- 1Department of Health Sciences, University of Burgos, Burgos, Spain
- 2Department of Civil Engineering, University of Burgos, Burgos, Spain
Nowadays in higher education, the teaching procedures that lead to better learning outcomes (LO) require continuous analysis. Rubrics such as teaching feedback procedures have demonstrated their effectiveness at heightening the reflection of university students on their own practice. The aims were (1) to study the effects of different types of rubric-based feedback [from process-oriented feedback to traditional feedback] on LO and on student perceptions of subject matter knowledge (SPKI) after training in a comparison between students in engineering and students in the social sciences; (2) to analyze the relation between metacognitive skills and motivation with LO and SPKI; and (3) to validate the results with structural equation modeling (SEM). The study comprised a total of 171 university students (n = 80 from the mechanical engineering degree and n = 91 from social sciences degree). A quasi-experimental design with a control group was used to test aim 1 and a descriptive correlational design to test aim 2. SEM was applied to validate the results (aim 3). Significant differences were found between both types of rubric-based feedback (process-oriented v. traditional) in relation to LO, though not in relation to SPKI. The effects of the degree type were noted in LO but no in SPKI. Likewise, a relation between SPKI and motivation skills was found in engineering students. Accordingly, the type of degree and the characteristics of the subject modules appear to be determining factors in successful learning, while SPKI is directly linked to motivation skills. The SEM results validated these conclusions.
Highlights
– The use of rubrics as evaluation instruments and orientative feedback facilitates learning motivation.
– Rubrics mean students can analyze and correct errors.
– Feedback prepared on task completion facilitates the development of self-assessment processes in the student.
– Self-assessment helps the student to situate the learning goals, to establish planning for their achievement, and to adjust both problem-solving tasks and acceptable evaluation of their learning responses.
– Rubrics are excellent tools to orient learning goals and to carry out good planning in the problem-solving stages.
– Success in the learning responses is related to the type of task, understanding of the learning goals, and the effectiveness of the support provided by the teacher during the instruction process.
Introduction
At present, one of the challenges of higher education is how to improve both learning processes and student performance. There are procedures in cognitive psychology that have been studied to facilitate the development of learning. From the standpoint of that discipline, however, the multiple variables that interact in the teaching–learning process must be considered. Some of those variables are related to the teacher, others with learning and others with the task, and they all interact through reciprocal determinism. Therefore, the analysis of the teaching–learning process must be multi-dimensional. The teacher-specific variables relate to the style of teaching in the instruction process. With regard to learning, influential variables include previous knowledge of the task to be learnt, task-related motivation, and learning skills among which metacognitive skills stand out (Flavell, 1981; Efklides, 2011; Van der Stel and Veenman, 2014; Sáiz and Montero, 2015).
Feedback and Instruction
Recent research has placed special emphasis on the importance of feedback from the teacher during instruction in successful learning outcomes. Feedback may be defined as information that the teacher gives to the learner on the task or problem and its solution (Hattie and Timperley, 2007; Harks et al., 2014). Different types of feedback may be identified: (1) process-oriented feedback that combines motivational, cognitive, and metacognitive characteristics and (2) grade-oriented feedback or information that the teacher provides on the task or the learning process, which is associated with traditional feedback. The difference is that the first places emphasis on error correction, motivation for change, and the analysis of metacognitive consequences. So, feedback must, therefore, be prepared and must aim to go beyond traditional instruction. Its development of motivation must be supported through the introduction of regulatory metacognitive processes (Hattie and Timperley, 2007; Alonso-Tapia et al., 2014). The minds of teachers must to be focused on learning processes, in which the student employs the correct skills and through which the teacher can reorient those skills that are not correct in an independent way (Kim, 2015; Wilkins et al., 2015). Moreover, the teacher must to consider the diversity of learners and the degree of their previous knowledge and their competences in relation to the learning task that is associated with the learning object (Daly et al., 2014; Sáiz and Montero, 2015). If well-structured, feedback will help increase student motivation and will provide responses to the following questions (Hattie, 2013):
• Where do I go?
• How I go?
• Where to next?
Hattie (2013) outlined another difference between different level of the information of feedback: (1) Feedback at task level: it provides information on the correct response. This level is important for novice learners, as it implies a relation between the type of task and the learning skills needed to solve it (task performance); (2) Feedback at process level, this process is related to learners’ self-efficacy in their own learning and refers to processes of understanding; (3) Feedback at self-regulation level: it refers both to the regulatory metacognitive process and to self-regulation. This is the highest level of feedback. In all, the first level is related to grade-oriented feedback, components of product-related orientation, which offer information on a specific domain, on a concrete task, and the second and the third with process-oriented feedback, components of process-related orientation. These approaches provide responses to questions of when, how, and why, through processes of self-regulation based on the use of appropriate skills (Hattie and Timperley, 2007; Mentzer et al., 2015; Panadero, 2017).
Likewise, process-oriented feedback includes two important sub-processes: (1) knowledge of mistakes, which means informing students of the weak points in their learning and (2) knowledge of how to continue, which includes problem-solving skills.
In summary, well-structured feedback can reduce the distance between what students know of their own learning in a specific field, in the “here-and-now,” and the goals of that learning (Molenaar and Chiu, 2014; Malmberg et al., 2015).
How Feedback Might Impact Students Thinking and Learning?
According to Narciss (2008), feedback with a process-oriented feedback provides reinforcement for the learner, facilitates problem-solving, and increases levels of learning-related motivation. Teachers must, therefore, provide instruction that facilitates information on the learning processes of their students in relation to the acquisition of competences. The teacher must, therefore, design the instruction carefully, paying attention to the following points (Hattie, 2013; Narciss, 2013):
1. Provide clear explanations to students on what they are expected to learn, as well as specifying and defining the competences that form the learning object.
2. Give precise criteria to students on what is understood by successful learning.
3. Know the beliefs that their students hold with regard to the learning goals.
4. Guarantee teaching that seeks to reduce the distance between what the students know and understand and what they believe and understand with regard to the knowledge to be learnt.
5. Guarantee feedback in steps directed at the reduction of that distance.
Feedback prepared on the completion of a task facilitates the development of self-assessment processes among students, helps them to monitor their progress, and promotes greater awareness with regard to their learning processes. This supervision orients learners toward more precise self-assessment of their knowledge and will improve their estimates of their learning capabilities (process and result) (Butler and Winne, 1995; Brown and Harris, 2013; Dinsmore and Parkinson, 2013).
Feedback is, therefore, a powerful tool for the development of successful results. Hattie and Gan (2011) reviewed 12 meta-analysis studies of feedback, which in turn analyzed 196 studies and 6,972 effect values. The learning outcomes indicated that the effect value was very high (explained variance d = 0.79), although the most successful feedback took the following into account:
1. The relation between the individual learning characteristics of the learners and the feedback skills that they employed.
2. The cognitive and the metacognitive skills of learners and their motivations.
3. Feedback frequency and duration.
The ultimate aim is to facilitate self-regulatory processes in learners of their own learning, which will lead to greater independence in the development of their knowledge and in their problem-solving processes (Panadero et al., 2013). The guiding principle behind this training of university students is to pass on tools that are, first of all, jointly employed with the assistance of the teacher. Progressively, these tools become linked to learner independence in their management and increase motivation levels in both teacher and learner alike (Zimmerman and Schunk, 2011). In this process, the skills of self-assessment and monitoring are essential for successful learning. Monitoring and self-assessment reveal the capability of students to assess their own actions. If these skills are accompanied by planning, effective learning outcomes are obtained. It is important that the student differentiate between what is correct and what needs to be improved. The teacher must, therefore, help the student to use appropriate skills that will facilitate independent self-assessment processes (Panadero and Romero, 2014). Self-assessment helps the students to fix learning goals, to plan their achievements, to adjust their problem-solving processes, and to evaluate their learning responses in acceptable ways. In this process, it is important to provide guidelines, criteria, and suitable evaluation templates, which will help the student to evaluate both learning process and product. In this sense, rubrics are one of the most appropriate tools to assist self-assessment (Panadero et al., 2012; Panadero and Jonsson, 2013; Menéndez-Varela and Gregori-Giralt, 2016). They are, in conclusion, excellent tools to orient learning goals and to follow correct planning at problem-solving stages (Sáiz et al., 2016; Watson et al., 2016). These rubrics help processes of instruction by clarifying how to complete a task and to specify the evaluation criteria at different qualitative levels of achievement (Panadero et al., 2013). In the same way, their use reduces the stress of individual learners in relation to the learning object, clarifies understanding of the assessment criteria, regulates the activation of learning skills, and orients them toward learning-based goals, reducing student drop-out rates (Sáiz and Román, 2011; Montfort et al., 2015).
In summary, self-regulation of learning (SRL) related behavior is conditioned by present and past cognitive, metacognitive, and motivational variables (the learning history of the individual learner) (Garzón Umerenkova et al., 2017). Both positive and negative experiences have conditioned the construction of previous knowledge and the attributions that individual learners may have with regard to their learning capabilities. These attributions are moreover subordinate to the learning object, in other words the type of task. All these variables will influence the proper development or the abandonment of the problem-solving tasks. So, it may be concluded that SRL is a complex process that is determined by the previously described variables: learning history, the perception of self-efficacy for problem solving, the type of tasks (intrinsic and extrinsic), motivation toward the task to be learnt, previous knowledge of the discipline, and the metacognitive skills related to problem-solving (planning, self-assessment, self-reflection) (Wäschle et al., 2014). These variables are proper to the individual learner but they also interact with other external variables that refer to the type of task (Finelli et al., 2015). Among which we may highlight: the form in which the task is presented, follow-up of student actions by the teacher, and feedback provided by the teacher on the actions of the student and on self-assessment processes (Sáiz and Bol, 2014; Schaffer et al., 2017). Hence, it is essential that self-assessment processes be oriented through the use of instruments that permit the student to clarify the following points (Sáiz and Bol, 2015; Sáiz et al., 2015):
1. The task.
2. The goals that the task requires.
3. The sequential evaluation criteria of the task.
4. Assistance for error-based determination of possible paths of change or re-direction that lead to successful task completion.
Recent research with rubric-based feedback has multiplied over the past few years in the context of “learning engineering.” Diefes-dux et al. (2012) found that formative feedback reduced the gap and that the students interpreted and responded to various forms of feedback (Narciss, 2008, 2013). Mavinkurve and Murthy (2014) asserted that rubrics prompt students to perform formative assessment of their own and correct themselves if necessary, thereby improving their performance in a new design problem.
Moreover, a further important variable is the ability of the instructor to interpret student work (Menekse et al., 2013). Likewise, recent studies have found that feedback improves metacognitive skills (Pate et al., 2015). Moreno et al. (2009) reported better results when the teacher monitored the problem-solving step-by-step and gradually increased the number of steps. Another important variable was also the level of the student’s prior knowledge of the subject.
Traditionally, performance on engineering degrees is lower than on social science degrees. Over the past decade, there has, therefore, been a lot of research on the differences between students following engineering degrees and other degree courses. For example, students following engineering courses are expected to exercise critical thinking in a different way to students studying degrees in the social sciences (Bumbaco and Douglas, 2015; Van Meter et al., 2016). Other research has found that students studying the social sciences used more self-knowledge and planning skills and less achievement motivation skills than engineering students (Sáiz et al., 2012). These results can be explained in terms of curricula content (Montfort et al., 2015) and the data may be related back to the results of earlier investigations, which suggest that students use a type of learning strategy depending on the type of degree and the academic year of the course (Sáiz et al., 2012). However, research into engineering education has shown that metacognitive instruction and the use of rubrics improve student performance (Narayanan, 2013; Ramírez-Corona et al., 2013; Mavinkurve and Murthy, 2014; Pate et al., 2015). Monitoring of problem-solving and self-regulation also facilitates student learning (Oenardi and Santoso, 2012). Another important aspect is the relation between prior knowledge and the problem-solving processes (Grohs et al., 2015).
The key may be found in the design of an innovative curriculum based on critical thinking in relation to problem-solving processes and can be achieved through the use of rubrics and self-regulated instruction (Parmar, 2014).
In view of the theoretical assumptions and investigations referred to above, the research questions of this work were as follows:
RQ1: “Will the students who receive process-oriented feedback have better LO than the students who receive grade-oriented feedback (traditional feedback) and could those LO be affected by the type of degree (engineering degree v. social science degree)?”
RQ2: “Will the students who receive process-oriented feedback have a better perception of their subject-related knowledge than the students who receive grade-oriented feedback (traditional feedback) following the intervention and will those results be affected by the type of degree (engineering degree v. social science degree)?”
RQ3: “Will there be a significant relation between LO and metacognitive skills and will there be differences in relation to the type of degree (engineering degree v. social science degree)?”
RQ4: “Will there be a significant relation between LO and motivation and will there be differences in relation to the type of degree (engineering degree v. social science degree)?”
RQ5: “Will there be a significant relation between SPKI and metacognitive skills and will there be differences in relation to the type of degree (engineering degree v. social science degree)?”
RQ6: “Will there be a significant relation between SPKI and motivation and will there be differences in relation to the type of degree (engineering degree v. social science degree)?”
RQ7: “Will the results be validated with the structural equation model?”
Materials and Methods
Participants
The students’ informed commitment was obtained and their participation was voluntary. The sample consisted of a total of 171 students from the University of North of Spain following three study modules from social sciences and mechanical engineering degree (MED) courses:
(1) Group 1:80 students following the subject-module on material elasticity and strength (MATES) in the first semester of the second year of the MED. 40 individuals in the experimental group (36 men Meanage = 20.49, SD = 2.47 and 4 women Meanage = 20.50, SD = 1.85) and 40 in the control group (32 men Meanage = 20.10 SD = 2.30 and 8 women Meanage = 20.30, SD = 1.53).
(2) Group 2:91 students following the subject-module on systematic observation in educational contexts (SOEC) in the first semester of the second year of the preschool education degree (PED), 58 in the experimental group (56 women Meanage = 20.20, SD = 2.19 and 2 men Meanage = 20.00, SD = 1.41) and 33 in the control group (31 women Meanage = 20.39, SD = 2.30 and 2 men Meanage = 20.50, SD = 1.14).
With a view to controlling the variable “type of teacher,” work on the three degree courses involved three teachers (Meanage rank 38–49 years old) who were members of teaching innovation groups, with ample experience in research (10 years) on innovative programs in university teaching. The three teachers had also received very good evaluations of their teaching activity on the teaching evaluation program.
Design
The assignment of students to either the experimental or the control groups was done through random assignment on the possible groups of intervention. The existence of significant differences between the groups was studied to establish whether they shared similar characteristics in the type of learning skills that they used before the intervention. Where differences were found, the variable “learning skills” would be used as a covariable in the analysis. A quasi-experimental design coupled with a control group was used to test RQ1 and RQ2, a descriptive correlational design to test RQ3, RQ4, RQ5, and RQ6, and the structural equation modeling (SEM) to RQ7 was employed to validate the results.
Instruments
Instruments for the evaluation of the dependent variables.
The ACRA Scale of Learning Skills
The ACRA Scale of learning skills (ACRAr) was developed by Román and Poggioli (2013). This scale is a widely tested research instrument on learning skills among Spanish-speaking populations (Camarero et al., 2000; Carbonero et al., 2013), which identifies 32 skills at different points in the processing of information: Information Acquisition Skills Scale (IASS) (attention and review skills) has an α = 0.78 and an inter-judge construct validity of r = 0.78 and a content validity of r = 0.85; Information Codification Skills Scale (ICSS) (mnemonic techniques, organization, and preparation) has an α = 0.92 and an inter-judge construct validity of r = 0.86 and a content validity of r = 0.87; Information Recovery Skills Scale (IRSS) answer planning and generation has an α = 0.83 and an inter-judge construct validity of r = 0.86 and a content validity of r = 0.86; Metacognitive and Information Processing Skills Scale (MIPSS) divided into Metacognition (self-knowledge, self-planning and regulation, and self-assessment) has an α = 0.90 and an inter-judge construct validity of r = 0.88 and a content validity of r = 0.88; and Processing skills (self-instructions, self-control, coping with distractions, social interaction, intrinsic–extrinsic motivation, and escape motivation) have an α = 0.90 and an inter-judge construct validity of r = 0.88 and a content validity of r = 0.88. In this study, the indicators of validity in the sample were IASS α = 0.78; ICSS α = 0.92; IRSS α = 0.83; MIPSS.
The reliability indices of the sample were, respectively, IASS α = 81,: ICSS α = 78, IRSS e α = 0.75, and MIPSS α = 0.77.
Rubrics
(a) Rubric for the MATES subject. This instrument has five evaluation criteria related to the competencies that the students should develop on the module: (1) identification of isostatic and hyper-static structures (α = 0.91); (2) solving problems related to structural cuts for the calculation of internal stress distributions (α = 0.87); (3) representation of internal stress diagrams (α = 0.86); (4) analysis of structural bending moments and shear stress diagrams (α = 0.87); and (5) interpretation of structural bending moments and shear stress diagrams (α = 0.87). These criteria were evaluated on a Likert-type scale of 1–5 points ranging from (1) unacceptable to (5) excellent. This instrument has a global reliability index of α = 0.91 and inter-judge indicators of construct validity of r = 0.89 and of content validity of r = 0.87. Likewise, it presented adjusted indicators of composite reliability (CR = 0.82) and mean variance extracted (AVE = 0.50) (see Appendix 1).
(b) Rubric for the SOEC module (Sáiz and Escolar, 2013). A rubric with five evaluation criteria was employed, related to the competences that students should develop on the module: (1) identification of the observation process (α = 0.86); (2) observational methods (α = 0.82); (3) application of descriptive statistics (α = 0.83); (4) application of pre-experimental designs (α = 0.81); and (5) application of quasi-experimental designs (α = 0.83). These criteria were evaluated on a Likert-type scale of 1–5 points from unacceptable to excellent. The instrument had a global reliability index of α = 0.85 and indicators of inter-judge construct validity of r = 0.86 and of content validity of r = 0.85. Likewise, it presented adjusted indicators of composite reliability (CR = 0.93) and of mean variance extracted (AVE = 0.66).
The two rubrics were prepared following the taxonomic criteria of Bloom (Anderson and Krathwohl, 2001) under the supervision of an expert researcher in didactic programs.
(c) The academic results of the MATES and SOEC subjects were analyzed to establish the degree of acquisition of the competences of each subject. The grades were measured on a scale of 0–10.
Procedure
At the start of the semester, the students were requested to participate in the research on a voluntary basis and were informed of the policy on data confidentiality. None of the students refused to participate in the study. The choice of modules was done by using incidental sampling. The distribution of the students between the experimental and the control groups within each of them was done by using random assignment.
Then, all the students were given the ACRAr scales and the rubric for each of the subjects, in the second week of the semester, which served to analyze the previous knowledge of each of the matters before beginning the instruction.
Third, throughout the course of the semester, different types of feedback were given, depending on whether the students had been assigned to the experimental or to the control group. Process-oriented feedback was applied to the first group. This type of feedback takes into account the motivational and metacognitive characteristics of students and places emphasis on error correction and motivation using self-regulatory skills. Grade-oriented feedback (traditional feedback) was applied to the second group, in which the student was given information on task completion, although the feedback contained no analysis of motivation and metacognitive skills. In this period, the teachers encouraged students to use the rubrics as a means of control and analysis of their learning process. The rubrics were progressively revised by the teacher, with a view to giving different types of feedback.
Fourth, the rubric was applied to all the students at the end of the semester and before completion of the final evaluation test, with a view to study the perceptions held by the students of their knowledge of the subject matter they were studying, immediately after which they completed the final evaluation test.
Data Analysis
In the first place, the normality and the linearity of the relations between the previously assigned dependent variables (ACRAr) were established. In second place, it was established whether the three situations were equal in relation to the tasks of orientation and previous experience.
Two multivariate ANCOVAs were performed with the independent variables LO and SPKI and the covariables Type of Degree (MED v. PED) RQ1 and RQ2. A Pearson correlation coefficient was employed to test RQ3, RQ4, RQ5, and RQ6, and the SEM to test RQ7. The analyses were done with the SPSS v.23 and the AMOS v.23 software packages.
Preliminary Analyses
Analysis of Correlations between the Variables
A correlation analysis was performed to study the relations between the learning skills, the SPKI, and the LO of the students. As may be seen in Table 1, significant correlations were detected between the scores on the four ACRAr scales. The rest of the correlations were not significant. Moreover, a negative significant correlation was found between LO and SPKI before (r = –0.48). Hence, the two variables LO and SPKI may be considered dependent variables in the ANCOVAs.
Previous Analysis of Samples
A normality test of the scores of each participant in the (ACRAr) learning skills was conducted before the intervention. The results indicated that no significant differences existed between the experimental group and the control group before the intervention on the four scales (IASS: F = 1.16, p = 0.20, η2 = 0.01; ICSS: F = 1.44, p = 0.23, η2 = 0.01; IRSS: F = 1.10, p = 0.29, η2 = 0.008; and MIPSS: F = 2.50, p = 0.11, η2 = 0.01). However, significant differences were found in relation to the variable “type of degree” (IASS: F = 19.41, p = 0.00, η2 = 0.12 and SPKI before: F = 295.23, p = 0.00, η2 = 0.67), so this variable will be used as a covariable in the ANCOVAs.
The indicators of asymmetry and kurtosis were also found for the distributions of ACRAr, LO and SPKI. Values higher than |2.00| for asymmetry indicate extreme asymmetry and values lower than the distribution are normal (Bandalos and Finney, 2001). Kurtosis values of between |8.00| and |20.00| suggest extreme kurtosis (Arias, 2008; Arias et al., 2013). Asymmetry values within an interval of between |0.17| and |1.09| kurtosis values of between |0.37| and |1.15| were found, which suggests that there was no acute deviation of normality in the distributions (see Table 2).
The Mahalanobis distance was also found, yielding no values lower than p > 0.001, making it unnecessary to eliminate any extreme values.
Results
Feedback’ Effects
The effect of feedback on LO and on SPKI was studied. In relation to Research RQ1 [“Will the students who receive process-oriented feedback have better LO than the students who receive grade-oriented feedback (traditional feedback) and could those LO be affected by the type of degree (engineering degree v. social science degree)?”]. Significant differences were found in response to the independent variable Type of feedback (F = 6.49, p = 0.01,η2 = 0.04). Moreover, significant differences were found in relation to the covariable “type of degree” with a low effect value (F = 35.61, p = 0.00,η2 = 0.19). In Table 3 and Figure 1, the mean values of LO in relation to the two sorts of feedback (process-oriented v. traditional feedback) are shown.
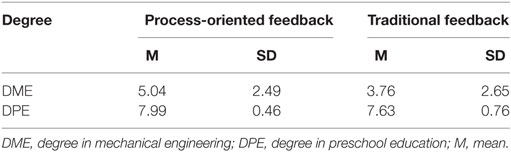
Table 3. Mean and SD of learning outcomes (LO) in relation to process-oriented v. traditional feedback in DME and DPE.
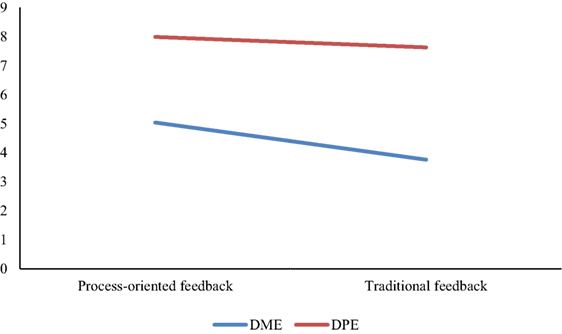
Figure 1. Mean means for learning outcomes (LO) against the independent variable “type of feedback” (process-oriented feedback vs. Traditional feedback) on the different degrees. Note. DME = Degree in Mechanical Engineering; Degree in Preschool Education = DPE.
Not significant differences were found in relation to Research RQ2 [“Will the students who receive process-oriented feedback have a better perception of their subject-related knowledge than the students who receive grade-oriented feedback (traditional feedback) following the intervention and will those results be affected by the type of degree (engineering degree v. social science degree)?”], (F = 0.01, p = 0.91, η2 = 0.001). Neither were significant differences found in relation to the covariable “type of degree” (F = 0.09, p = 0.76, η2 = 0.001). In Table 4 and Figure 2, the mean of SPKI after in relation to the two sorts of feedback (process-oriented v. traditional feedback) are shown.
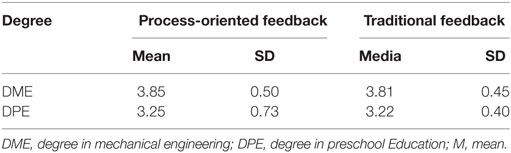
Table 4. Mean and SD of SPKI after in relation to process-oriented v. traditional feedback in DME and DPE.
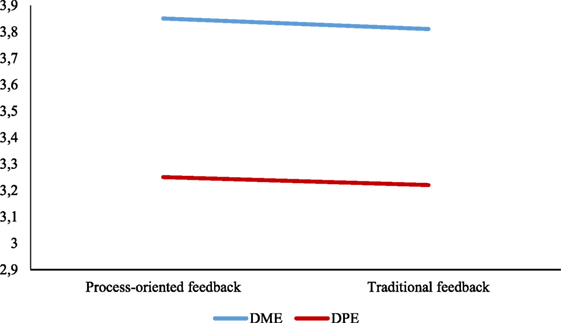
Figure 2. Mean means for student perceptions of knowledge (SPKI) after training in the independent variable “type of feedback” (process-oriented feedback v. traditional feedback) on the two degrees. Note. DME = Degree in Mechanical Engineering; Degree in Preschool Education = DPE.
Relation between Metacognitive Skills and Motivation and LO and SPKI
With regard to Research Q3 [“Will there be a significant relation between LO and metacognitive skills and will there be differences in relation to the type of degree (engineering degree v. social science degree)?”], Research Q4 [“Will there be a significant relation between LO and motivation and will there be differences in relation to the type of degree (engineering degree v. social science degree)?”], Research RQ5: “Will there be a significant relation between SPKI and metacognitive skills and will there be differences in relation to the type of degree (engineering degree v. social science degree)?” and Research RQ6: “Will there be a significant relation between SPKI and motivation and will there be differences in relation to the type of degree (engineering degree v. social science degree)?.” We observed relations between SPKI after and self-knowledge (r = 0.30), Planning (r = 0.30) and motivation skills (r = 0.34) among engineering students (DME), but not relations with LO (see Table 5). With regard to students studying the social sciences (PED), we found relations between LO and self-knowledge (r = 0.21) and motivation skills (r = 0.21) but not with SPKI (see Table 6).
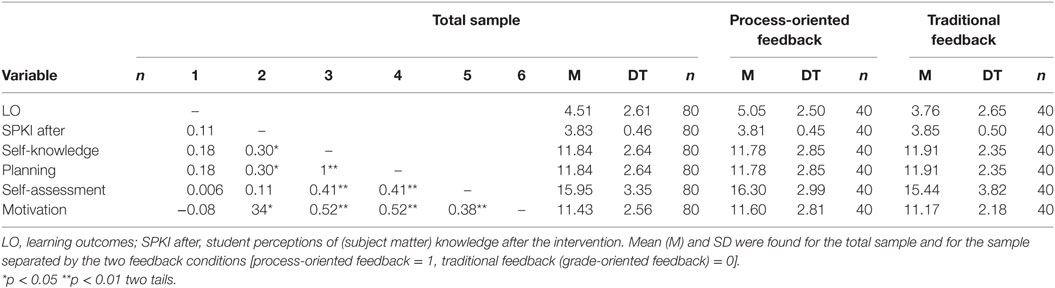
Table 5. Correlations between metacognitive skills and information-processing skills and the results of academic learning and in rubrics after the intervention in DME.
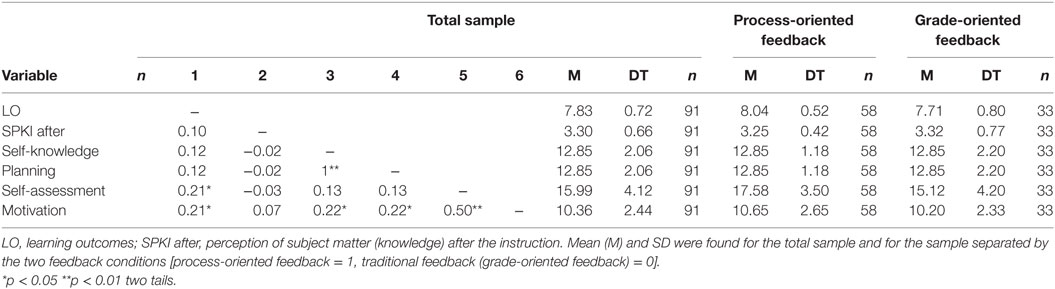
Table 6. Correlations between metacognitive skills and information-processing skills and the results of academic learning and in rubrics after the intervention in preschool education degree.
We applied “SEM” to the three models, in order to answer Research RQ7 (“Will the results be validated with the structural equation model?”):
(a) Model 1 presents the relations between the “Metacognitive skills of Planning and Self-assessment,” “Motivation skills,” and “SPKI before” with LO and “SPKI after” (uninfluenced by “SPKI before”).
(b) Model 2 refers to the results on the influence of “type of students” (engineering v. social sciences), “type of feedback” (process-oriented feedback v. traditional feedback), “metacognitive skills” (planning and self-assessment) and “motivation skills” on LO and the effect on “SPKI after” of “type of students,” “planning,” and “motivation skills.” Relations were found between all of these variables.
(c) Model 3 is similar to Model 2, except that only “Metacognitive skills” (Planning and Self-assessment) and “Motivation skills” have a relation between each other.
Model 2 was found to have a good fit (see Table 7 and Figures 3–5).
Discussion and Conclusion
In this study, we have compared the effects of two types of feedback (Process-oriented feedback v. Traditional feedback) on LO (RQ1) and on SPKI after (RQ2). We have also analyzed the relation between metacognitive skills and motivational skills among two types of students (engineering and social sciences students) in terms of LO and SPKI after [RQ3, RQ4 and RQ5, RQ6, respectively]. We found better results when the professor used process-oriented feedback rather than traditional feedback. The first type of feedback is based on error correction through self-regulated instruction and it improves motivation and metacognitive skills. This result is in accordance with feedback research (Hattie and Timperley, 2007; Alonso-Tapia et al., 2014). Another important conclusion is that the use of rubrics alone will not ensure a better learning outcome. If rubrics are to be successful, then their use must be accompanied by self-regulated instruction (Hattie, 2013). There were also differences between social science students and engineering students in terms of LO. These differences can be explained in terms of curricula content and the different metacognitive skills that the students use (Sáiz et al., 2012; Daly et al., 2014; Sáiz and Montero, 2015). The engineering students used more motivation skills and self-knowledge and planning skills in relation to SPKI after than in relation to LO. This result implies that the engineering students organize their learning in terms of self-perception of knowledge. For them, self-perception is more important in learning knowledge than the LO. This result suggests a deep level of self-perception. Conversely, the students of social sciences attach greater importance to the LO in relation to knowledge acquisition. In future research, we will analyze those characteristics of the learning tasks that might have a strong relation with the LO and the SPKI after.
As regards the SPKI after, the results in no way revealed that the type of feedback provided by the professor was a determining factor in the estimates that the students made of their own learning processes, on either of the two degree courses under study. In this research, those estimates were found to be connected with self-knowledge, planning, and the motivation skills of the engineering students (Sáiz and Román, 2011). This is a relevant aspect for future investigations in which the relation between motivation and success could be studied, both in relation to LO and in relation to SPKI before and after, analyzing the effects of both over-estimation and under-estimation to different degrees (Zimmerman and Moylan, 2009). So, if the student is to learn, SRL is essential (Brown and Harris, 2013; Panadero et al., 2013; Malmberg et al., 2015). Likewise, in future studies, we should analyze why the type of feedback had not influence on the students’ self-perceptions of their own knowledge.
In summary, the teacher’s feedback based on self-regulation is more effective than traditional feedback. Nevertheless, the effects of this type of feedback are not similar on other types of students. Successful learning appears to be conditioned by the type of learning task (Finelli et al., 2015). Likewise, self-perception of knowledge is directly related to achievement motivation (Flavell, 1981; Efklides, 2011; Van der Stel and Veenman, 2014; Sáiz and Montero, 2015). Both the individual and the interactive processes that influence the regulation of learning should be considered. Hence, the student must perceive relevant information in the environment and to integrate it with their previous knowledge of the task in an individual and social setting (Mentzer et al., 2015). In this investigation, the level of student motivation toward the task has been studied, but not the level of collective motivation of the group toward the development of the tasks. Likewise, the previous subject matter knowledge of the students in the different groups under analysis was assessed, but not the collective relation between that knowledge for problem-solving in groups (Molenaar and Chiu, 2014). As challenges in future investigations, the relation that the error analysis conducted by the student has on both variables (previous knowledge and student motivation) could be studied. If differences between students are found in that analysis, they could be compared with data-mining techniques to examine individual and interactive learning tasks in different types of degree courses.
With regard to the analysis of the adjustment of the theoretical model on successful learning from the viewpoint of different investigations (Zimmerman and Moylan, 2009; Hattie, 2013; Panadero and Jonsson, 2013; Panadero et al., 2013). The results indicate that although planning and self-assessment metacognitive skills are relevant to the perceptions of the knowledge held by students, they cannot explain successful learning, which is linked to variables related to the type of task, the understanding of learning goals and the effectiveness of the support that the teacher provides during the instruction process. Future lines of research will, therefore, be directed at evaluating those variables on different degree courses and at analyzing whether significant differences may be found. The present-day educational system in higher education is structured around the development of competences (Daly et al., 2014), so the question of whether that structure is, in practice, similar in the different degrees should be analyzed. These results point to a need for training on improvements in the use of self-knowledge planning and self-instruction skills among engineering students not only in terms of SPKI after but also in terms of LO, a training that teachers can initiate in the classroom (Sáiz and Bol, 2014).
Limitations of This Study
The present research has been completed with a sample of students studying for degrees in the social sciences and in engineering, although the sample may be enlarged and other degrees included in future investigations to arrive at a broader comparison. Likewise, the effects of feedback on the academic results of students have been analyzed in relation to their global score. Nevertheless, in future studies, the global score could be broken down into partial scores obtained in the different assessment procedures, which refer to the analysis of different types of competences, so as to confirm the effects of both feedback type and learning skills on those competences.
It should likewise be recalled that the percentage of women on engineering degrees is much lower than the percentage of men; an aspect that is reversed on education degrees. So, it may be that the gender covariable explains some of the differences that are found. Therefore, future investigations will be directed at studying whether the gender covariable can in some way influence the effects of feedback on learning. A study could also be conducted on the reasons students have for their choice of degree course.
Ethics Statement
The ethics committee of the University of Burgos approved this study. Written informed consent was obtained from all participants.
Author Contributions
MCSM has performed the statistical analysis and interpretation of the data and she has elaborated the manuscript. JMAC and IICS have applied the evidence and have collaborated in the drafting of the conclusions of the paper. LPA she has applied the tests in the different groups.
Conflict of Interest Statement
The authors declare that the research was conducted in the absence of any commercial or financial relationships that could be construed as a potential conflict of interest.
Acknowledgments
Thanks to all the students who participated in this study. Also to the grants for funding the dissemination of research results from the Vice-Rectorate for Research and Knowledge Transfer, 2017 at University of Burgos. We also would like to thank the reviewers for their detailed comments and suggestions to the initial manuscript.
Funding
The work was supported by University of Burgos.
Supplementary Material
The Supplementary Material for this article can be found online at http://journal.frontiersin.org/article/10.3389/feduc.2017.00034/full#supplementary-material.
References
Alonso-Tapia, J., Panadero, E., and Díaz, M. (2014). Development and validity of the emotion and motivation self-regulation questionnaire (EMSR-Q). Span. J. Psychol. 17, 1–15. doi: 10.1017/sjp.2014.41
Anderson, L. W., and Krathwohl, D. R. (eds) (2001). A Taxonomy for Learning, Teaching, and Assessing. A Revision of Bloom’s Taxonomy of Educational Objectives. New York: Longman.
Arias, B. (2008). Desarrollo de un ejemplo de análisis factorial confirmatorio con LISREL. AMOS y SAS. Seminario de Actualización en Investigación sobre Discapacidad SAID 2008. [Development of an example of confirmatory factor analysis with LISREL. AMOS and SAS. Updated Seminar on Disability Research SAID 2008]. Available at: http://sid.usal.es/idocs/F8/FDO20749/said_2008.pdf
Arias, B., Verdugo, M. A., Navas, P., and Gómez, L. E. (2013). Factor structure of the construct of adaptive behavior in children with and without intellectual disability. Int. J. Clin. Health Psychol. 13, 155–166. doi:10.1016/S1697-2600(13)70019-X
Bandalos, D. L., and Finney, S. J. (2001). “Item parceling issues in structural equation modeling,” in New Development and Techniques in Structural Equation Modeling, eds G. A. Marcoulides and R. E. Schumacker (Mahwah, NJ: Lawrence Erlbaum), 269–296.
Brown, G. T. L., and Harris, L. R. (2013). “Student self-assessment,” in Sage Handbook of Research on Classroom Assessment, ed. E. J. McMillan (Thousand Oaks, CA: SAGE), 367–393.
Bumbaco, A. E., and Douglas, E. P. (2015). “A thematic analysis comparing critical thinking in engineering and humanities undergraduates,” in ASEE Annual Conference and Exposition, Conference Proceedings. 122nd ASEE Annual Conference and Exposition, Seattle, WA.
Butler, D. L., and Winne, P. H. (1995). Feedback and self-regulated learning: a theoretical synthesis. Rev. Educ. Res. 65, 245–281. doi:10.3102/00346543065003245
Camarero, F., Martín del Buey, F., and Herrero Díez, J. (2000). Estilos y estrategias de aprendizaje en estudiantes universitarios [Styles and learning strategies in university students]. Psicothema 12, 615–622.
Carbonero, M. A., Román, J. M., and Ferrer, M. (2013). Programa para “aprender estratégicamente” con estudiantes universitarios: diseño y validación experimental. [Program to “learn strategically” with university students: design and experimental validation]. Anales de Psicología 29, 876–885. doi:10.6018/analesps.29.3.165671
Daly, S. R., Mosyjowski, E. A., and Seifert, C. M. (2014). Teaching creativity in engineering courses. J. Eng. Educ. 103, 417–449. doi:10.1002/jee.20048
Diefes-dux, H. A., Zawojewski, J. S., Hjalmarson, M. A., and Cardella, M. E. (2012). A framework for analyzing feedback in a formative assessment system for mathematical modeling problems. J. Eng. Educ. 101, 375–406. doi:10.1002/j.2168-9830.2012.tb00054.x
Dinsmore, D. L., and Parkinson, M. M. (2013). What are confidence judgments made of? Students’ explanations for their confidence ratings and what that means for calibration. Learn. Instruct. 24, 4–14. doi:10.1016/j.learninstruc.2012.06.001
Efklides, A. (2011). Interactions of metacognition with motivation and affect in self-regulated learning: the MASRL model. Educ. Psychol. 46, 6–25. doi:10.1080/00461520.2011.538645
Finelli, C. J., Borrego, M., and Rasoulifar, G. (2015). “I Am an Engineer AND”: a mixed methods study of socially engaged engineers. J. Eng. Educ. 104, 365–387. doi:10.1002/jee.20101
Flavell, J. H. (1981). “Cognitive monitoring,” in Children’s Oral Communication Skills, ed. W. En Dickson (New York: Academic Press), 35–60.
Garzón Umerenkova, A., de la Fuente Arias, J., Martínez Vicente, J. M., Zapata Sevillano, L., Pichardo, M. C., and García-Berbén, A. B. (2017). Validation of the Spanish short self-regulation questionnaire (SSSRQ) through Rasch analysis. Front. Psychol. 8:276. doi:10.3389/fpsyg.2017.00276
Grohs, J. R., Kinoshita, T., Novoselich, B. J., and Knight, D. B. (2015). “Exploring learner engagement and achievement in large undergraduate engineering mechanics courses,” in ASEE Annual Conference and Exposition (Seattle, Washington). Available at: https://peer.asee.org/exploring-learner-engagement-and-achievement-in-large-undergraduate-engineering-mechanics-courses
Harks, B., Rakoczy, K., Hattie, J., Besser, M., and Klieme, E. (2014). The effects of feedback on achievement, interest and self-evaluation: the role of feedback’s perceived usefulness. Int. J. Exp. Educ. Psychol. 34, 269–290. doi:10.1080/01443410.2013.785384
Hattie, J. (2013). Calibration and confidence: where to next? Learn. Instruct. 24, 62–66. doi:10.1016/j.learninstruc.2012.05.009
Hattie, J., and Gan, M. (2011). “Instructions based on feedback,” in Handbook of Research on Learning and Instruction: Educational Psychology Handbook series, eds R. E. Mayer and P. A. Alexander (New York: Routledge), 249–271.
Hattie, J., and Timperley, H. (2007). The power of feedback. Rev. Educ. Res. 77, 81–112. doi:10.3102/003465430298487
Kim, M. K. (2015). Models of learning progress in solving complex problems: expertise development in teaching and learning. Contemp. Educ. Psychol. 42, 1–16. doi:10.1016/j.cedpsych.2015.03.005
Malmberg, J., Järvelä, S., Järvenoja, H., and Panadero, E. (2015). Promoting socially shared regulation of learning in CSCL: progress of socially shared regulation among high- and low-performing groups. Comput. Human Behav. 52, 562–572. doi:10.1016/j.chb.2015.03.082
Mavinkurve, M., and Murthy, S. (2014). “Self-assessment rubrics as metacognitive scaffolds to improve design thinking,” in Proceedings of the 22nd International Conference on Computers in Education, ICCE, 750–755.
Menekse, M., Stump, G. S., Krause, S., and Chi, M. T. H. (2013). Differentiated overt learning activities for effective instruction in engineering classrooms. J. Eng. Educ. 102, 346–374. doi:10.1002/jee.20021
Menéndez-Varela, J. L., and Gregori-Giralt, E. (2016). The contribution of rubrics to the validity of performance assessment: a study of the conservation–restoration and design undergraduate degrees. Assess. Eval. High. Educ. 41, 228–244. doi:10.1080/02602938.2014.998169
Mentzer, N., Becker, K., and Sutton, M. (2015). Engineering design thinking: high school students’ performance and knowledge. J. Eng. Educ. 104, 417–432. doi:10.1002/jee.20105
Molenaar, I., and Chiu, M. M. (2014). Dissecting sequences of regulation and cognition: statistical discourse analysis of primary school children’s collaborative learning. Metacogn. Learn. 9, 137–160. doi:10.1007/s11409-013-9105-8
Montfort, D., Herman, G. L., Brown, S., Matusovich, H. M., Streveler, R. A., and Adesope, O. (2015). Patterns of student conceptual understanding across engineering content areas. Int. J. Eng. Educ. 31, 1587–1604.
Moreno, R., Reisslein, M., and Ozogul, G. (2009). Optimizing worked-example instruction in electrical engineering: the role of fading and feedback during problem-solving practice. J. Eng. Educ. 98, 83–92. doi:10.1002/j.2168-9830.2009.tb01007
Narayanan, M. (2013). “Assessment of learning based on the principles of discovery and metacognition,” in 120th ASEE Annual Conference and Exposition, Conference Proceedings. Paper ID #6562, 2–22. Retrieved at: https://peer.asee.org/assessment-of-learning-based-on-the-principles-of-discovery-and-metacognition.pdf
Narciss, S. (2008). “Feedback strategies for interactive learning tasks,” in Handbook of Research on Educational Communications and Technology, 3rd Edn, eds J. M. Spector, M. D. Merrill, J. J. G. van Merrienboer, and M. P. Driscoll (Mahwah, NJ: Lawrence Erlbaum Associates), 125–144.
Narciss, S. (2013). Designing and evaluating tutoring feedback strategies for digital learning environments on the basis of the interactive tutoring feedback model. Digit. Educ. Rev. 23, 7–26.
Oenardi, L., and Santoso, H. B. (2012). “Implementation of enhanced guided notes to promote students’ metacognitive self-regulated learning strategies while learning electric circuit concepts,” in Proceedings – Frontiers in Education Conference, FIE, 1–6. Retrieved at: http://ieeexplore.ieee.org/stamp/stamp.jsp?arnumber=6462450
Panadero, E. (2017). A review of self-regulated learning models: Zimmerman, Boekaerts, Winne & Hadwin, Pintrich, Efklides, and Hadwin, Järvelä & Miller. Front. Psychol. 8:422. doi:10.3389/fpsyg.2017.00422
Panadero, E., Alonso-Tapia, A., and Reche, E. (2013). Rubrics vs. self-assessment scripts effect on self-regulation, performance and self-efficacy in pre-service teachers. Stud. Educ. Eval. 39, 125–132. doi:10.1016/j.stueduc.2013.04.001
Panadero, E., Alonso-Tapia, J., and Huertas, J. A. (2012). Rubrics and self-assessment scripts on self-regulation, learning and self-efficacy in secondary education. Learn. Individ. Differ. 22, 806–813. doi:10.1016/j.lindif.2012.04.007
Panadero, E., and Jonsson, A. (2013). The use of scoring rubrics for formative assessment purposes revisited: a review. Educ. Res. Rev. 9, 129–144. doi:10.1016/j.edurev.2013.01.002
Panadero, E., and Romero, M. (2014). To rubric or not to rubric? The effects of self-assessment on self-regulation, performance and self-efficacy. Assess. Educ. Princ. Policy Pract. 21, 133–148. doi:10.1080/0969594X.2013.877872
Parmar, A. J. (2014). “Bridging gaps in engineering education: design thinking a critical factor for project based learning,” in Proceedings – Frontiers in Education Conference, FIE, 1–8. Available at: http://ieeexplore.ieee.org/xpls/abs_all.jsp?arnumber=7044081
Pate, M. L., Beard, F. R., Miller, B., Miller, R., Hatch, R., and Hall, K. (2015). “Project based learning: integration across agricultural systems technology courses,” in ASABE Annual International Meeting, 152156630. doi:10.13031/aim.20152156630
Ramírez-Corona, N., Zaira, R. A. L., López-Malo, A., and Palou, E. (2013). “Assessing metacognitive awareness during problem-solving in a kinetics and homogeneous reactor design course,” in 120th ASEE Annual Conference and Exposition, Conference Proceedings. Paper ID #6294. Retrieved at: https://www.engr.uky.edu/~aseeched/papers/2013/6294.pdf
Román, J. M., and Poggioli, L. (2013). ACRAr: Escalas de estrategias de aprendizaje [Scales of Learning Skills]. Caracas: Publicaciones UCAB (Postgrado: Doctorado en Educación).
Sáiz, M. C., and Bol, A. (2014). Aprendizaje basado en la evaluación mediante rúbricas en educación superior [Learning based on assessment with rubrics: a study in higher education]. Suma Psicol. 21, 28–35. doi:10.1016/S0121-4381(14)70004-9
Sáiz, M. C., and Bol, A. (2015). “Cómo enseñar y cómo evaluar la resolución de problemas en Física: una reflexión sobre la propia práctica [How to teach and how to assess problem solving in physics: a reflection on own practice],” in Innovación en la enseñanza de las ciencias: reflexiones, experiencias y buenas prácticas [Science Teaching Innovation: An Reflections, Experiences and Best Practices], ed. M. A. Queiruga Dios (A Coruña: Editorial Q), 129–146. Available at: http://scientix.fecyt.es/2015/05/divulgacion-innovacion-en-la-ensenanza.html
Sáiz, M. C., Cuesta, I., Alegre, J. M., Barrio, L., and Peñacoba, L. (2016). “Evaluación a través de rúbricas en la asignatura de Elasticidad y Resistencia de Materiales del Grado en Ingeniería Mecánica. [Assessment through rubrics on the subject-module elasticity and strength of materials on the mechanical engineering degree],” in La docencia universitaria: desafíos y perspectivas [Universitary Teaching: Challenges and Perspectives], eds P. Membiela, N. Casado, and M. I. Cebreiros (Madrid: Educación Editora), 51–55.
Sáiz, M. C., and Escolar, M. C. (2013). Observación Sistemática e Investigación en Contextos Educativos [Systematic Observation and Research in Educational Contexts]. Burgos: Servicio de Publicaciones de la Universidad de Burgos.
Sáiz, M. C., and Montero, E. (2015). “Metacognition, self-regulation and assessment in problem-solving processes at university,” in Metacognition: Fundaments, Applications, and Trends, ed. A. Peña-Ayala (Switzerland: Springer), 1–27.
Sáiz, M. C., Montero, E., Bol, A., and Carbonero, M. A. (2012). An analysis of learning to learning competencies at the university. Electron. J. Res. Educ. Psychol. 10, 253–270.
Sáiz, M. C., and Román, J. M. (2011). Cuatro formas de evaluación en educación superior gestionadas desde la tutoría. [Four forms of assessment in higher education managed from the tutorial]. Revista de Psicodidáctica 16, 145–161. doi:10.1387/RevPsicodidact.1116
Sáiz, M. C., Sánchez-Báez, M. A., Ortega-López, V., and Manso-Villalaín, J. M. (2015). Self-regulation and rubrics assessment in structural engineering subjects. Educ. Res. Int. 340521, 129–146. doi:10.1155/2015/340521
Schaffer, H. E., Young, K. R., Ligon, E. W., and Chapman, D. D. (2017). Automating individualized formative feedback in large classes based on a directed concept graph. Front. Psychol. 8:260. doi:10.3389/fpsyg.2017.00260
Van der Stel, M., and Veenman, M. V. J. (2014). Metacognitive skills and intellectual ability of young adolescents: a longitudinal study from a developmental perspective. Eur. J. Psychol. Educ. 29, 117–137. doi:10.1007/s10212-013-0190-5
Van Meter, P. N., Firetto, C. M., Turns, S. R., Litzinger, T. A., Cameron, C. E., and Shaw, C. W. (2016). Improving students’ conceptual reasoning by promoting cognitive operations. J. Eng. Educ. 105, 245–277. doi:10.1002/jee.20120
Wäschle, K., Allagaier, A., Lachner, A., Siegfried, F., and Nückles, M. (2014). Procrastination and self-efficacy: tracing vicious and virtuous circles in self-regulated learning. Learn. Instruct. 29, 103–114. doi:10.1016/j.learninstruc.2013.09.005
Watson, M. K., Pelkey, J., Noyes, C. R., and Rodgersc, M. O. (2016). Assessing conceptual knowledge using three concept map scoring methods. J. Eng. Educ. 105, 118–146. doi:10.1002/jee.20111
Wilkins, K. G., Bernstein, B. L., and Bekki, J. M. (2015). Measuring communication skills: the STEM interpersonal communication skills assessment battery. J. Eng. Educ. 104, 433–453. doi:10.1002/jee.20105
Zimmerman, B. J., and Moylan, A. R. (2009). “Self-regulation: where metacognition and motivation intersect,” in Handbook of Metacogntion in Education, eds E. D. J. Hacker, J. Dunlosky, and A. C. Graesser (New York: Routledge), 299–315.
Keywords: institutional change, rubric, feedback, higher education, self-regulation of learning, assessment
Citation: Sáiz-Manzanares MC, Cuesta Segura II, Alegre Calderon JM and Peñacoba Antona L (2017) Effects of Different Types of Rubric-Based Feedback on Learning Outcomes. Front. Educ. 2:34. doi: 10.3389/feduc.2017.00034
Received: 15 April 2017; Accepted: 26 June 2017;
Published: 13 July 2017
Edited by:
Jesus de la Fuente, University of Almería, SpainReviewed by:
Barbara McCombs, University of Denver, United StatesEdgar Galindo, University of Évora, Portugal
Copyright: © 2017 Sáiz-Manzanares, Cuesta Segura, Alegre Calderon and Peñacoba Antona. This is an open-access article distributed under the terms of the Creative Commons Attribution License (CC BY). The use, distribution or reproduction in other forums is permitted, provided the original author(s) or licensor are credited and that the original publication in this journal is cited, in accordance with accepted academic practice. No use, distribution or reproduction is permitted which does not comply with these terms.
*Correspondence: María Consuelo Sáiz-Manzanares, bWNzbWFuemFuYXJlcyYjeDAwMDQwO3VidS5lcw==