission committee whether preventing rejection of applicants that would be successful students (preventing misses) is considered more important than preventing admission of students that will fail (preventing false alarms).
We did not compare the selection outcomes for different subgroups of students such as vocational pathway applicants or applicants who traveled for a year between secondary and tertiary school, in contrast with applicants entering directly after secondary school. Our focus was on a concise comparison of SSGPA, ACS, and NCS and combinations thereof in a relatively homogeneous group of applicants and a lack of power for the smaller subgroups would have prevented any firm conclusions. A sensible next step would be to investigate these and other possible individual differences in the predictive validity of instruments and personal factors or profiles (Wingate and Tomes, 2017). Comparisons of the predictive validity of program-specific admission test scores and program-specific non-cognitive admission test scores in different programs would also be valuable. Following the argument of homogeneous vs. heterogeneous student groups, for other programs and other types of students (e.g., O'Neill et al., 2013) program-specific admission tools might not improve selection and therefore we refrain from advocating the inclusion of specific admission tools or specific toolboxes. However, the recent focus on diversity in tertiary education (Haaristo et al., 2010; Reumer and Van der Wende, 2010; Kent and McCarthy, 2016) warrants the search for a more diverse admission toolbox. Adding tailor-made program-specific admission tools to SSGPA might better suit the goals of admission committees than the addition of standardized tests: they can be chosen to measure, or investigate, program-specific predictors of academic success (Kuncel and Hezlett, 2010). They also allow admission based on desirable characteristics other than general cognitive capacities and investigation of the effects thereof.
The results of the current study can be formulated as implications for selection committees and policy makers:
• The time and effort invested in organizing “studying for a week,” can be worth it; adding multiple cognitive and non-cognitive admission test scores to the admission toolbox prevents rejection of applicants that did not do very well in secondary education but will be successful students.
• The differential predictive validity of work samples and non-cognitive admission test scores for academic achievement in different programs is still work in progress.
• All seven selection tools in the current study were imperfect.
• The best-performing tool was the combination tool.
• Signal Detection Theory is useful in both short and long-term evaluation of admission and selection instruments and outcomes; findings on the effects of different tools and criteria on both group and individual level allow policy makers to adjust admission and selection instruments to meet their goals.
This study was carried out in accordance with the recommendations of The Netherlands Code of Conduct for Academic Practice and the Faculty Ethics Review Board of the Faculty of Social and Behavioral Sciences of Utrecht University with written informed consent from the students from cohorts 2014–2015 and 2015–2016. The data obtained from cohort 2013–2014 was entirely desk-based and the students whose data we used were not required to give consent. The protocol was approved by the Faculty Ethics Review Board of the Faculty of Social and Behavioral Sciences of Utrecht University stating that this research project does not belong under the regimen of the Dutch Act on Medical Research Involving Human Subjects and therefore there is no need for approval of a Medical Ethics Committee.
The data were anonymized by the first author before analysis. All analyses were run retrospectively, after having awaited the academic success of the participants. Since the data used from cohort 2013–2014 only consisted of (anonymized) administrative information (for which no informed consent is needed as per our institution's guidelines and national regulations), we did not retrospectively try to obtain such consent. As we added optional questionnaires to the standard matching program for cohorts 2014–2015 and 2015–2016 to strengthen our research (although these data were not used in the presented analyses), we asked applicants to give written and informed consent. For these cohorts we only used data of applicants who gave their written consent. Our project as a whole, including the use of the data of cohort 2013–2014, was approved by the Ethics Committee of the Faculty of Social and Behavioral Sciences of Utrecht University (FETC15-057) before commencement of this research.
LvO-v: prepared the data; LvO-v and MvdS: planned the data analysis; LvO-v: performed the data analysis; all authors contributed to the interpretation of the findings; LvO-v: wrote the first draft of the article; MvdS and StP: provided critical revisions. All authors read and approved the final manuscript for submission.
The authors declare that the research was conducted in the absence of any commercial or financial relationships that could be construed as a potential conflict of interest.
The Supplementary Material for this article can be found online at: https://www.frontiersin.org/articles/10.3389/feduc.2018.00030/full#supplementary-material
Cortes, C. M. (2013). Profile in action: linking admission and retention. New Dir. High. Educ. 161, 59–69. doi: 10.1002/he.20046
De Witte, K., and Cabus, S. J. (2013). Dropout prevention measures in the Netherlands, an explorative evaluation. Educ. Rev. 65, 155–176. doi: 10.1080/00131911.2011.648172
Edwards, D., Friedman, T., and Pearce, J. (2013). Same admissions tools, different outcomes: a critical perspective on predictive validity in three undergraduate medical schools. BMC Med. Educ. 13:173. doi: 10.1186/1472-6920-13-173
Green, D. M., and Swets, J. A. (1966). Signal Detection Theory and Psychophysics. New York, NY: Wiley.
Haaristo, H., Orr, D., and Little, B. (2010). Intelligence brief: is higher education in Europe socially inclusive? Eurostudent. Available online at: http://www.eurostudent.eu/download_files/documents/IB_HE_Access_120112.pdf
Kent, J. D., and McCarthy, M. T. (2016). Holistic Review in Graduate Admissions: A Report from the Council of Graduate Schools. Washington, DC: Council of Graduate Schools.
Kiernan, M., Kraemer, H. C., Winkleby, M. A., King, A. C., and Taylor, C. B. (2001). Do logistic regression and signal detection identify different subgroups at risk? Implications for the design of tailored interventions. Psychol. Methods 6, 35–48. doi: 10.1037//1082-989X.6.1.35
Kuncel, N. R., and Hezlett, S. A. (2010). Fact and fiction in cognitive ability testing for admissions and hiring decisions. Curr. Direct. Psychol. Sci. 19, 339–345. doi: 10.1177/0963721410389459
Macmillan, N. A., and Creelman, C. D. (2005). Detection Theory: A User's Guide (2nd edn.). Mahwah, NJ: Lawrence Erlbaum Associates.
Makransky, G., Havmose, P., Vang, M. L., Andersen, T. E., and Nielsen, T. (2016). The predictive validity of using admissions testing and multiple mini-interviews in undergraduate university admissions. High. Educ. Res. Dev. 36, 1003–1016. doi: 10.1080/07294360.2016.1263832
Niessen, A. S. M., and Meijer, R. R. (2017). On the use of broadened admission criteria in higher education. Perspect. Psychol. Sci. 12, 436–448. doi: 10.1177/1745691616683050
Niessen, A. S. M., Meijer, R. R., and Tendeiro, J. N. (2016). Predicting performance in higher education using proximal predictors. PLoS ONE 11:e0153663. doi: 10.1371/journal.pone.0153663
Niessen, A. S. M., Meijer, R. R., and Tendeiro, J. N. (2017). Measuring non-cognitive predictors in high-stakes contexts: the effect of self-presentation on self-report instruments used in admission to higher education. Pers. Individ. Diff. 106, 183–189. doi: 10.1016/j.paid.2016.11.014
O'Neill, L., Vonsild, M. C., Wallstedt, B., and Dornan, T. (2013). Admission criteria and diversity in medical school. Med. Educ. 47, 557–561. doi: 10.1111/medu.12140
Patterson, F., Knight, A., Dowell, J., Nicholson, S., Cousans, F., and Cleland, J. (2016). How effective are selection methods in medical education? A systematic review. Med. Educ. 50, 36–60. doi: 10.1111/medu.12817
Pau, A., Chen, Y. S., Lee, V. K. M., Sow, C. F., and De Alwis, R. (2016). What does the multiple mini interview have to offer over the panel interview? Med. Educ. 21:29874. doi: 10.3402/meo.v21.29874
Reumer, C., and Van der Wende, M. (2010). Excellence and Diversity: The Emergence of Selective Admission Policies in Dutch Higher Education - A Case Study on Amsterdam University College. Research & Occasional Paper Series.
Richardson, M., Abraham, C., and Bond, R. (2012). Psychological correlates of university students' academic performance: A systematic review and meta-analysis. Psychol. Bull. 138, 353–387. doi: 10.1037/a0026838
Robbins, S. B., Lauver, K., Le, H., Davis, D., Langley, R., and Carlstrom, A. (2004). Do psychosocial and study skill factors predict college outcomes? A meta-analysis. Psychol. Bull. 130, 261–288. doi: 10.1037/0033-2909.130.2.261
Schmitt, N. (2012). Development of rationale and measures of noncognitive college student potential. Educ. Psychol. 47, 18–29. doi: 10.1080/00461520.2011.610680
Schripsema, N. R., van Trigt, A. M., Borleffs, J. C. C., and Cohen-Schotanus, J. (2014). Selection and study performance: comparing three admission processes within one medical school. Med. Educ., 48, 1201–1210. doi: 10.1111/medu.12537
Schripsema, N. R., van Trigt, A. M., Borleffs, J. C. C., and Cohen-Schotanus, J. (2017). Impact of vocational interests, previous academic experience, gender and age on Situational Judgement Test performance. Adv. Health Sci. Educ. 22, 521–532. doi: 10.1007/s10459-016-9747-9
Sedlacek, W. E. (2003). Alternative admission and scholarship selection measures in higher education. Meas. Eval. Couns. Dev. 35, 263–272.
Shulruf, B., Bagg, W., Begun, M., Hay, M., Lichtwark, I., Turnock, A., et al. (2018). The efficacy of medical student selection tools in Australia and New Zealand. Med. J. Austr. 208, 214–218. doi: 10.5694/mja17.00400
Shulruf, B., and Shaw, J. (2015). How the admission criteria to a competitive-entry undergraduate programme could be improved. High. Educ. Res. Dev. 34, 397–410. doi: 10.1080/07294360.2014.956693
Sladek, R. M., Bond, M. J., Frost, L. K., and Prior, K. N. (2016). Predicting success in medical school: a longitudinal study of common Australian student selection tools. BMC Med. Educ. 16:3. doi: 10.1186/s12909-016-0692-3
Stanislaw, H., and Todorov, N. (1999). Calculation of signal detection theory measures. Behav. Res. Methods. Instrum. Comput. 31, 137–149. doi: 10.3758/BF03207704
Stegers-Jager, K. M. (2017). Lessons learned from 15 years of non-grades-based selection for medical school. Med. Educ. 52, 86–95. doi: 10.1111/medu.13462
Stegers-Jager, K. M., Themmen, A. P. N., Cohen-Schotanus, J., and Steyerberg, E. W. (2015). Predicting performance: relative importance of students? Background and past performance. Med. Educ. 49, 933–945. doi: 10.1111/medu.12779
Steenman, S. C., Bakker, W. E., and van Tartwijk, J. W. F. (2016). Predicting different grades in different ways for selective admission: disentangling the first-year grade point average. Stud. High. Educ. 41, 1408–1423. doi: 10.1080/03075079.2014.970631
Sternberg, R. J., Bonney, C. R., Gabora, L., and Merrifield, M. (2012). WICS: a model for college and university admissions. Educ. Psychol. 47, 30–41. doi: 10.1080/00461520.2011.638882
Urlings-Strop, L. C., Themmen, A. P. N., and Stegers-Jager, K. M. (2017). The relationship between extracurricular activities assessed during selection and during medical school and performance. Adv. Health Sci. Educ. 22, 287–298. doi: 10.1007/s10459-016-9729-y
van Ooijen-van der Linden, L., van der Smagt, M. J., Woertman, L., and te Pas, S. F. (2017). Signal detection theory as a tool for successful student selection. Assess. Eval. High. Educ. 42, 1193–1207. doi: 10.1080/02602938.2016.1241860
Visser, K., Van der Maas, H., Engels-Freeke, M., and Vorst, H. (2012). Het effect op studiesucces van decentrale selectie middels proefstuderen aan de poort. Tijdschrift Voor Hoger Onderwijs, 30, 161–173.
Wainer, H. (1976). Estimating coefficients in linear models: it don't make no nevermind. Psychol. Bull. 83, 213–217. doi: 10.1037/0033-2909.83.2.213
Wingate, T. G., and Tomes, J. L. (2017). Who's getting the grades and who's keeping them? A person-centered approach to academic performance and performance variability. Learn. Individ. Diff. 56, 175–182. doi: 10.1016/j.lindif.2017.02.007
Wouters, A., Croiset, G., Schripsema, N. R., Cohen-Schotanus, J., Spaai, G. W. G., Hulsman, R. L., et al. (2017). A multi-site study on medical school selection, performance, motivation and engagement. Adv. Health Sci. Educ. 22, 447–462. doi: 10.1007/s10459-016-9745-y
Keywords: signal detection, admission, selection, university, academic success, grade point average, unique
Citation: van Ooijen-van der Linden L, van der Smagt MJ, te Pas SF and Woertman L (2018) A Signal Detection Approach in a Multiple Cohort Study: Different Admission Tools Uniquely Select Different Successful Students. Front. Educ. 3:30. doi: 10.3389/feduc.2018.00030
Received: 29 January 2018; Accepted: 16 April 2018;
Published: 01 May 2018.
Edited by:
Bernard Veldkamp, University of Twente, NetherlandsReviewed by:
Giray Berberoglu, Başkent University, TurkeyCopyright © 2018 van Ooijen-van der Linden, van der Smagt, te Pas and Woertman. This is an open-access article distributed under the terms of the Creative Commons Attribution License (CC BY). The use, distribution or reproduction in other forums is permitted, provided the original author(s) and the copyright owner are credited and that the original publication in this journal is cited, in accordance with accepted academic practice. No use, distribution or reproduction is permitted which does not comply with these terms.
*Correspondence: Linda van Ooijen-van der Linden, TC52YW5Pb2lqZW5AdXUubmw=
Disclaimer: All claims expressed in this article are solely those of the authors and do not necessarily represent those of their affiliated organizations, or those of the publisher, the editors and the reviewers. Any product that may be evaluated in this article or claim that may be made by its manufacturer is not guaranteed or endorsed by the publisher.
Research integrity at Frontiers
Learn more about the work of our research integrity team to safeguard the quality of each article we publish.