- 1Centro de Ciencias Matemáticas, Universidad Nacional Autónoma de México, Morelia, Mexico
- 2Departamento de Producción Agrícola y Animal, División de Ciencias Biológicas y de la Salud, Universidad Autónoma Metropolitana-Unidad Xochimilco (UAM-X), Ciudad de México, Mexico
- 3Centro de Ciencias de la Complejidad (C3), Universidad Nacional Autónoma de México, Ciudad Universitaria, Ciudad de México, Mexico
Megaphylls, present in the majority of vascular plants, show in many plant lineages an abaxial-adaxial polarity in their dorsoventral axis. This polarity commonly translates into different tissues developing on each side of the leaf blade. This is important because it promotes better photosynthetic efficiency as related to light absorption and gas exchange. Many researchers have studied the molecular bases of the emergence of leaf abaxial-adaxial polarity, showing that it is produced by the interaction and differential expression of particular genes and other molecules. However, until now, it is still unclear if the molecular components documented thus far are sufficient to explain the emergence of leaf polarity. In this work, we integrated the available experimental data to construct a graph of the Gene Regulatory Network (GRN) involved in the formation of abaxial-adaxial polarity in the leaf primordium of Arabidopsis thaliana. This graph consisted of 21 nodes and 47 regulations. We extracted the main components of the graph to obtain a Minimum Network consisting of six genes and 22 possible regulations. Then, we used the Boolean network (BN) formalism to describe the dynamics of this Minimum Network. We identified 1905 distinct BNs that comprised the regulations of the Minimum Network and exclusively generated the two attractors representing the abaxial and adaxial cell types. This highlights the fact that most graphs, including our network, can describe experimentally observed behaviors with many BN dynamics. By performing mutant simulations and robustness analysis, we found that two of the 1905 BNs better reproduce experimentally available information. To produce the expected attractors, both BNs predict the same missing regulations, which we propose should be experimentally analyzed to confirm their existence. Interestingly, these two BNs have low robustness to perturbations compared with previously analyzed GRNs. This was an unexpected result since abaxial-adaxial polarity is a robust biological trait, which suggests more components or regulations of the network are missing.
1 Introduction
A typical leaf in vascular plants is a flat organ that consists of three main axes: proximal-distal, center-lateral, and dorso-ventral (also called abaxial-adaxial), resulting from differential growth during development (Bowman et al., 2002). Leaf formation begins with the differentiation of peripheral cells derived from the Shoot Apical Meristem (SAM), which form a protrusion called a leaf primordium (Kalve et al., 2014). During leaf development the upper part of the primordium - adjacent to the SAM - will become the adaxial side of the leaf, while the lower part will give rise to the abaxial side (see Dkhar and Pareek, 2014 for a review on leaf shape determination). In eudicots the two sides typically acquire different cellular tissues (Figure 1): the adaxial side develops a palisade mesophyll with few and small intercellular spaces and an epidermis with a higher number of trichomes. In contrast, the abaxial side has a spongy mesophyll characterized by large intercellular spaces and an epidermis with more stomata (see Kuhlemeier and Timmermans, 2016 for a review on abaxial-adaxial polarity determination in leaves). Once dorsiventral polarity is established in the leaf primordium, it will promote lateral growth (Qi et al., 2017), resulting in a flat leaf with bilateral and dorsiventral symmetry. Dorsiventral leaves are present in most angiosperms, with a few exceptions in some lineages that develop unifacial leaves or show an inversion in the arrangement of both sides (Fukushima and Hasebe, 2014; Tsukaya, 2014). The dominance of bifacial leaves suggests that abaxial-adaxial polarity is a highly stable characteristic that has been maintained throughout the evolution of angiosperms, likely due to the photosynthetic efficiency of dorsiventral leaves, which present a high surface area for light assimilation, allowing for a high surface-to-volume ratio, while reducing water loss by maintaining a higher density of stomata on the abaxial side (Kuhlemeier and Timmermans, 2016; Wall et al., 2022).
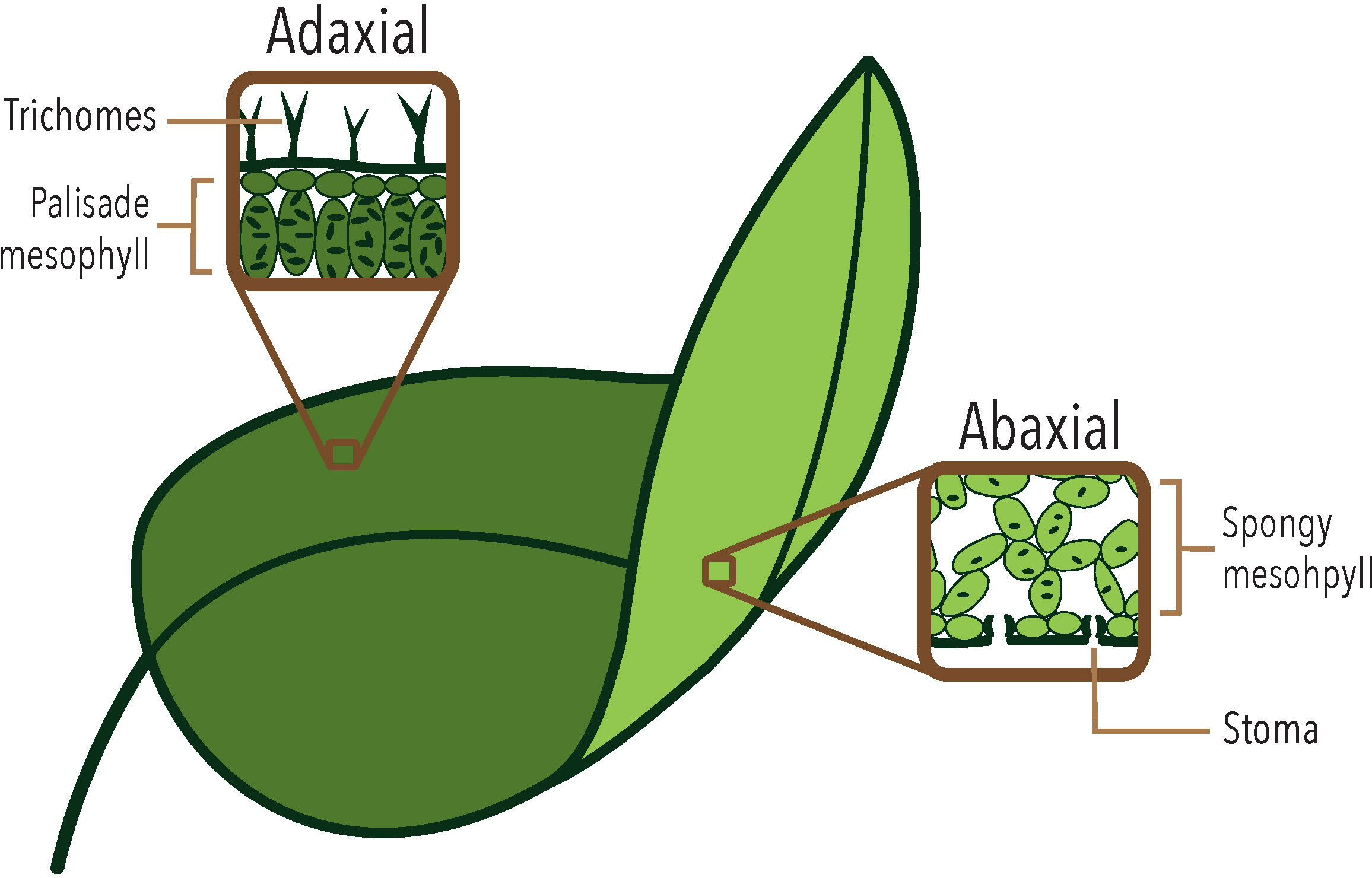
Figure 1 The different tissues that differentiate in the abaxial and adaxial sides of a typical eudicot leaf, such as Arabidopsis thaliana.
Numerous works have studied the genetic factors involved in the formation of abaxial-adaxial polarity in several plant species, with special emphasis in the model plants Arabidopsis thaliana, Zea mays, Oryza sativa and Antirrhinum majus (Husbands et al., 2009; Yamaguchi et al., 2012; Reinhart et al., 2013). In A. thaliana, the genes that appear to be responsible for the formation of leaf abaxial-adaxial polarity are transcription factors from the KANADI (specially KAN1, KAN2, KAN3 and KAN4), YABBY (YAB1, YAB3 and YAB5), HD-ZipIII (PHABULOSA or PHB, PHAVOLUTA or PHV and REVOLUTA or REV), AUXIN RESPONSE FACTORS (ARF3 and ARF4) and ASYMMETRIC LEAVES (AS1 and AS2) gene families (Siegfried et al., 1999; Byrne et al., 2000; Ori et al., 2000; Eshed et al., 2001; Emery et al., 2003; Pekker et al., 2005). Homologs of the majority of these genes have been found throughout angiosperms, as well as in non-seed plants (Sarojam et al., 2010). In addition to the role of these genes, post-transcriptional regulation plays an important part in the formation of leaf polarity, with important roles for miRNA165, miRNA166 and tasiR-ARF (Emery et al., 2003; Allen et al., 2005; Li et al., 2005) in the regulation of several of the genes listed above. The involvement of microRNAs in the regulation of dorsiventrality suggests that genes from the ARGONAUTE (AGO) family are relevant to this process, since they are required for the microRNA silencing of target mRNA through the formation of the RISC complex (Kidner and Martienssen, 2005; Liu et al., 2011). Genes from this family whose role in the regulation of abaxial-adaxial polarity has been documented are AGO1 and AGO10 (Zhang et al., 2006; Liu et al., 2009; Zhu et al., 2011). Some genes involved in SAM identity and maintenance may also play a part in the generation of polarity in lateral organs. The transcription factor SHOOT MERISTEMLESS (STM) could be involved in both processes, as its ectopic expression leads to the formation of meristems on the adaxial surface of lateral organs (Hay et al., 2002). Finally, an additional factor contributing to polarity development is the phytohormone auxin, which presents a high concentration in lateral organ primordia and is transported back to the SAM during the development of the new organ, leading to a lower hormone concentration on the adaxial side and a higher on the abaxial side (Heisler et al., 2005; Qi et al., 2014). This has prompted suggestions that auxin is a potential candidate for the Sussex signal – a hypothetical signal thought to originate in the SAM, that reaches the lateral organ primordia and induces their polarity (Sussex, 1951; Reinhardt et al., 2005). Most of these molecular factors can be divided into two groups: promoters of abaxial or adaxial fate. The abaxial-promoting factors include the KANADI, YABBY and ARF genes listed above, as well as the post-transcriptional factor miRNA165/166. The adaxial-promoting factors are the HD-ZipIII and AS genes as well as tasiR-ARF. These two groups regulate the same set of target genes in opposite ways, while they interact with genes from the other group in a mutually repressive manner (Husbands et al., 2009; Yamaguchi et al., 2012; Qi et al., 2017). Furthermore, all of these molecular factors show an expression corresponding to the identity they promote and none of them seem to have intercellular mobility (Husbands et al., 2009), with the exception being the post-transcriptional factors and the HD-ZipIII transcription factor REV, whose mRNA appears to move through cells (Thieme et al., 2015).
Although all of these genetic factors have been extensively studied, the mechanism by which the genetic network’s dynamics generates an abaxial-adaxial polarity is not fully comprehended. In fact, there are still some regulations where the effect towards the target gene is uncertain. For example, the regulation of AS1 and AS2 by YABBY shows some contradictory results where some experimental analyses suggest is an activation (Bonaccorso et al., 2012), while others propose that the same regulation is an inhibition (Lin et al., 2003; Bonaccorso et al., 2012) and some reports even suggest an absence of regulation (Kumaran et al., 2002). Also, the exact function of many of these genes in generating polarity is not well-understood. For example, it is unknown whether the YABBY genes are important for the onset of leaf polarity or for its maintenance (Sarojam et al., 2010). Mathematical and computational models can help us to elucidate these and other uncertainties. They can be used to simulate the behavior of genetic networks, which can help us identify the key factors that control polarity and to what degree. Additionally, computational models can be used to test hypotheses about how genetic networks work, which can help us refine our understanding of these systems and propose new regulatory hypotheses that can be experimentally tested. Also, these theoretical approaches can help to integrate the vast amount of new experimental information and proceed from a descriptive to a predictive analysis.
As far as we know, there is only one previous model analyzing the genetic dynamics of abaxial-adaxial polarity in lateral organs, constructed by La Rota et al. (2011). This model focuses on the emergence of polarity in sepals, which are floral organs akin to leaves. However, until now, there is no model that explicitly addresses how polarity is established during the development of leaf primordia. Given that the elements of a Gene Regulatory Network (GRN) are molecules whose concentration can change over time depending on their interactions, it is important to create a dynamic model of the process. Broadly speaking, there are two general mathematical approaches: continuous models, such as those based on differential equations, or discrete models. Discrete models provide an alternative to the challenges encountered within models based on differential equations by not requiring quantitative information about the components and the value of the parameters of the system. Moreover, discrete models are easily modified and can handle a larger number of components than their continuous counterpart (Saadatpour and Albert, 2013). The simplest type of discrete modeling of a GRN are Boolean networks, where nodes can only take two values: 1 or 0, representing whether the molecule is active or above a certain threshold (1), or inactive or below a certain threshold (0), respectively. For instance, in the case of a gene, 1 would indicate that the gene is being expressed, and 0 would indicate that it is not. The value of each node is updated based on the values of the nodes that regulate it. Despite its simplicity, Boolean networks can provide important information. For example, by updating the values of all nodes in the network, a steady state (or set of states) called an attractor is reached. It has been shown that the node values in an attractor represent their expression in the cell, so each attractor can be interpreted as a cell type (Kauffman, 1969; Espinosa-Soto et al., 2004; Ortiz-Gutiérrez et al., 2015; García-Gómez et al., 2017).
In this study, we have systematized the available experimental data regarding the molecules involved in establishing the abaxial-adaxial polarity. Although all the genes briefly described above have an important role in abaxial-adaxial polarity in all the angiosperm species where they have been studied, their relative contribution and expression vary between species and even between organs in the same plant (Yamada et al., 2011; Sablowski, 2015; Kuhlemeier and Timmermans, 2016). Given the extensive diversity of leaf development and the genetics behind it, this work focuses on the genetic elements responsible for establishing the abaxial-adaxial polarity during the early stages of leaf primordium differentiation from the SAM, based on documented information in A. thaliana.
Using the gathered information, we constructed a network graph incorporating all identified agents, which we called Base Network. The Base Network was subsequently reduced into a Minimum Network, comprising the core polarity genes. The motivation for focusing on a reduced network was to gain a clearer understanding of the abaxial-adaxial trait by isolating the main regulators involved in its generation. Employing this Minimum Network, we searched the BN models capable of producing the abaxial-adaxial polarity and analyzed their ability to produce mutants that have been previously characterized in laboratory experiments. Additionally, we assessed the BN’s robustness under various perturbations. We concluded that the obtained Minimum Network presented in this work contains the core genes responsible for the generation of abaxial-adaxial polarity in early developing leaves of our model system.
2 Materials and methods
2.1 Network graphs
Network graphs were built by gathering all the available experimental data, characterizing genes and molecules essential for adaxial and abaxial identity in A. thaliana leaf primordium. The information included the genes involved in the emergence of leaf polarity, and the regulatory interactions between these genes. All the regulations within the Base Network, along with their respective references, are available in Supplementary Material Table S1. A more detailed explanation of some of these regulations, those constituting the Minimum Network, is shown in Supplementary Material Table S2. This information was represented by a directed graph , where the set of nodes were the genes, microRNAs, hormones, or any other molecule involved and the set of edges were the regulations between the nodes. Each regulation was described by its sign as positive or negative (+ if activating and – if inhibiting) and by the experimental information supporting the regulation as mandatory or hypothetical. Mandatory regulations were experimentally confirmed as a physical direct regulation where the two involved molecules join physically, which can be proved experimentally through techniques such as Double Hybrid Assay (see Trigg et al., 2017), Chromatin Immunoprecipitation (see Merelo et al., 2013 and Brandt et al., 2012) or by Co-immunoprecipitation (see Husbands et al., 2016). Also, all mandatory regulations were functionally confirmed by experiments such as mutant analysis, which also allows to infer if the interaction generates a positive or negative regulation towards the target gene. On the other hand, hypothetical regulations lack an experiment to confirm the physical interaction between molecules or their functionality. In other words, hypothetical regulations did not have enough experimental information to confirm that they exist but have some experimental results suggesting there is an interaction between two molecules.
2.2 Network graph reduction
To reduce the network graph we followed Saadatpour and collaborators (2013) by removing isolated nodes (nodes with no mandatory regulations), nodes that were outputs (nodes with no target genes considering only their mandatory regulations), mediators (nodes with only one input and one output mandatory regulation) and inputs (nodes with no input regulations). When eliminating a mediator node, its two regulations were fused as one and the sign of the generated regulation was the product of the original two: if both original regulations were positive or both negative, then the fused regulation was positive. If only one original regulation was negative, then the fused regulation was negative towards the target node. We removed these nodes because they do not affect important dynamic behaviors produced by a network, such as the fixed-point attractors (Veliz-Cuba, 2011; Saadatpour and Albert, 2013).
2.3 Boolean networks
To study the dynamic of the MN we used Boolean networks (BN). In BNs the nodes can have two values: 1 or 0. 1 represents an expression/activation of that molecule while 0 represents lack of expression/activity. The value of each node changes based on a Boolean function:
where are the values at time t of the regulators of node . The Boolean functions of all nodes of the network are based on experimental evidence (Wang et al., 2012; Azpeitia et al., 2014). The set of values of all the nodes of a network at a specific time is called the state of the network and is represented as a vector of ones and zeros containing the values of each node in that time. Since each node can have two values, there are possible states for a network with nodes.
An attractor is a self-sustained group of states where, once a network has reached it, it will stay in it unless a perturbation is made. If the attractor is made of one state it is called a fixed-point attractor. If the attractor comprises more than one state, it is called a cyclic attractor since once the network has reached it, it will stay oscillating through that group of states indefinitely. To find the attractors of a network one can start from all possible states of the network and follow its trajectory through time until reaching an attractor.
There are two types of updates in a BN: Synchronous, where all the nodes of the network update their values every time step, and Asynchronous, where not all the nodes update their values on each time step (Thomas and D’Ari, 1990). Unless specified, BNs were analyzed using a Synchronous update. When an Asynchronous update was used, we applied a general method (as shown in Harvey and Bossomaier, 1997 and Saadatpour et al., 2010) where at each step a single node is randomly chosen to update its value, all nodes having the same probability of being chosen. To randomly select the node, the Random module in Python was employed (Van Rossum, 2020).
2.4 Definition of the abaxial-adaxial attractors
The expected attractors correspond to the genetic expression patterns in the cell. For this reason, we decided to define one attractor corresponding to the expression of the MN genes in the cells on the abaxial side and another on the adaxial side based on their documented expression patterns (see Supplementary Material Table S3).
Although the three post-transcriptional regulatory factors exhibit mobility between cells, they exhibit a gradient expression through the leaf with a predominant accumulation on the side where they are produced — miRNA165/166 on the abaxial and tasiR-ARF on the adaxial side (Chitwood et al., 2009; Husbands et al., 2015). Because of this, we made the assumption that the expression site of all factors is also where they are being transcribed and where they regulate their targets.
For each attractor the nodes can have three different states: On (represented as 1) if the gene is expressed on that side of the leaf primordium, Off (represented as 0) if the gene doesn’t show expression on that side, and Undefined (represented with an *) if the gene shows a gradient expression and has only a minimum expression on that side compared to the other, or if contradictory experimental information exists regarding its expression on a particular side of the leaf primordium. If a gene has an Undefined state, it means it can be On or Off on that attractor.
2.5 Types of regulations on Boolean terms
Both the Base Network and the Minimum Network contain mandatory and hypothetical regulations, with their classification determined by their biological experimental support (refer to the subsection 2.0). This differentiation has implications for the Boolean Networks: in Boolean terms, mandatory regulations must be functional regulations as defined in previous works (Richard et al., 2012; Comet et al., 2013; Abou-Jaoudé et al., 2016; Mori and Mochizuki, 2017). According to this definition, a regulation is functional if a change in the value of the regulatory node alone is sufficient to modify the value of the regulated node. In the model put forth here, hypothetical regulations can be functional or not.
We defined positive and negative regulations following previous authors (Comet et al., 2013; Muñoz et al., 2018). In Boolean terms, a positive (resp. negative) regulation is defined as a functional regulation that activates (resp. inactivates) the target node in all network states where the regulation is functional. If the experimental information is not enough to define if the regulation is positive or negative, the sign is marked as unknown, implying that both positive or negative signs will be accepted in the Boolean function.
2.6 Exploration of the possible BNs using Griffin
We used the computational tool Griffin (Muñoz et al., 2018) in order to find the BNs that satisfy the regulations of the MN and generate the two expected attractors. Griffin allows to automate the necessary steps for the inference and analysis of synchronous BNs, admitting different information regarding the various types of regulations between nodes (including positive, negative, mandatory and hypothetical), as well as certain biological restrictions that the BNs most support, which in our case where the generation of only the two expected attractors.
The MN contains 22 regulations: six mandatory and 16 hypothetical. All BNs will contain the six mandatory regulations, but each one can have different hypothetical regulations.
Different combinations of hypothetical regulations can generate the same attractors, and the analysis of all possible combinations of hypothetical regulations requires a large amount of computer time and resources. To overcome this problem, we applied the radial exploration strategy of Griffin. This strategy fixes a maximal number of hypothetical regulations - called the radius - that can be included in the network. Then, it searches the BNs that produce the expected attractors among all possible BNs with the maximal number of accepted hypothetical regulations. A more detailed explanation can be found in Figure S1 on the Supplementary Material. With this strategy, we were able to find the BNs with the least number of hypothetical regulations that satisfy the regulations and biological restrictions. More details regarding the use of Griffin can be found on its page http://turing.iimas.unam.mx/griffin/index.html or in Muñoz et al., 2018.
2.7 Mutant simulations
To simulate a gain or loss of function of a gene, the value of the mutated node is fixed as 1 or 0, respectively. A BN is said to satisfy a mutant when the attractors of the mutant simulation correspond to the reported experimental results.
To simulate mutant plants, we recovered two different types of experimental results observed in the mutant. We named Expression changes to an alteration in the expression of non-mutated genes observed in plants with a mutation compared with their expression on the WT plant. This represents an observed increase or decrease, respectively, in the expression of this non-mutated gene. On the other hand, we named Cell type changes to a loss of the abaxial or adaxial cell type caused by the mutation. This biological information as well as can be found in Supplementary Material Table S4.
The mutant simulations were implemented with both synchronous and asynchronous updates in order to compare the obtained attractors. The asynchronous update was implemented as indicated in subsection 2.3. Boolean networks.
2.8 Robustness analysis
As a complementary strategy to validate the BNs outlined above, we performed two robustness analyses. The first analysis consisted in making bitflip perturbations. Boolean functions can be represented as truth tables. Bitflip perturbations consisted in changing one by one each line of the truth table of each node (if the output is 0, the bitflip changes it to 1 and vice versa). The second analysis required the comparison between a BN and random networks with a similar structure (i.e., same number of nodes with the same number of input interactions for each node). For each BN, 100 randomly created networks were generated using the package BoolNet in R (Müssel et al., 2010). Afterwards, the same bitflip alterations were made to these randomly created BNs and the mean number of perturbations without changes in the attractors in these 100 networks was compared with the total amount of perturbations without changes obtained in the original BN.
2.9 Biological data and codes
The biological experimental data employed to construct both the Base and Minimum Networks is detailed in Supplementary Material Tables S1, S2. Information related to the expression patterns of the Minimum Network molecules, used to define the expected attractors, is available in Supplementary Material Table S3. Additionally, the experimental details and references used to define the mutant simulations can be found in Supplementary Material Table S4.
All the project codes, including those used for the Griffin search, mutant simulations, and robustness analysis, can be accessed at https://github.com/marianayuste/Polaridad.git.
3 Results
A flowchart with an overview of the methodological process employed in this study can be found in Figure 2.
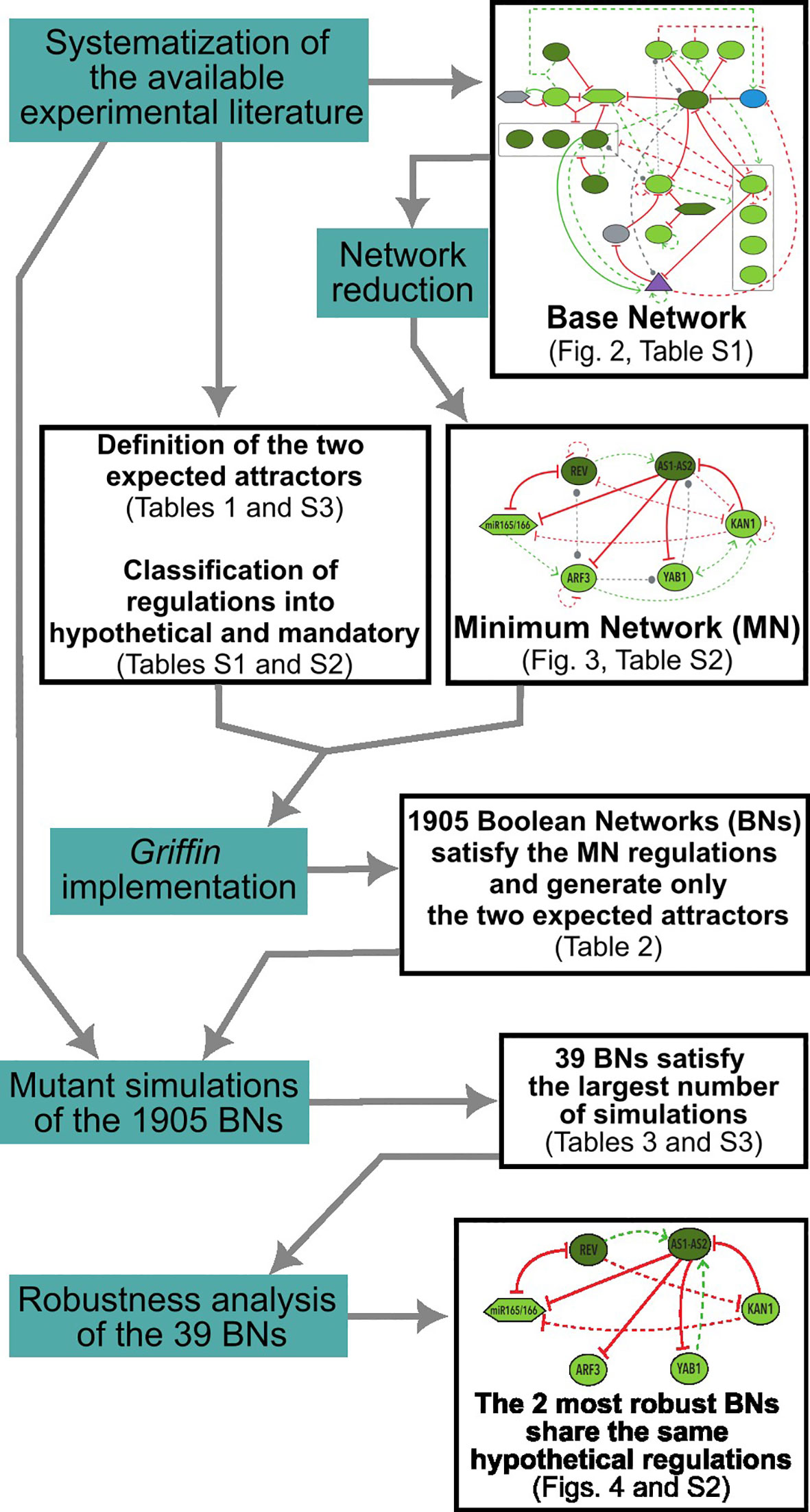
Figure 2 Flowchart illustrating the methodological process employed in this study. Methodological actions are highlighted in blue, while the corresponding results are presented in black boxes. Note that some of the output results served as inputs for subsequent actions.
3.1 The base network
The Base Network incorporate all genes, microRNAs and hormones that appear to have an important role in the abaxial-adaxial polarity determination in leaf primordia of A. thaliana, as well as their regulations (Figure 3). Supplementary Material Table S1 in Supplementary Material provides a listing of all the regulations in the Base Network, along with their corresponding references. The regulations represent the activation or inhibition (also called positive or negative regulation, respectively) of a regulatory node towards the target node. Based on the experimental evidence analyzed, we defined two types of regulations: mandatory and hypothetical. Mandatory regulations must be experimentally confirmed as a physical direct regulation between two molecules (by analyses such as Double Hybrid Assay, Chromatin Immunoprecipitation or Co-immunoprecipitation) and have enough empirical data to support if such interaction is an activation or an inhibition (by mutant analyses). Hypothetical regulations have experimental results that suggest an interaction between the two nodes but is not enough to confirm it (more details in the Methods section). The Base Network includes 22 molecules/nodes and 52 regulations, including 22 mandatory and 30 hypothetical regulations (Figure 3, Supplementary Material Table S1).
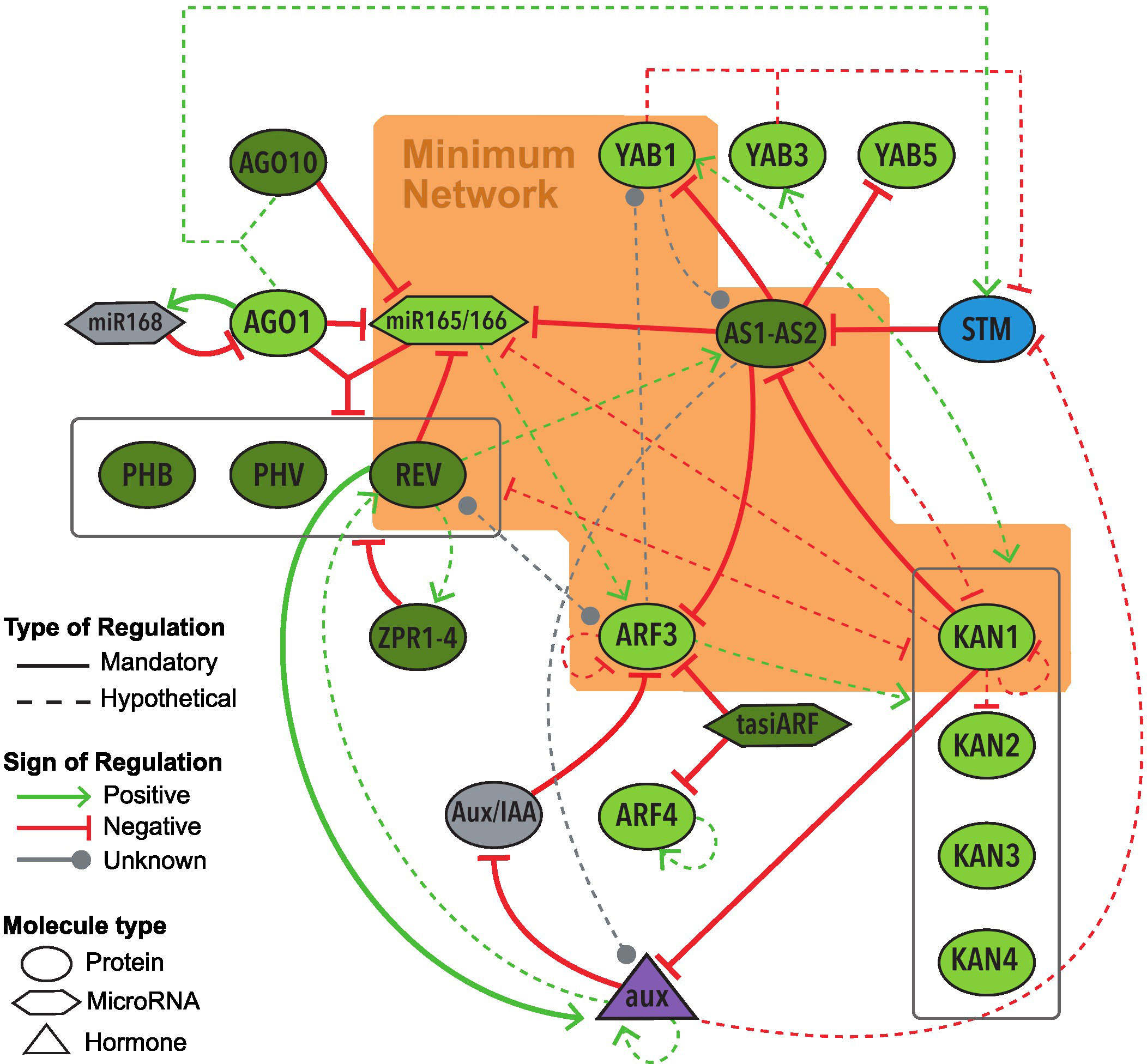
Figure 3 Graphic representation of the Base Network of the GRN underlying the abaxial-adaxial polarity on leaves of Arabidopsis thaliana. The nodes inside the orange polygon form the MN. Node colors depict their expression on the leaf primordium: dark green for adaxial expression, light green abaxial, blue meristematic expression, purple expression on the whole primordium, gray unknown expression. All regulations included in the Base Network, along with their respective references, are detailed in Supplementary Material Tables S1.
With the exception of STM, all of the molecules of the Base Network show expression on the leaf primordium during its early stages (Lynn et al., 1999; Eshed et al., 2001; Kerstetter et al., 2001; Kidner and Martienssen, 2004; Iwakawa et al., 2007; Wenkel et al., 2007; Chitwood et al., 2009; Sarojam et al., 2010). Several of these molecules, including miR165/166 (Kidner and Martienssen, 2004), KAN1 (Caggiano et al., 2017) and REV (Caggiano et al., 2017), also show an expression on the SAM, where the gene STM is being expressed (Lynn et al., 1999). Later on, in the context of the leaf development, adaxial and abaxial genes become restricted to each side. In other words, KANADI, YABBY and ARF genes expression, as well as miRNA165/166, become restricted to the abaxial side, while HD-ZipIII and AS genes expression, as well as tasiR-ARF, to the adaxial side (see Supplementary Material Table S3).
All the nodes of the network represent one molecule except for AS1-AS2, miR165/166, and ZPR1-4. AS1 and AS2 belong to different gene families; AS1 is a myb (SANT) domain protein, while AS2 is part of the AS2/LOB family (Iwakawa et al., 2007; Machida et al., 2015), however, they regulate their targets’ expression as an obligate heterodimer (Iwasaki et al., 2013; Kuhlemeier and Timmermans, 2016). Hence, the two were added in a single node as AS1-AS2. The microRNAs miR165 and miR166 share the same target sequences and their own sequences differ by only one nucleotide (Zhou et al., 2007; Merelo et al., 2016). For this reason, they were included in the same node as miR165/166. The node ZPR1-4 represents four genes from the family LITTLE ZIPPER (ZPR), all of whom codify proteins which join and inhibit HD-ZipIII family genes including PHB, PHV and REV, and who are inhibited by the product of REV (Wenkel et al., 2007; Reinhart et al., 2013). Consequently, these four genes are depicted as one node.
3.2 Reduction of the base network to a minimum network
In order to create a network that contained only the necessary and sufficient elements to produce the abaxial-adaxial polarity we decided to reduce the Base Network to a Minimum Network (MN, Figure 4) by removing all the nodes that do not affect the attractors; these included isolated nodes (i.e., nodes with no mandatory regulations), output nodes (nodes with no target genes considering only their mandatory regulations), mediators (nodes with only one input and one output mandatory regulation) and inputs (nodes with no input regulations) (see Methods for more details). As a result, the MN provides a dynamical behavior that allows us to identify the genes and regulations essential for the apparition of the abaxial-adaxial polarity in the leaf primordium. Supplementary Material Table S2 presents the regulations forming this Minimum Network, along with the experimental evidence that supports each one of them.
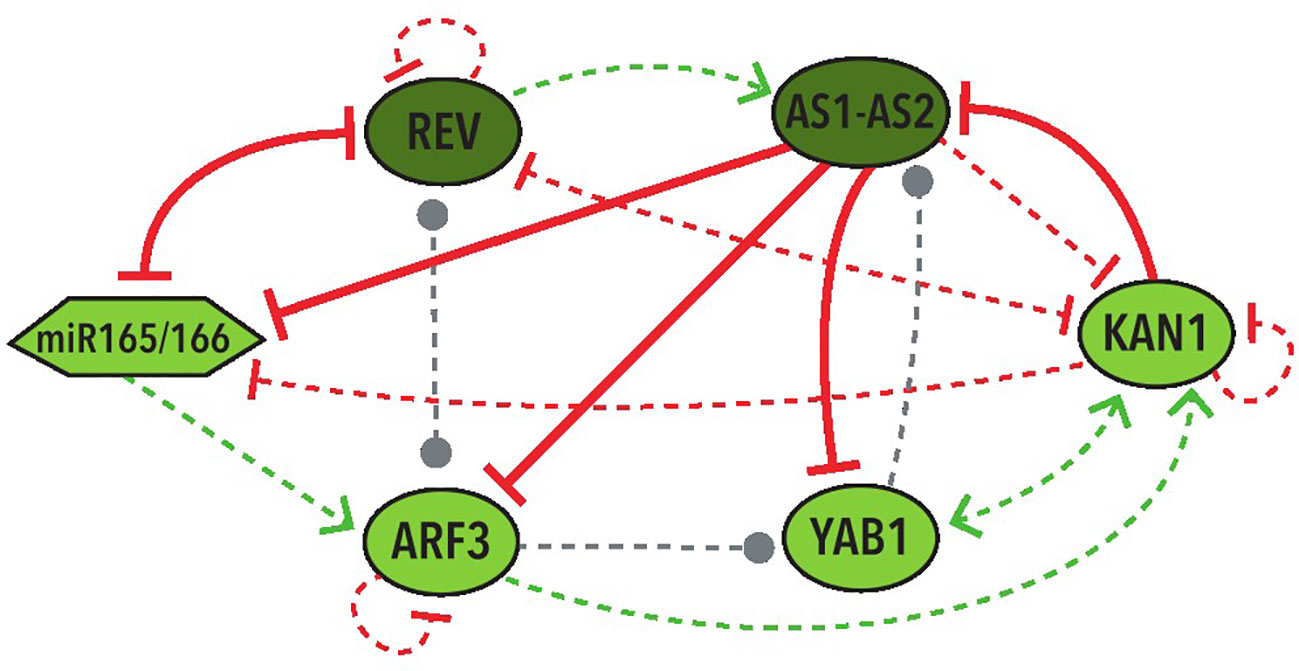
Figure 4 Graphic representation of the Minimum Network (MN). All the nodes and regulations of the MN formed after the different reductions made to the Base Network. A detailed explanation of the biological information supporting each regulation in the MN is provided in Supplementary Material Tables S2. Both hypothetical and mandatory regulations are shown. Colors and shapes of nodes and arrows share the same meaning with the ones in Figure 3.
A couple of exceptions were considered due to their biological relevance. First, even though YAB1 is an isolated node, we maintained it in the MN because it plays an important biological role in the generation of polarity (Siegfried et al., 1999; Eshed et al., 2001; Kumaran et al., 2002; Yamada et al., 2011; Bonaccorso et al., 2012). We expected that keeping YAB1 in the MN would help us inquire if its hypothetical regulations can explain its importance. Second, ARF3 is an output node. However, there is strong experimental evidence that confirms a direct union between ARF3 and YAB1 (García et al., 2006; Trigg et al., 2017). Since there is a lack of experimental information regarding whether the regulation is positive or negative (Tiwari et al., 2003), the ARF3 node was kept in the MN to help us explore which type of regulation from this gene towards YAB1 is supported by the model. Finally, we decided to remove STM and auxin nodes from the MN because they do not seem to be involved in the generation of leaf polarity. While STM has an important role for meristem maintenance, its expression diminishes once the leaf primordium starts to form (Lynn et al., 1999). Auxin is a hormone responsible for the initiation of leaf primordium differentiation and the genetic programs in charge of leaf development, but not necessarily for the establishment of polarity once the leaf starts to develop (Rast and Simon, 2012; Kuhlemeier, 2017; Yu et al., 2017; Conklin et al., 2019).
For the MN to contain positive feedback loops in its regulations, which are necessary for the generation of fixed-point attractors, all the hypothetical regulations between the nodes of the MN were incorporated into it.
As a result, the MN consists of six nodes (five transcription factors and one miRNA), six mandatory regulations and 16 hypothetical regulations. Interestingly, all the experimentally confirmed mandatory regulations are inhibitions. These regulations can be found in Supplementary Material Tables S1, S2. The expression patterns in the leaf primordium of these six molecules can be found in Supplementary Material Table S3. To verify if the MN can explain the emergence of leaf polarity it is necessary to provide a dynamical description of the network. Consequently, this was our next step.
3.3 Numerous Boolean networks satisfy the MN and generate abaxial-adaxial polarity
To describe the dynamics of the MN we used a Boolean networks (BN) approach. It has been established that the same network topology, which is the set of nodes and regulations that conform the network, can be satisfied with several different BNs (Azpeitia et al., 2017). In general, BNs are commonly constructed without any clear criteria to select the logical rules for each node. However, in recent years different methodologies have been proposed for the construction of BNs following clear criteria (Azpeitia et al., 2013; Muñoz et al., 2018; Maheshwari et al., 2022). We decided to implement Griffin, a computational tool that allows the inference of all the different synchronous BNs that satisfy the regulations as well as some biological constraints (Muñoz et al., 2018). We used the two expected attractors as the biological constraint that the BNs must satisfy. The expected attractors of the network correspond to the genetic expression patterns on each side (abaxial and adaxial) of the leaf primordium (Table 1).

Table 1 The two expected attractors in the Minimum Network. The references for these expressions can be found in Supplementary Material Table S3.
In summary, we fed Griffin all the regulations of the MN and the two expected attractors. Griffin, in turn, generated all the BNs composed of these regulations that exclusively produce those attractors. Specifically, these BNs must include all six mandatory regulations of the MN and may or may not include one or more of the hypothetical regulations. We implemented a radial exploration strategy in Griffin, which sets a maximum number of hypothetical regulations to be included. Using Griffin to explore the possible BNs generated by the MN, we allowed a maximum of 4 hypothetical regulations in each network, finding 1905 different BNs that satisfy the regulations of the MN and only generate the two expected attractors. Furthermore, none of the possible BNs with zero or one hypothetical regulation were able to generate only the two expected attractors (Table 2). Consequently, the MN requires two or more hypothetical regulations besides the six mandatory regulations in order to satisfy the biological constraint of generating only the two expected attractors.
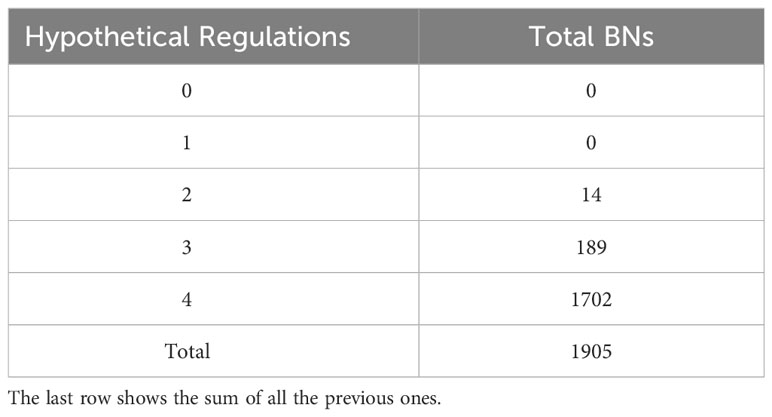
Table 2 Total of Boolean networks (BNs) with each amount of hypothetical regulations that generate only the two expected attractors, obtained from the radial exploration strategy implemented in Griffin.
The most frequent hypothetical regulations in the networks producing the expected attractors are REV→AS1-AS2 (in 61% of the BNs), ARF3→KAN1 (45.4%) and REV⊣KAN1 (41.5%).
3.4 Several BNs satisfy the majority of the mutant simulations
To recognize which of the 1905 BNs found by Griffin give a better representation of the abaxial-adaxial polarity, we executed mutant simulations on each of them. For each mutant simulation, the obtained attractors were compared with the genetic expression patterns reported experimentally (See Supplementary Material Table S4). We compared our mutant simulations against experimental reports describing Expression changes and Cell type changes. The Expression change refers to modifications in gene expression and, in our BN models, do not necessarily modify the attractors. On the other hand, Cell type changes should modify the attractors. Therefore, 14 mutations were simulated on each of the BNs and compared with 8 Expression changes and 10 Cell type changes described in the literature (Table 3). While simulating the mutants, we realized that 1879 of the 1905 BNs generated cyclic attractors in at least one of the simulations. In BNs, cyclic attractors can be an artifact of a synchronous update. Since the asynchronous update allows for each state to have more than one possible successor, it removes possible oscillations (Thomas and D’Ari, 1990). Hence, in order to verify if these attractors were an artifact, we repeated all simulations with an asynchronous update. Indeed, implementing an asynchronous update reduces the BNs with cyclic attractors to 314. Thus, an asynchronous update eliminates the majority of the oscillations.
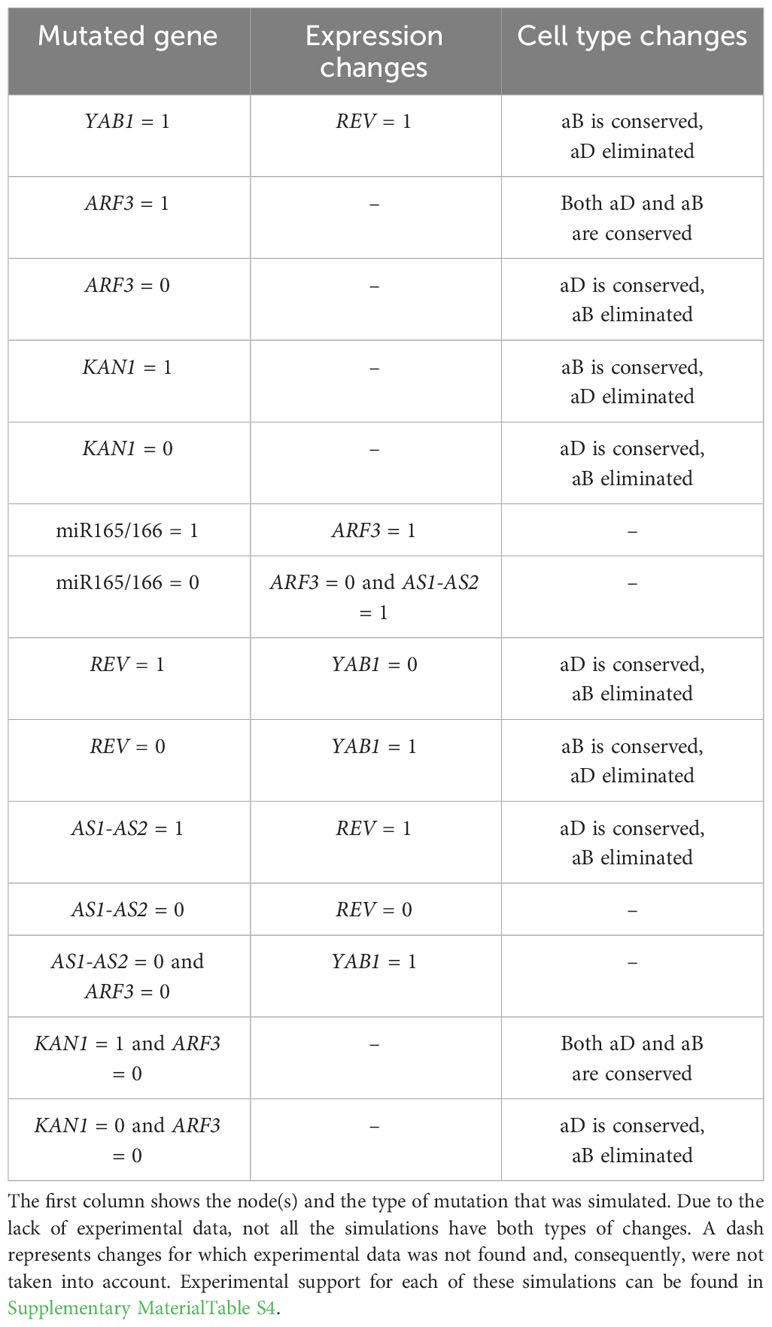
Table 3 Mutant simulations and their Expression and Cell type changes observed in experimental results.
Three BNs satisfy 16 changes, the largest number obtained, but still generate cyclic attractors even with an asynchronous update. That is why we also considered the 36 BNs that satisfy 15 changes and do not generate any cyclic attractor in the asynchronous update. With this approach, we obtained 39 BNs that satisfy the largest number of changes. Interestingly, all 39 selected BNs share the hypothetical regulation of activation from REV to AS1-AS2 (the regulation graphs of the selected BNs are shown in Supplementary Material Figure S2). Moreover, we found that none of these BNs satisfy the simulation of the gain-of-function of YAB1 (YAB1 = 1) nor the simulation of the double mutant kan1 arf3 (KAN1 = 1 and ARF3 = 0). We realized that fulfilling these simulations is impossible because experiments report that having a gain of function of KAN1 and a loss of function of ARF3 produce the appearance of both phenotypes (Pekker et al., 2005). However, this generated a contradiction with the expected attractors where KAN1 must be 0 in the adaxial attractor and ARF3 must be 1 in the abaxial attractor.
To analyze if any of these 39 BNs dynamic is more similar to what is biologically observed, we analyzed their robustness, since biological GRN are usually highly robust to perturbations (Morohashi et al., 2002).
3.5 Robustness of the BNs
In the 39 BNs recovered from the Griffin analysis we performed systemic alterations to the logical rules of each BN using a bitflip method and found that two BNs have the largest percentage (33%) of perturbations that keep the original attractors without any change. We then looked at the represented cell types of the attractors obtained from these perturbations. We discovered that, by allowing changes in the expression of the nodes with an Undefined state, the same 2 BNs keep the two abaxial and adaxial attractors in the most perturbations, both counting the perturbations that generate only the two expected attractors (37.5%) and the ones that generate new attractors besides the two expected ones (37.5%). These two BNs share the same network topology with the same 4 hypothetical regulations: REV→ AS1-AS2, YAB1→AS1-AS2, REV⊣KAN1, KAN1⊣miR165/166 (Figure 5).
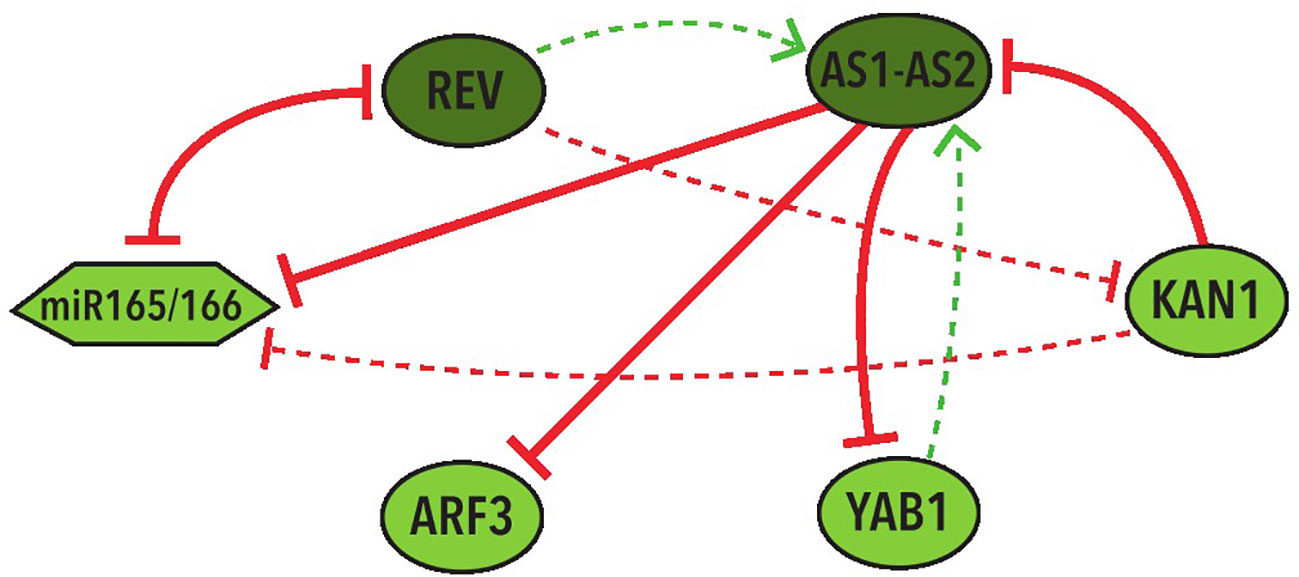
Figure 5 Graphic representation of the shared network topology between the two BNs that obtained more robustness. Colors and shapes of nodes and arrows share the same meaning with the ones in Figure 3.
By comparing the robustness of the 39 BNs with randomly generated networks, we found that 32 of them, including the two BNs illustrated in Figure 5, are more robust than the average of their 100 random networks. In the BN with the largest difference between these two percentages, 31.8% of its perturbations keep the original attractors, in comparison with the 18.9% (12.8 SD) on average of the perturbations that keep the original attractors in its 100 random networks. These results indicate that the model is more robust than what would be expected from BNs with similar structure to the MN but with random regulations between the nodes.
4 Discussion
Refer to Figure 2 for a visual guide to the methodological actions and results undertaken during the construction of our model.
4.1 Abaxial and adaxial attractors can be generated by the MN
Abaxial-adaxial polarity is a well conserved trait among leaves of vascular plants (Fukushima and Hasebe, 2014; Tsukaya, 2014). It has been proposed that the different tissues generated on each side of a polarized leaf promote a higher photosynthetic efficiency (Kuhlemeier and Timmermans, 2016) and enable the control, to an extent, of water loss during gas exchange (Sack and Buckley, 2016). Furthermore, once the abaxial-adaxial polarity is established in the leaf primordium, it will promote lateral growth (Qi et al., 2017), creating a flat leaf with bilateral and dorsiventral symmetry. For these reasons the molecular bases underlying leaf development have been extensively studied. However, until now, it is still unclear if the molecular components documented thus far in A. thaliana are sufficient to explain the establishment of adaxial-abaxial polarity in its leaves.
In this work we provide a model of the Gene Regulatory Network involved in the formation of the abaxial-adaxial polarity in the initial stages of differentiation of a leaf primordium. To this end, we integrated the available experimental data from A. thaliana into a Base Network that included all genes and other molecules that exhibit a role in the generation of abaxial-adaxial polarity. After reducing this initial network by removing nodes that do not affect the attractors of the network, we end up with a Minimum Network which was then analyzed using a BN formalism to describe its dynamic. We found that the nodes and regulations comprising the Minimum Network can generate the abaxial-adaxial attractors, while also recovering most of the mutant phenotypes that have been previously documented experimentally.
4.2 Biological implications of the model
This study proposes the nodes of the MN as the main genetical actors behind abaxial-adaxial polarity generation in A. thaliana leaf primordium. It also predicts some of the regulations within the MN as the mechanisms governing this trait (discussed below in this section). While our model provides valuable theoretical insights, we emphasize that the results need experimental validation in order to corroborate the biological significance of the predictions.
4.2.1 Hypothetical regulations were needed in the model
The regulations within the network were characterized not only by their positive or negative sign but also by their experimental evidence: mandatory regulations are validated as a direct physical regulation between the two molecules by one or more experimental results. In contrast, hypothetical regulations have a less robust experimental support (additional details in the Methods section). During model analyses, the presence of all mandatory regulations in the BN is mandatory, whereas hypothetical regulations may or may not be included. The values of each node in an attractor can be interpreted as their expression inside the cell, meaning each attractor can be considered a cell type (Kauffman, 1969). In this model we looked for the generation of two fixed-point attractors representing the abaxial and the adaxial cells in the leaf primordium. In order to generate multiple fixed-point attractors, a BN must contain positive feedback loops in its regulations (Thomas and D’Ari, 1990). Considering only the mandatory regulations we could derive from the analysis of the experimental data, neither the Base Network nor the MN contain this type of motifs, thus, we incorporated several hypothetical regulations to the analysis.
4.2.2 1905 different BNs produce the abaxial-adaxial attractors
Once different hypothetical regulations were introduced into the MN, we found that a variety of MN topologies contain the required positive feedback loops. We used the computational tool Griffin for network analysis, which further confirmed that such hypothetical regulations are required to generate the two expected attractors, as all networks that recover these two attractors need at least two hypothetical regulations. An exploration of all the possible BNs generated by adding up to four hypothetical regulations give a total of 1905 BNs that only generate the abaxial and adaxial attractors. This large number of possible networks suggests that there is experimental data and interactions that have yet to be tested and described. It is possible that such interactions, once incorporated into our model, could reduce the amount of BNs that recover the desired attractors. However, some works show the opposite result, where an increase on the biological information available for a network model does not necessarily reduce the possible BNs. For example, the GRN involved with flower development can produce, at least, hundreds of thousands of BNs that generate the expected attractors from the network structure, despite the fact that it has been updated multiple times and that it contains a much larger amount of experimental information (Dinh et al., 2017; Muñoz et al., 2018). An alternative interpretation of our result could be that the amount of BNs and hypothetical regulations that can recover the two expected attractors are a quality of the system to be robust and evolvable at the same time. In fact, theoretical work has shown that highly robust and evolvable networks can produce the same behavior despite multiple modifications (Wagner, 2008). This could be the case of leaf polarity, which seems to be evolutionary robust because it is present in a huge number of plant lineages, but which also seems to be highly evolvable due to the implications it has over the establishment of other foliar characteristics, including laminarity and lateral growth (Huang et al., 2014; Sablowski, 2015). It has been observed that alterations on the abaxial-adaxial establishment mechanisms cause dramatic modifications on leaf morphology, such as the generation of cylindrical leaves on some monocots, the union of the petiole at the center of the lamina, or the emergence of ectopic laminar structures (Yamaguchi et al., 2012; Fukushima and Hasebe, 2014), making abaxial-adaxial polarity an important starting point for leaf diversity.
4.2.3 Biological relevance of the hypothetical regulations
It is important to stress that the most repeated hypothetical regulations in these networks are three positive and one negative: REV→AS1-AS2, ARF3→KAN1, REV⊣KAN1, and YAB1→KAN1. Two of them were included in the regulations of the two most robust BNs (Figure 5). Furthermore, each one of these regulations generate the positive feedback loop required for the establishment of the two fixed-point stable attractors in the MN.
The mandatory regulations we were able to infer from experimental data are composed of negative regulations between components that are expressed on opposite sides of the developing leaf primordium, representing the mutual inhibition between the abaxial and adaxial sides that enables the establishment of a polarized leaf. Several experimental studies had proposed that these antagonistic regulations are what allow the separation between the two sides of the primordium (Bowman et al., 2002; Liu et al., 2011; Yamaguchi et al., 2012). However, we show in this work that the missing positive feedback loops enable coupling among components of the same side and that the activation between genes on the same side is a key factor in the establishment and maintenance of polarity. Further investigations focused on these four currently hypothetical regulations are essential for a more comprehensive understanding of the mechanisms underlying polarity generation and maintenance in the leaf primordium.
Other regulations of particular importance for further investigation are the four hypothetical regulations that are present in the two most robust networks (Figure 5). These include two of the most repeated regulations (REV→AS1-AS2 and REV⊣KAN1) as well as two additional hypothetical regulations: YAB1→AS1-AS2 and KAN1⊣miR165/166.
More than half of the BNs obtained in the radial exploration using Griffin exhibit one or both of the hypothetical regulations generated by YAB1, putting into question whether keeping YAB1 in the network made these regulations necessary to fulfill its expected values. Yet, nearly half of the BNs lack regulations generated by YAB1, confirming that these regulations are not necessary to achieve the expected values for this gene. In addition to testing the two hypothetical regulations of YAB1 in the laboratory, our results suggest that the apparent biological importance of YAB1 in the generation of abaxial-adaxial polarity is indeed due to the regulations it generates towards other genes. The experimental literature describing the regulation of YAB1 towards the AS1-AS2 heterodimer has conflicting findings, with some experimental results suggesting this interaction to be a positive regulation (Bonaccorso et al., 2012), while other experiments suggest it to be a negative regulation (Lin et al., 2003; Bonaccorso et al., 2012), and in yet other papers no regulation between these genes was observed (Kumaran et al., 2002; Bonaccorso et al., 2012). Interestingly, in a biomathematical study by La Rota et al. (2011) where they modeled abaxial-adaxial polarity in A. thaliana sepal primordia - an organ which is akin to a specialized leaf that is part of the flower - the YAB1 to AS1 regulation was not maintained in any of the found solutions. However, these authors proposed that this regulation is an activation and likely occurs only in early stages of sepal primordia, where co-expression of these two genes has been observed. Consistent with this inference, our study found this regulation in numerous BNs only as an activation, in agreement with the description of YAB1 as an activator by Bonaccorso et al. (2012) and supporting the hypothesis that its relative importance decreases during development due to the change of expression of each gene towards the abaxial or adaxial side. Thus, by allowing the expression of YAB1 in both attractors and not necessarily having this change in the models, its expression is maintained.
In the case of ARF3, it remained as an output node in the two most robust models recovered here. This result could potentially be attributed to the absence of auxin and tasiARF in the MN, which are the two primary regulators of ARF3 (Tiwari et al., 2003; Chitwood et al., 2009).
The node with the highest number of both incoming and outgoing regulations in the two most robust BNs is AS1-AS2. This node also exhibits the highest number of mandatory regulations in both the Base Network and the MN. This could be attributed to it representing a heterodimer composed by two distinct gene products, thus combining the available information from both into a single node. Nonetheless, this dimer plays a significant role during leaf primordium development as it holds important regulations in the genetic network behind abaxial-adaxial polarity, in addition to the constant repression it maintains towards several KNOX genes (Husbands et al., 2015).
4.3 Mathematical and computational implications of the model
BNs have been used for many decades to study molecular dynamics of biological systems (Schwab et al., 2020). Initially, BNs were based on random network graphs with random Boolean functions. However, in the last decades, the graphs have been successfully constructed using experimental data in many systems, such as plant (Espinosa-Soto et al., 2004; García-Gómez et al., 2017; Maheshwari et al., 2019), animal (Weinstein and Mendoza, 2013 and Weinstein et al., 2020), and human (Méndez-López et al., 2017) development, diseases (Herrmann et al., 2012; Dahlhaus et al., 2016; Rodríguez et al., 2019), aging (Siegle et al., 2018), among many other biological processes (e.g., Ayala-Zambrano et al., 2023). However, with a notable exception (Raeymaekers, 2002), it had not been until recently that efforts to standardize the formalization of biological data into Boolean functions started appearing in the literature (e.g., Azpeitia et al., 2013; Dinh et al., 2017; Muñoz et al., 2018; Maheshwari et al., 2022). Here, we used Griffin (Muñoz et al., 2018), as it enables a systematic exploration of all possible synchronous BNs that produce a desired set of attractors and whose Boolean functions respect all known experimental data. Thus, Griffin is a powerful tool to infer the Boolean functions of a GRN. Part of its power rests on the fact that it assumes that GRNs are updated synchronously. While synchronicity is considered an unrealistic assumption in Boolean networks (Thomas and D’Ari, 1990), the fixed-point attractors produced by any GRN, such as the attractors representing the abaxial-adaxial polarity, are the same under a synchronous and an asynchronous update. Hence, the update regime did not affect our main results. Moreover, it allows us to use Griffin to analyze the possible role of important players in abaxial-adaxial polarity, such as YAB1 and ARF3, and to include missing information about their regulations (hypothetical regulations) and attractors (genes whose state is not yet known in an attractor). It is important to note that in the mutant analyses we did use an asynchronous update to eliminate cyclic attractors produced by the synchronous update. Hence, we believe that a combination of regimes (synchronous and asynchronous) and methods to formalize biological data into Boolean functions (e.g., Muñoz et al., 2018 with Maheshwari et al., 2022) is a promising avenue to improve the construction of BNs based on biological modules.
4.4 Robustness of the model
Abaxial-adaxial leaf polarity is a strongly conserved trait among vascular plants (Kuhlemeier and Timmermans, 2016), and the expression and function of several of the genes behind it are also conserved throughout their different homologs (Husbands et al., 2009). This suggests that leaf polarity is robust to various evolutionary changes and molecular perturbations.
Bitflip perturbations were performed on each of the BNs with the highest number of fulfilled mutants. It was found that in two of these BNs, 54.17% of the perturbations maintain the abaxial and adaxial cell types, which represents the highest percentage achieved. This shows less robustness than what we would expect from a model of this trait, especially when compared with the robustness obtained in other biological network models (e.g., Sánchez-Corrales et al., 2010; Ortiz-Gutiérrez et al., 2015).
Previous studies, such as Morohashi et al. (2002) investigating the cell cycle on Xenopus embryos, or Benítez and Alvarez-Buylla (2010) examining GRN models of trichomes patterns on A. thaliana epidermis, have shown that different models generate congruent results with the experimental information, but robustness to perturbations is higher in models with more complex structures, including a larger number of components in their feedback loops. By reducing the Base Network to a MN of 6 nodes, the network structures are small, likely reducing the network’s robustness. The two cell types can be generated, but the model is fragile to perturbations. Therefore, the MN is composed of the genes that form the network’s core responsible for polarity, but they are not enough to explain the robustness of this biological system. Also, in order to reduce functional redundancy, we removed or merged together into a single representative node, genes of the same family with similar function as redundancy has been observed in KANADIs (Eshed et al., 2001; Eshed et al., 2004), ARF3 and ARF4 (Pekker et al., 2005), HD-ZipIII (PHB, PHV, and REV) (Emery et al., 2003) and YABBY genes (Siegfried et al., 1999; Kumaran et al., 2002). Removing these nodes simplified the network and reduced functional redundancy, which is a key mechanism promoting system robustness (Kitano, 2004). Another phenomenon that could underlie the low robustness could potentially be attributed to missing components or regulations within the network, which have yet to be experimentally identified.
However, the discovery of 1905 distinct BNs that exclusively produce the two abaxial and adaxial attractors suggests some robustness of the model as a whole. By existing so many possible BNs with this capacity, it is implied that functional variations in the genes exist, in a Boolean rule level, which could represent different alleles. In other words, from an evolutionary perspective, there might be allelic variations in the network’s genes that do not modify the required attractors to produce the abaxial-adaxial polarity.
5 Conclusion
Here we show that to explain the appearance of the expected attractors of leaf polarity based on the current knowledge it is necessary to include hypothetical regulations. Once hypothetical regulations are considered, there is a large number of networks that produce the expected steady states. This result suggests that either there is still important missing information regarding the genes and the regulatory interactions producing leaf polarity in A. thaliana, or that multiple networks have evolved with the same capacity, which might give evolutionary robustness and evolvability to polarity, or both. Future studies on A. thaliana and other species will aid to solve this issue. Through mutant simulations and robustness’ analyses we also showed that there are a few networks that reproduce more experimental observations with a higher robustness. Interestingly, these networks are similar in the sense that they all share a set of hypothetical regulations. We believe that due to their similarity, these networks could be used as a guide to explore putative missing information. In any case, our work provides a first attempt to integrate the current knowledge about the development of leaf polarity and opens new questions that could be used to direct future experimental studies.
Data availability statement
The original contributions presented in the study are included in the article/Supplementary Material. Further inquiries can be directed to the corresponding authors.
Author contributions
MY: Conceptualization, Data curation, Formal analysis, Investigation, Methodology, Visualization, Writing – original draft. AP-N: Conceptualization, Investigation, Methodology, Project administration, Supervision, Validation, Writing – review & editing. EA: Conceptualization, Investigation, Methodology, Project administration, Supervision, Validation, Writing – review & editing.
Funding
The author(s) declare financial support was received for the research, authorship, and/or publication of this article. MY received a scholarship from the Consejo Nacional de Humanidades, Ciencias y Tecnologías (CONAHCyT), Mexico. CVU 859893. Part of AP-N’s work was funded with grant A1-S-43879 from CONAHCyT.
Acknowledgments
We acknowledge Elena Álvarez-Buylla for her input during the generation of the original idea for this research, as well as for her valuable feedback in early stages of this work. We also acknowledge Lynna Kiere for her assistance in the final proofreading.
Conflict of interest
The authors declare that the research was conducted in the absence of any commercial or financial relationships that could be construed as a potential conflict of interest.
Publisher’s note
All claims expressed in this article are solely those of the authors and do not necessarily represent those of their affiliated organizations, or those of the publisher, the editors and the reviewers. Any product that may be evaluated in this article, or claim that may be made by its manufacturer, is not guaranteed or endorsed by the publisher.
Supplementary material
The Supplementary Material for this article can be found online at: https://www.frontiersin.org/articles/10.3389/fevo.2024.1330827/full#supplementary-material
References
Abou-Jaoudé W., Traynard P., Monteiro P. T., Saez-Rodriguez J., Helikar T., Thieffry D., et al. (2016). Logical modeling and dynamical analysis of cellular networks. Front. Genet. 7. doi: 10.3389/fgene.2016.00094
Allen E., Xie Z., Gustafson A. M., Carrington J. C. (2005). microRNA-directed phasing during trans-acting siRNA biogenesis in plants. Cell 121, 207–221. doi: 10.1016/j.cell.2005.04.004
Ayala-Zambrano C., Yuste M., Frias S., Garcia-de-Teresa B., Mendoza L., Azpeitia E., et al. (2023). A Boolean network model of the double-strand break repair pathway choice. J. Theor. Biol. 573, 111608. doi: 10.1016/j.jtbi.2023.111608
Azpeitia E., Davila-Velderrain J., Villarreal C., Alvarez-Buylla E. R. (2014). “Gene Regulatory Network Models for Floral Organ Determination,” in Flower Development: Methods and Protocols, Methods in Molecular Biology. Eds. Riechmann J. L., Wellmer F. (New York, NY: Springer), 441–469. doi: 10.1007/978-1-4614-9408-9_26
Azpeitia E., Muñoz S., González-Tokman D., Martínez-Sánchez M. E., Weinstein N., Naldi A., et al. (2017). The combination of the functionalities of feedback circuits is determinant for the attractors’ number and size in pathway-like Boolean networks. Sci. Rep. 7. doi: 10.1038/srep42023
Azpeitia E., Weinstein N., Benítez M., Mendoza L., Azpeitia E., Alvarez-Buylla E. R. (2013). Finding missing interactions of the arabidopsis thaliana root stem cell niche gene regulatory network. Front. Plant Sci. 4. doi: 10.3389/fpls.2013.00110
Benítez M., Alvarez-Buylla E. R. (2010). Dynamic-module redundancy confers robustness to the gene regulatory network involved in hair patterning of Arabidopsis epidermis. BioSystems 102, 11–15. doi: 10.1016/j.biosystems.2010.07.007
Bonaccorso O., Lee J. E., Puah L., Scutt C. P., Golz J. F. (2012). FILAMENTOUS FLOWER controls lateral organ development by acting as both an activator and a repressor. BMC Plant Biol. 12, 176. doi: 10.1186/1471-2229-12-176
Bowman J. L., Eshed Y., Baum S. F. (2002). Establishment of polarity in angiosperm lateral organs. Trends Genet. 18, 134–141. doi: 10.1016/S0168-9525(01)02601-4
Brandt R., Salla-Martret M., Bou-Torrent J., Musielak T., Stahl M., Lanz C., et al. (2012). Genome-wide binding-site analysis of REVOLUTA reveals a link between leaf patterning and light-mediated growth responses. Plant J. 72, 31–42. doi: 10.1111/j.1365-313X.2012.05049.x
Byrne M. E., Barley R., Curtis M., Arroyo J. M., Dunham M., Hudson A., et al. (2000). Asymmetric leaves1 mediates leaf patterning and stem cell function in Arabidopsis. Nature 408, 967–971. doi: 10.1038/35050091
Caggiano M. P., Yu X., Bhatia N., Larsson A., Ram H., Ohno C. K., et al. (2017). Cell type boundaries organize plant development. eLife 6, 1–32. doi: 10.7554/eLife.27421
Chitwood D. H., Nogueira F. T. S., Howell M. D., Montgomery T. A., Carrington J. C., Timmermans M. C. P. (2009). Pattern formation via small RNA mobility. Genes Dev. 23, 549–554. doi: 10.1101/gad.1770009.contribute
Comet J. P., Noual M., Richard A., Aracena J., Calzone L., Demongeot J., et al. (2013). On circuit functionality in boolean networks. Bull. Math. Biol. 75, 906–919. doi: 10.1007/s11538-013-9829-2
Conklin P. A., Strable J., Li S., Scanlon M. J. (2019). On the mechanisms of development in monocot and eudicot leaves. New Phytol. 221, 706–724. doi: 10.1111/nph.15371
Dahlhaus M., Burkovski A., Hertwig F., Mussel C., Volland R., Fischer M., et al. (2016). Boolean modeling identifies Greatwall/MASTL as an important regulator in the AURKA network of neuroblastoma. Cancer Lett. 371, 79–89. doi: 10.1016/j.canlet.2015.11.025
Dinh J.-L., Farcot E., Hodgman C. (2017). The logic of the floral transition: Reverse-engineering the switch controlling the identity of lateral organs. PloS Comput. Biol. 13, e1005744. doi: 10.1371/journal.pcbi.1005744
Dkhar J., Pareek A. (2014). What determines a leaf’s shape? EvoDevo. 5, 47. doi: 10.1186/2041-9139-5-47
Emery J. F., Floyd S. K., Alvarez J., Eshed Y., Hawker N. P., Izhaki A., et al. (2003). Radial patterning of arabidopsis shoots by class III HD-ZIP and KANADI genes. Curr. Biol. 13, 1768–1774. doi: 10.1016/j.cub.2003.09.035
Eshed Y., Baum S. F., Perea J. V., Bowman J. L. (2001). Establishment of polarity in lateral organs of plants. Curr. Biol. 11, 1251–1260. doi: 10.1016/s0960-9822(01)00392-x
Eshed Y., Izhaki A., Baum S. F., Floyd S. K., Bowman J. L. (2004). Asymmetric leaf development and blade expansion in Arabidopsis are mediated by KANADI and YABBY activities. Development 131, 2997–3006. doi: 10.1242/dev.01186
Espinosa-Soto C., Padilla-Longoria P., Alvarez-Buylla E. R. (2004). A gene regulatory network model for cell-fate determination during Arabidopsis thalianal flower development that is robust and recovers experimental gene expression profiles. Plant Cell 16, 2923–2939. doi: 10.1105/tpc.104.021725.2
Fukushima K., Hasebe M. (2014). Adaxial-abaxial polarity: The developmental basis of leaf shape diversity. Genesis 52, 1–18. doi: 10.1002/dvg.22728
García R., Collier S. A., Byrne M. E., Martienssen R. A. (2006). Specification of leaf polarity in arabidopsis via the trans-acting siRNA pathway. Current Biol. 16 (9), 933–938. doi: 10.1017/CBO9781107415324.004
García-Gómez M. L., Azpeitia E., Álvarez-Buylla E. R. (2017). A dynamic genetic-hormonal regulatory network model explains multiple cellular behaviors of the root apical meristem of Arabidopsis thalian. PloS Comput. Biol. 13 (4). doi: 10.1371/journal.pcbi.1005488
Harvey I., Bossomaier T. (1997). “Time out of joint: Attractors in asynchronous random boolean networks,” in Proceedings of the Fourth European Conference on Artificial Life. (Cambridge: MIT Press), 67–75.
Hay A., Kaur H., Phillips A., Hedden P., Hake S., Tsiantis M. (2002). The gibberellin pathway mediates KNOTTED1-type homeobox function in plants with different body plans. Curr. Biol. 12, 1557–1565. doi: 10.1016/S0960-9822(02)01125-9
Heisler M. G., Ohno C., Das P., Sieber P., Reddy G. V., Long J. A., et al. (2005). Patterns of auxin transport and gene expression during primordium development revealed by live imaging of the Arabidopsis inflorescence meristem. Curr. Biol. 15, 1899–1911. doi: 10.1016/j.cub.2005.09.052
Herrmann F., Groß A., Zhou D., Kestler H. A., Kühl M. (2012). A boolean model of the cardiac gene regulatory network determining first and second heart field identity. PloS One 7, e46798. doi: 10.1371/journal.pone.0046798
Huang T., Harrar Y., Lin C., Reinhart B., Newell N. R., Talavera-Rauh F., et al. (2014). Arabidopsis KANADI1 acts as a transcriptional repressor by interacting with a specific cis-element and regulates auxin biosynthesis, transport, and signaling in opposition to HD-ZIPIII factors. Plant Cell 26, 246–262. doi: 10.1105/tpc.113.111526
Husbands A. Y., Aggarwal V., Ha T., Timmermans M. C. P. (2016). In planta single-molecule pull-down reveals tetrameric stoichiometry of HD-ZIPIII : LITTLE ZIPPER complexes. Plant Cell 28, 1783–1794. doi: 10.1105/tpc.16.00289
Husbands A. Y., Benkovics A. H., Nogueira F. T. S., Lodha M., Timmermans M. C. P. (2015). The ASYMMETRIC LEAVES complex employs multiple modes of regulation to affect adaxial-abaxial patterning and leaf complexity. Plant Cell 27, 3321–3335. doi: 10.1105/tpc.15.00454
Husbands A. Y., Chitwood D. H., Plavskin Y., Timmermans M. C. P. (2009). Signals and prepatterns: New insights into organ polarity in plants. Genes Dev. 23, 1986–1997. doi: 10.1101/gad.1819909
Iwakawa H., Iwasaki M., Kojima S., Ueno Y., Soma T., Tanaka H., et al. (2007). Expression of the ASYMMETRIC LEAVES2 gene in the adaxial domain of Arabidopsis leaves represses cell proliferation in this domain and is critical for the development of properly expanded leaves. Plant J. 51, 173–184. doi: 10.1111/j.1365-313X.2007.03132.x
Iwasaki M., Takahashi H., Iwakawa H., Nakagawa A., Ishikawa T., Tanaka H., et al. (2013). Dual regulation of ETTIN (ARF3) gene expression by AS1-AS2, which maintains the DNA methylation level, is involved in stabilization of leaf adaxial-abaxial partitioning in Arabidopsis. Development 140, 1958–1969. doi: 10.1242/dev.085365
Kalve S., De Vos D., Beemster G. T. S. (2014). Leaf development: A cellular perspective. Front. Plant Sci. 5. doi: 10.3389/fpls.2014.00362
Kauffman S. A. (1969). Metabolic stability and epigenesis in randomly constructed genetic nets. J. Theor. Biol. 22, 437–467. doi: 10.1016/0022-5193(69)90015-0
Kerstetter R. A., Bollman K., Taylor R. A., Bomblies K., Poethig R. S. (2001). KANADI regulates organ polarity in Arabidopsis. Nature 411, 706–709. doi: 10.1038/35079629
Kidner C. A., Martienssen R. A. (2004). Spatially restricted microRNA directs leaf polarity through ARGONAUTE1. Nature 428, 81–84. doi: 10.1038/nature02366
Kidner C. A., Martienssen R. A. (2005). The role of ARGONAUTE1 (AGO1) in meristem formation and identity. Dev. Biol. 280, 504–517. doi: 10.1016/j.ydbio.2005.01.031
Kuhlemeier C., Timmermans M. C. P. (2016). The Sussex signal: insights into leaf dorsiventrality. Development 143, 3230–3237. doi: 10.1242/dev.131888
Kumaran M. K., Bowman J. L., Sundaresan V. (2002). YABBY polarity genes mediate the repression of KNOX homeobox genes in arabidopsis. Plant Cell 14, 2761–2770. doi: 10.1105/tpc.004911
La Rota C., Chopard J., Das P., Paindavoine S., Rozier F., Farcot E., et al. (2011). A data-driven integrative model of sepal primordium polarity in arabidopsis. Plant Cell 23, 4318–4333. doi: 10.1105/tpc.111.092619
Li H., Xu L., Wang H., Yuan Z., Cao X., Yang Z., et al. (2005). The putative RNA-dependent RNA polymerase RDR6 acts synergistically with ASYMMETRIC LEAVES1 and 2 to repress BREVIPEDICELLUS and microRNA165/166 in arabidopsis leaf development. Plant Cell Online 17, 2157–2171. doi: 10.1105/tpc.105.033449
Lin W., Shuai B., Springer P. S. (2003). The arabidopsis LATERAL ORGAN BOUNDARIES –domain gene ASYMMETRIC LEAVES2 functions in the repression of KNOX gene expression and in adaxial-abaxial patterning. Plant Cell 15, 2241–2252. doi: 10.1105/tpc.014969.initiation
Liu Z., Jia L., Wang H., He Y. (2011). HYL1 regulates the balance between adaxial and abaxial identity for leaf flattening via miRNA-mediated pathways. J. Exp. Bot. 62, 4367–4381. doi: 10.1093/jxb/err167
Liu Q., Yao X., Pi L., Wang H., Cui X., Huang H. (2009). The ARGONAUTE10 gene modulates shoot apical meristem maintenance and establishment of leaf polarity by repressing miR165/166 in Arabidopsis. Plant J. 58, 27–40. doi: 10.1111/j.1365-313X.2008.03757.x
Lynn K., Fernandez A., Aida M., Sedbrook J., Tasaka M., Masson P., et al. (1999). The PINHEAD/ZWILLE gene acts pleiotropically in Arabidopsis development and has overlapping functions with the ARGONAUTE1 gene. Development 126, 469–481. doi: 10.1242/dev.126.3.469
Machida C., Nakagawa A., Kojima S. (2015). The complex of ASYMMETRIC LEAVES (AS) proteins plays a central role in antagonistic interactions of genes for leaf polarity specification in Arabidopsis. Wiley Interdisciplinary Reviews: Developmental Biol. 4 (6), 655–671. doi: 10.1002/wdev.196
Maheshwari P., Assmann S. M., Albert R. (2022). Inference of a boolean network from causal logic implications. Front. Genet. 13. doi: 10.3389/fgene.2022.836856
Maheshwari P., Du H., Sheen J., Assmann S. M., Albert R. (2019). Model-driven discovery of calcium-related protein-phosphatase inhibition in plant guard cell signaling. PloS Comput. Biol. 15. doi: 10.1371/journal.pcbi.1007429
Méndez-López L. F., Dávila-Velderrain J., Domínguez-Hüttinger E., Enríquez-Olguín C., Martínez-García J., Álvarez-Buylla E. R. (2017). Gene regulatory network underlying the immortalization of epithelial cells. BMC Syst. Biol. 11, 1–15. doi: 10.1186/s12918-017-0393-5
Merelo P., Ram H., Pia Caggiano M., Ohno C., Ott F., Straub D., et al. (2016). Regulation of MIR165/166 by class II and class III homeodomain leucine zipper proteins establishes leaf polarity. Proc. Natl. Acad. Sci. 113, 11973–11978. doi: 10.1073/pnas.1516110113
Merelo P., Xie Y., Brand L., Ott F., Weigel D., Bowman J. L., et al. (2013). Genome-wide identification of KANADI1 target genes. PLoS ONE 8 (10). doi: 10.1371/journal.pone.0077341
Mori F., Mochizuki A. (2017). Expected number of fixed points in boolean networks with arbitrary topology. Phys. Rev. Lett. 119, 1–5. doi: 10.1103/PhysRevLett.119.028301
Morohashi M., Winn A. E., Borisuk M. T., Bolouri H., Doyle J., Kitano H. (2002). Robustness as a measure of plausibility in models of biochemical networks. J. Theor. Biol. 216, 19–30. doi: 10.1006/jtbi.2002.2537
Muñoz S., Carrillo M., Azpeitia E., Rosenblueth D. A. (2018). Griffin: A tool for symbolic inference of synchronous boolean molecular networks. Front. Genet. 9. doi: 10.3389/fgene.2018.00039
Müssel C., Hopfensitz M., Kestler H. A. (2010). BoolNet—an R package for generation, reconstruction and analysis of Boolean networks. Bioinformatics 26, 1378–1380. doi: 10.1093/bioinformatics/btq124
Ori N., Eshed Y., Chuck G., Bowman J. L., Hake S. (2000). Mechanisms that control knox gene expression in the Arabidopsis shoot. Development 127, 5523–5532. doi: 10.1242/dev.127.24.5523
Ortiz-Gutiérrez E., García-Cruz K., Azpeitia E., Castillo A., de la Paz Sánchez M., Álvarez-Buylla E. R. (2015). A dynamic gene regulatory network model that recovers the cyclic behavior of arabidopsis thaliana cell cycle. PloS Comput. Biol. 11, 1–28. doi: 10.1371/journal.pcbi.1004486
Pekker I., Alvarez J. P., Eshed Y. (2005). Auxin response factors mediate arabidopsis organ asymmetry via modulation of KANADI activity. The Plant Cell 17 (11), 2899–2910. doi: 10.1105/tpc.105.034876.1
Qi J., Wang Y., Yu T., Cunha A., Wu B., Vernoux T., et al. (2014). Auxin depletion from leaf primordia contributes to organ patterning. Proc. Natl. Acad. Sci. 111, 18769–18774. doi: 10.1073/pnas.1421878112
Qi J., Wu B., Feng S., Lü S., Guan C., Zhang X., et al. (2017). Mechanical regulation of organ asymmetry in leaves. Nat. Plants 3, 724–733. doi: 10.1038/s41477-017-0008-6
Raeymaekers L. (2002). Dynamics of Boolean networks controlled by biologically meaningful functions. J. Theor. Biol. 218, 331–341. doi: 10.1006/jtbi.2002.3081
Rast M. I., Simon R. (2012). Arabidopsis JAGGED LATERAL ORGANS acts with ASYMMETRIC LEAVES2 to coordinate KNOX and PIN expression in shoot and root meristems. Plant Cell 24, 2917–2933. doi: 10.1105/tpc.112.099978
Reinhardt D., Frenz M., Mandel T., Kuhlemeier C. (2005). Microsurgical and laser ablation analysis of leaf positioning and dorsoventral patterning in tomato 1. doi: 10.1242/dev.01544
Reinhart B. J., Liu T., Newell N. R., Magnani E., Huang T., Kerstetter R., et al. (2013). Establishing a framework for the ad/abaxial regulatory network of arabidopsis: ascertaining targets of class III HOMEODOMAIN LEUCINE ZIPPER and KANADI regulation. The Plant Cell 25 (9), 3228–3249. doi: 10.1105/tpc.113.111518
Richard A., Rossignol G., Comet J. P., Bernot G., Guespin-Michel J., Merieau A. (2012). Boolean models of biosurfactants production in Pseudomonas fluorescens. PloS One 7. doi: 10.1371/journal.pone.0024651
Rodríguez A., Naveja J. J., Torres L., García De Teresa B., Juárez-Figueroa U., Ayala-Zambrano C., et al. (2019). WIP1 contributes to the adaptation of fanconi anemia cells to DNA damage as determined by the regulatory network of the fanconi anemia and checkpoint recovery pathways. Front. Genet. 10. doi: 10.3389/fgene.2019.00411
Saadatpour A., Albert R. (2013). Boolean modeling of biological regulatory networks: A methodology tutorial. Methods 62, 3–12. doi: 10.1016/j.ymeth.2012.10.012
Saadatpour A., Albert I., Albert R. (2010). Attractor analysis of asynchronous Boolean models of signal transduction networks. J. Theor. Biol. 266, 641–656. doi: 10.1016/j.jtbi.2010.07.022
Sablowski R. (2015). Control of patterning, growth, and differentiation by floral organ identity genes. J. Exp. Bot. 66, 1065–1073. doi: 10.1093/jxb/eru514
Sack L., Buckley T. N. (2016). The developmental basis of stomatal density and flux. Plant Physiol. 171, 2358–2363. doi: 10.1104/pp.16.00476
Sánchez-Corrales Y.-E., Álvarez-Buylla E. R., Mendoza L. (2010). The Arabidopsis thaliana flower organ specification gene regulatory network determines a robust differentiation process. J. Theor. Biol. 264, 971–983. doi: 10.1016/j.jtbi.2010.03.006
Sarojam R., Sappl P. G., Goldshmidt A., Efroni I., Floyd S. K., Eshed Y., et al. (2010). Differentiating arabidopsis shoots from leaves by combined YABBY activities. Plant Cell Online 22, 2113–2130. doi: 10.1105/tpc.110.075853
Schwab J. D., Kühlwein S. D., Ikonomi N., Kühl M., Kestler H. A. (2020). Concepts in Boolean network modeling: What do they all mean? Comput. Struct. Biotechnol. J. 18, 571–582. doi: 10.1016/j.csbj.2020.03.001
Siegfried K. R., Eshed Y., Baum S. F., Otsuga D., Drews G. N., Bowman J. L. (1999). Members of the YABBY gene family specify abaxial cell fate in Arabidopsis. Development 126, 4117–4128. doi: 10.1242/dev.126.18.4117
Siegle L., Schwab J. D., Kühlwein S. D., Lausser L., Tümpel S., Pfister A. S., et al. (2018). A Boolean network of the crosstalk between IGF and Wnt signaling in aging satellite cells. PloS One 13, e0195126. doi: 10.1371/journal.pone.0195126
Sussex I. M. (1951). Experiments on the cause of dorsiventrality in Leaves. Nature. 167, 651–652. doi: 10.1038/167651a0
Thieme C. J., Rojas-Triana M., Stecyk E., Schudoma C., Zhang W., Yang L., et al. (2015). Endogenous Arabidopsis messenger RNAs transported to distant tissues. Nat. Plants 1 (15025). doi: 10.1038/nplants.2015.25
Thomas R., D’Ari R. (1990). Biological Feedback (Boca Raton: CRC Press, Inc.). Available at: https://hal.science/hal-00087681.
Tiwari S. B., Hage G., Guilfoyle T. (2003). The roles of auxin response factor domains in auxin-responsive transcription. Plant Cell 15, 533–543. doi: 10.1105/tpc.008417.All
Trigg S. A., Garza R. M., MacWilliams A., Nery J. R., Bartlett A., Castanon R., et al. (2017). CrY2H-seq: A massively multiplexed assay for deep-coverage interactome mapping. Nat. Methods 14, 819–825. doi: 10.1038/nmeth.4343
Tsukaya H. (2014). Comparative leaf development in angiosperms. Curr. Opin. Plant Biol. 17, 103–109. doi: 10.1016/j.pbi.2013.11.012
Veliz-Cuba A. (2011). Reduction of Boolean network models. J. Theor. Biol. 289, 167–172. doi: 10.1016/j.jtbi.2011.08.042
Wagner A. (2008). Robustness and evolvability: A paradox resolved. Proc. R. Soc. B: Biol. Sci. 275, 91–100. doi: 10.1098/rspb.2007.1137
Wall S., Vialet-Chabrand S., Davey P., Van Rie J., Galle A., Cockram J., et al. (2022). Stomata on the abaxial and adaxial leaf surfaces contribute differently to leaf gas exchange and photosynthesis in wheat. New Phytol. 235, 1743–1756. doi: 10.1111/nph.18257
Wang R.-S., Saadatpour A., Albert R. (2012). Boolean modeling in systems biology: an overview of methodology and applications. Phys. Biol. 9, 55001. doi: 10.1088/1478-3975/9/5/055001
Weinstein N., Mendoza L. (2013). A network model for the specification of vulval precursor cells and cell fusion control in Caenorhabditis elegans. Front. Genet. 4. doi: 10.3389/fgene.2013.00112
Weinstein N., Mendoza L., Álvarez-Buylla E. R. (2020). A computational model of the endothelial to mesenchymal transition. Front. Genet. 11. doi: 10.3389/fgene.2020.00040
Wenkel S., Emery J., Hou B.-H., Evans M. M. S., Barton M. K. (2007). A feedback regulatory module formed by LITTLE ZIPPER and HD-ZIPIII genes. Plant Cell Online 19, 3379–3390. doi: 10.1105/tpc.107.055772
Yamada T., Yokota S., Hirayama Y., Imaichi R., Kato M., Gasser C. S. (2011). Ancestral expression patterns and evolutionary diversification of YABBY genes in angiosperms. Plant J. 67, 26–36. doi: 10.1111/j.1365-313X.2011.04570.x
Yamaguchi T., Nukazuka A., Tsukaya H. (2012). Leaf adaxial-abaxial polarity specification and lamina outgrowth: Evolution and development. Plant Cell Physiol. 53, 1180–1194. doi: 10.1093/pcp/pcs074
Yu T., Guan C., Wang J., Sajjad M., Ma L., Jiao Y. (2017). Dynamic patterns of gene expression during leaf initiation. J. Genet. Genomics 44, 599–601. doi: 10.1016/j.jgg.2017.11.001
Zhang X., Yuan Y., Pei Y., Lin S., Tuschl T., Patel D. J., et al (2006). Cucumber mosaic virus-encoded 2b suppressor inhibits Arabidopsis Argonaute1 cleavage activity to counter plant defense. Genes and Development 20, 3255–3268. doi: 10.1101/gad.1495506.hang
Zhou G. K., Kubo M., Zhong R., Demura T., Ye Z. H. (2007). Overexpression of miR165 affects apical meristem formation, organ polarity establishment and vascular development in Arabidopsis. Plant Cell Physiol. 48, 391–404. doi: 10.1093/pcp/pcm008
Keywords: abaxial-adaxial polarity, leaf dorsiventrality, leaf primordium, gene regulatory network, Boolean networks, mathematical model
Citation: Yuste M, Piñeyro-Nelson A and Azpeitia E (2024) A gene regulatory network model that recovers the abaxial-adaxial polarity in Arabidopsis thaliana leaf primordium. Front. Ecol. Evol. 12:1330827. doi: 10.3389/fevo.2024.1330827
Received: 31 October 2023; Accepted: 18 January 2024;
Published: 07 February 2024.
Edited by:
Maria Ina Arnone, Stazione Zoologica Anton Dohrn, ItalyReviewed by:
Roberto Feuda, University of Leicester, United KingdomXiujun Zhang, Chinese Academy of Sciences (CAS), China
Copyright © 2024 Yuste, Piñeyro-Nelson and Azpeitia. This is an open-access article distributed under the terms of the Creative Commons Attribution License (CC BY). The use, distribution or reproduction in other forums is permitted, provided the original author(s) and the copyright owner(s) are credited and that the original publication in this journal is cited, in accordance with accepted academic practice. No use, distribution or reproduction is permitted which does not comply with these terms.
*Correspondence: Alma Piñeyro-Nelson, YWxtYXBpbmV5cm9AZ21haWwuY29t; Eugenio Azpeitia, ZWF6cGVpdGlhQG1hdG1vci51bmFtLm14