- School of Surveying and Land Information Engineering, Henan Polytechnic University, Jiaozuo, China
Alterations in vegetation cover serve as a significant indicator of land ecology. The Shendong Coal Mining Area, being the largest coal base globally, holds significant importance for national energy security. Moreover, it has gained recognition for its environmentally conscious approach to coal mining, characterized by the simultaneous implementation of mining activities and effective governance measures. In order to assess the ongoing vegetation recovery and the temporal changes in vegetation within the Shendong Coal Mining Area, we initially utilized Landsat TM/ETM+/OLI remote sensing data. Using the Google Earth Engine (GEE), we developed a novel kernel-normalized vegetation index (kNDVI) and subsequently generated a comprehensive kNDVI dataset spanning the years 2000 to 2020. In addition, the Sen (Theil-Sen median) trend analysis method and MK (Mann-Kendall) test were utilized to examine the temporal trends over a span of 21 years. Furthermore, the Hurst exponent model was employed to forecast the persistent changing patterns of kNDVI. The utilization of the intensity analysis model was ultimately employed to unveil the magnitude of vegetation dynamics. The findings indicated a notable positive trend in the overall kNDVI of vegetation within the study area. In relation to the analysis of changing trends, the vegetation in the region underwent a slight improvement from 2000 to 2010, followed by a significant improvement from 2010 to 2020. During this transition period, a total of 289.07 km2, which represents 32.36% of the overall transition area, experienced a shift in vegetation. The predictive findings from the Hurst model indicate that while the majority of areas within the mining region will exhibit an upward trend in vegetation growth, there will be certain areas that will demonstrate a decline. These declining areas account for 39.08% of the total transition area. Furthermore, the intensity analysis results reveal notable disparities in the characteristics of vegetation growth and evolution between the periods of 2000-2010 and 2010-2020. Throughout the entirety of the transformation process, the transition from slight improvement to significant improvement prevails in terms of both relative intensity and absolute intensity, surpassing alternative transformation processes. Various trend transitions display diverse intensity characteristics that adhere to the overarching principles governing shifts in vegetation growth. Furthermore, the utilization of the intensity analysis framework and intensity spectrum employed in this study demonstrates their efficacy in elucidating the temporal dynamics of vegetation changes. Furthermore, this study plays a pivotal role in the surveillance and assessment of the efficacy of ecological restoration in mining regions. It carries substantial implications for comparable land ecological restoration efforts in mining and reclamation, thereby furnishing a theoretical foundation.
1 Introduction
According to Zeng et al. (2023), vegetation serves as a reliable indicator of ecological changes and offers a comprehensive depiction of land and environmental conditions. The investigation of vegetation growth and its dynamic fluctuations in mining regions has consistently been a focal point of scholarly inquiry (Han et al., 2021b). Hence, the assessment of vegetation degradation and analysis of spatiotemporal dynamics in mining regions are of paramount importance in terms of their theoretical and practical implications for ecological restoration and enhancement of environmental quality in such areas (Guo et al., 2019; Jiang et al., 2022).
The Shendong Mining Area, situated in the loess-wind deposit sand mining region within the middle and upper reaches of the Yellow River basin, is characterized by an arid climatic condition (Xu et al., 2021). The area in question holds significant ecological fragility and serves as a crucial monitoring site for soil and water erosion in the context of governance in China (Chi et al., 2022). The ecological environment of the area is significantly impacted by the extensive coal mining activities (Xiao et al., 2020; Yang et al., 2022b). The restoration of vegetation in coal mining areas has gained widespread acceptance among nations (Roy et al., 2022). In addition, the monitoring of the fluctuating patterns of vegetation in mining regions is a fundamental aspect of initiatives aimed at restoring vegetation and holds significant importance in the planning, execution, and supervision of vegetation-related activities in mining areas (Liu et al., 2021a; Wang et al., 2021a; Xu et al., 2023b). Hence, the monitoring of vegetation dynamics and alterations in the Shendong Coal Mine carries significant importance. The research findings provide a theoretical basis for the implementation of vegetation management, soil erosion control, and ecological restoration efforts within the Shendong Coal Mine.
The production of coal has an undeniable impact on the ecological environment. Within the context of coal production, monitoring the ecological environment plays a crucial role in attaining economic sustainability (Burchart-Korol et al., 2016; Li et al., 2021a). At present, the predominant method for ecological monitoring in coal mines involves the utilization of remote sensing methods in conjunction with vegetation indices to evaluate the extent of vegetation coverage and the prevailing growth conditions on the terrain (He et al., 2019; Han et al., 2021a; Shang et al., 2022). The subject of long-term ecological monitoring has gained significant attention in recent times. Long-term ecological monitoring predominantly depends on the utilization of Landsat data, which offers a consistent supply of high-resolution multi-spectral remote sensing data spanning several decades, starting from the 1970s (Shan et al., 2019; Jiang et al., 2021; Pei et al., 2023). The utilization of remote sensing technology enables the temporal monitoring of vegetation, facilitating the investigation of alterations in the ecological environment. The Landsat TM/ETM satellite provides data with a high level of spatial resolution, which has led to its extensive utilization in the monitoring of land cover and land use change (Garioud et al., 2021; Pérez-Cabello et al., 2021; Zhou et al., 2022). The Normalized Difference Vegetation Index (NDVI) has emerged as the predominant vegetation index employed in long-term monitoring studies. Extensive research utilizing NDVI has contributed significantly to the understanding and characterization of spatiotemporal variations in vegetation cover, both at a national level within China and on a global scale (Huang et al., 2020; Jimenez et al., 2022; Martinez and Labib, 2023). Nevertheless, the Normalized Difference Vegetation Index (NDVI) does possess certain limitations. The relationship between the subject and green biomass exhibits a non-linear and saturated pattern (Carlson and Ripley, 1997). Additionally, it is important to consider potential errors that may arise when working with atmospheric noise, soil background, and saturation (Liu and Huete, 1995). Despite efforts made by researchers to address these limitations by integrating data from different spectral bands, the problem of saturation has yet to be resolved (Andualem and Berhan, 2021; Huang et al., 2021). In the year 2021, the kernel-normalized difference vegetation index (kNDVI) was introduced by Camps-Valls et al. (2021). The present vegetation index incorporates the benefits of machine learning principles and employs kernel methods for the extraction and computation of NDVI, with the objective of mitigating the constraints associated with the conventional methodology (Gustavo and Lorenzo 2009; Luis et al., 2018). Camps-Valls et al. (2021) conducted an evaluation and comparison of the performance of three vegetation indices, namely kNDVI, NDVI, and NIRv. Based on the outcomes of their research, it was observed that kNDVI demonstrated a higher level of effectiveness compared to NDVI and NIRv in diverse applications, biomes, and climatic zones. This study highlighted the distinct benefits of utilizing kNDVI in mitigating saturation effects, managing intricate phenological cycles, and accounting for seasonal variations. The suitability of the index for effectively representing the status of vegetation coverage in both natural and agricultural systems has been demonstrated in several studies (Liu et al., 2021b; Forzieri et al., 2022; Gensheimer et al., 2022; Wang et al., 2022b). Furthermore, the suitability of kNDVI for evaluating the growth conditions and temporal variations of vegetation in the mining region has been well-recognized (Wang et al., 2023). Therefore, in the present study, we opted for kNDVI as the preferred metric for assessing the condition of the vegetation ecosystem.
Nevertheless, previous research in the field of vegetation dynamics has predominantly concentrated on the gradual and uniform alterations within vegetation ecosystems, while investigations into the magnitude of spatiotemporal patterns have been relatively scarce. Presently, the main focus of scholarly inquiry pertaining to vegetation cover in mining regions revolves around the utilization of extensive time-series remote sensing data. This approach entails an examination of the effects of coal mining activities on the surrounding vegetation and broader ecological landscapes. The objective of this study is to analyze the dynamic patterns of vegetation cover in response to coal mining disturbances. The study aims to contribute valuable data and technical assistance for future restoration and management initiatives in mining regions (Wang et al., 2021b; Chen et al., 2022; Qi et al., 2023). Nevertheless, the extent to which vegetation growth is affected in mining areas remains uncertain. Therefore, the present study aims to introduce the intensity analysis method proposed by Pontius et al. (2004) for the purpose of assessing the intensity of vegetation dynamics. The analysis of intensity and vegetation change shows the extent to which vegetation change is influenced by factors such as climate change and human activities. This is accomplished by examining variables such as vegetation cover, vegetation growth rate, and vegetation types (Guesewell et al., 2007; Tong et al., 2016; Guo et al., 2018). In recent years, there has been a significant amount of scholarly research dedicated to the examination of vegetation change intensity (Murwira and Skidmore, 2006; Liu and Liu, 2018). One the one hand, the utilization of remote sensing technology enables the acquisition of vegetation index data, which in turn facilitates the examination of fluctuations in vegetation growth. Consequently, this approach enables the quantitative assessment of the magnitude of changes in vegetation (Siteur et al., 2014). Alternatively, an avenue for further exploration lies in examining the variations in response to climate change across different types of cover, thereby elucidating the connection between intensity analysis and vegetation change (Xu et al., 2013; Sun et al., 2023). This methodology not only examines the relative stability or dynamics of transitioning vegetation growth trend types, but also determines the dominant category during the transition process. Consequently, this enables us to gain understanding and discern the potential ramifications of ecological restoration initiatives on the recuperation of vegetation in the area.
In summary, this research employed Landsat TM/ETM+/OLI data spanning from 2000 to 2020 to construct a kNDVI dataset on the Google Earth Engine (GEE) platform. The primary objective is to analyze the spatial and temporal variations in vegetation cover and the magnitude of its alterations within the Shendong Coal Mine area. The research utilized Theil-Sen median slope analysis, Mann-Kendall (MK) test, and Hurst exponent analysis to investigate the spatiotemporal characteristics of vegetation cover and its future development trends in Shendong Coal Mine. Furthermore, the application of the intensity analysis framework is utilized to examine the evolutionary attributes of various types of vegetation growth trends during two distinct time periods: 2000-2010 and 2010-2020. The primary objective is to evaluate the current state of vegetation restoration in the mining region and offer informed suggestions for the long-term sustainability of Shendong Coal Mine.
2 Materials and methods
2.1 Study area
Shendong mining area (38°52′N–39°41′N, 109°51′E–110°46′E) is situated in the southeastern part of the Ordos Plateau and the northern edge of the Loess Plateau. It is situated at the geographical border between Yulin City in Shaanxi Province and Ordos City in Inner Mongolia Autonomous Region (as depicted in Figure 1A). The estimated land area encompasses approximately 900 km2. The region exhibits an average annual temperature of 6.2 °C, characterized by extreme minimum temperatures of -31.4 °C and extreme maximum temperatures of 36.6 °C. The annual precipitation in the region varies between 300 and 400 mm. Additionally, the rate of evaporation surpasses the amount of rainfall by more than fourfold, suggesting a characteristic arid to semi-arid continental climate. The region exhibits variations in topography, characterized by elevated terrain in the northwestern portion and comparatively lower terrain in the southeastern part (as depicted in Figure 1B). On average, the altitude of the area hovers around 1200 meters. The mining area’s eastern and northeastern regions are comprised of loess hills and mountains, which are distinguished by a network of gullies. The region is situated within a transitional ecological zone characterized by a blend of steppe and forest-steppe ecosystems (as depicted in Figure 1C). The dominant vegetation types in this area include grasslands, deciduous broadleaf shrubs, and sand-based vegetation. These areas display three distinct landforms, namely ridges, gullies, and loess tablelands. These regions are prone to erosion and significant soil degradation. The western and southwestern regions are characterized by the presence of mobile, semi-fixed, and fixed sand dunes, which provide a suitable environment for sand-based vegetation communities. These communities include sand-based pioneer plant communities and Artemisia communities. Wetland vegetation is commonly observed in low-lying areas, adjacent to river courses, and encircling bodies of water such as lakes.
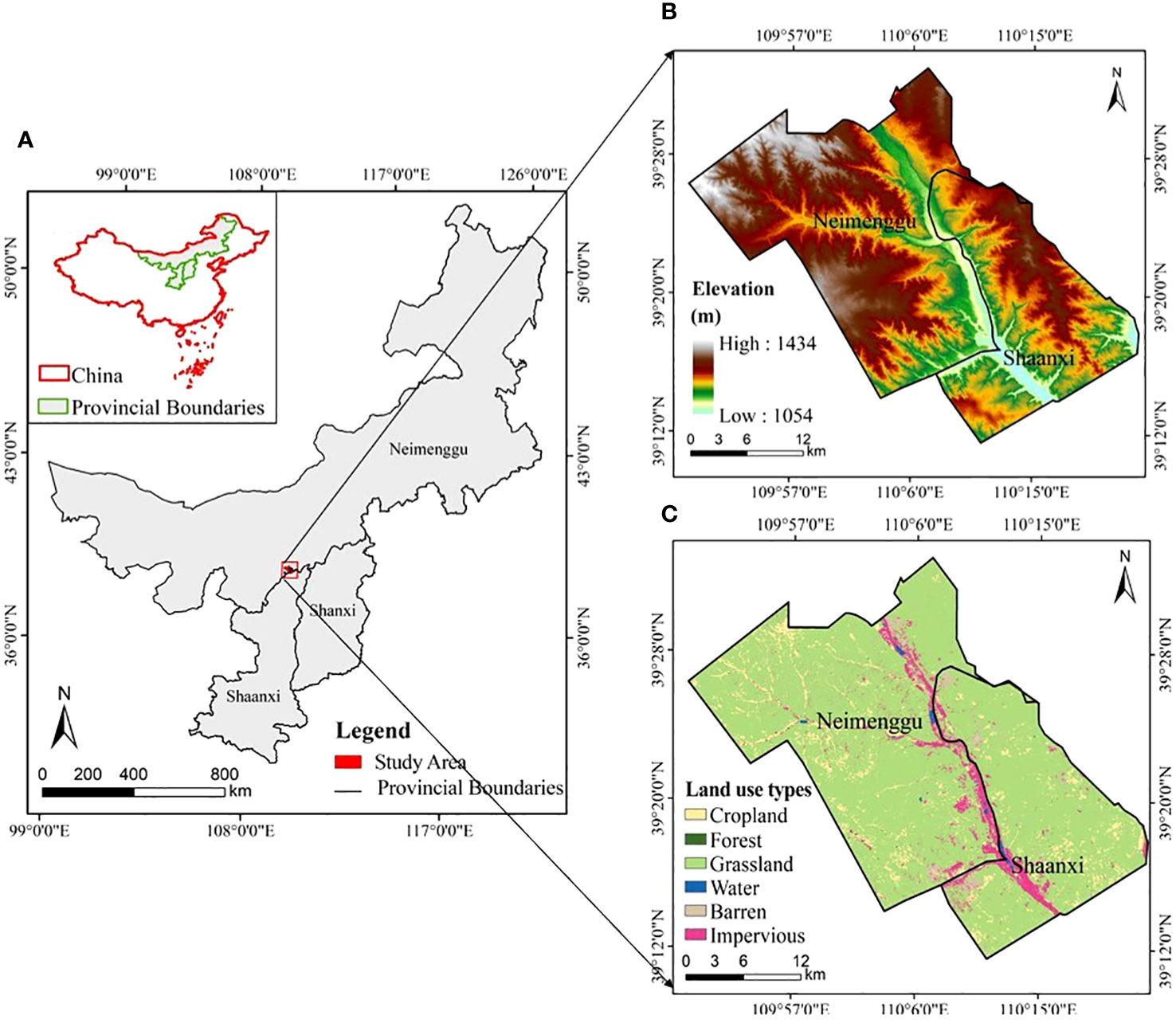
Figure 1 The study area in the Shendong Coal Mine (A) location in China, (B) elevation, and (C) land-cover class.
2.2 Data collection and preprocessing
The remote sensing image data utilized in this investigation were acquired from the United States Geological Survey (USGS) as Landsat T1_L2 products encompassing the time period from 2000 to 2020. The data were obtained through the utilization of the Google Earth Engine (GEE) platform. The resolution of the images was 30 meters, while the temporal resolution was 16 days. The data underwent preprocessing techniques, such as atmospheric correction, radiometric calibration, and cloud removal, specifically targeting data with cloud cover below 20% within the local vegetation growth season spanning from July to October. Table 1 presents comprehensive details regarding the data utilized in the present study. In order to mitigate the problem of data striping observed in Landsat 7 satellite imagery, a destriping algorithm provided by the Google Earth Engine (GEE) platform was utilized. Following that, the computation of the Normalized Difference Vegetation Index (NDVI) and Kernel Normalized Difference Vegetation Index (kNDVI) was carried out on the cloud. The study employed a median composite algorithm to generate composite images. Additionally, the Quality Mosaic algorithm available online was employed for image clipping in order to address the negative impacts of clouds, atmosphere, and satellite sensor angles on the remote sensing data.
The present study examines the accessibility of Landsat images within the Shendong mining area, utilizing the GEE cloud platform. A comprehensive analysis yielded a total of 276 images that were deemed suitable for further investigation. Figure 2 illustrates the temporal distribution of satellite images from the years 2000 to 2020, as depicted in Figure 2A, along with the corresponding number of available images, as shown in Figure 2B.
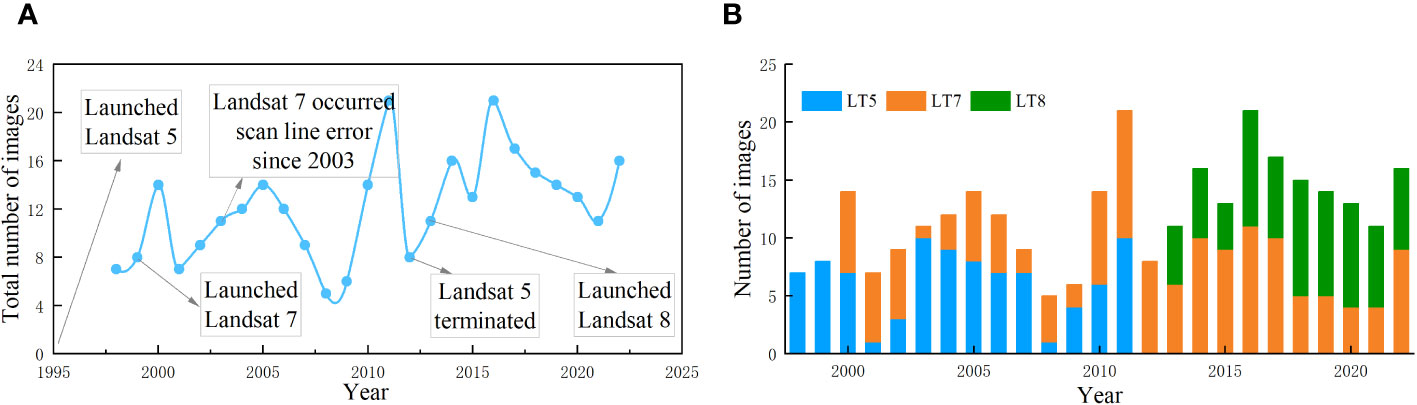
Figure 2 Availability of Landsat images of a time series of the reserve from 2000 to 2020, (A) Landsat image time distribution, (B) total number of sensor image (Landsat5/7/8).
2.3 Research methods
As illustrated in Figure 3, this study acquired a dataset of kNDVI spanning 21 years, from 2000 to 2020, specifically from the Shendong Coal Mine. The dataset was partitioned into two distinct periods, namely 2000-2010 and 2010-2020, in order to investigate the patterns of vegetation change. The Theil-Sen Median slope estimation and Mann-Kendall trend test methods were utilized to discern patterns of vegetation change. The Hurst exponent was employed to assess the long-term persistence of vegetation dynamics in the studied area. We employed the intensity analysis framework model to evaluate the intensity of transition trends in vegetation changes during the two periods. This assessment took into account both absolute and relative intensity perspectives.
2.3.1 kNDVI vegetation index calculation
The kNDVI is a normalized vegetation index that utilizes kernel functions, which are a type of machine learning techniques. The proposed approach represents an advancement of the conventional NDVI index, with the primary objective of addressing the challenges related to scale transformation and nonlinearity. According to Camps-Valls et al., 2021), the integration of kernel technology enables kNDVI to offer enhanced vegetation information that is both dependable and precise, even in scenarios involving nonlinear variations and across various scales. The calculation formula is as follows (Equation 1):
Where σ represents a length scale directly proportional to the mean values of near-infrared and red reflectance obtained from the remote sensing image. A τ =0.5 strikes a favorable compromise between accuracy and simplicity (Wang et al., 2023). Using σ = τ(NIR+Red). The calculation formulas are as follows : (Equations 2, 3)
2.3.2 Sen+Mann-Kendall vegetation trend analysis
The Theil-Sen median trend analysis, also referred to as Sen trend analysis, is a resilient non-parametric statistical technique employed to compute trends. In contrast to linear regression trend analysis, the Sen trend analysis method has the ability to mitigate the influence of missing time series data and the shape of the data distribution. Additionally, it effectively eliminates the interference caused by outliers in the time series data (Gocic and Trajkovic, 2013). The mathematical expression denoted as Equation 4 provides the formula for determining the magnitude of the Sen trend.
Where kNDVIi and kNDVIj represent kNDVI time series. A βkNDVI > 0.0005 indicates an improved kNDVI trend. Conversely, a βkNDVI < 0.0005 implies a degraded kNDVI trend.
The Mann-Kendall test, also known as the MK test, is frequently employed in conjunction with Sen trend analysis. The aforementioned approach is a non-parametric statistical test that exhibits robustness in the presence of missing values and outliers. Additionally, it does not make any assumptions regarding the underlying data distribution (Yue and Wang, 2004). The statistical test procedure is demonstrated in (Equations 5–8).
Where kNDVIj and kNDVIi refer to kNDVI time series; sign represents the sign function; S denotes the test statistic; Z is the standardized test statistic; n is the number of data points. At a given significance level α, if | Z | > , it suggests the presence of a significant trend change. In this study, α is set as to 0.05, implying the evaluation of the significance of kNDVI time series changes at a 0.05 significance level.
2.3.3 Analysis of vegetation change sustainability
The calculation of the Hurst exponent is derived from the application of the rescaled range (R/S) analysis method. This exponent is utilized as a metric to discern whether a given set of time series data adheres to a random walk or a biased random walk process. The description of time series patterns is a widely employed approach in the fields of hydrology, geology, and climate studies (Sioris et al., 2016). This study employs the Hurst exponent to characterize the future temporal evolution of pixel values within the study area. The computation method is as follows:
For a given time series { kNDVI ( t ), 1, 2,..., n }, the mean sequence is defined by Formula (Equation 9):
The cumulative deviation formula is (Equation 10) :
The value range formula of and is (Equation 11) :
The standard deviation formula is (Equation 12) :
And using the above formula, we can get Formula (Equation 13) :
A indicates the presence of the Hurst phenomenon in the analyzed sequence. Here, H represents the Hurst exponent, which can be obtained by fitting log , using the least squares method. A 0< H< 0.5 indicates anti-persistent kNDVI in the time series, implying that the future trend is opposite to the past. As H gets closer to 0, the degree of anti-persistence increases. Similarly, a 0.5 < H < 1 suggests positive correlation in the kNDVI time series, meaning that the future trend is consistent with the past. As H approaches 1, the degree of positive correlation strengthens. A H = 0.5 signifies that the variation trend of kNDVI in the time series is a random sequence with no significant correlation.
2.3.4 Intensity analysis framework model
The analysis of intensity focuses on the quantitative assessment of vegetation change trends, which occur at various time intervals. This examination is conducted from two distinct viewpoints: absolute intensity and relative intensity. The concept of absolute intensity pertains to the absolute number of trend conversions occurring within a specific time frame. This measure can be examined from two perspectives: the conversion from a particular trend type to different trend types, and the conversion from other trend types to the specified trend type. The calculation formulas for each intensity pattern are specified as follows:
The absonorlute transition intensity, denoted as AIij, represents the conversion of the initial vegetation trend level i to a specific final vegetation trend level j within the time interval [Tn,Tn+1] (where i≠j). Its calculation formula is as follows (Equation 14):
The mean absolute transition intensity (MAIj) for the conversion of all vegetation trend grades except j to grade j within the time interval [Tn,Tn+1]; Its calculation formula is as follows (Equation 15):
The absolute transition intensity, denoted as AOxy, represents the conversion of the initial vegetation trend grade x to a specific final vegetation trend y within the time interval [Tn,Tn+1] (where x≠y). Its calculation formula is as follows (Equation 16):
The average absolute transition intensity (MAOx) is calculated for all vegetation trend grades except x within a specific time interval [Tn,Tn+1]; Its calculation formula is as follows (Equation 17):
Where i and y represent the initial and final vegetation trend grades, while j and x represent the transition-in and transition-out vegetation trend grades. φij and φxy represent the area of the transition from grade i to grade j and the transition from grade x to grade y, respectively, within the given time interval. φjj and φxx represent the area in which the grade remains unchanged within the time interval. I and Y denote the number of initial and final vegetation trend grades, respectively.
The concept of absolute intensity pertains to the absolute number of trend type conversions, encompassing both the process of transitioning into a trend type and the process of transitioning out of it. Relative intensity, in continuation of this foundational analysis, conducts a further examination of the influence of the intensity of land cover conversion on the structure of vegetation change trends in the study area.
The transition intensity, denoted as RIij, represents the conversion of the initial vegetation trend grade i to a specific final vegetation trend grade j within the time interval [Tn,Tn+1] (where i≠j), as: Its calculation formula is as follows (Equation 18):
The average relative transition intensity, denoted as MRIj, is the conversion of all other vegetation trend grades except j to grade j within the time interval [Tn,Tn+1], as: Its calculation formula is as follows (Equation 19):
The relative transfer strength ROxy (x≠y) represents the conversion of the initial vegetation trend level x to a final vegetation trend level y within the time interval [Tn,Tn+1], as: Its calculation formula is as follows (Equation 20):
The average relative transfer strength MROx represents the conversion of vegetation trend level x within the time interval [Tn,Tn+1] to all other vegetation trend levels except x, as: Its calculation formula is as follows (Equation 21):
In the equation above, φix represents the area where the initial vegetation trend level i transitions to level x, and φiy represents the area within the time interval [Tn,Tn+1] where the initial vegetation trend level i transitions to the final levely.
3 Results
3.1 Temporal and spatial variations of vegetation coverage
3.1.1 Temporal dynamics of vegetation coverage
The representative kNDVI values for each year between 2000 and 2020 were obtained by utilizing the median value of kNDVI pixels in the images from 2000 to 2020, which serves as a comprehensive indicator of vegetation conditions. The annual kNDVI values were utilized in order to generate a fitted curve that illustrates the fluctuations in kNDVI, as depicted in Figure 4. As depicted in Figure 3, there exists a notable disparity in kNDVI values over the course of multiple years. Between the years 2000 and 2020, as depicted by the red line, the normalized difference vegetation index (kNDVI) exhibited an upward trajectory from 0.040 to 0.185. This corresponds to an annual growth rate of 0.0065, suggesting a notable and swift enhancement in vegetation coverage.
A model was developed to analyze the spatiotemporal variation of vegetation coverage within the protected area. This model utilized the pixel-based intermediate approach. The findings of the study indicate a statistically significant rise in the mean annual vegetation coverage within the mining region between 2000 and 2020 (p< 0.05). The Mann-Kendall mutation test was utilized to construct a map depicting the annual mutation of vegetation coverage in the mining area. The analysis revealed that this mutation occurred in the year 2010, as illustrated in Figure 5. Given the clear presence of discernible mutation points in both the UF and UB curves in the year 2010, the present study opted to partition the time series data pertaining to vegetation coverage within the protected area into two distinct stages: the period spanning from 2000 to 2010, and the subsequent interval from 2010 to 2020. Figure 4 illustrates the kNDVI trends from 2000 to 2010, as represented by the blue line. During this time frame, a gradual growth phase is observed, characterized by an increment from 0.04 to 0.079 in kNDVI values. The corresponding growth rate is calculated to be 0.0041 per annum. In contrast, the time span from 2010 to 2020 (represented by the green line) exhibited a notable period of expansion, as indicated by the increase in kNDVI from 0.079 to 0.185 and a growth rate of 0.013 per annum. During the period from 2000 to 2010, the Shendong Coal Mine undertook extensive afforestation initiatives and implemented diverse ecological and environmental comprehensive management approaches throughout its development and construction endeavors. Nevertheless, the challenging conditions of the mining region posed significant obstacles to the process of vegetation reconstruction and restoration, thereby impeding the rate of growth in comparison to the timeframe spanning from 2010 to 2020. Between the years 2010 and 2020, there was a notable establishment of a foundation for vegetation coverage, accompanied by intensified efforts towards vegetation recovery. Consequently, there was a significant and rapid augmentation in vegetation coverage. From the year 2000 to 2020, there was a noticeable pattern of substantial vegetation expansion within the mining region.
3.1.2 Spatial distribution characteristics of vegetation coverage
Figure 6 illustrates the distribution characteristics of median values of kNDVI during various stages of the 21-year duration of Shendong Coal Mine. The calculation of the overall vegetation for the time periods of 2000-2010, 2010-2020, and 2000-2020 was conducted using the median values of the annual kNDVI. The selection of median values was made to serve as a representation of the vegetation coverage across the three stages in question. The findings demonstrated a strong correlation between the extent of vegetation coverage and the corresponding rates of vegetation growth observed at each stage. The vegetation coverage during the time frame of 2010-2020 exhibited the highest value of 0.149, indicating the most favorable conditions. Subsequently, the periods of 2000-2020 and 2000-2010 displayed values of 0.087 and 0.065, respectively, suggesting relatively lower levels of vegetation coverage. Based on the analysis of Figure 6, it is evident that the three stages (Figures 6A–C) exhibit a notable concentration of elevated kNDVI values in the eastern and western regions of the Shendong mining area. Additionally, these high values are also observed in the mountainous areas flanking the town, as well as in the southern sections of the Huojitu and Daliuta mining areas. Conversely, the diminished values primarily manifest in the urban regions of the mining vicinity and adjacent areas that experience substantial anthropogenic impact, such as the Ulan Mulun and Shigetai coal mining regions. In general, the spatial distribution of kNDVI in the Shendong mining area demonstrates a consistent pattern. The vegetation coverage within the entirety of the Shendong mining area exhibits minimal fluctuations. The vegetation coverage in certain mining regions, such as Da Lita and Ulan Mulun Mine, exhibited a lower extent. In general, the vegetation coverage along the eastern and western boundaries of the Shendong Coal Mine exhibited superior characteristics. Moreover, this geographical area serves as a crucial site for the execution of ecological conservation initiatives in China, including afforestation and reforestation schemes. These endeavors have yielded noteworthy benefits in terms of enhancing vegetation coverage as a result of human interventions.
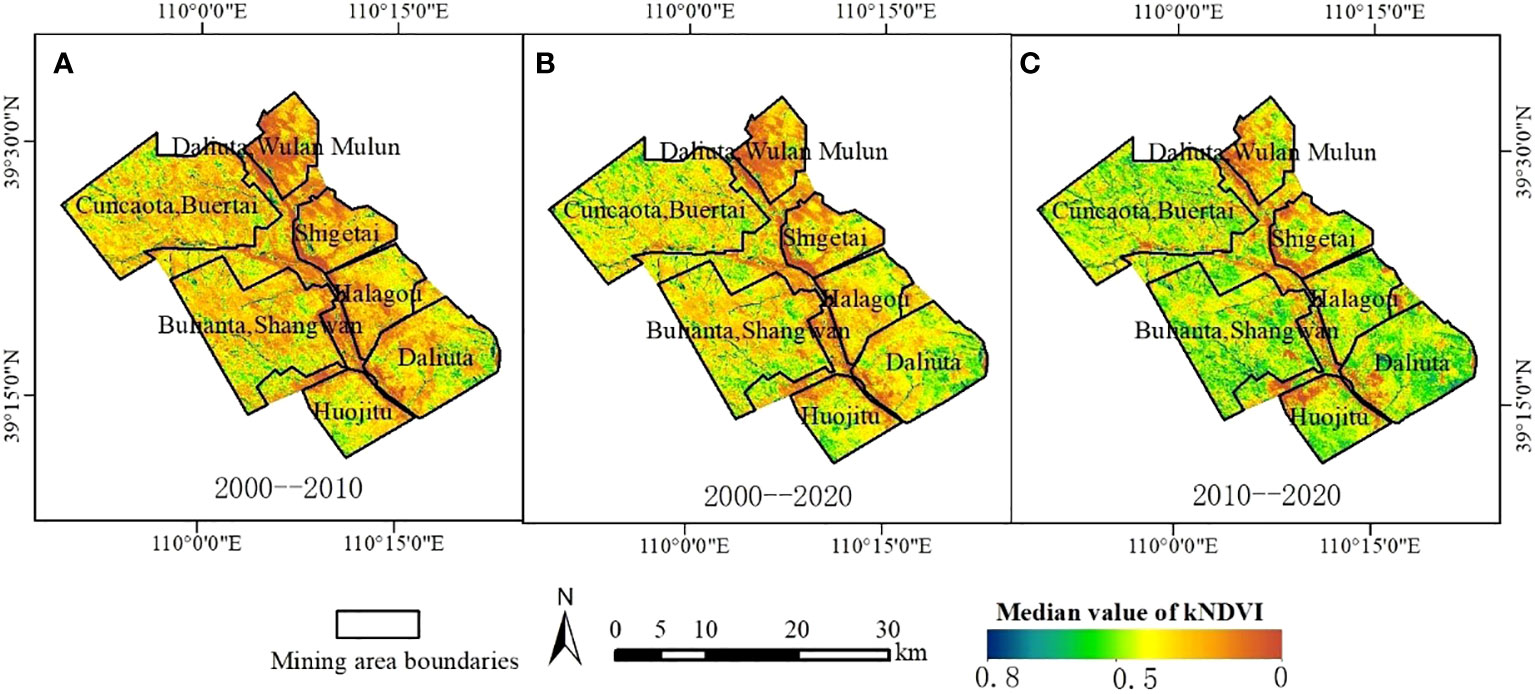
Figure 6 Spatial distribution characteristics of vegetation cover (A) 2000—2010, (B) 2000—2020, (C) 2010—2020.
3.1.3 Spatial variation characteristics of vegetation coverage
In order to accurately capture the patterns of vegetation changes and spatial distribution characteristics in the area, this research integrates Sen’s trend analysis with the Mann-Kendall test. The Sen’s values can be categorized into three distinct groups. The first group consists of values falling within the range of -0.0005 to 0.0005, which are considered as indicative of a stable condition. The second group includes regions with Sen’s values equal to or greater than 0.0005, which are classified as areas showing improvement. Lastly, the third group comprises regions with Sen’s values less than -0.0005, which are identified as areas experiencing degradation. The outcomes of the Mann-Kendall test are categorized into two groups: statistically significant changes (Z > 1.96 or Z< -1.96) and statistically insignificant changes (-1.96 ≤ Z ≤ 1.96), with a confidence level of 0.05. The vegetation change trend map for the entire region is derived by aggregating the results at each pixel scale. Based on the aforementioned classification criteria, the entire region can be categorized into five distinct groups, as illustrated in Table 2.
Based on the analysis of the annual inter-annual variation trend of kNDVI in the Shendong Coal Mine spanning from 2000 to 2020 (Figure 7), it is evident that the vegetation growth within the region has exhibited a notable enhancement, encompassing approximately 89.47% of the entire mining area. The proportion of the total area that is classified as degraded is 2.74%. The degraded areas primarily exist within different open-pit mining sites, specifically the northern section of Huojitu Mine and the southern section of Shigetai Mine. The mining region has adopted strategies for concurrent mining and restoration, with each individual mine making efforts to mitigate environmental harm and engage in active restoration of impacted areas. Consequently, the areas exhibiting slight improvements are primarily localized along the perimeters of the open-pit regions of each mine, constituting approximately 4.65% of the overall land area. The Shendong Coal Mine holds significant importance as a focal region for the implementation of ecological conservation initiatives within China. From the year 2000 to 2020, there was a notable increase in vegetation coverage within the mining area.
To provide a comprehensive depiction of vegetation dynamics from 2000 to 2020 and elucidate patterns of vegetation change, the temporal span is partitioned into two distinct stages: 2000-2010 and 2010-2020. This division is predicated on the growth rate of vegetation coverage, as illustrated in Figure 8. The examination of the trend in vegetation growth during two distinct stages provides insight into the intensity of vegetation restoration and the mode of transition observed in the mining area at different points in time. According to the data presented in Figure 8, the period from 2000 to 2010 witnessed a discernible but modest upward trajectory in the Shendong Coal Mine. This particular phase accounted for 54.83% of the overall area. The trend of significant improvement represents the second largest proportion, comprising 31.47% of the total area. In contrast, the period from 2010 to 2020 witnessed a notable upward trend in the dominance of the mining sector, with its share increasing from 31.47% in the 2000-2010 period to 61.16% in the 2010-2020 period. The percentage of the area exhibiting a slight improvement trend decreases from 54.83% to 32.39%. In general, the trajectory of vegetation dynamics progresses from a slight improvement to a significant improvement pattern.
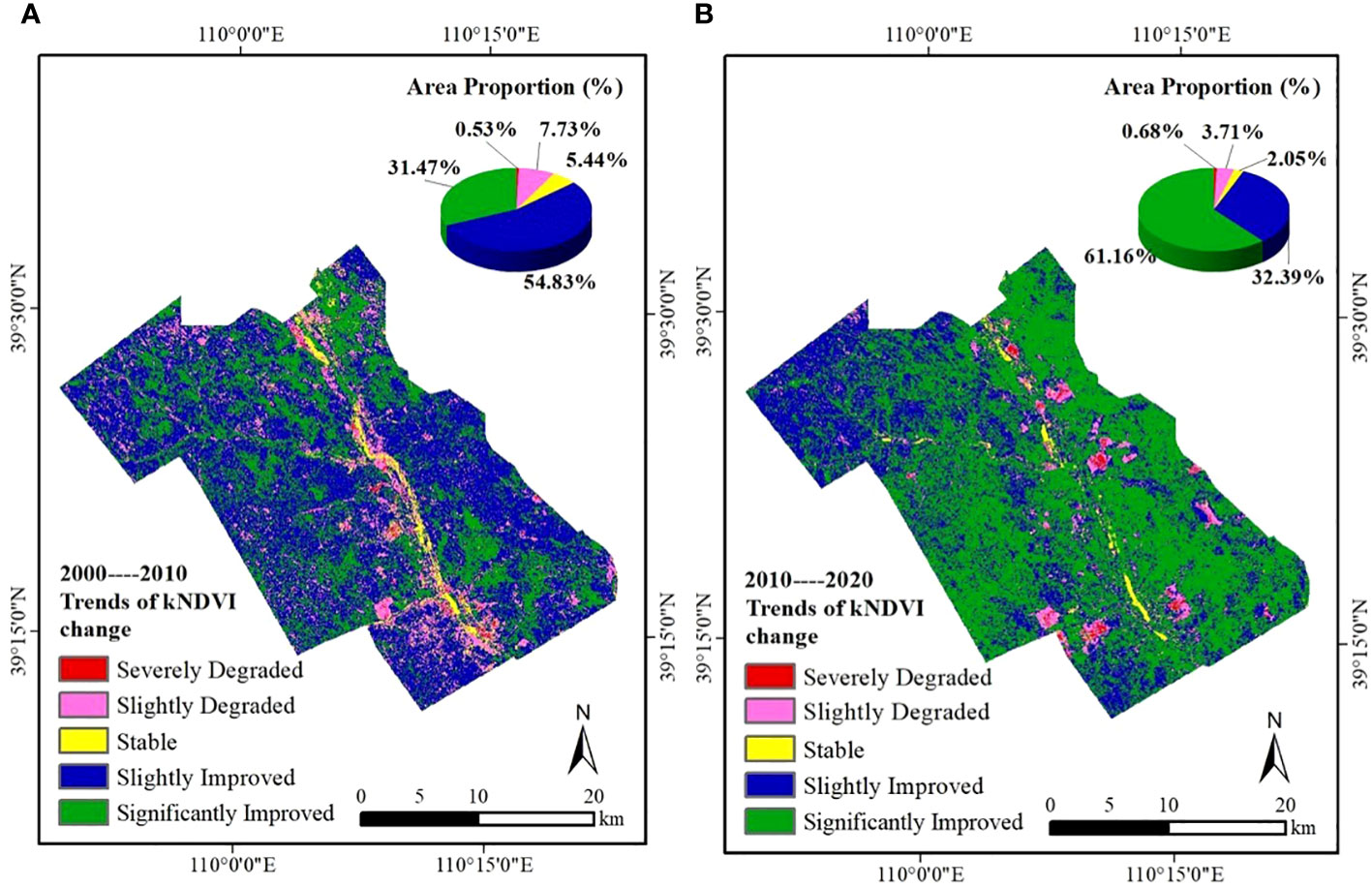
Figure 8 Trends of inter-annual kNDVI change in the Shendong coalfield: (A) 2000—2010, (B) 2010—2020.
3.2 The spatial distribution and future development trend of the Hurst exponent for vegetation coverage
3.2.1 Spatial distribution of Hurst exponent for vegetation coverage
According to the data presented in Figure 9, the average Hurst exponent for kNDVI in the Shendong Coal Mine is 0.521. The regions exhibiting Hurst values below 0.5 are primarily concentrated in the northwestern section of the mining area, encompassing the Cuncaota and Buertai Mines. These regions account for approximately 40.12% of the total area. Conversely, it can be observed that regions exhibiting Hurst values exceeding 0.5 are predominantly situated in the southeastern portion of the mining area, encompassing Bulianta, Shanwan, and Daliuta Mines. These specific regions account for approximately 59.88% of the overall area. The comprehensive examination of the mining region reveals that the kNDVI in Shendong Coal Mine demonstrates a spatial pattern characterized by clustering, accompanied by a certain level of variability.
3.2.2 Future development trend of vegetation coverage
To enhance comprehension regarding the trajectory and long-term viability of vegetation, an examination is conducted on the kNDVI trend, which is subsequently juxtaposed with the Hurst exponent. This integration yields interconnected insights, as depicted in Figure 10. The findings can be categorized into four distinct groups: a consistent downward trend, a consistent upward trend, a decline in the present with an anticipated increase in the future, and an increase in the present with an expected decrease in the future. The persistent decline in vegetation levels within the region signifies a sustained and pronounced downward trajectory. A continuous increase denotes a persistent and consistent upward trajectory in vegetation. The present reduction and forthcoming improvement signify a contemporary pattern of diminishing vegetation, with a prospective shift towards an ascending trajectory. The present improvement and forthcoming diminution signify a prevailing positive trajectory in vegetation, yet a prospective shift towards a negative trajectory.
Based on the data presented in Figure 10, it is evident that the majority of regions exhibit an increasing trend in vegetation, with certain areas projected to undergo a decline in the future. These declining areas encompass a total land area of 349.22 km², constituting approximately 39.10% of the overall region. The aforementioned regions primarily encompass the Cuncaota and Buertai coal mine areas. Nevertheless, it is important to acknowledge that the observed decrease in vegetation in Buertai coal mine and similar regions may not provide an accurate representation of the true state of vegetation on the terrain. Based on field investigations, it has been discovered that the Cuncaota and Buertai coal mines have adopted an “ecological restoration and utilization model” that aligns with the local ecological conditions. This model involves the establishment of ecological restoration bases in areas affected by coal subsidence, as well as the implementation of photovoltaic-assisted planting techniques across a designated land area spanning 42,000 acres. As a result, the utilization of photovoltaic panels has made remote sensing techniques insufficient for accurately monitoring the current state of ground vegetation. The areas in question exhibit a consistent pattern of growth, encompassing a total land area of 510.87 km², which corresponds to 57.20% of the overall territory. These areas hold significant influence within the context of the Shendong Coal Mine. Only a small proportion, specifically 2.85%, of the entire region exhibits a persistent decline, predominantly concentrated within the open-pit areas of diverse mining operations.
3.3 Analysis of the intensity of vegetation change in Shendong coal mine
3.3.1 Analysis of vegetation growth trend changes in Shendong coal mine
The vegetation growth trend observed in Shendong Coal Mine can be divided into two stages: 2000-2010 and 2010-2020. During the first stage, there was a slight improvement in vegetation growth, while during the second stage, there was a significant improvement. The combined area percentage of vegetation growth during the 2000-2010 period was 95%, whereas it was 86.30% during the 2010-2020 period. A transition matrix (Table 3) is utilized to conduct a more comprehensive examination of the change characteristics of various vegetation trend types in Shendong Coal Mine. During the transition from the 2000-2020 period to the 2010-2020 period, the prevailing pattern of vegetation change predominantly exhibits a modest improvement, as evidenced by an area measuring 489.75 km² transitioning away from this particular category. The predominant form of vegetation change that occurs during the transition process is characterized by a substantial improvement, encompassing a total area of 546.29 square kilometers. Among the diverse categories of vegetation trend transitions, the transition from a slight to a significant improvement stands out as the most notable. This transition encompasses an area of 289.07 km², constituting approximately 58.02% of the total area undergoing a transition away from the slight improvement category. In general, the vegetation trends observed in Shendong Coal Mine during the two stages exhibit a consistent and stable pattern of cross-transition. This pattern primarily involves a shift between slight improvement and significant improvement types, indicating the favorable influence of local ecological restoration initiatives on the recovery of vegetation.
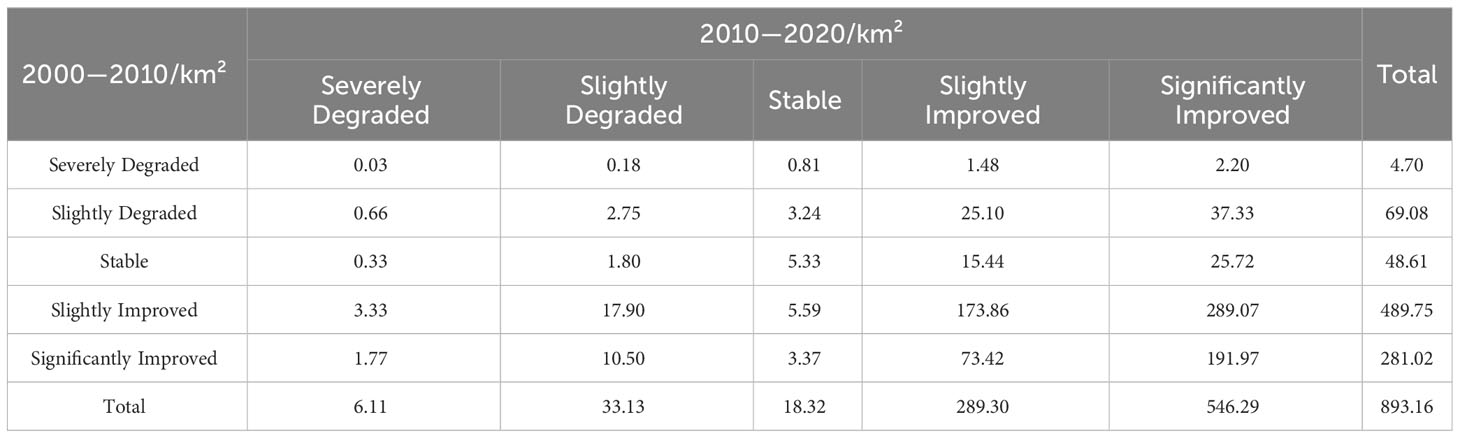
Table 3 Transition matrix of vegetation growth trends from the period of 2000-2010 to the period of 2010-2020.
The existing research primarily emphasizes the direct utilization of area change information from the transition matrix, without considering the underlying relationship between the structure of vegetation trend and its transformation. To fully harness the information contained in the transition matrix, this study proposes an intensity analysis model. This model aims to delve into the deeper-level information within the transition matrix and comprehensively analyze the characteristics of vegetation trend transitions in the region.
Figure 11 displays the chart depicting the intensity of change in vegetation growth trends. The chart comprises units that symbolize the reciprocal transformation between the initial vegetation growth trend (i) and the final vegetation growth trend (j) within a specific time interval. The x-axis is indicative of the initial trend in vegetation growth, whereas the y-axis represents the final trend in vegetation growth. The intensity chart comprises four components for each unit: absolute inflow intensity, absolute outflow intensity, relative inflow intensity, and relative outflow intensity. The filling rules can be described as follows: the color light green is used to represent a tendency, while the color orange is used to represent an inhibition. Specifically, when all units in the chart are horizontally filled with light green, it signifies a transformation process from the initial vegetation growth trend i to the final vegetation growth trend j. This transformation is characterized by both the absolute inflow intensity and absolute outflow intensity exhibiting a tendency, which reflects an overall absolute tendency in the transformation. When all units are occupied by the color orange, it signifies a relative inclination within the process of transformation. When a collection of entities is populated exclusively with either light green or orange, it signifies the presence of a systematic tendency or inhibition.
The intensity analysis framework offers a comprehensive examination of the transfer matrix data, thereby enhancing the availability of decision-making information for local ecological restoration efforts. Figure 11 illustrates discernible attributes in the alteration of vegetation growth patterns during two distinct time periods: 2000-2010 and 2010-2020. There exist four primary forms of intensity conversion, with the relative tendency emerging as the prevailing type. Furthermore, there is a relatively balanced distribution of tendencies and inhibitions in general. The shift from significant improvement to slight improvement signifies a complete transformation in trend. This suggests that the vegetation within the mining region exhibits a propensity for degradation in terms of absolute intensity, yet demonstrates an increasing trend in terms of relative intensity. For instance, the transitions observed between significant degradation and slight degradation, significant degradation and stability, and slight degradation and stability demonstrate inherent tendencies that are relative in nature. The observed shift from slight to significant improvement indicates a consistent pattern, providing additional evidence for the positive impact of ecological restoration initiatives on vegetation in the mining region. The observed results are consistent with the patterns of local vegetation evolution. The intensity spectrum encompasses the fundamental principles governing vegetation growth trends as well as the dynamic variations in vegetation growth characteristics. The transition from slight to significant improvement aligns with the overarching principles governing alterations in vegetation growth patterns, thus affirming the viability of the intensity analysis framework and visualization spectrum employed in this research. The forthcoming analysis will concentrate on conducting a comprehensive examination of these patterns of change.
3.3.2 Analysis of the transformation pattern from slight improvement to significant improvement
The primary analysis was centered on examining the transitional region and variations in the intensity of various types of vegetation growth trends. In the examination of the intensity chart pertaining to the transition from slight improvement to significant improvement (Figure 12), the dashed line denotes the uniform transitional intensity expressed as a percentage. When the transitional intensity surpasses the uniform intensity, it signifies a preferential focus on gains and losses within a specific growth trend category. The analysis reveals that the significant improvement trend type demonstrates a notable expansion in its coverage area, exhibiting an intensity that surpasses the average level by a significant margin (0.016%). This is in contrast to the slight improvement trend type, as depicted in Figure 12A. These findings suggest a propensity for transitioning from the slight improvement trend type to the significant improvement trend type. Furthermore, this transition appears to impede the shift from the slight degradation trend type and the remaining four types. In a similar vein, the slight improvement trend type demonstrates a heightened intensity surpassing the average level (0.016%) during the transition to the significant improvement trend type. This suggests a propensity towards transitioning to the significant improvement, while inhibiting transitions to the significant degradation trend type and other types (see Figure 12C). When considering the relative intensity, if each type transitions to the significant improvement trend type in proportion to its initial area, the inflow intensities of each type should be equal. The data reveals that the slight improvement trend type experiences a relatively higher inflow intensity (0.058%) towards the significant improvement trend type (Figure 12B). This suggests that there is a tendency for the slight improvement type to transition into the significant improvement type with a larger proportion of its area, resulting in a more substantial impact on the percentage of the significant improvement type in the study area. In a similar vein, the type characterized by a slight improvement trend demonstrates a relatively higher outflow intensity (0.052%) towards the slight degradation and significant improvement types (Figure 12D). This suggests a greater increase in the proportions transitioning to other types, as opposed to the inhibited transition to the stable type. This implies that the process of transformation has a substantial influence on the proportion of the significant improvement category within the study region.
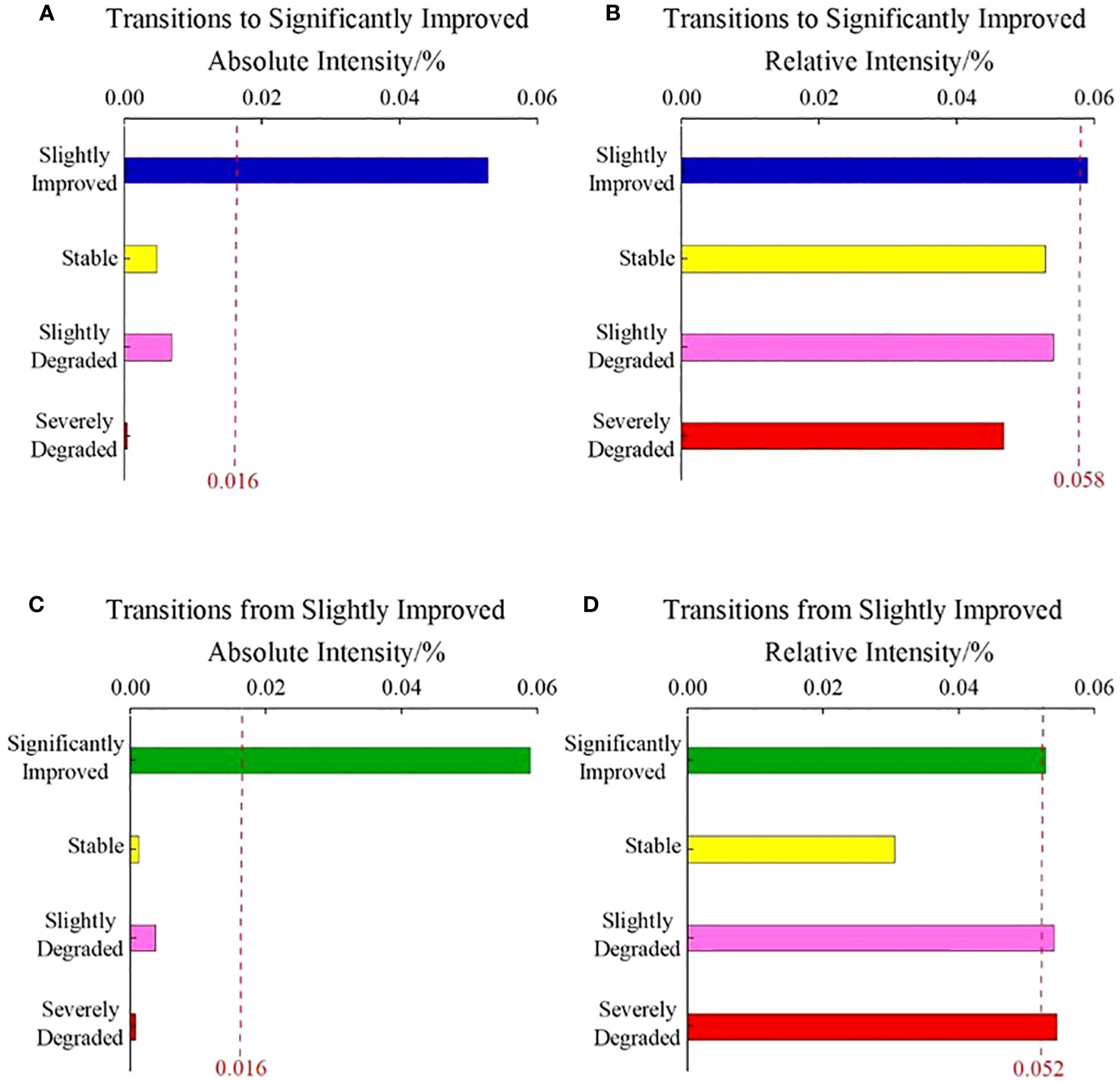
Figure 12 Intensity analysis of Slightly Improved to Significantly Improved, (A) Transitions to significantly improved absolute intensity, (B) Transitions to significantly improved relative intensity, (C) Transitions from slightly improved absolute intensity, (D) Transitions from slightly improved relative intensity.
3.3.3 Analysis of the transformation pattern from stable to slight degradation
The examination of the intensity chart depicting the transition from stable to slight degradation (Figure 13) reveals that Shendong Coal Mine exhibits a discernible inclination in the progression from the stable vegetation growth category to the slight degradation category. Regarding absolute intensity, it is observed that both the inflow from stable to slight degradation and the outflow from stable to slight degradation exhibit values that are below the average absolute intensity, specifically 0.023% and 0.022% respectively. This observation suggests that the size of the transition zone between stable and slightly degraded types is relatively limited when compared to other types. This implies that there is an inhibitory effect in terms of the overall quantity, as depicted in Figures 13A, C. However, in terms of relative intensity, the slight degradation type demonstrates a greater inflow from both the stable and significant degradation types compared to the average level (0.0037%). In a similar vein, the stable type demonstrates a greater outflow towards the slight degradation type from both the stable and significant degradation types compared to the average level of 0.005% (as depicted in Figures 13B, D). The aforementioned findings demonstrate a notable inclination towards transitions from the stable category to the slightly degraded category. It is important to acknowledge that the inhibitory behavior observed in absolute intensity does not directly constrain the relative tendency in terms of intensity. Although the transition area between the stable type and the slight degradation type is small, it can still exert a notable influence on the distribution of these types within the region.
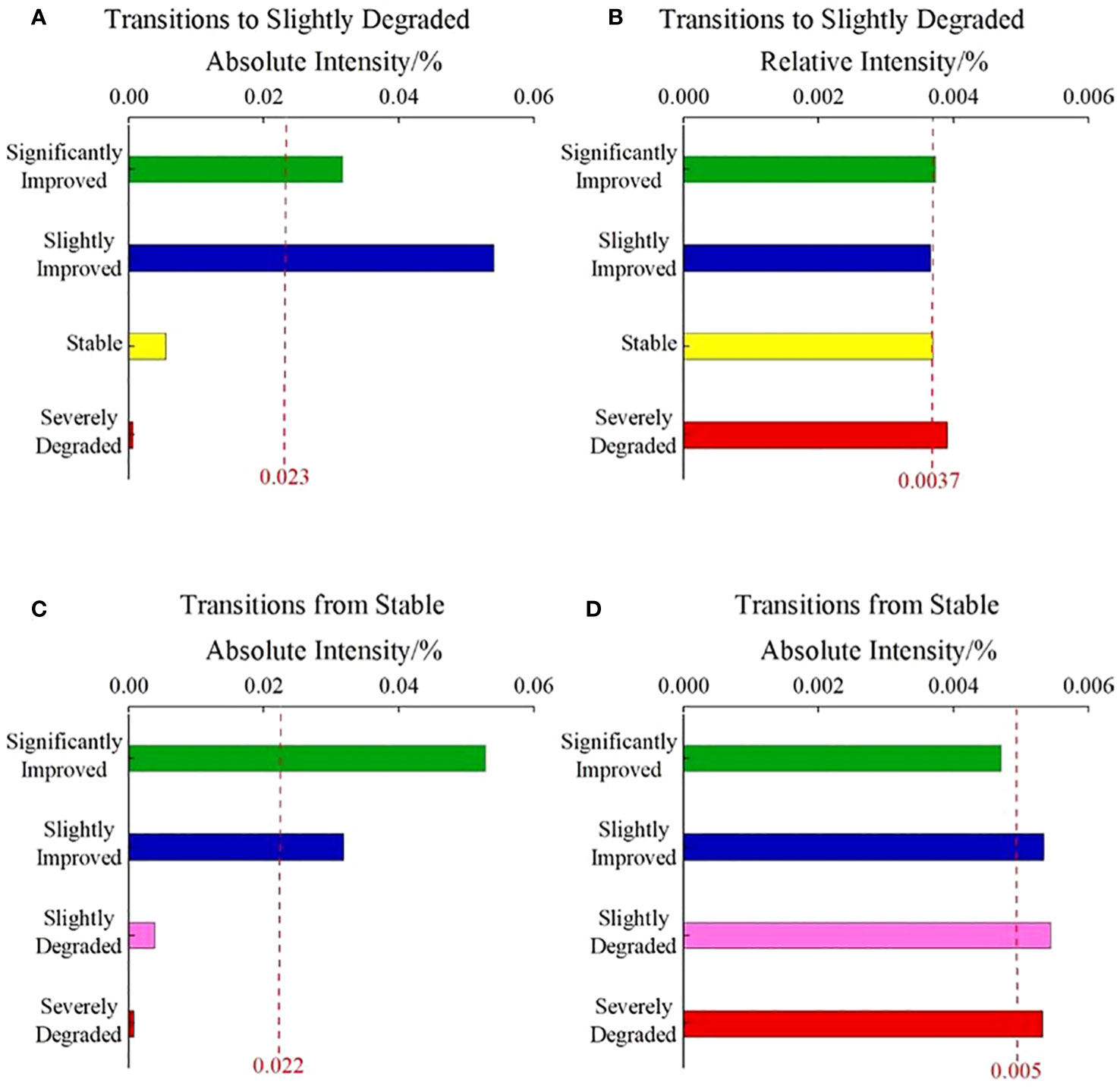
Figure 13 Intensity analysis of Stable to Slightly Degraded, (A) Transitions to slightly degraded absolute intensity, (B) Transitions to slightly degraded relative intensity, (C) Transitions from stable absolute intensity, (D) Transitions from stable absolute intensity.
4 Discussion
4.1 The kNDVI index and spatiotemporal changes
This study provides a comprehensive examination of the spatial distribution, inter-annual variability, and intensity transitions of the kNDVI (kernel Normalized Difference Vegetation Index) in the Shendong Coal Mine area from 2000 to 2020. The analysis is conducted using the Google Earth Engine (GEE) platform. In contrast to prior research, the primary emphasis of this paper lies in the enhancement of vegetation indices and the examination of spatiotemporal variations in vegetation. The current body of research primarily relies on NDVI products, primarily derived from MODIS data, which may not provide an accurate representation of vegetation changes in mining areas (Li et al., 2020; Li et al., 2021b; Xu et al., 2022). Nevertheless, the utilization of kNDVI in this research significantly deviates from the conventional NDVI approach by effectively addressing the challenge of mixed pixels. Both the kNDVI and NIRv indices operate within the near-infrared spectrum. Pixels exhibiting high vegetation coverage demonstrate a robust association between kNDVI and variables such as chlorophyll fluorescence. However, this correlation diminishes as vegetation coverage declines (Zhang et al., 2022; Ma et al., 2023b; Wang et al., 2023). Yet, the reduction in correlation observed for kNDVI is comparatively less pronounced than that observed for NDVI, suggesting that the kNDVI index exhibits a notable capability in distinguishing reflectance across various vegetation levels (Ding et al., 2022; Qiu et al., 2022). Furthermore, the kNDVI metric possesses a robust theoretical foundation, rendering it straightforward to compute and implement. Moreover, it holds significant utility in the examination of both natural and agricultural systems. The index demonstrates a strong association with GPP and SIF in grasslands, farmland, mixed forests, and arid areas. This suggests that the index effectively addresses saturation and mixed pixel challenges that are commonly encountered by conventional indices (Deng et al., 2020; Wang et al., 2022a). Moreover, the scope of its application extends beyond the monitoring of vegetation, encompassing change and anomaly detection, phenology, and greening research. Furthermore, this exemplifies the viability and significance of utilizing this index as a means of monitoring alterations in vegetation restoration within the Shendong mining area. A comprehensive analysis of vegetation spatiotemporal changes over a period of 21 years in the Shendong mining area has been conducted, focusing on the utilization of the kNDVI index to address saturation effects in vegetation analysis. The current body of literature predominantly centers on the examination of spatiotemporal variations in vegetation within a specific geographic area over an extended duration (Zhang et al., 2021; Guo et al., 2023). However, there is a dearth of research investigating vegetation dynamics across distinct time periods within the same region, as well as the evolving nature of these changes over time. The Shendong Coal Mine is situated in a region that serves as a transitional zone between the Loess Plateau and the Mu Us Desert. This location renders it a representative mining area of significant importance for ecological restoration efforts (Yang et al., 2022a). In order to evaluate the efficacy of vegetation restoration and elucidate the temporal evolution of vegetation recovery in Shendong Coal Mine over a span of 21 years, this study undertakes a comprehensive analysis of the spatial and temporal patterns as well as the magnitude of changes in vegetation. Drawing upon prior research, the objective of this study is to enhance the breadth of knowledge regarding the fluctuations in vegetation within the Shendong Coal Mine. By doing so, it aims to contribute valuable insights that can inform ecological and environmental restoration endeavors in the area.
4.2 Analysis of factors influencing kNDVI spatiotemporal changes
The study utilized Sen’s and Mann-Kendall trend analysis to examine the spatiotemporal variation trend of vegetation coverage in the research area. The findings of the study revealed a statistically significant alteration in the extent of vegetation coverage when implementing the concurrent mining and restoration approach in the Shendong East mining region. The study area exhibited a consistent upward trajectory in vegetation coverage from 2000 to 2010, followed by a substantial acceleration in growth from 2010 to 2020. Consequently, there was a notable enhancement in the overall vegetation coverage. This discovery is consistent with the research findings of Wu et al. (2023). The recovery of vegetation in the mining area can be attributed to the successful implementation of various strategies such as reforestation, grassland enclosure, and rotational grazing policies in the Shendong East mining area since 2000. These measures have had a positive impact on the restoration of grassland ecology. The Shendong East mining area is characterized by its geographical location within a semi-arid and arid climate zone, which contributes to the presence of a delicate natural environment and challenging climatic conditions. The degradation of the ecological environment in this mining area has been intensified by the large-scale, high-intensity, and multi-layered mining activities that have been repeatedly conducted. The intensive mining activities in the region have initiated a cascade of interconnected consequences, encompassing diverse environmental and societal concerns. Among these, the degradation of soil and vegetation has been identified as the most profoundly affected aspect (Xu et al., 2021). In the Shendong East mining area, the simultaneous mining and remediation model has been implemented, incorporating vegetation restoration practices since the initiation of production in 1985. In light of the recurrent sandstorms and significant soil erosion observed in the mining region, early-stage efforts in vegetation restoration involved the implementation of measures such as grid fixation and sand flow improvement. These measures were aimed at stabilizing the areas characterized by mobile sand. Water storage and soil conservation were accomplished utilizing techniques such as “horizontal ditches” and “fish-scale pits.” Following this, various models for vegetation restoration were developed, taking into account the varied ecological conditions found in different regions (Song et al., 2022). Liu et al. (2021a) have classified the vegetation restoration models in the Shendong East mining area as economic forest, ecological forest, photovoltaic grassland, and sand control models. Subsequently, crops and vegetation have been cultivated in accordance with these models. Following an extensive period of ecological restoration, the Shendong East mining area has witnessed a substantial augmentation in vegetation coverage, surging from a mere 3% to an impressive figure exceeding 64%. The plant community has undergone a transition from an herbaceous community primarily governed by Artemisia ordosica to a shrub-grass community predominantly governed by Hippophae rhamnoides. The plant species have experienced a substantial increase in their numbers, expanding from the initial count of 16 to approximately 100. This notable growth has had a significant impact on the populations of microorganisms and animals. The enhancement of the existing delicate ecological environment has been observed (Xu et al., 2023a). Nevertheless, the notable augmentation in vegetation coverage within the mining region cannot be exclusively ascribed to artificial ecological restoration initiatives. Temperature and precipitation are significant factors that contribute to the promotion of vegetation growth in mining areas (Yu et al., 2020). In brief, the notable reestablishment of vegetation within the mining region can be attributed to the collaborative endeavors of local afforestation initiatives and climatic influences.
4.3 Limitations and future work
Moreover, this study employs intensity analysis as a means to further investigate the transformation characteristics exhibited by various types of vegetation growth trends within the designated study area. The intensity analysis method was employed to visually represent the transition patterns of vegetation growth trends in the region. This analysis revealed distinct tendencies and inhibitions in the transformation processes of different types of vegetation growth trends. For example, the trend types of significant improvement and slight improvement demonstrate an absolute tendency in their transformations, whereas the trend types of slight and significant improvement exhibit a relative tendency in their transformations. The results of this study further confirm the efficacy of the intensity analysis approach employed, while also offering additional insights into the comprehension of alterations in vegetation growth within the research site. Furthermore, through the integration of the kNDVI trend and the Hurst exponent, this investigation unveils the long-term viability of alterations in vegetation. The findings indicate the existence of four distinct sustainability patterns: a consistent decline, a consistent growth, a decline in the present with projected growth in the future, and growth in the present with projected decline in the future. These findings provide additional evidence of the lack of sustainability and the existence of a positive correlation between vegetation changes in the study area. Moreover, they contribute to enhancing our comprehension of the dynamic processes associated with vegetation changes.
Nevertheless, this study exhibits specific constraints and deficiencies. The scope of this study was limited to the Shendong East mining area, which may restrict the generalizability and applicability of the research findings. Furthermore, the present study exclusively utilized remote sensing data for analysis, without taking into account field data and other relevant factors. In addition, there was a lack of investigation into the influence of meteorological factors on the spatial and temporal variations of local vegetation, as well as a failure to explore the underlying mechanisms driving these changes. The specific contributions of human activities and meteorological conditions to the observed substantial increase in vegetation coverage remain uncertain. Furthermore, the long-term dynamics of vegetation coverage are subject to various non-climatic influences, including urban expansion, construction projects, grazing, and land use changes (Ma et al., 2023a). Hence, it is imperative for future studies to integrate field surveys and other pertinent data sources in order to holistically and precisely elucidate the mechanisms by which coal mining activities affect vegetation coverage. In addition, it is imperative to integrate human activities and meteorological factors into the analysis in order to enhance comprehension of the spatial distribution of diverse climatic and non-climatic driving factors. This will ultimately enable a more profound exploration of the correlation between coal mining activities and vegetation ecological environments.
5 Conclusions
The analysis focused on the spatiotemporal pattern of vegetation kNDVI in the Shendong mining area from 2000 to 2020, utilizing Landsat kNDVI data. This examination provided insights into the sustainability and intensity of evolution in vegetation change trends. The findings indicated a consistent upward trajectory in kNDVI values throughout the span of 21 years. The implementation of ecological restoration initiatives resulted in a significant improvement of kNDVI throughout the entire region. Specifically, during the period from 2010 to 2020, there was a notable and swift growth rate of 0.013 per annum. The analysis of vegetation coverage in the mining area using the Theil-Sen median trend and Mann-Kendall tests demonstrated a noteworthy enhancement in vegetation growth over the course of the previous two decades. Specifically, the vegetation now encompasses 89.47% of the total area, while only 2.74% of the area has experienced degradation. In general, there was a notable upward trajectory observed in the vegetation coverage within the mining region. The examination of various stages indicated that during the period from 2000 to 2010, there was primarily a marginal enhancement trend, constituting approximately 54.83% of the observed data. Conversely, from 2010 to 2020, a substantial improvement trend emerged as the prevailing pattern, encompassing approximately 61.16% of the analyzed data. In general, there was a transition in the vegetation dynamic trend from a slight improvement to a significant improvement.
Despite observing a general improvement and notable enhancement in vegetation within the mining region, analysis of the Hurst index distribution reveals that approximately 40.12% of the area is projected to experience unsustainable vegetation growth in the coming years. Consequently, the vegetation in these regions will experience a distinct transformation from its initial state of growth. Upon analyzing the vegetation growth patterns, a majority of the studied regions displayed an upward trajectory. However, certain areas that exhibited an initial increase in vegetation are projected to experience a decline in the future. These areas encompass a landmass of 349.22 km2, accounting for approximately 39.10% of the overall mining area. Moreover, the transformation characteristics and transition intensities of vegetation growth trends in the Shendong mining area during the periods of 2000-2010 and 2010-2020 were visually depicted within the framework of intensity analysis. As an illustration, the shift from a slight to a significant increase demonstrated a consistent pattern, whereas the shift from a substantial increase to a slight increase displayed an unequivocal pattern. The observed characteristics suggest that the vegetation within the mining site exhibits a degree of adherence to the principles of ecological restoration during the mining process.
Data availability statement
The original contributions presented in the study are included in the article/supplementary material. Further inquiries can be directed to the corresponding author.
Author contributions
ZC: Conceptualization, Writing – original draft. XZ: Investigation, Methodology, Writing – original draft. YJ: Data curation, Validation, Writing – review & editing. YC: Software, Supervision, Writing – original draft. ZZ: Investigation, Resources, Writing – original draft. SW: Project administration, Resources, Writing – original draft. HZ: Funding acquisition, Project administration, Visualization, Writing – review & editing.
Funding
The author(s) declare that financial support was received for the research, authorship, and/or publication of this article. This work supported by the State Key Project of National Natural Science Foundation of China-Key projects of joint fund for regional innovation and development (grant number U22A20620 U21A20108), Doctoral Science Foundation of Henan Polytechnic University (grant number B2021-20), China Shenhua Shendong Science and Technology Innovation Project (grant number E210100573).
Acknowledgments
We appreciate reviewers and their valuable comments. Also, we thank Editors for the editing and comments.
Conflict of interest
The authors declare that the research was conducted in the absence of any commercial or financial relationships that could be construed as a potential conflict of interest.
Publisher’s note
All claims expressed in this article are solely those of the authors and do not necessarily represent those of their affiliated organizations, or those of the publisher, the editors and the reviewers. Any product that may be evaluated in this article, or claim that may be made by its manufacturer, is not guaranteed or endorsed by the publisher.
References
Andualem A. T., Berhan G. A. (2021). Evaluation of the saturation property of vegetation indices derived from sentinel-2 in mixed crop-forest ecosystem. Spatial Inf. Res. 29 (1), 109–121. doi: 10.1007/s41324-020-00339-5
Burchart-Korol D., Fugiel A., Czaplicka-Kolarz K., Turek M. (2016). Model of environmental life cycle assessment for coal mining operations. Sci. Total Environ. 562, 61–72. doi: 10.1016/j.scitotenv.2016.03.202
Camps-Valls G., Campos-Taberner M., Moreno-Martinez A., Walther S., Duveiller G., Cescatti A., et al. (2021). A unified vegetation index for quantifying the terrestrial biosphere. Sci. Adv. 7 (9). doi: 10.1126/sciadv.abc7447
Carlson T. N., Ripley D. A. (1997). On the relation between NDVI, fractional vegetation cover, and leaf area index. Remote Sens. Environ. 62 (3), 241–252. doi: 10.1016/S0034-4257(97)00104-1
Chen L., Zhang H., Zhang X., Liu P., Zhang W., Ma X. (2022). Vegetation changes in coal mining areas: Naturally or anthropogenically Driven? Catena 208, 105712. doi: 10.1016/j.catena.2021.105712
Chi M.-b., Li Q.-S., Cao Z.-G., Fang J., Wu B.-Y., Zhang Y., et al. (2022). Evaluation of water resources carrying capacity in ecologically fragile mining areas under the influence of underground reservoirs in coal mines. J. Clean. Prod. 379. doi: 10.1016/j.jclepro.2022.134449
Deng Y., Wang S., Bai X., Luo G., Wu L., Chen F., et al. (2020). Vegetation greening intensified soil drying in some semi-arid and arid areas of the world. Agric. For. Meteorology 108103, 292–293. doi: 10.1016/j.agrformet.2020.108103
Ding Y., He X., Zhou Z., Hu J., Cai H., Wang X., et al. (2022). Response of vegetation to drought and yield monitoring based on NDVI and SIF. Catena 219, 106328. doi: 10.1016/j.catena.2022.106328
Forzieri G., Dakos V., McDowell N. G., Ramdane A., Cescatti A. (2022). Emerging signals of declining forest resilience under climate change. Nature 608 (7923), 534–53+. doi: 10.1038/s41586-022-04959-9
Garioud A., Valero S., Giordano S., Mallet C. (2021). Recurrent-based regression of Sentinel time series for continuous vegetation monitoring. Remote Sens. Environ. 263, 112419. doi: 10.1016/j.rse.2021.112419
Gensheimer J., Turner A. J., Kohler P., Frankenberg C., Chen J. (2022). A convolutional neural network for spatial downscaling of satellite-based solar-induced chlorophyll fluorescence (SIFnet). Biogeosciences 19 (6), 1777–1793. doi: 10.5194/bg-19-1777-2022
Gocic M., Trajkovic S. (2013). Analysis of changes in meteorological variables using Mann-Kendall and Sen's slope estimator statistical tests in Serbia. Glob. Planet. Change 100, 172–182. doi: 10.1016/j.gloplacha.2012.10.014
Guesewell S., Pohl M., Gander A., Strehler C. (2007). Temporal changes in grazing intensity and herbage quality within a Swiss fen meadow. Botanica Helv. 117 (1), 57–73. doi: 10.1007/s00035-007-0798-7
Guo B., Han F., Jiang L. (2018). An improved dimidiated pixel model for vegetation fraction in the yarlung zangbo river basin of qinghai-tibet plateau. J. Indian Soc. Remote Sens. 46 (2), 219–231. doi: 10.1007/s12524-017-0692-8
Guo H., Wang Y., Yu J., Yi L., Shi Z., Wang F. (2023). A novel framework for vegetation change characterization from time series landsat images. Environ. Res. 222, 115379. doi: 10.1016/j.envres.2023.115379
Guo W. B., Guo M. J., Tan Y., Bai E. H., Zhao G. B. (2019). Sustainable development of resources and the environment: mining-induced eco-geological environmental damage and mitigation measures-A case study in the henan coal mining area, China. Sustainability 11 (16). doi: 10.3390/su11164366
Gustavo C. V., Lorenzo B. (2009). Kernel methods for remote sensing data analysis (John Wiley & Sons, Ltd). doi: 10.1002/9780470748992
Han X. Y., Cao T. Y., Yan X. L. (2021a). Comprehensive evaluation of ecological environment quality of mining area based on sustainable development indicators: a case study of Yanzhou Mining in China. Environ. Dev. Sustain. 23 (5), 7581–7605. doi: 10.1007/s10668-020-00935-3
Han Y., Ke Y. H., Zhu L. J., Feng H., Zhang Q., Sun Z., et al. (2021b). Tracking vegetation degradation and recovery in multiple mining areas in Beijing, China, based on time-series Landsat imagery. GIScience Remote Sens. 58 (8), 1477–1496. doi: 10.1080/15481603.2021.1996319
He D. K., Le B. T., Xiao D., Mao Y. C., Shan F., Ha T. T. L. (2019). Coal mine area monitoring method by machine learning and multispectral remote sensing images. Infrared Phys. Technol. 103. doi: 10.1016/j.infrared.2019.103070
Huang S., Tang L. N., Hupy J. P., Wang Y., Shao G. F. (2021). A commentary review on the use of normalized difference vegetation index (NDVI) in the era of popular remote sensing. J. For. Res. 32 (1), 1–6. doi: 10.1007/s11676-020-01155-1
Huang C. L., Yang Q. K., Guo Y. H., Zhang Y. Q., Guo L. A. (2020). The pattern, change and driven factors of vegetation cover in the Qin Mountains region. Sci. Rep. 10 (1). doi: 10.1038/s41598-020-75845-5
Jiang H., Fan G. W., Zhang D. S., Zhang S. Z., Fan Y. B. (2022). Evaluation of eco-environmental quality for the coal-mining region using multi-source data. Sci. Rep. 12 (1). doi: 10.1038/s41598-022-09795-5
Jiang L., Liu Y., Wu S., Yang C. (2021). Analyzing ecological environment change and associated driving factors in China based on NDVI time series data. Ecol. Indic. 129, 107933. doi: 10.1016/j.ecolind.2021.107933
Jimenez R. B., Lane K. J., Hutyra L. R., Fabian M. P. (2022). Spatial resolution of Normalized Difference Vegetation Index and greenness exposure misclassification in an urban cohort. J. Expo. Sci. Environ. Epidemiol. 32 (2), 213–222. doi: 10.1038/s41370-022-00409-w
Li J., Garshick E., Al-Hemoud A., Huang S., Koutrakis P. (2020). Impacts of meteorology and vegetation on surface dust concentrations in Middle Eastern countries. Sci. Total Environ. 712. doi: 10.1016/j.scitotenv.2020.136597
Li S., Xu L., Jing Y., Yin H., Li X., Guan X. (2021b). High-quality vegetation index product generation: A review of NDVI time series reconstruction techniques. Int. J. Appl. Earth Obs. Geoinf. 105. doi: 10.1016/j.jag.2021.102640
Li P., Yu H. Y., Zhang J. S., Du M. Y., Xiong J. (2021a). Coal supply sustainability in China: A new comprehensive evaluation methodology. Front. Energy Res. 9. doi: 10.3389/fenrg.2021.701719
Liu H. Q., Huete A. (1995). A feedback based modification of the NDVI to minimize canopy background and atmospheric noise. IEEE Trans. Geosci. Remote Sens. 33 (2), 457–465. doi: 10.1109/TGRS.1995.8746027
Liu Y., Lei S., Chen X., Chen M., Zhang X., Long L. (2021a). Study of plant configuration pattern in guided vegetation restoration: A case study of semiarid underground mining areas in Western China. Ecol. Eng. 170, 106334. doi: 10.1016/j.ecoleng.2021.106334
Liu Z., Liu Y. (2018). Does anthropogenic land use change play a role in changes of precipitation frequency and intensity over the loess plateau of China? Remote Sens. 10 (11). doi: 10.3390/rs10111818
Liu Y., Zhao W., Chen S., Ye T. (2021b). Mapping crop rotation by using deeply synergistic optical and SAR time series. Remote Sens. 13 (20). doi: 10.3390/rs13204160
Luis R. Á. J., Manel M. R., Jordi M. M., Gustau C. V. (2018). Digital signal processing with kernel methods (John Wiley & Sons, Ltd).
Ma S., Qiu H., Zhu Y., Yang D., Tang B., Wang D., et al. (2023b). Topographic Changes, Surface Deformation and Movement Process before, during and after a Rotational Landslide. Remote Sens. 15 (3). doi: 10.3390/rs15030662
Ma M., Wang Q., Liu R., Zhao Y., Zhang D. (2023a). Effects of climate change and human activities on vegetation coverage change in northern China considering extreme climate and time-lag and -accumulation effects. Sci. Total Environ. 860, 160527. doi: 10.1016/j.scitotenv.2022.160527
Martinez A. D., Labib S. M. (2023). Demystifying normalized difference vegetation index (NDVI) for greenness exposure assessments and policy interventions in urban greening. Environ. Res. 220. doi: 10.1016/j.envres.2022.115155
Murwira A., Skidmore A. K. (2006). Monitoring change in the spatial heterogeneity of vegetation cover in an African savanna. Int. J. Remote Sens. 27 (11), 2255–2269. doi: 10.1080/01431160500396683
Pei Y., Qiu H., Yang D., Liu Z., Ma S., Li J., et al. (2023). Increasing landslide activity in the Taxkorgan River Basin (eastern Pamirs Plateau, China) driven by climate change. Catena 223, 106911. doi: 10.1016/j.catena.2023.106911
Pérez-Cabello F., Montorio R., Alves D. B. (2021). Remote sensing techniques to assess post-fire vegetation recovery. Curr. Opin. Environ. Sci. Health 21, 100251. doi: 10.1016/j.coesh.2021.100251
Pontius R. G., Shusas E., McEachern M. (2004). Detecting important categorical land changes while accounting for persistence. Agric. Ecosyst. Environ. 101 (2-3), 251–268. doi: 10.1016/j.agee.2003.09.008
Qi J. W., Zhang Y. C., Zhang J. Q., Wu C. Y., Chen Y. A., Cheng Z. S. (2023). Study on the restoration of ecological environments in mining area based on GIS technology. Sustainability 15 (7). doi: 10.3390/su15076128
Qiu H., Zhu Y., Zhou W., Sun H., He J., Liu Z. (2022). Influence of DEM resolution on landslide simulation performance based on the Scoops3D model. Geomatics Natural Hazards Risk 13 (1), 1663–1681. doi: 10.1080/19475705.2022.2097451
Roy R., Sultana S., Wang J. X., Mostofa M. G., Sarker T., Shah M. M. R., et al. (2022). Revegetation of coal mine degraded arid areas: The role of a native woody species under optimum water and nutrient resources. Environ. Res. 204. doi: 10.1016/j.envres.2021.111921
Shan W., Jin X., Ren J., Wang Y., Xu Z., Fan Y., et al. (2019). Ecological environment quality assessment based on remote sensing data for land consolidation. J. Clean. Prod. 239, 118126. doi: 10.1016/j.jclepro.2019.118126
Shang H., Zhan H. Z., Ni W. K., Liu Y., Gan Z. H., Liu S. H. (2022). Surface environmental evolution monitoring in coal mining subsidence area based on multi-source remote sensing data. Front. Earth Sci. 10. doi: 10.3389/feart.2022.790737
Sioris C. E., Zou J., McElroy C. T., Boone C. D., Sheese P. E., Bernath P. F. (2016). Water vapour variability in the high-latitude upper troposphere - Part 2: Impact of volcanic eruptions. Atmospheric Chem. Phys. 16 (4), 2207–2219. doi: 10.5194/acp-16-2207-2016
Siteur K., Eppinga M. B., Karssenberg D., Baudena M., Bierkens M. F. P., Rietkerk M. (2014). How will increases in rainfall intensity affect semiarid ecosystems? Water Resour. Res. 50 (7), 5980–6001. doi: 10.1002/2013WR014955
Song W., Feng Y., Wang Z. (2022). Ecological restoration programs dominate vegetation greening in China. Sci. Total Environ. 848, 157729. doi: 10.1016/j.scitotenv.2022.157729
Sun L., Li H., Wang J., Chen Y., Xiong N., Wang Z., et al. (2023). Impacts of climate change and human activities on NDVI in the qinghai-tibet plateau. Remote Sens. 15 (3). doi: 10.3390/rs15030587
Tong S., Zhang J., Ha S., Lai Q., Ma Q. (2016). Dynamics of fractional vegetation coverage and its relationship with climate and human activities in inner Mongolia, China. Remote Sens. 8 (9). doi: 10.3390/rs8090776
Wang X., Biederman J. A., Knowles J. F., Scott R. L., Turner A. J., Dannenberg M. P., et al. (2022b). Satellite solar-induced chlorophyll fluorescence and near-infrared reflectance capture complementary aspects of dryland vegetation productivity dynamics. Remote Sens. Environ. 270. doi: 10.1016/j.rse.2021.112858
Wang W., Liu R. Y., Gan F. P., Zhou P., Zhang X. W., Ding L. (2021a). Monitoring and evaluating restoration vegetation status in mine region using remote sensing data: case study in inner Mongolia, China. Remote Sens. 13 (7). doi: 10.3390/rs13071350
Wang Q., Moreno-Martinez A., Munoz-Mari J., Campos-Taberner M., Camps-Valls G. (2023). Estimation of vegetation traits with kernel NDVI. ISPRS J. Photogramm. Remote Sens. 195, 408–417. doi: 10.1016/j.isprsjprs.2022.12.019
Wang L., Qiu H., Zhou W., Zhu Y., Liu Z., Ma S., et al. (2022a). The post-failure spatiotemporal deformation of certain translational landslides may follow the pre-failure pattern. Remote Sens. 14 (10). doi: 10.3390/rs14102333
Wang Y., Wu X., He S., Niu R. (2021b). Eco-environmental assessment model of the mining area in Gongyi, China. Sci. Rep. 11 (1). doi: 10.1038/s41598-021-96625-9
Wu Q., Xu H., Yang Y., Hou H., Mi J., Wang X., et al. (2023). Identifying structure change of vegetation under long-term disturbance in the Shendong mining area. Environ. Earth Sci. 82 (19), 450. doi: 10.1007/s12665-023-11005-y
Xiao W., Zhang W. K., Ye Y. M., Lv X. J., Yang W. F. (2020). Is underground coal mining causing land degradation and significantly damaging ecosystems in semi-arid areas? A study from an Ecological Capital perspective. Land Degrad. Dev. 31 (15), 1969–1989. doi: 10.1002/ldr.3570
Xu Y., Guo L., Li J., Zhang C., Ran W., Hu J., et al. (2023b). Automatically identifying the vegetation destruction and restoration of various open-pit mines utilizing remotely sensed images: Auto-VDR. J. Clean. Prod. 414, 137490. doi: 10.1016/j.jclepro.2023.137490
Xu Q.-X., Wang T.-W., Cai C.-F., Li Z.-X., Shi Z.-H., Fang R.-J. (2013). Responses of runoff and soil erosion to vegetation removal and tillage on steep lands. Pedosphere 23 (4), 532–541. doi: 10.1016/S1002-0160(13)60046-6
Xu H., Xu F., Lin T., Xu Q., Yu P., Wang C., et al. (2023a). A systematic review and comprehensive analysis on ecological restoration of mining areas in the arid region of China: Challenge, capability and reconsideration. Ecol. Indic. 154, 110630. doi: 10.1016/j.ecolind.2023.110630
Xu Y., Yang Y., Chen X., Liu Y. (2022). Bibliometric analysis of global NDVI research trends from 1985 to 2021. Remote Sens. 14 (16). doi: 10.3390/rs14163967
Xu J., Zhu W., Xu J., Wu J., Li Y. (2021). High-intensity longwall mining-induced ground subsidence in Shendong coalfield, China. Int. J. Rock Mechanics Min. Sci. 141, 104730. doi: 10.1016/j.ijrmms.2021.104730
Yang X., Yao W., Li P., Hu J., Latifi H., Kang L., et al. (2022a). Changes of SOC content in China's shendong coal mining area during 1990-2020 investigated using remote sensing techniques. Sustainability 14 (12). doi: 10.3390/su14127374
Yang X. T., Yao W. Q., Li P. F., Hu J. F., Latifi H., Kang L., et al. (2022b). Changes of SOC content in China's shendong coal mining area during 1990-2020 investigated using remote sensing techniques. Sustainability 14 (12). doi: 10.3390/su14127374
Yu L., Liu Y., Liu T., Yan F. (2020). Impact of recent vegetation greening on temperature and precipitation over China. Agric. For. Meteorology 295, 108197. doi: 10.1016/j.agrformet.2020.108197
Yue S., Wang C. Y. (2004). The Mann-Kendall test modified by effective sample size to detect trend in serially correlated hydrological series. Water Resour. Manage. 18 (3), 201–218. doi: 10.1023/B:WARM.0000043140.61082.60
Zeng Y., Hao D., Park T., Zhu P., Huete A., Myneni R., et al. (2023). Structural complexity biases vegetation greenness measures. Nat. Ecol. Evol 7, 1790–1798. doi: 10.1038/s41559-023-02187-6
Zhang M., Lin H., Long X., Cai Y. (2021). Analyzing the spatiotemporal pattern and driving factors of wetland vegetation changes using 2000-2019 time-series Landsat data. Sci. Total Environ. 780, 146615. doi: 10.1016/j.scitotenv.2021.146615
Zhang J., Xiao J., Tong X., Zhang J., Meng P., Li J., et al. (2022). NIRv and SIF better estimate phenology than NDVI and EVI: Effects of spring and autumn phenology on ecosystem production of planted forests. Agric. For. Meteorology 315, 108819. doi: 10.1016/j.agrformet.2022.108819
Keywords: kNDVI (kernel normalized difference vegetation index), vegetation coverage, spatio-temporal changes, Sen’s + Mann-Kendall trend analysis, intensity analysis, Shendong coal mine
Citation: Chen Z, Zhang X, Jiao Y, Cheng Y, Zhu Z, Wang S and Zhang H (2023) Investigating the spatio-temporal pattern evolution characteristics of vegetation change in Shendong coal mining area based on kNDVI and intensity analysis. Front. Ecol. Evol. 11:1344664. doi: 10.3389/fevo.2023.1344664
Received: 26 November 2023; Accepted: 11 December 2023;
Published: 22 December 2023.
Edited by:
Haijun Qiu, Northwest University, ChinaReviewed by:
Jinping Liu, North China University of Water Resources and Electric Power, ChinaPei Liu, Hainan Academy of Ocean and Fisheries Sciences, China
Copyright © 2023 Chen, Zhang, Jiao, Cheng, Zhu, Wang and Zhang. This is an open-access article distributed under the terms of the Creative Commons Attribution License (CC BY). The use, distribution or reproduction in other forums is permitted, provided the original author(s) and the copyright owner(s) are credited and that the original publication in this journal is cited, in accordance with accepted academic practice. No use, distribution or reproduction is permitted which does not comply with these terms.
*Correspondence: Zhichao Chen, Y3pjQGhwdS5lZHUuY24=