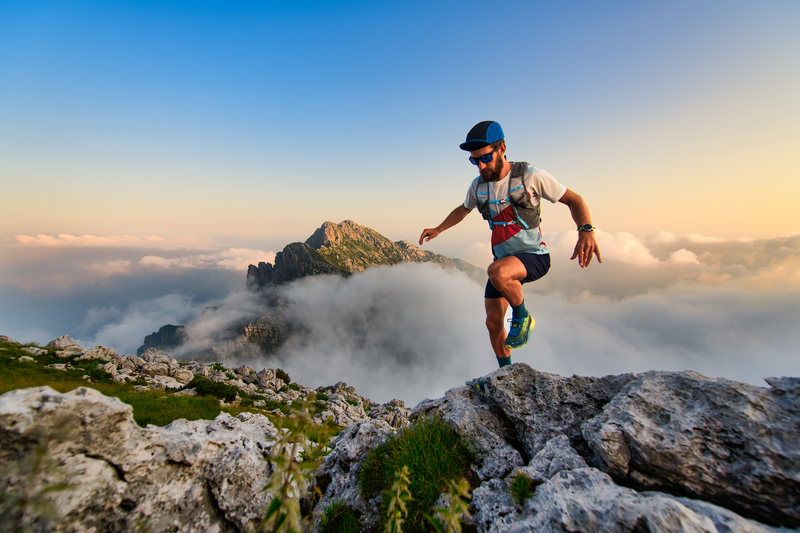
95% of researchers rate our articles as excellent or good
Learn more about the work of our research integrity team to safeguard the quality of each article we publish.
Find out more
ORIGINAL RESEARCH article
Front. Ecol. Evol. , 22 September 2023
Sec. Interdisciplinary Climate Studies
Volume 11 - 2023 | https://doi.org/10.3389/fevo.2023.1203075
This article is part of the Research Topic New Directions of Digital Economy, Energy Transition, and Climate Change in the Post-Covid-19 Era: Application of Machine Learning and Other Advanced Analytical Techniques View all 17 articles
Green and low-carbon development is the direction of the current technological revolution and industrial transformation, while China is still in the historical stage of deep industrialization and has yet to completely break away from the high-input, high-consumption, and high-emission development method, and is still facing serious challenges in terms of improving the efficiency of resource utilization and reducing pollution emissions. To effectively promote China’s industrial green transformation, it is necessary to accurately grasp its development connotations and scientifically realize the measurement of industrial green transformation. Therefore, this paper measures the efficiency of China’s industrial green transformation, based on the directional distance function and the Global Malmquist-Luenberger (GML) index, to portray its distribution dynamics, regional differences and further identify its growth drivers. The results found that the overall efficiency of China’s industrial green transformation has been steadily increasing, and that the regional pattern is characterized by northwestern, northeastern, central, eastern and southwestern regions, in that order. The Markov chain estimates show that industrial green transformation efficiency is most likely to remain in its original state, with probabilities of 88.31%, 63.54%, 42.86%, and 75.61% for low, medium-low, medium-high, and high levels respectively, but also has a jump shift characteristic, with a certain possibility of falling back from the high-efficiency state to the low state. Dagum Gini coefficient estimation results show that differences between groups in the five major regions are the main source of the widening differences in the overall industrial green transformation, with the contribution remaining at around 60%. Further research suggests that economic growth, technological progress, foreign trade, and foreign direct investment (FDI) may lead to a widening of the efficiency gap in industrial green transformation, while the industrial structure and outward foreign direct investment (OFDI) help to reduce spatial differences to some extent. Based on the above conclusions, this paper proposes some countermeasures to promote the overall improvement and coordinated development of China’s industrial green transformation.
Environmental degradation is closely related to the productive life of human society and directly affects the quality of economic and social development, which, if left unchecked, will have a serious and destructive impact on natural ecosystems and economic and social systems (Wang and Wang, 2023). Since the Industrial Revolution, the rapid growth of the global economy has met the material needs of humankind, and the development of the real economy, represented by the industrial sector, has been the fundamental engine of economic growth, but it has also left countries facing great challenges in terms of resources and the environment. The transformation of the original traditional extensive growth mode has become a global consensus, and the green transformation of industry is imperative. The Organization for Economic Cooperation and Development (OECD, 2005) suggests that in order for industry to achieve a green economy, it must rise to the level of “green transformation”, which connotes the realization of the transformation of the economy from unsustainable to sustainable development, the transformation of the “black” or “brown” economy to a green economy, as well as the transformation of low-quality development to a high-quality development model. Against the backdrop of tightening global pressure on both resources and the environment, the United Nations has proposed the 2030 Sustainable Development Goals, advocating that developing countries should follow a sustainable development path and avoid the “pollute first, treat later” development approach of developed countries.
With the reform and opening up and active integration into the international cycle, China’s industrial system has gradually improved and its industrial volume has expanded rapidly, making it the world’s number one industrial and trading nation (Yang et al., 2017; Shao et al., 2019; Wang et al., 2020a). However, a status quo that cannot be ignored is that the share of new technology and high-tech industrial sectors is relatively low, the economic growth drivers are still dominated by traditional industrial sectors, and the crude development model has not been fundamentally changed. Although spectacular economic growth has been achieved, the long-term expansionary use of resources has also pushed industrial economic growth close to the boundaries of ecological constraints, and the resource dividend is gradually being depleted (Yao et al., 2019; Gao and Yuan, 2022a). According to the Chinese Statistical Yearbook, industrial value added accounted for 31.0% of total GDP in 2020 but consumed 66.1% of final energy and emitted 79.8% of sulfur dioxide and 85.1% of carbon dioxide. Under the development trend that the green economy has become a new engine of global economic growth and a new advantage in international competition, China’s new industrialization process is bound to be affected. Thus, it is urgent to accelerate the green transformation of China’s industry (Chen et al., 2022a; Li et al., 2018). However, most current studies only cut in from a certain perspective to study its specific impact on industrial green transition, such as Hou et al. (2018) examined the impact of technological progress on industrial green transition, and Liu et al. (2022a) examined the impact of economic growth on industrial green transition. Yet fewer studies have examined industrial green transformation in depth from its own perspective.
To effectively promote China’s industrial green transformation, it is necessary to accurately grasp its development connotation and scientifically quantify the industrial green transformation. Therefore, this paper measures the efficiency of China’s industrial green transformation based on the directional distance function and GML index, portrays its distribution dynamics and regional differences in both time and space dimensions, and further identifies its growth drivers. The main contributions of this paper can be summarized as follows. Firstly, drawing on scholars such as (Cheng and Zervopoulos, 2014; Gao et al., 2021), this paper constructs a directional distance function based on a slack measure and GML index model to measure the efficiency of industrial green transformation, and incorporates CO2 in the non-desired output to demonstrate China’s industrial green transformation that takes into account the carbon attainment and carbon neutrality targets. Secondly, considering that the traditional three major regions may not accurately reveal the regular characteristics of China’s industrial green transformation, this paper combines natural, economic, and social development characteristics to regroup China’s 30 provinces into five major regions. Then we combine the use of kernel density estimation, Markov chains, and Dagum Gini coefficients to paint a more detailed picture of the evolutionary characteristics and spatial differences of China’s industrial green transformation, providing reference ideas for promoting the overall improvement of industrial green transformation in each region. Thirdly, a quantile model is used to examine the role of economic development, industrial structure, technological progress, foreign trade, FDI, and OFDI, to identify the key influencing factors of China’s industrial green transformation at different levels, and then to target the potential green values driving the industrial green transformation.
The rest of this paper is structured as follows. Section 2 reviews the literature on industrial green transformation. Section 3 introduces the methodology and data. Section 4 presents the measured results and evolutionary trends of industrial green transformation. Section 5 details the regional differences in industrial green transition and the sources of the differences. Section 6 discusses the factors influencing the industrial green transition. Finally, Section 7 draws conclusions and policy implications.
Since the industrial revolution, the rapid growth of the global economy has met the material needs of human beings, but it has also left countries facing huge challenges in terms of resources and the environment, such as the global warming problem caused by massive greenhouse gas emissions threatening sustainable economic development (Jordaan et al., 2017; Wang et al., 2020b), environmental pollution seriously affecting human health (Wei et al., 2018; Li et al., 2020), extreme weather reducing wheat production (Elahi et al., 2021) and Water stress in agriculture (Razzaq et al., 2022). Given the increasing resource depletion and environmental pollution, it has become a global consensus to change the original traditional extensive growth mode, and a green transformation of industry is imperative. The Organisation for Economic Co-operation and Development (OECD) suggests that for the industry to achieve a green economy, it must rise to the level of a “green transition”, which includes a shift from an unsustainable to a sustainable economy, a shift from a “black” or “brown” economy to a green economy, and a shift from a low-quality to a high-quality development model (OECD, 2005). Graedel et al. (2012) believes that the green transformation of industry connotes a shift in industrial development from crude to intensive and from highly polluting to less polluting. Kemp and Never (2017) state that industrial green transformation is an industrial green production process from unsustainable to sustainable, which is characterized by reduced environmental impact, reduced pollution emissions, increased production efficiency, increased resource utilization rate, and sustainable development (Du et al., 2021). Ran et al. (2023) argue that fundamental to China’s industrial green transformation is the promotion of sustained improvements in industrial green total factor productivity. Ren et al. (2022a) identify industrial green transformation as a series of shifts based on the transformation of the production function from one characterized by natural factor inputs to one characterized by green factor inputs, with the underlying growth drivers stemming from institutional change and technological change (Mao et al., 2019).
The existing measurement methods can be divided into two categories: the evaluation system method and the indicator selection method, where the indicator selection method can be divided into single-factor indicators and total-factor indicators.
In terms of constructing the evaluation system, scholars have used hierarchical analysis and entropy methods to fit the multi-dimensional evaluation system to quantify industrial green transformation. For example, Yuan et al. (2020b), Han et al. (2020), and Gao and Yuan (2022b), based on China’s Industrial Green Development Plan (2016–2020) and the Green Development Indicator System, build an evaluation index system for industrial green transformation from the aspects of industrial economic development, resource and environmental carrying capacity, and industrial structure optimization, etc. The advantage of the index system is that it has a wide coverage, but the tertiary indicators are mainly derived through subjective screening, which tends to duplicate information (Cao et al., 2021).
In terms of single factor indicators, Mensah et al. (2019) measure the industrial green transformation of OECD countries from three indicators: total CO2 emissions, production-side carbon emissions, and demand-side carbon emissions. Yu et al. (2018) and Mao et al. (2019) quantify the greening degree of industrial structure with the proportion of highly polluting industries. Liu and Chen (2022) directly take the number of green patent applications as the proxy variable of industrial green transformation. Such indicators visualize a particular feature of industrial green transformation, but also ignore the quality of economic growth and fail to capture the desirable outputs and undesirable outputs generated by energy consumption (Cheng et al., 2020).
In terms of total factor indicator, improving green total factor productivity (GTFP) is an important way to achieve industrial green transformation (Zhang et al., 2020). Since GTFP can reflect both desired and undesired outputs and the harmony between economic and ecological development, a large number of scholars have chosen this indicator to measure industrial green transformation (Wu et al., 2022; Yu et al., 2022; Zeng et al., 2023). In addition, some other scholars believe that the root of China’s industrial green transformation is to promote the continuous improvement of industrial green total factor productivity through technological innovation. Subsequently, based on this definition, many scholars have chosen industrial green total factor productivity as an indicator for measuring industrial green transformation. For example, Cheng et al. (2020) use the global Malmquist-Luenberger index to measure green total factor productivity to analyze the green transformation in 30 Chinese provinces. Qu et al. (2020) use NDDF and DEA methods to measure the green transformation efficiency of manufacturing industries in China. Tian et al. (2022) calculate the green transformation efficiency of enterprises in heavily polluting industries in China by using super-efficient SBM. Ran et al. (2023) measure China’s industrial green transition using industrial green total factor productivity calculated by the super-efficient SBM.
Domestic factors of industrial green transformation include economic level (Gao and Yuan, 2022b; Liu et al., 2022b), industrial structure (Lin and Wang, 2023; Lin and Xie, 2023), technological progress (Wu and Zhang, 2020; Yan et al., 2020), and environmental regulation (Hou et al., 2018; Guo and Yuan, 2020). In terms of influence of the economic level, Gai et al. (2022) believe that economic development is the direct driving force for the efficiency of industrial green development. Chen et al. (2022b) further find that this positive effect has a significant spatial spillover effect. In terms of industrial structure optimization, Yuan et al. (2020a) find that the impact of manufacturing agglomeration on green economic efficiency shows a positive U-shaped characteristic of first inhibiting and then promoting and emphasize that to achieve high-quality manufacturing development requires promoting industrial structure upgrading and reducing the congestion effect generated by industrial agglomeration. Similarly, Yang et al. (2023) points out that at this stage, China’s industrial green transformation needs to focus on the efficiency and quality of industrial development, rather than blindly pursuing quantity. In terms of R&D investment and technological progress, Li et al. (2019) and Fu et al. (2020) argue that the slow progress of green technology is an important limiting factor for the improvement of industrial green development, and that increasing investment in science and technology innovation and environmental protection can effectively improve industrial green development. In terms of environmental regulation, Zhai and An (2020) believe that it helps to achieve industrial green transformation. However, Sun et al. (2022) find that strict environmental regulation inhibits the contribution of technological progress to industrial green transformation in the eastern provinces of China. Yuan and Xiang (2018) and Li (2019) suggest that environmental regulation has not contributed to China’s industrial green development.
Under closed conditions, technological advancement depends only on the domestic stock of intellectual capital, whereas under open conditions, intellectual capital is characterized by cross-country diffusion (Pan et al., 2020), trade openness, foreign direct investment, and outward foreign direct investment all influence industrial green transformation. For trade opening, some scholars argue that trade liberalization will facilitate the spillover and diffusion of advanced and clean technologies, helping to promote industrial green transformation (Hao et al., 2021). For example, Ding et al. (2022) find that trade openness has contributed to the green transformation of Chinese industry using provincial panel data from China. The opposing view is that trade expansion increases production, which requires more energy to be consumed, thus increasing pollution emissions (Xu et al., 2020). For example, Yu et al. (2022) use cross-country panel data to find that imports and exports contribute to green productivity in high-income countries, but not in low-income countries. Ren et al. (2014) find that a widening trade surplus is an important cause of increased industrial pollution emissions in China. In terms of foreign direct investment, Liu et al. (2022a) discuss the impact of FDI on industrial green transformation in terms of both quantity and quality using a provincial panel in China. They find that FDI quality has no significant effect on industrial green transformation and that FDI quantity inhibits industrial green transformation in neighboring areas. Qiu et al. (2021) finds that the impact of FDI on industrial green transformation has both pollution halo and pollution paradise effects, and that environmental regulation and policy guidance can weaken the negative effect of FDI. Hu et al. (2018) develop a discussion that foreign direct investment entry has a promoting effect on the green transformation of industrial sectors that are greener but has a restraining effect on less green industries. In terms of outward foreign direct investment, through cross-border M&As and greenfield investment, OFDI not only broadens the international market for multinational enterprises, but also enables them to gain access to key technologies in the host country, thus forming reverse technological spillovers, promoting the productivity and technological innovation capacity of the home country and influencing the high industrial green transformation (Piperopoulos et al., 2018; Hao et al., 2020). For example, Kong et al. (2021) find that market-seeking OFDI provides a channel for the transfer of gradually saturated production capacity in home countries, which in turn provides more scope for domestic production segments to adjust to higher value-added segments, helping to promote industrial green transformation. Zhang (2022) believes that in the face of high environmental standards in developed countries, multinational companies will continue to develop and innovate new technologies to achieve green production to gain a more stable market share, and that the application of R&D results in home countries will help to achieve industrial green transformation. Peng et al. (2023) point out that OFDI can improve the sustainable productive capacity of Chinese industrial firms, but the impact is heterogeneous depending on the absorptive capacity in terms of human capital, R&D intensity, and technology gaps.
Industrial green transformation should balance production efficiency and environmental quality. It is difficult for a single indicator to measure both factors simultaneously, while an evaluation system built through subjectivity tends to duplicate information (Cao et al., 2021), therefore, referring to Cheng and Zervopoulos (2014); Gao et al. (2021), this paper quantifies industrial green transformation using industrial green total factor productivity measured by the DDF-GML method.
Firstly, construct an undesired output efficiency model and an expected output efficiency model based on the DDF. According to Chung et al. (1997), assuming that there are n DMUs, each with i inputs , yielding j desired outputs and m non-desired outputs , and let the directionality vector be and represent each period, the DDF for period t is:
where is a vector of capital, labor, and energy inputs; and denote the vector of desired and undesired outputs, respectively; and is the value of the directional distance function that maximizes desired output and minimizes undesired output is the set of production possibilities, which includes both desired and undesired outputs, and the undesired outputs are weakly disposable.
Secondly, propose the non-desired output efficiency model and desired output efficiency model by specifically varying the above directional vectors. The non-desired output efficiency model is:
where a is the optimal solution of the non-desired output efficiency model; x, y, and b denote the factor input, desired output, and non-desired output values respectively; and c is a vector of weight coefficients relative to the DMU being evaluated in the effective DMU portfolio. is the ratio of the potential optimal non-desired output to the actual non-desired output of the decision unit under the given conditions of factor inputs and desired outputs. The higher the value of , the lower the potential for a reduction in the undesired outputs of the DMU.
And the desired output efficiency model is:
where is the desired output expansion potential under the non-desired output constraint, the higher the value of , the greater the desired output expansion potential of the DMU.
Finally, the GML index analysis method is used to measure the efficiency under environmental constraints. According to Oh (2010), the GML index from period t to t+1 is defined as:
If industrial activities produce more desired output and less undesired output, then GMLt,t+1> 1, indicating higher productivity and contributing to industrial green transformation; if they produce less desired output and more undesired output, then GMLt,t+1< 1, indicating lower productivity and inhibiting industrial green transformation.
The Dagum Gini coefficient method is used to systematically analyze the regional differences in China’s industrial green transformation. According to Dagum (1997), the inter-group Gini coefficient can be calculated as Eq.(5):
where h and k are two different regions, and are the number of provinces in each region, and denote the level of industrial green transformation of province i in region h and province l in region k, respectively, and denote the mean value of the level of industrial green transformation of all provinces in the corresponding region. When the two provinces involved in the calculation are in the same region, the result is the intra-group Gini coefficient ().
Further, assuming the existence of n provinces divided into m regions and defining , , the overall Dagum Gini coefficient is calculated as follows:
where is the total contribution of intra-regional variation to the overall industrial green transition variation, is the total contribution of all inter-regional variation to the overall variation; is the relative influence of the level of industrial green transition between two different regions, calculated as:
In the Eq.(10)–(12), represents the total influence between the industrial green transformation of region h and region k. is the hypervariable first order moment between region h and region k. and are the cumulative distribution functions of industrial green transformation in region h and region k.
Markov chain is a horizontal space of a stochastic process that reflects the distribution and evolutionary trends at different types of time and states by dividing continuous discrete values into N types. For any period t and possible types i, j, and jk (k=0,1,…, t-2), the Markov chain satisfies Eq.(13), from which it can be argued that the probability of an industrial green transition efficiency being of type i in period t+1 depends only on its type in period t.
Then, the quartile method is used to divide all industrial green transformation efficiency values in the sample period into four levels: low, medium-low, medium-high, and high, on average according to their magnitudes, and a dimensional probability matrix of industrial green transformation type transfer can be obtained through Markov chains, as in Eq.(14).
where represents the probability that a region is of type j in period t and shifts to type i in period t+1. The shifting probability is calculated by using a maximum likelihood estimate, calculated as in Eq.(15):
where represents the number of times that industrial green transition efficiency shifted from type j to type i during the observation period, and is the total number of occurrences of type j.
The quantile regression method is more precise than OLS in describing the effects of explanatory variables on the range of variation in the explanatory variable and the shape of the conditional distribution. Following the cross-sectional quantile, Koenker (2004) proposed a panel quantile, combining the quantile regression method with a panel data model, further extending the application of the quantile regression method. To investigate the underlying causes of the spatial and temporal evolutionary characteristics of China’s industrial green transition, a fixed effect panel quantile model was used, following Powell (2022), and an adaptive Monte Carlo method was selected for estimation, with five representative quantile levels estimated: 10%, 25%, 50%, 75%, and 90%.
Based on data completeness and comparability, this paper finally uses data for 30 Chinese provinces for the period 2004-2020 from the China Industrial Statistics Yearbook, the China Energy Statistics Yearbook, the China Environment Yearbook, the CEADs database, the China Statistical Yearbook, and the Foreign Direct Investment Statistics Bulletin1.
For the calculation of the industrial green transition indicator, the industrial sectors of each Chinese province from 2003 to 2020 are used as the production decision unit. The input factors are labor, capital, and energy. And labor input is measured using the average number of workers employed by industrial enterprises above the scale, capital input is measured using the average annual balance of net fixed assets, and energy input is measured using industrial end-use consumption. Desired output is measured using industrial sales output. Undesired outputs include industrial emissions of sulfur dioxide, chemical oxygen demand in wastewater, solid waste, and carbon dioxide. Using 2003 as the base period, capital input is deflated using the fixed asset investment price index and the desired output is deflated using the ex-factory industrial price index, in order to exclude price factors.
For the analysis of the factors influencing the spatial and temporal evolution of the industrial green transition, two types of indicators are selected. The first category is domestic factors, including economic growth, industrial structure, and technological progress. The second category is international factors, including foreign trade, foreign direct investment, and outward foreign direct investment. Specific definitions are listed in Table 1.
The results of the industrial green transition efficiency calculations for each province in China are shown in Table 2, based on matlab2021. From an overall perspective, the efficiency of China’s industrial green transformation shows a steady upward growth trend, with the national average rising from 1.0107 in 2004 to 1.2922 in 2020, an average annual increase of 1.65%, indicating that China’s industrial green transformation has steadily advanced and made some progress. In terms of regional comparisons, the Northwest region has the highest industrial green transition efficiency with an in-sample mean of 1.2559, followed by the Northeast, Central, Eastern, and Southwest, and the Southwest region with a mean of 1.08062. In terms of comparison of transformation among provinces, the top three industrial green transformation efficiency rankings in 2004 are Tianjin, Hebei, and Guangdong, distributed in the eastern region; by 2020, the top three are Qinghai, Beijing, and Jilin, distributed in the northwest, east and northeast regions respectively; furthermore, Qinghai, Gansu, and Inner Mongolia ranked the top three in terms of the annual average of industrial green transformation efficiency, distributed in the northwest region. The change in the ranking of the transformation indicates that China’s industrial green transformation is characterized by a “geese formation” with the eastern part leading the way and other regions following, that is, a gradient transformation.
To further investigate more precisely the dynamic evolution of the distribution of industrial green transformation, a kernel density map is drawn to portray the overall shape of the efficiency of China’s industrial green transformation. According to Figure 1, the dynamic evolution of the distribution is characterized by three aspects. Firstly, the distribution of industrial green transition efficiency shifted to the right overall, indicating that industrial green transition efficiency in each region has gradually improved. Secondly, the height of the wave decreases, becomes flatter, and becomes wider, indicating a gradual widening of the gap in industrial green transformation efficiency across regions. Thirdly, the distribution of industrial green transition efficiency extends and widens, meaning that the gap between the extremes and the mean within the region does not gradually narrow, with some provinces and municipalities maintaining higher or lower industrial green transition efficiency. The task of China’s industrial green transformation has remained arduous, possibly because China is in the mid-to-late stages of industrialization, with higher levels of energy consumption due to greater resource dependence on the one hand, and lower value added due to a lower industrial chain on the other.
To predict the trend characteristics of China’s industrial green transformation, the quartile method is first used to classify industrial green transformation efficiency into four levels, low (L), medium-low (ML), medium-high (MH), and high (H). And the five regions are similarly graded. The Markov chain method is then used to obtain a probability transfer matrix as shown in Table 3. The results show that:
There is a general club convergence effect in China’s industrial green transformation efficiency across the country and the five regions, and a “low-level trap” and “high-level monopoly” phenomenon. In the national probability transfer matrix, the probability values on the main diagonal are greater than the values on the non-main diagonal, and the probabilities of low, medium-low, medium-high, and high-level provinces maintaining their status one year after industrial green transformation are 88.31%, 63.54%, 42.86% and 75.61% respectively, indicating that the different levels of industrial green transformation efficiency are more stable and there is a club convergence effect. The eastern, northeast, and northwest show club convergence in all four categories. The central region shows club convergence in three categories except for the high-level group. And the southwest region only has the characteristic of club convergence in the low and middle-low levels. In addition, the probabilities of the low-level industrial green transformation group maintaining its status in the whole country and five regions are 88.31%, 90.27%, 89.66%, 76.00%, 93.94%, and 80.43%, respectively, all of which are much higher than the probability of upward shift, indicating the existence of a “low-level trap”. The probabilities of high-level industrial green transformation group maintaining their status are 75.61%, 85.71%, and 81.48% for the whole country, eastern and northwestern regions respectively, while the central, northeastern, and southwestern regions have a lower number of high-level industrial green transformation efficiency and a lower transfer probability. This indicates that industrial green transformation on the whole is characterized by a “high-level monopoly”, which originates from the higher level of green transformation in some provinces in the eastern and northwestern regions.
The level of industrial green transformation is characterized by a “leapfrog” shift and a long-term growth trend, but care needs to be taken to prevent a regression in industrial green transformation. From the whole nation, the transfer not only occurs between adjacent levels, but also exists a “leapfrog” transfer from low level to medium-high level or even high level. This transfer comes from the leap in the north-western provinces, such as Qinghai, Gansu, and Xinjiang, which have jumped from medium to high levels nationally. At the same time, the probabilities of shifting to the right of the main diagonal are greater than the probability of shifting to the left, implying a long-term growth trend in industrial green transformation. Further, the probabilities of upward transfer after one year are 11.69%, 22.92%, and 31.43% for low, medium-low and medium-high levels respectively, which shows that the probability of upward transfer of China’s industrial green transformation increases after crossing the low level. While the probabilities of downward transfer after one year are 13.54%, 25.71%, and 24.39% for medium-low, medium-high, and high levels respectively. This indicates that there is a certain risk that the level of China’s industrial green transformation will fall and may drop from a high level to a medium-low level in a “precipitous” manner. Therefore, all provinces should be alert to the risk of a downward transfer, prevent a reversal of industrial green transformation, keep the existing development results solid and strive to achieve an upward transfer. From the perspective of the five regions, the eastern, central, and southwestern regions do not have the characteristics of “leapfrog” transfer. In the northeast, the probability of downward transfer is higher than the probability of upward transfer, while the opposite is true in the northwest.
In order to more intuitively grasp the regional differences, the Dagum Gini coefficient analysis method is used to calculate and decompose the overall variation of China’s industrial green transformation from 2004 to 2020. As can be seen from the line graph presented in Figure 2, the overall variation in China’s industrial green transformation over the sample period shows an upward trend. Specifically, the overall variation of the industrial green transformation is only 0.0155 in 2004, and after reaching its first peak in 2011, it has remained high, showing a fluctuating and rising “W”-shaped trend. By 2020, the overall variation is 0.0538, an increase of 2.47 times, indicating that the gap in China’s industrial green transformation among the provinces is widening. The bar chart shows the decomposition of the overall difference in China’s industrial green transformation. It can be seen from the changing trend that the between-group variation accounts for a relatively high proportion, suggesting that the change of the overall variation may depend more on between-group variation. The intra-group variation remained essentially at the same level, indicating a small variation within the region. And the supervariable density function tends to decline after a “W” pattern of “gentle decline–rapid upward movement–gentle downward movement”, indicating that the overlap between regions has less impact on the overall variation. Comparing the development of the contribution rates of the three variations, although the percentages of the three have shown a tendency to change over the sample period, the contribution rate of between-group variation to the overall variation has always remained around 60%, except for a few years, which exceeds the total contribution rate of within-group variation and supervariable density, thus indicating that the spatial differences in the level of China’s industrial green transformation mainly come from the differences among the five regions, and how to narrow the transformation gap among regions is a key direction for future efforts. This result is similar to the findings of Zhang et al. (2022). They concluded that the key to improving industrial competitiveness in China is also to reduce inter-regional differences.
There is regional heterogeneity in the upward trend of variation within the five regions. As shown in Table 4, in general, the within-group differences all exhibit a fluctuating upward trend of variation. The largest increase in intra-group variation is observed in the northwest, followed by the eastern, southwest, and central regions, with the smallest increase in the northeast. In terms of values, the intra-group variation in the southwest is consistently the largest among the five regions, indicating a high degree of imbalance in Southwest provinces’ industrial green transformation. For example, Yunnan’s industrial green transformation efficiency in 2020 is 1.3591, while Guangxi in the same year is only 0.8798, a large gap between the two. The northwest ranks second, but unlike the southwest, the intra-group variation in the northwest has undergone an upward trend from low to high, especially since 2018, evolving in a “sharp upward” trend. Besides, the differences within the northeast region are relatively small, compared with the eastern and central regions. It may be that the provinces and cities in the eastern and central regions show a “wild goose mode” of industrial development, with both “star” provinces and relatively “mediocre” provinces. While the three provinces in the northeast have the same industrial base, are closely linked and have a faster technology transfer. This comparison shows that attention should be paid to the coordinated development within the eastern and central regions.
Figure 3 plots the Between-group variation in industrial green transformation among the five regions. In terms of the overall trend, the gradual increase in the shaded area in the figure indicates that the level of industrial green transformation among China’s five regions is differentiated, with some provinces able to rapidly advance their industrial green transformation in terms of both optimizing industrial structure and fostering or introducing new technologies for green production, while other regions are constrained by the historical baggage of slow progress. In terms of differences between regions, the differences between the eastern, central, and northeast are small, with the difference between the eastern and central is the lowest in the full sample, with a mean value of 0.0300. Large values of differences are all derived from the southwest and other regions. For example, the difference between the southwest and northwest has the largest value in the sample period, with a mean of 0.0758, twice as large as the difference between the eastern and central. In terms of time-varying trends in inter-regional differences, the most significant increases in differences between the northwest and other regions, such as 10.28%, 9.92%, and 9.64% between central and northwest, southwest and northwest, and eastern and northwest, respectively, ranked among the top three increases in differences between regions.
For investigating the deep-seated reasons for the spatial-temporal evolution characteristics of China’s industrial green transformation, the results were examined with the help of a quantile model, as shown in Table 5.
In terms of domestic factors, the estimated coefficients of lnpgdp, stru, and tech are significantly positive in all quartiles, indicating that economic development, industrial structure optimization, and technological progress significantly improve industrial green transformation efficiency. However, the manifestations of the improving effect are different. The coefficient of lnpgdp is positive at all quantile levels and shows an increasing trend as the quantile level increases, implying that economic development contributes more significantly to provinces with more efficient industrial green transformation. The coefficient of stru undergoes an “inverted U-shaped” process of change, which means that there is an optimal interval for the promotion of industrial green transformation by adjusting the ratio of the third sector to the second sector. And the promotion of industrial green transformation by industrial structure is more obvious when the efficiency of industrial green transformation is at an intermediate level. The magnitude of the coefficient of tech is second only to lnpgdp, indicating that technological progress is an important driver of industrial green transformation, but the value of the coefficient falls back at the 90 percentiles, probably because the contribution of science and technology carried by the number of patents granted gradually falls back when the green transformation of industry is more efficient. At this time, more attention should be paid to the development and application of green technology. As found by Qing et al. (2022), proactive green innovation, including process innovation and product innovation, has a significant positive effect on improving corporate earnings.
In terms of international factors, the estimated coefficients of trade and lnfdi are significantly negative at all quartiles, and the coefficient of lnofdi is significantly positive, indicating that foreign trade and foreign direct investment inhibit China’s industrial green transformation, while outward foreign direct investment promotes it. Specifically, the coefficient of trade is at a low level until the 75 percentiles, but the inhibiting effect increases significantly at the 90 percentiles. The possible reason is that China used to be in a low position in the international division of labor system, which to some extent led to the restructuring of import and export trade inhibiting the green transformation of industry. The magnitude of the coefficient of lnfdi gradually decreases with the quantile, probably because foreign investors have transferred some highly polluting enterprises to China, but as the efficiency of China’s industrial green transformation continues to increase, such enterprises gradually increase their green output under the influence of competitive effects, reducing the inhibiting effect on industrial green transformation. Unlike the other two factors, the estimated coefficient of lnofdi is positive at all quartiles, contributing to the industrial green transformation and showing a “U-shaped” change characteristic of first decreasing and then increasing.
Industrial green transformation is an important element in achieving Chinese-style modernization and has attracted extensive attention from scholars, but a review of the literature reveals a relative lack of research on the evolution of trends, regional differences, and causes. Therefore, this paper uses China’s provincial panel data from 2004-2020 to measure the efficiency of industrial green transformation adopting the directional distance function and the GML index and carries out systematic verification and analysis based on kernel density estimation, Markov chain analysis, the Dagum Gini coefficient, and the quantile model. The following main findings are obtained: (1) From the measurement results, the efficiency of China’s industrial green transformation has steadily increased over the sample period, showing a decreasing distribution of the northwest, northeast, central, eastern, and southwest in that order. The northwestern and northeastern provinces have the highest annual average value increase, making the top three provinces shift from being exclusively shared by the east to being shared by the northwest, east and northeast. (2) From the distribution characteristics, the industrial green transformation efficiency shows the characteristics of “ transformation efficiency increasing and absolute difference expanding”. In addition, there is a “low-level trap” and a “high-level monopoly” in China’s industrial green transformation, and it is most likely to remain in its original state, but it also has the characteristic of “jumping” transfer. There is also a certain risk that the industrial green transition will fall in rank, especially if the medium-high rank is reduced to a medium-low rank. (3) From the regional differences, the overall Gini coefficient shows a fluctuating upward “W” trend, highlighting the widening gap in industrial green transformation efficiency among provinces. The decomposed results show that the differences are mainly between groups that is, the differences between the five regions, and the intra-group differences are also increasing. (4) From the influencing factors, the absolute values of the estimated coefficients of economic growth, technological progress, foreign trade, and FDI expand as the quantile point increases, suggesting that the factors above may pull the industrial green transformation efficiency to achieve rapid improvement (reduction) in higher level provinces, while relatively slow improvement (reduction) in lower level provinces, thus leading to the expansion of overall spatial differences to a certain extent. The estimated coefficients of industrial structure and OFDI help to narrow the spatial differences.
According to the research conclusion, we obtain the following policy implications: (1) we should face up to the shortcomings in the process of industrial green transformation and prevent the efficiency of industrial green transformation from “backtracking”. Provinces should focus on the relationship between “quantity” and “quality” in the process of promoting industrial green transformation, as the two are not separate. In particular, for provinces locked in low levels of transformation efficiency and at risk of declining grades, it is all the more important to achieve a significant improvement in quality while maintaining quantitative growth. (2) Pay attention to regional differences in the process of industrial green transformation, especially the development differences among the five major regions. In the process of building a unified domestic market, exchanges and cooperation between regions should be further strengthened, resources should be rationally allocated, a synergy of industrial green transformation should be formed, and regional development imbalances should be prevented from further widening. Although the efficiency of industrial green transformation has increased fastest in the northwest, the eastern provinces have strong economic strength and always have a leading edge, so the eastern region needs to take on more tasks of tackling cutting-edge technologies and the cost of pilot tolerance. The southwest region, on the other hand, has the lowest efficiency of transformation, so the priority is to improve the efficiency of transformation by learning from successful transformation experiences. (3) Make full use of both domestic and international markets and resources to promote industrial green transformation. Based on the identification of key influencing factors at different sub-levels of industrial green transformation, will help provinces to introduce policies to promote industrial green transformation according to the characteristics of the region. For provinces with a higher industrial green transformation efficiency, they can increase investment in green technology research and development and adjust their industrial structure, which will not only help them realize their industrial green transformation, but also generate spillover effects and play a leading role. For provinces and cities with a lower efficiency rating, they can develop their economies and raise their GDP per capita, and in addition, they can increase OFDI, which will help accelerate the industrial green transformation to a higher value.
Although this paper discusses the evolutionary characteristics and influencing factors of China’s industrial green transformation, there are still some limitations that deserve further study. Firstly, this paper uses kernel density estimation, Markov chain method and Dagum Gini coefficient to demonstrate the evolution trend, transfer probability and regional differences of China’s industrial green transformation, but neglects the convergence analysis between regions, which is worthy of further discussion. And in the future, it can be combined with the measure of spatial convergence to examine the convergence of industrial green transformation under the condition of spatial interconnection. In addition, this paper analyzes China’s industrial green transformation only at the regional level, however, China has many industrial sub-divisions, which can be classified as labor-intensive, capital-intensive, and technology-intensive, so further attention can be paid to the green transformation of different types of industrial sectors in the future.
Publicly available datasets were analyzed in this study. This data can be found here: the China Industrial Statistics Yearbook, the China Energy Statistics Yearbook, the China Environment Yearbook, the CEADs database, the China Statistical Yearbook, and the Foreign Direct Investment Statistics Bulletin.
CL: Conceptualization, Writing – review & editing, Writing – original draft. JX: Writing – review & editing, Methodology, Validation, funding acquisition. JZ: Conceptualization, Formal analysis, Writing – review & editing.
This research was supported by the Project of Xinjiang Social Science Fund of China (Grant No. 2023CYJ041) and the PhD Graduate Innovation Project of Xinjiang Uygur Autonomous region (Grant No. XJ2023G008).
The authors declare that the research was conducted in the absence of any commercial or financial relationships that could be construed as a potential conflict of interest.
All claims expressed in this article are solely those of the authors and do not necessarily represent those of their affiliated organizations, or those of the publisher, the editors and the reviewers. Any product that may be evaluated in this article, or claim that may be made by its manufacturer, is not guaranteed or endorsed by the publisher.
Cao J., Law S. H., Samad A. R. B. A., Mohamad W. N. B. W., Wang J., Yang X. (2021). Impact of financial development and technological innovation on thevolatility of green growth-evidence from China. Environ. Sci. pollut. Res. Int. 28, 48053–48069. doi: 10.1007/s11356-021-13828-3
Chen H., Shi Y., Xu M., Xu Z., Zou W. (2022a). China's industrial green development and its influencing factors under the background of carbon neutrality. Environ. Sci. Pollut. Res. Int. 30, 81929–81949. doi: 10.1007/s11356-022-23636-y
Chen H., Yang Y., Yang M., Huang H. (2022b). The impact of environmental regulation on China's industrial green development and its heterogeneity. Front. Ecol. Evol. 10, 967550. doi: 10.3389/fevo.2022.967550
Cheng Z., Li L., Liu J. (2020). Natural resource abundance, resource industry dependence and economic green growth in China. Resour Policy 68, 101734. doi: 10.1016/j.resourpol.2020.101734
Cheng G., Zervopoulos P. D. (2014). Estimating the technical efficiency of health care systems: A cross-country comparison using the directional distance function. Eur. J. Oper Res. 238, 899–910. doi: 10.1016/j.ejor.2014.05.007
Chung Y. H., Färe R., Grosskopf S. (1997). Productivity and undesirable outputs: A directional distance function approach. J. Environ. Manage 51, 229–240. doi: 10.1006/jema.1997.0146
Dagum C. (1997). Decomposition and interpretation of Gini and the generalized entropy inequality measures. Statistica 57, 295–308. doi: 10.6092/issn.1973-2201/1060
Ding L., Wu M., Jiao Z., Nie Y. (2022). The positive role of trade openness in industrial green total factor productivity-provincial evidence from China. Environ. Sci. pollut. Res. Int. 29, 6538–6551. doi: 10.1007/s11356-021-16164-8
Du K., Cheng Y., Yao X. (2021). Environmental regulation, green technology innovation, and industrial structure upgrading: The road to the green transformation of Chinese cities. Energy Econ 98, 105247. doi: 10.1016/j.eneco.2021.105247
Elahi E., Khalid Z., Tauni M. Z., Zhang H. X., Xing L. R. (2021). Extreme weather events risk to crop-production and the adaptation of innovative management strategies to mitigate the risk: A retrospective survey of rural Punjab, Pakistan [J]. Technovation 117, 102255. doi: 10.1016/j.technovation.2021.102255
Fu Y., Supriyadi A., Wang T., Wang L., Cirella G. T. (2020). Effects of regional innovation capability on the green technology efficiency of China's manufacturing industry: evidence from listed companies. Energies (Basel) 13, 5467. doi: 10.3390/en13205467
Gai Y., Qiao Y., Deng H., Wang Y. (2022). Investigating the eco-efficiency of China's textile industry based on a firm-level analysis. Sci. Total Environ. 833, 155075. doi: 10.1016/j.scitotenv.2022.155075
Gao K., Yuan Y. (2022a). Spatiotemporal pattern assessment of China's industrial green productivity and its spatial drivers: Evidence from city-level data over 2000-2017. Appl. Energy 307, 118248. doi: 10.1016/j.apenergy.2021.118248
Gao K., Yuan Y. (2022b). Does market-oriented reform make the industrial sector “Greener” in China? Fresh evidence from the perspective of capital-labor-energy market distortions. Energy (Oxf) 254, 124183. doi: 10.1016/j.energy.2022.124183
Gao Y., Zhang M., Zheng J. (2021). Accounting and determinants analysis of China's provincial total factor productivity considering carbon emissions. China Econ Rev. 65, 101576. doi: 10.1016/j.chieco.2020.101576
Graedel T. E., Allenby B. R., Comrie P. R. (2012). Matrix approaches to abridged life cycle assessment. Environ. Sci. Technol. 29, 134A–139A. doi: 10.1021/es00003a751
Guo R., Yuan Y. (2020). Different types of environmental regulations and heterogeneous influence on energy efficiency in the industrial sector: Evidence from Chinese provincial data. Energy Policy 145, 111747. doi: 10.1016/j.enpol.2020.111747
Han D., Li T., Feng S., Shi Z. (2020). Does renewable energy consumption successfully promote the green transformation of China's industry? Energies (Basel) 13, 229. doi: 10.3390/en13010229
Hao Y., Ba N., Ren S., Wu H. (2021). How does international technology spillover affect China's carbon emissions? A new perspective through intellectual property protection. Sustain Prod Consum 25, 577–590. doi: 10.1016/j.spc.2020.12.008
Hao Y., Guo Y., Guo Y., Wu H., Ren S. (2020). Does outward foreign direct investment (OFDI) affect the home country's environmental quality? The case of China. Struct. Chang Econ Dyn 52, 109–119. doi: 10.1016/j.strueco.2019.08.012
Hou J., Teo T. S. H., Zhou F., Lim M. K., Chen H. (2018). Does industrial green transformation successfully facilitate a decrease in carbon intensity in China? An environmental regulation perspective. J. Clean Prod 184, 1060–1071. doi: 10.1016/j.jclepro.2018.02.311
Hu J., Wang Z., Lian Y., Huang Q. (2018). Environmental regulation, foreign direct investment and green technological progress-evidence from chinese manufacturing industries. Int. J. OF Environ. Res. AND Public Health 15, 221. doi: 10.3390/ijerph15020221
Jordaan S. M., Romo-Rabago E., McLeary R., Reidy L., Nazari J., Herremans I. M. (2017). The role of energy technology innovation in reducing greenhouse gas emissions: A case study of Canada. Renewable \& Sustain. Energy Rev. 78, 1397–1409. doi: 10.1016/j.rser.2017.05.162
Kemp R., Never B. (2017). Green transition, industrial policy, and economic development. Oxf Rev. Econ Policy 33, 66–84. doi: 10.1093/oxrep/grw037
Koenker R. (2004). Quantile regression for longitudinal data. J. Multivar Anal. 91, 74–89. doi: 10.1016/j.jmva.2004.05.006
Kong Q., Peng D., Zhang R., Wong Z. (2021). Resource misallocation, production efficiency and outward foreign direct investment decisions of Chinese enterprises. Res. Int. Bus Finance 55, 101343. doi: 10.1016/j.ribaf.2020.101343
Li C. (2019). How does environmental regulation affect different approaches of technical progress?-Evidence from China's industrial sectors from 2005 to 2015. J. Clean Prod 209, 572–580. doi: 10.1016/j.jclepro.2018.10.235
Li X., Lai X., Zhang F. (2021). Research on green innovation effect of industrial agglomeration from perspective of environmental regulation: Evidence in China. J. Clean Prod 288, 125583. doi: 10.1016/j.jclepro.2020.125583
Li J., Lu G., Niu T., Zhang J. (2020). Developing an online air quality warning system based on streaming data for dynamic environmental management. J. Clean Prod 273, 122953. doi: 10.1016/j.jclepro.2020.122953
Li W., Wang J., Chen R., Xi Y., Liu S. Q., Wu F., et al. (2019). Innovation-driven industrial green development: The moderating role of regional factors. J. Clean Prod 222, 344–354. doi: 10.1016/j.jclepro.2019.03.027
Li Q., Xue Q., Truong Y., Xiong J. (2018). MNCs' industrial linkages and environmental spillovers in emerging economies: The case of China. Int. J. Prod Econ 196, 346–355. doi: 10.1016/j.ijpe.2017.12.008
Lin B., Wang C. (2023). Does industrial relocation affect regional carbon intensity? Evidence from China?s secondary industry. Energy Policy 173, 113339. doi: 10.1016/j.enpol.2022.113339}
Lin B., Xie J. (2023). Does environmental regulation promote industrial structure optimization in China? A perspective of technical and capital barriers. Environ. Impact Assess. Rev. 98, 106971. doi: 10.1016/j.eiar.2022.106971
Liu X., Chen S. (2022). Has environmental regulation facilitated the green transformation of the marine industry? Mar. Policy 144, 105238. doi: 10.1016/j.marpol.2022.105238
Liu X., Zhang Y., Li Y. (2022b). How does energy consumption and economic development affect carbon emissions? A multi-process decomposition framework. Energies (Basel) 15, 8802. doi: 10.3390/en15238802
Liu X., Zhang W., Liu X., Li H. (2022a). The impact assessment of FDI on industrial green competitiveness in China: Based on the perspective of FDI heterogeneity. Environ. Impact Assess. Rev. 93, 106720. doi: 10.1016/j.eiar.2021.106720
Mahadevan R., Sun Y. (2020). Effects of foreign direct investment on carbon emissions: Evidence from China and its Belt and Road countries. J. Environ. Manage 276, 111321. doi: 10.1016/j.jenvman.2020.111321
Mao W., Wang W., Sun H. (2019). Driving patterns of industrial green transformation: A multiple regions case learning from China. Sci. Total Environ. 697, 134134. doi: 10.1016/j.scitotenv.2019.134134
Mensah C. N., Long X., Dauda L., Boamah K. B., Salman M., Appiah-Twum F., et al. (2019). Technological innovation and green growth in the Organization for Economic Cooperation and Development economies. J. Clean Prod 240, 118204. doi: 10.1016/j.jclepro.2019.118204
OECD (2005). Climate change, economic instruments and income distribution (Paris: Organisation for Economic Co-operation and Development).
Oh D. (2010). A global Malmquist-Luenberger productivity index. J. Product Anal. 34, 183–197. doi: 10.1007/s11123-010-0178-y
Pan X., Li M., Wang M., Chu J., Bo H. (2020). The effects of outward foreign direct investment and reverse technology spillover on China's carbon productivity. Energy Policy 145, 111730. doi: 10.1016/j.enpol.2020.111730
Peng D., Ji Y., Kong Q. (2023). OFDI and firms? sustainable productive capacity: Evidence from Chinese industrial firms. Int. Rev. OF ECONOMICS \& FINANCE 83, 641–652. doi: 10.1016/j.iref.2022.10.010
Piperopoulos P., Wu J., Wang C. (2018). Outward FDI, location choices and innovation performance of emerging market enterprises. Res. Policy 47, 232–240. doi: 10.1016/j.respol.2017.11.001
Powell D. (2022). Quantile regression with nonadditive fixed effects. Empir Econ 63, 2675–2691. doi: 10.1007/s00181-022-02216-6
Qing L., Chun D., Dagestani A. A., Li P. (2022). Does proactive green technology innovation improve financial performance? Evidence from listed companies with semiconductor concepts stock in China. Sustainability 14, 4600. doi: 10.3390/su14084600
Qiu S., Wang Z., Geng S. (2021). How do environmental regulation and foreign investment behavior affect green productivity growth in the industrial sector? An empirical test based on Chinese provincial panel data. J. Environ. Manage 287, 112282. doi: 10.1016/j.jenvman.2021.112282
Qu C., Shao J., Cheng Z. (2020). Can embedding in global value chain drive green growth in China's manufacturing industry? J. Clean Prod 268, 121962. doi: 10.1016/j.jclepro.2020.121962
Ran Q., Yang X., Yan H., Xu Y., Cao J. (2023). Natural resource consumption and industrial green transformation: Does the digital economy matter? Resour Policy 81, 103396. doi: 10.1016/J.RESOURPOL.2023.103396
Razzaq A., Xiao M., Zhou Y., Anwar M., Liu H., Luo F. (2022). Towards sustainable water use: factors influencing farmers’ participation in the informal groundwater markets in Pakistan. Front. Environ. Sci. 10, 944156. doi: 10.3389/fenvs.2022.944156
Ren S., Hao Y., Wu H. (2022a). The role of outward foreign direct investment (OFDI) on green total factor energy efficiency: Does institutional quality matter? Evidence from China. Resour Policy 76, 102587. doi: 10.1016/j.resourpol.2022.102587
Ren S., Hao Y., Wu H. (2022b). How does green investment affect environmental pollution? Evidence from China. Environ. Resour. Econ. 81, 25–51. doi: 10.1007/s10640-021-00615-4
Ren S., Yuan B., Ma X., Chen X. (2014). International trade, FDI (foreign direct investment) and embodied CO2 emissions: A case study of Chinas industrial sectors. China Econ Rev. 28, 123–134. doi: 10.1016/j.chieco.2014.01.003
Shao S., Yang Z., Yang L., Ma S. (2019). Can China's energy intensity constraint policy promote total factor energy efficiency? Evidence from the industrial sector. Energy J. 40, 101–127. doi: 10.5547/01956574.40.4.ssha
Sun H., Zhang Z., Liu Z. (2022). Regional differences and threshold effect of clean technology innovation on industrial green total factor productivity. Front. Environ. Sci. 10, 985591. doi: 10.3389/fenvs.2022.985591
Tian C., Li X., Xiao L., Zhu B. (2022). Exploring the impact of green credit policy on green transformation of heavy polluting industries. J. Clean Prod 335, 130257. doi: 10.1016/j.jclepro.2021.130257
Wang K., Pang S., Ding L., Miao Z. (2020a). Combining the biennial Malmquist-Luenberger index and panel quantile regression to analyze the green total factor productivity of the industrial sector in China. Sci. Total Environ. 739, 140280. doi: 10.1016/j.scitotenv.2020.140280
Wang Y., Sun X., Wang B., Liu X. (2020b). Energy saving, GHG abatement and industrial growth in OECD countries: A green productivity approach. Energy (Oxf) 194, 116833. doi: 10.1016/j.energy.2019.116833
Wang C., Wang L. (2023). Green credit and industrial green total factor productivity: The impact mechanism and threshold effect tests. J. Environ. Manage 331, 117266. doi: 10.1016/j.jenvman.2023.117266
Wei W., Li P., Wang H., Song M. (2018). Quantifying the effects of air pollution control policies: A case of Shanxi province in China. Atmos pollut. Res. 9, 429–438. doi: 10.1016/j.apr.2017.11.010
Wu J., Xia Q., Li Z. (2022). Green innovation and enterprise green total factor productivity at a micro level: A perspective of technical distance. J. Clean Prod 344, 131070. doi: 10.1016/j.jclepro.2022.131070
Wu L., Zhang Z. (2020). Impact and threshold effect of Internet technology upgrade on forestry green total factor productivity: Evidence from China. J. Clean Prod 271, 122657. doi: 10.1016/j.jclepro.2020.122657
Xu H., Zhao G., Xie R., Zhu K. (2020). A trade-related CO2 emissions and its composition: Evidence from China. J. Environ. Manage 270, 110893. doi: 10.1016/j.jenvman.2020.110893
Yan Z., Zou B., Du K., Li K. (2020). Do renewable energy technology innovations promote China's green productivity growth? Fresh evidence from partially linear functional-coefficient models. Energy Econ 90, 104842. doi: 10.1016/j.eneco.2020.104842
Yang Z., Fan M., Shao S., Yang L. (2017). Does carbon intensity constraint policy improve industrial green production performance in China? A quasi-DID analysis. Energy Econ 68, 271–282. doi: 10.1016/j.eneco.2017.10.009
Yang R., Hu Z., Hu S. (2023). The failure of collaborative agglomeration: From the perspective of industrial pollution emission. J. Clean Prod 387, 135952. doi: 10.1016/j.jclepro.2023.135952
Yao Y., Jiao J., Han X., Wang C. (2019). Can constraint targets facilitate industrial green production performance in China? Energy-saving target vs emission-reduction target. J. Clean Prod 209, 862–875. doi: 10.1016/j.jclepro.2018.10.274
Yu Y., Yamaguchi K., Kittner N. (2022). How do imports and exports affect green productivity? New evidence from partially linear functional-coefficient models. J. Environ. Manage 308, 114422. doi: 10.1016/j.jenvman.2021.114422
Yu S., Zheng S., Zhang X., Gong C., Cheng J. (2018). Realizing China's goals on energy saving and pollution reduction: Industrial structure multi-objective optimization approach. Energy Policy 122, 300–312. doi: 10.1016/j.enpol.2018.07.034
Yuan H., Feng Y., Lee C., Cen Y. (2020a). How does manufacturing agglomeration affect green economic efficiency? Energy Econ 92, 104944. doi: 10.1016/j.eneco.2020.104944
Yuan B., Xiang Q. (2018). Environmental regulation, industrial innovation and green development of Chinese manufacturing: Based on an extended CDM model. J. Clean Prod 176, 895–908. doi: 10.1016/j.jclepro.2017.12.034
Yuan Q., Yang D., Yang F., Luken R., Saieed A., Wang K. (2020b). Green industry development in China: An index based assessment from perspectives of both current performance and historical effort. J. Clean Prod 250, 119457. doi: 10.1016/j.jclepro.2019.119457
Zeng M., Zheng L., Huang Z., Cheng X., Zeng H. (2023). Does vertical supervision promote regional green transformation? Evidence from Central Environmental Protection Inspection. J. Environ. Manage 326, 116681. doi: 10.1016/j.jenvman.2022.116681
Zhai X., An Y. (2020). Analyzing influencing factors of green transformation in China's manufacturing industry under environmental regulation: A structural equation model. J. Clean Prod 251, 119760. doi: 10.1016/j.jclepro.2019.119760
Zhang D. (2022). Are firms motivated to greenwash by financial constraints? Evidence from global firms' data. J. Int. Fin. Manag. Acc. 33, 459–479. doi: 10.1111/jifm.12153
Zhang L., Ma X., Ock Y. S., Qing L. (2022). Research on regional differences and influencing factors of chinese industrial green technology innovation efficiency based on dagum gini coefficient decomposition. Land 11, 122. doi: 10.3390/land11010122
Zhang Y., Song Y., Zou H. (2020). Transformation of pollution control and green development: Evidence from China's chemical industry. J. Environ. Manage 275, 111246. doi: 10.1016/j.jenvman.2020.111246
Keywords: industrial green transformation, dynamic evolution, regional differences, influencing factors, China
Citation: Liu C, Xu J and Zhao J (2023) Regional differences and evolution trends of China’s industrial green transformation. Front. Ecol. Evol. 11:1203075. doi: 10.3389/fevo.2023.1203075
Received: 10 April 2023; Accepted: 04 September 2023;
Published: 22 September 2023.
Edited by:
Xander Wang, University of Prince Edward Island, CanadaReviewed by:
Amar Razzaq, Huanggang Normal University, ChinaCopyright © 2023 Liu, Xu and Zhao. This is an open-access article distributed under the terms of the Creative Commons Attribution License (CC BY). The use, distribution or reproduction in other forums is permitted, provided the original author(s) and the copyright owner(s) are credited and that the original publication in this journal is cited, in accordance with accepted academic practice. No use, distribution or reproduction is permitted which does not comply with these terms.
*Correspondence: Jun Xu, amd4eWp1bkAxNjMuY29t; Jun Zhao, amd4eXpqMTcwN0AxNjMuY29t
Disclaimer: All claims expressed in this article are solely those of the authors and do not necessarily represent those of their affiliated organizations, or those of the publisher, the editors and the reviewers. Any product that may be evaluated in this article or claim that may be made by its manufacturer is not guaranteed or endorsed by the publisher.
Research integrity at Frontiers
Learn more about the work of our research integrity team to safeguard the quality of each article we publish.