- 1College of Landscape and Architecture, Zhejiang A&F University, Hangzhou, China
- 2Institute of Mountain Hazards and Environment, Chinese Academy of Sciences, Chengdu, China
Research on the evolution and driving forces of landscape patterns can provide important support for ecological governance decision-making. However, the heterogeneity of landscape patterns at the microscale (grain size and extent) and the enforceability of the zoning scale at the macroscale deserve more attention. The optimal grain size (30 ×30 m) and the extent (500 m) for landscape pattern research were obtained by analyzing the fluctuation of landscape metrics and semivariogram models in this study. The research area was divided into environmental functional regions (EFRs), which were defined according to the main ecological functions and protection objectives of each region. The analysis results of land use and land cover changes (LUCCs) showed that land use transfer in the past 20 years occurred mainly between woodland and cultivated land at the county scale, but this was not always the case in EFRs. The results of the landscape pattern analysis showed that landscape fragmentation, aggregation, and heterogeneity increased at the county scale during 1999–2020. Moreover, except within agricultural environmental protection areas (AEP) and living environment guaranteed areas (LEG), the degree and the speed of landscape damage decreased by 2020, and the turning point occurred in 2006–2013. The analysis results of geographical detectors showed that the digital elevation mode (DEM) and GDP were the main driving factors in most regions. At the county scale, the average explanatory power of the selected factors increased by 13.27% and 16.16% in 2006–2013 and 2013–2020, respectively. Furthermore, the study area was divided into three categories according to the intensity of human disturbance. The areas with high human disturbance need to focus on increasing land-use intensification and strengthening the development in low-slope hill regions. The areas of moderate human disturbance need to focus on improving the connectivity of ecological patches and optimizing industrial structures. Attention should be given to the monitoring of natural drivers and policy support for ecological governance in low human disturbance areas. The methods and findings in this study can provide a reference for decision-makers to formulate land-use policies, especially for integration into relevant urban planning, such as the spatial planning of national land that is being widely implemented in China.
Introduction
Land provides necessary food and energy for organisms, and its governance plays a key role in ecological protection and improvement of the living environment (Chi et al., 2019; Dadashpoor et al., 2019). Landscape pattern evolution caused by land use and land cover changes (LUCCs) is one of the most important factors affecting human wellbeing and ecosystem services worldwide (Nahuelhual et al., 2014; Wang et al., 2021; Hebin et al., 2022). Therefore, revealing the law of landscape pattern evolution has been the focus of research on global environmental change (Sterling et al., 2013). In recent decades, LUCCs caused by rapid urbanization and extensive construction modes have placed great pressure on regional sustainable development (Li et al., 2010). Under such pressure, the scale, speed, and degree of landscape pattern changes are also occurring at unprecedented levels (Gao et al., 2016; Feng et al., 2018). A quantitative study of the evolution of landscape patterns and their driving forces is considered to be a necessary process to grasp the consequences of LUCCs (Fan and Ding, 2016; Ma et al., 2019).
Research on the evolution of landscape patterns increasingly emphasizes the importance of spatiotemporal scales (Quintero-Gallego et al., 2018). In recent years, the scale of research has mainly focused on different administrative scales such as global, national, provincial, county, and township scales (Amuti and Luo, 2014; Luo et al., 2020), while several studies elected to use landscape types such as watersheds, wetlands, agricultural areas, and grasslands as the research boundary (Enaruvbe and Atafo, 2019; Xu et al., 2022). In addition, the relevant quantitative methods of research gradually diversified. Landscape metrics, spatial correlation, land use prediction models, and the use of land suitability evaluation models have been widely studied (Frondoni et al., 2011; Kienast et al., 2015; Peng et al., 2017). In terms of research content, the analysis of diversity, vulnerability, and structure of landscape patterns has been the focus (Herold et al., 2002; Zhang M. et al., 2020; Liu Y. et al., 2021), and some studies analyzed the causes and future changes in landscape pattern evolution through natural and socioeconomic factors such as terrain, vegetation, population, and industry (Abadie et al., 2018; Hoyos et al., 2018; Jiao et al., 2019; Petersen et al., 2021). Recently, the scale effect of landscape patterns and economic development as driving factors have attracted increasing attention (Fu et al., 2011; Chi et al., 2019; Zhang Q. et al., 2020). Zhou et al. (2020) studied the characteristics and driving forces of LUCCs and landscape pattern evolution in rural China from 1995 to 2015 at different administrative scales. Zhang et al. (2021a) quantified the homogenization and heterogeneity of landscape patterns during evolution and analyzed the driving forces of socioeconomic factors.
However, there are still some aspects neglected in previous studies that are worthy of scholarly attention. First, although previous studies covered an abundance of different scales, most of them used the original resolution of the land use data to analyze landscape patterns directly and considered the whole research area as the analysis extent, thus ignoring granularity and extent effects. Grain size corresponds to the maximum resolution or pixel size of the research data (Zhang Q. et al., 2020), which refers to the resolution of land use data in this study. Extent is a smaller scale used to divide the whole research scale evenly to spatially display landscape features (Miguet et al., 2016; Moraga et al., 2019). In this study, the optimal extent can be used to spatialize the landscape pattern and better reflect spatial heterogeneity (Asgarian et al., 2015). Changes in grain size and extent can substantially affect the results of landscape pattern analysis (Luo et al., 2020). Previous studies that focused on the effect of granularity and extent mostly considered only one of them (Feng et al., 2018; Zhang Q. et al., 2020; Li et al., 2021); although some studies covered both (Zhang et al., 2014; Meng-wen et al., 2020), few used both in the study of landscape pattern evolution. Second, quantitative analyses of landscape pattern changes at the overall research scale alone cannot strongly support spatial planning and ecological governance. The data must be used in tandem with ecological spatial planning and landscape pattern analyses to increase the operability of the research.
Counties are middle-scale landscapes in China and are comparable to shires in England and counties in America, and they form the most basic administrative scale for formulating and implementing land policy (Fan and Ding, 2016). Anji County, located in Zhejiang province, is a typical mountainous county in China. The area of mountainous counties covers 74.7% of the land surface in China and is home to half of its people (Ying and Fang, 2017; Du et al., 2020). In recent years, the urbanization rate of mountainous areas in mountain regions has gradually caught up with or exceeded that of plain areas (Jia et al., 2020). In addition, the geographic environment of mountainous areas is relatively fragile, and there are great challenges in maintaining their ecological functions while the population and urbanization rates are increasing rapidly. Therefore, there is a great potential and urgent need to study the evolution and driving mechanisms of landscape patterns in mountain regions at the county scale. Owing to the limitation of mountain space and rapid urbanization, Anji County urgently needs reasonable land and ecological planning to ensure sustainable development. However, it is important to conduct research at a scale conducive to operational strategies (Saarikoski et al., 2018). Currently, some studies have combined landscape patterns with urban land use planning (Liu et al., 2011; Abrantes et al., 2016), which is usually divided based on administrative boundaries and thus may damage natural attributes (Simonit and Perrings, 2013). The environmental functional regions (EFRs) may be a suitable choice for dividing the research area. The EFRs were defined to clarify the regional ecological functions and protection objectives, and their basis of zoning includes natural and administrative attributes simultaneously (Xie et al., 2012; Liu Y. et al., 2018). Therefore, using EFRs as the spatial unit in research not only will quantify the characteristics of landscape patterns but also will improve the operability of the ecological governance strategies given in this study.
Using Anji County as the subject, we analyzed the evolution and driving forces of landscape patterns at the county and EFR scales. The results can provide a reference for ecological management in mountainous areas or areas with high urbanization rates. The highlights and objectives of this study are as follows: (1) the transfer matrix and change trajectory of land use were analyzed simultaneously to better understand the process of LUCCs; (2) both microscale (grain size and extent) and macroscale (zoning of the study area) effects were considered and analyzed at multiple temporal scales; and (3) the environmental functional regions were used to demarcate the research area, classify it according to the intensity of human activities, and then give corresponding land management strategies. Together, when combined with urban planning, these findings will provide substantial guidance.
Materials and Methods
Study Area
Anji County is located in Huzhou city in northern Zhejiang Province, with an east longitude of 119°14′−119°53′, a north latitude of 30°23′−30°53′, and a total area of 1,885.7 km2. Anji County is a developed area in the Yangtze River Delta, with a high urbanization rate (61% in 2020 and over 72% is expected in 2025) and a GDP of 4.871 billion yuan in 2020, which ranks among the top 3% of counties in China. In terms of geographic attributes, Anji County is a typical mountainous area (mountainous and hilly landforms account for 74.6% of the total area), with high landscape heterogeneity and includes diversified landscapes. In the stage of rapid urbanization, how to balance land construction and ecological protection is a key issue because of the limitation of the mountainous area in Anji County. Therefore, Anji County planned environmental functional regions in 2018 to set ecological protection objectives and environmental access thresholds for development projects in different regions (Figure 1).
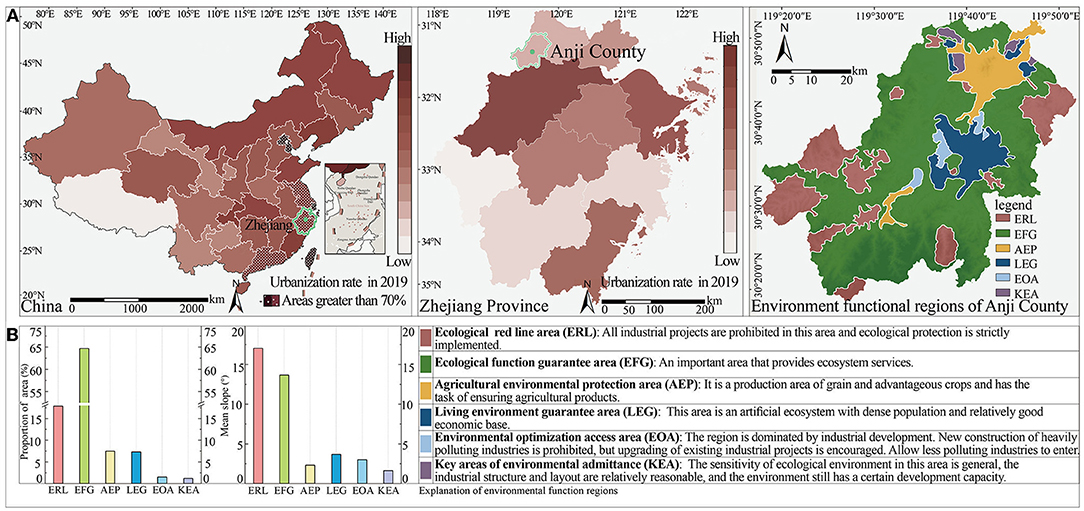
Figure 1. Location and basic information of the study area. (A) Is the location and environmental function regions of Anji County and (B) is the basic information and legend explanation of each environmental functional region.
Data Sources
Several datasets were used to support this study. (1) Landsat 7 and 8 remote sensing images including panchromatic bands with a resolution of 15 × 15 m, in 1999, 2006, 2013, and 2020 were obtained from the USGS (https://earthexplorer.usgs.gov). These remote sensing images were used to interpret land use in the study area. (2) Digital elevation models (DEM) selected as one of the driving factors were derived from Geospatial Data Clouds with a resolution of 30 × 30 m (https://www.gscloud.cn). (3) GDP and population data with a resolution of 1 × 1 km selected as driving factors (human interference factors) were obtained from the National Center for Earth Systems Science Data (http://www.geodata.cn) and the Data Center for Resources and Environmental Sciences (http://www.resdc.cn). (4) Night lighting data selected as a driving factor (human interference factor) with a resolution of 1 × 1 km were obtained from the NOAA-funded EOG Network (https://eogdata.mines.edu). (5) Temperature and precipitation selected as driving factors (natural factors) were obtained from the CRU dataset (https://sites.uea.ac.uk/cru/), and the delta downscaling method was adopted to improve the data resolution to 30 × 30 m (Hijmans et al., 2005). (6) Last, administrative boundary and environmental functional region data were obtained from the Natural Resources and Planning Bureau of Anji County.
Research at the county scale requires higher precision land use data. Therefore, when interpreting land use, we used the Gram–Schmiat Spectral Sharpening tool in ENVI software to fuse the eighth band of remote sensing image into other bands and to improve the resolution of remote sensing images to 15 × 15 m. Then, the land use was divided into six types, woodland, cultivated land, construction land, waters, grassland, and unused land by combining supervised classification and artificial visual interpretation (Figure 2). In addition, we used high-resolution Google maps to verify the accuracy of the classification results. The results showed that the kappa coefficients are all >80%, which meets the requirements of the research (Xu et al., 2021). The other data were unified to 15 × 15 m by the resampling method.
Methods and Procedures
The research framework was divided into four parts. First, land use data were obtained by interpreting remote sensing images. Then, the land use transfer and changes trajectory at each scale were analyzed. Third, optimal granularity and extent of the research were obtained through an analysis of landscape metrics and the semivariogram model. Fourth, the evolution and driving forces of the landscape pattern were analyzed at the scale of the county and the environmental functional regions, and the corresponding strategies for ecological governance and land use planning were given according to the research results. The specific methods are discussed in the following four sections.
Transfer Matrix and Transfer Trajectory of Land Use
We used the Sandkey graph function of Origin 2022 software to visualize the land use transfer matrix data that can reveal the direction and quantity of land use transfer (Zhang et al., 2021a). However, using only the transfer matrix may ignore the process of LUCCs (Wuyun et al., 2021). Therefore, this study adds the land use change trajectory (LUCT) analysis to provide a complete process of LUCCs. The changing process of LUCCs was represented by codes in LUCT, such as 1,112, 2,223, and 3,224. Each number represents the land use type of the grid in different years. The detailed calculation methods used are presented in Supplementary Section 1.1.
Grain Size Resampling and Evaluation
To select the optimal grain size for landscape pattern analysis, 15 sets of data were resampled at intervals of 10 m based on the land use grid of the study region in 1999. Starting with a 10 × 10 m resolution, the maximum resolution was 150 × 150 m. Then, a stable interval was selected according to the fluctuation of metric values. The optimum grain sizes were determined according to the landscape area loss evaluation index of different grain sizes in this interval. The selection of landscape pattern metrics and the process of the calculation are listed in Supplementary Table 1 and Supplementary Section 1.2.
Moving Window Method and Semivariogram Model
The moving window method in Fragstats 4.3 can be used to analyze the landscape pattern metrics at different extents by moving grid by grid, starting from the upper left corner of the study area and assigning the values to the central grid (Chefaoui, 2014; Li et al., 2021). In addition, the semivariogram model (SVM) in geostatistics was used to determine the optimal size of the moving window. It can analyze the point-to-point relationship between landscape metrics and spatial variables and is widely used in geography and landscape ecology (Trangmar et al., 1986; Li et al., 2021). The formula is as follows:
where λ(h) represents the semivariance of the value of variable X at positions i and i+h and N(h) represents the number of sampling points when the space distance is H. There are two important variables in the SVM. One is the nugget (Co), which represents the spatial heterogeneity caused by random samples, and the other is Still (CO + C), which is the value at which λ(h) reaches a relatively stable constant from the initial Co value with increasing sampling point spacing. The ratio of these two values, RNS (Co/Co+C), was used to measure the degree of spatial heterogeneity under the interference of random factors and can be used as the basis to determine the size of the moving window (Qiao and Li, 2006). The calculations were performed by the use of the Geostatistical Analyst tool in ArcGIS 10.7.
Geographical Detector
As a tool to explore driving forces, a geographical detector is based on the assumption that, in geographical space, if an independent variable has an impact on the dependent variable, then the spatial distribution of the two variables should be similar (Wang et al., 2010).
According to the needs of this study, the factor detector and the interaction detector were used to describe the explanatory power of driving factors and the type of interaction between factors. Details of the driving factors and types of interaction between factors are given in Supplementary Tables 2, 3.
where L is the classification of driving factors, and Ni and N are the number of units in the whole region of class i and the whole region, respectively. σ2 and are the variances of class i and the whole region, respectively. The value of q is between 0 and 1, and a larger value indicates a stronger driving force.
Results
Spatiotemporal Changes in Land Use
The LUCCs during 1999–2020 at the county scale are shown in Figure 3A. The results showed that the land use transfer was mainly between woodland and cultivated land during 1999–2020. The expansion of construction land was mainly from cultivated land. This is related to the “grain for green” plan implemented by the Anji County Government, which advocates converting cultivated land with a slope of more than 25° into woodland (Feng et al., 2005). In addition, LUCCs were significantly different at each period on the county scale (Figure 3B). The most obvious result was that the average annual growth rate of construction land decreased from 11.24% in 1999–2013 to 2.7% in 2013–2020 (Figure 3C). This was mainly because development land was gradually saturated after a long period of rapid expansion under the limitation of mountain space. In 2013, 73.75% of the land with an elevation below 50 m was occupied by cultivated land and construction land. Land with a slope of <25° is one of the basic conditions for allowing development (Liu Q. H. et al., 2018), and such land was occupied by 45.23% of cultivated land and construction land in 2013. Therefore, in the context of cultivated land and ecological protection policies, the available space has been unable to meet the needs of long-term rapid urban expansion. Furthermore, the decrease in the growth rate of construction land is also related to the Beautiful Rural Construction and Rural Revitalization strategy implemented by Anji County in recent years (Zhang et al., 2021b). These policies shifted the focus of development from urban areas to rural areas. Rural development mostly occurs through the transformation of construction land and the transfer of property rights, rather than greatly increasing the scale of construction land (Gao et al., 2020).
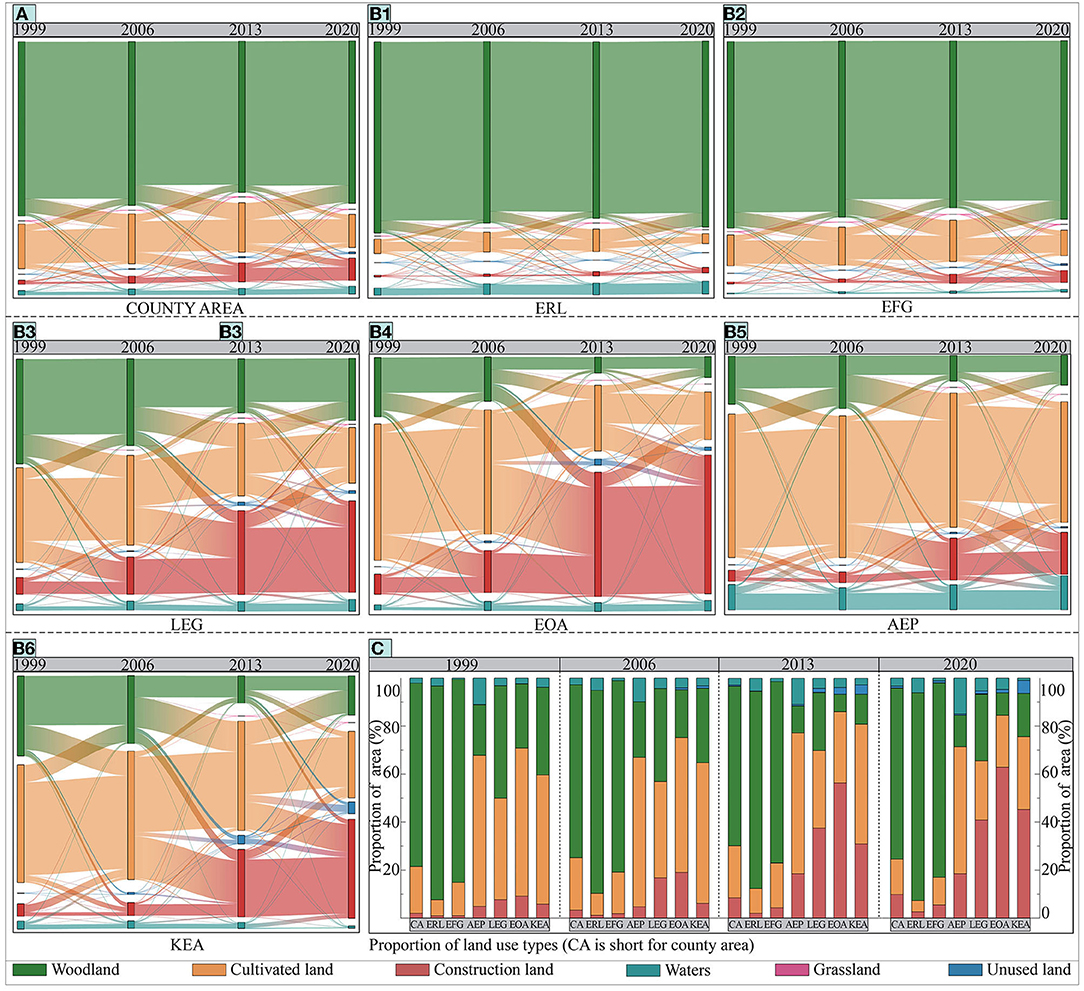
Figure 3. Land use transfer at county scale (A) and in each environmental function region (B) and proportion of land use types at the county scale and each environmental function region (C). Those below 1% in (C) are not counted.
The analysis of land use transfer in EFRs is seen in Figure 3B. The results show that only the ERL and EFG were essentially consistent with the characteristics at the county scale. This was probably because these two areas are relatively large, covering most of the county (82.55%). Conversion of cultivated land to construction land was the most dominant type in LEG, EOA, AEP, and KEA, which indicates that human development was mainly concentrated in these areas. This feature is also essentially consistent with the definition of each environmental functional region. In addition, land use transfer was also dependent on the temporal scale in EFRs. For example, the main type of LUCC in ERL and EFG was cultivated land to woodland in 2013–2020, which was opposite to the previous two periods. This may be related to the Woodland Protection and Utilization Plan promulgated by Anji County in 2008. This plan's emphasis on increasing the scale of woodland has led to a larger scope for the implementation of the “grain for green” plan, especially in areas with better ecological quality, as these areas are more effective in protecting woodland.
To study the process of LUCCs in more detail, the trajectory of land use change was analyzed in EFRs. The top 10 track codes of each region were selected for analysis (Table 1). In ERL and EFG, the main land change trajectory was mainly about the conversion between woodland and cultivated land, among which 87.85% of the cultivated land converted from woodland was eventually restored to woodland. As the increasing population required more food, the cultivated land converted from woodland was still partly retained. The trajectory codes of LEG, EOA, and KEA showed an increase in construction land, derived mainly from cultivated land, accounting for 75.56, 80.30, and 76.67% of the three regions, respectively, with most land transferred during 2006–2013. The government of Anji County increased the proportion of prohibited construction areas from 19 to 52% of the county area in 2015. The proportion of cultivated land occupied by planned construction decreased by 21.54% (Group, 2017). These measures have effectively alleviated the serious occupation of cultivated land.
Temporal and Spatial Changes of Landscape Patterns
The calculation results of landscape metrics under different grain sizes showed that the landscape metrics except for ED (edge density) have obvious inflection points (Figure 4A). According to the inflection point, the grid-scale domain of the study area can be divided into three intervals: 10–60 m (number of patches, NP; patch density, PD; landscape division, DIVISION; Shannon's diversity, SHDI; and Shannon's evenness, SHEI), 60–90 m (NP), and 90–140 m (LPI, DIVISION, SHDI, and SHEI). In these scale domains, the value of landscape metrics was relatively stable, which can effectively reflect the landscape characteristics of the research area. Considering the loss of information, the optimal grain size should be selected on the “first scale” (Wu and Hobbs, 2002; Chen et al., 2016), namely, 10–60 m in this study. Within this domain, 30 m had the least information loss (Figure 4B); therefore, it was taken as the most suitable grain size for the analysis of landscape patterns. Then, according to the studies by Hu et al. (2021) and Li et al. (2021), some landscape metrics were selected and calculated using a semivariogram. The results showed that the RNS values were in a stable state with an extent between 500 and 700 m (Figure 4C). Considering that more spatial information would be lost in the large size, 500 m was selected as the analysis extent in this study.
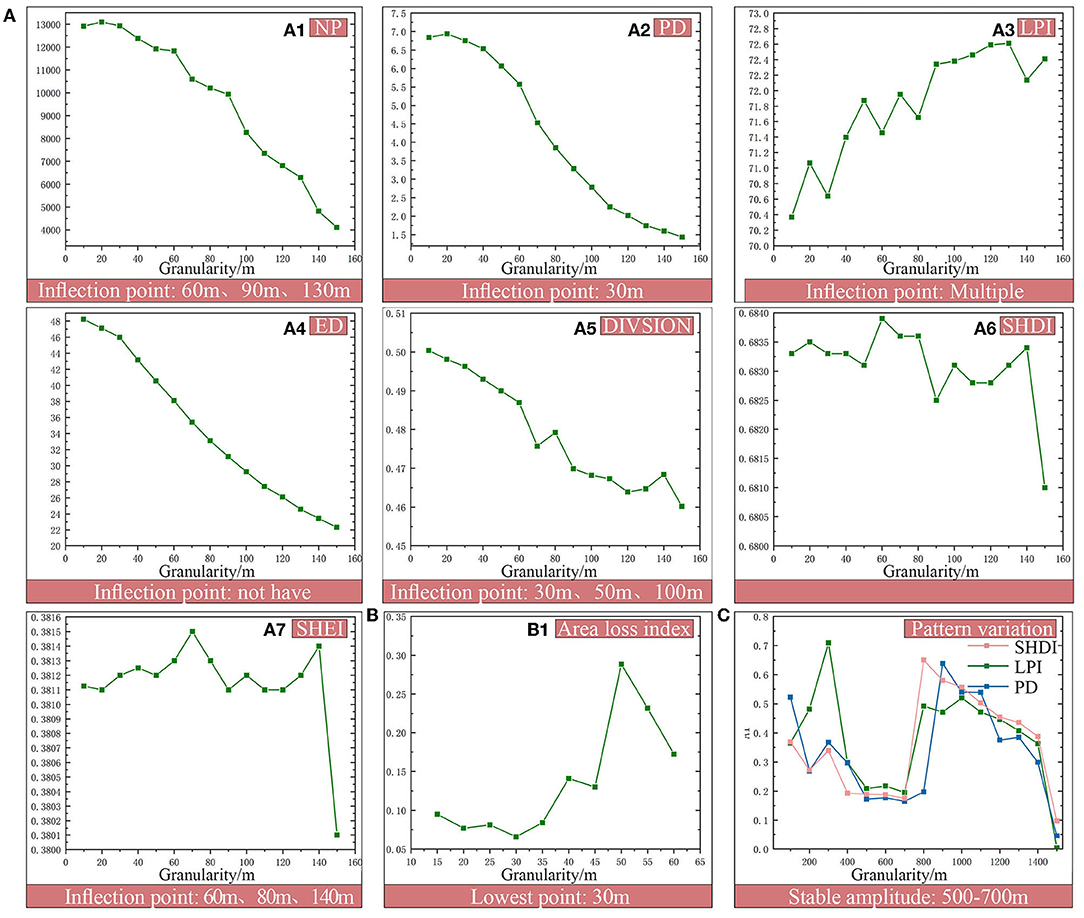
Figure 4. Landscape pattern index (A) and area loss index (B) spatial variation eigenvalues and trends of landscape pattern index under different extent (C).
At the county scale, the landscape division index (DIVISION) and the contagion index (CONTAG), the largest patch index (LPI) and the number of patches (NP), and Shannon's diversity index (SHDI) and Shannon's evenness index (SHEI) were selected to evaluate the fragmentation, aggregation, and diversity of the study area, respectively (Zhang et al., 2021a; Zhu et al., 2021).
The landscape pattern at the county scale seen in Figure 5 indicates that (1) the central urban area has a high degree of aggregation, (2) the high-aggregation areas in the outer suburbs are relatively scattered, and (3) the areas with severe landscape fragmentation are mainly in the northern agricultural areas and central towns. Compared with that in 1999, the landscape pattern of the central urban was more concentrated in 2020, while the other areas were more fragmented. The data of DIVISION, CONTAG, SHDI, and SHEI showed the highest point in 2013, indicating that the competition between urban expansion and ecological restoration reached a certain threshold in 2013. It also shows that the early implementation of the ecological protection policies had a certain time delay effect in optimizing the ecological pattern. In addition, the increase in DIVSONG indicated a gradual increase in landscape segmentation, but the increase in CONTAG indicated that artificial land was more concentrated. This weakens the transitional role of the buffer zone between artificial and ecological lands and further threatens biological habitats. The changes in LPI and NP showed that the landscape was continuously fragmented at the county scale. This indicates that it is difficult to reverse the segmentation of landscape patches caused by urbanization in the whole region, although it may be improved locally.
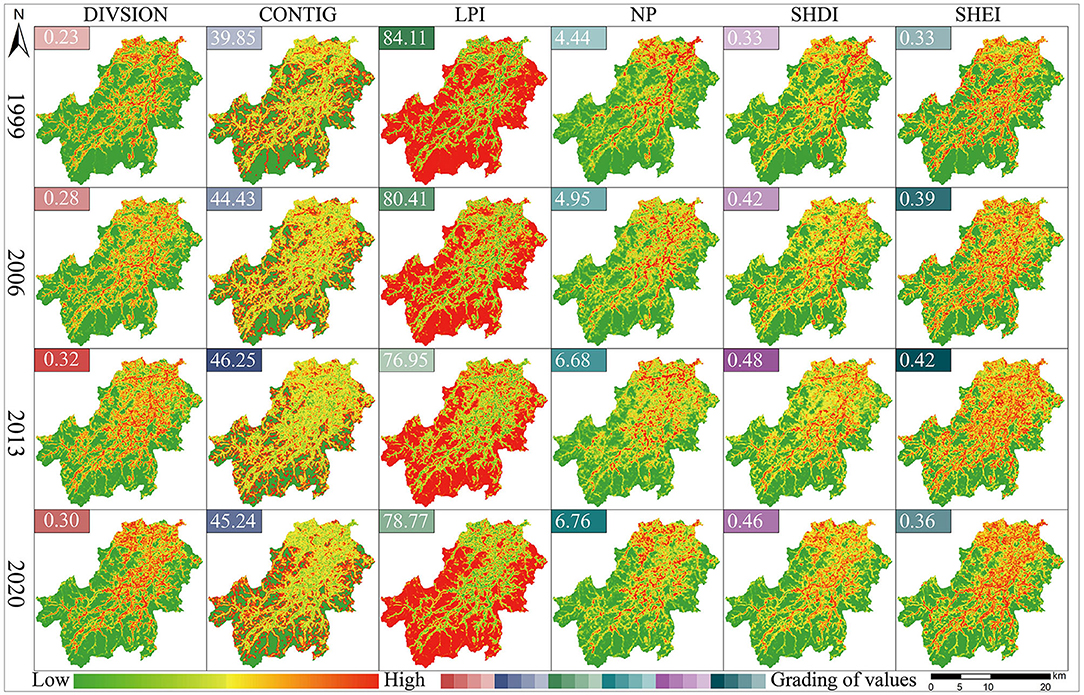
Figure 5. Spatial distributions of landscape metrics for the periods 1999–2020. The value in the figure is the average value of each landscape metric.
The same landscape metrics were also analyzed in EFGs. As seen in Figure 6, DIVISION and CONTAG mainly increased in most of the EFGs during 1999–2006, among which the DIVISION value in ERL from 1999 to 2006 increased the most, reaching 33.7%. During 2013–2020, these two metrics mainly declined, but the CONTAG of KEA and DIVISION of AEP and LEG continued to increase. This was mainly because urban expansion mainly occurred in these areas and was difficult to avoid. The changes in LPI and NP showed that the problem of landscape fragmentation in some areas improved in 2013–2020, especially the value of LPI, which increased in most areas. This indicates that the continuous fragmentation of the landscape at the county scale is more related to the increase in NP than the decrease in LPI. Both the SHDI and the SHEI increased in each region in the early stage, indicating that the area proportion of different patch types in the landscape gradually became similar and landscape diversity increased. Furthermore, the SHDI and SHEI remained high in the AEP and LEG during 2013–2020. This may be due to the current ecological governance measures as an early intervention that has not yet reached the threshold for improved fragmentation.
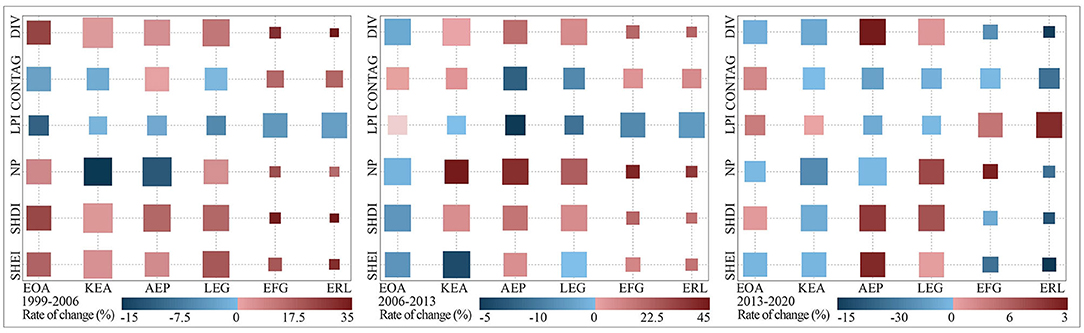
Figure 6. Heat maps of landscape metrics changes in each environment functional region from 1999 to 2006, 2006 to 2013, and 2013 to 2020; the square sizes in the figure are the normalized values of landscape metrics of each environmental function zone in 1999, 2006, and 2013; The color in the figure is the rate of change of landscape metrics. The value of change refers to the average of the landscape pattern metrics of each environmental functional region.
Effects of Driving Factors on the Spatiotemporal Evolution of Landscape Patterns
The geographical detector was used to quantify the explanatory power of each driving factor on the change in landscape metrics (Figure 7). More than 80% of the factors have q values <0.01; therefore, the driving factors passed the correlation test. From 1999 to 2020, the average explanatory power of each driving factor at the county scale was more than 15%, of which the DEM (39.10%) and GDP (34.40%) were the most important factors. In LEG, EFG, and ERL, DEM and GDP also have the largest explanatory power, both of which were above 30%. This indicates that both human disturbance and natural conditions have important effects on landscape pattern changes in mountainous areas. Population density and temperature were the main drivers in KEA and EOA. These two areas are located in the central urban area and the plain area in Anji County, with no obvious changes in topography and geomorphology, and are also the main land for urban expansion. Therefore, the driving forces of the natural environment were not obvious. However, owing to the urban heat island effect, the temperature and population density data were positively correlated with the intensity of urban development; therefore, the explanatory power was strong. In AEP, the explanatory power of the eight factors was low, and the highest was the population density, which was 8.06%. This was the case because the types of agricultural activities were relatively stable during the study period, and the impact of mechanized tillage on landscape patterns was less different than that of manual tillage. In addition, the landform dominated by plains in the AEP also led to the weak driving force of each factor.
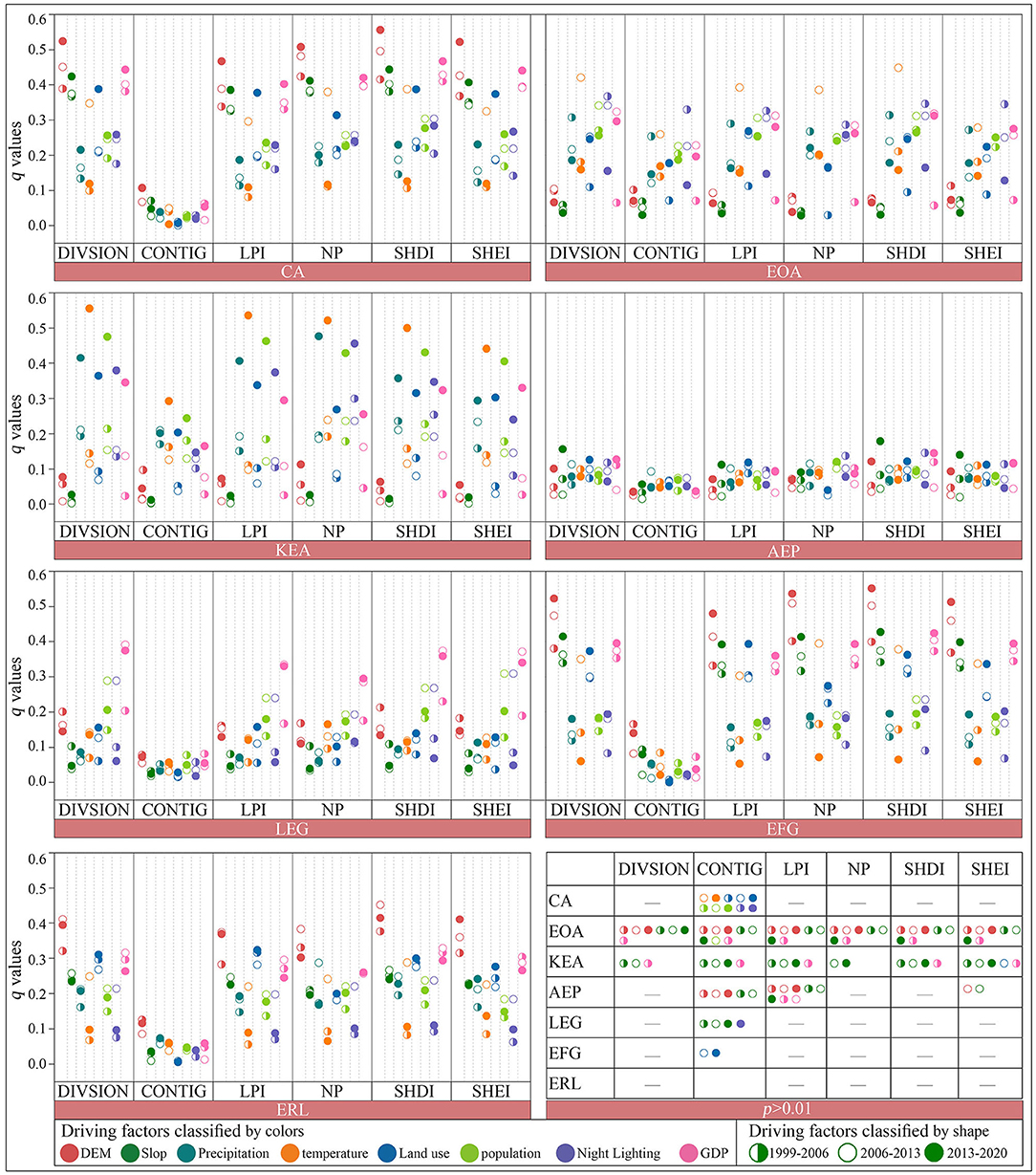
Figure 7. The q and p-values of driving factors in the county scale and environmental functional regions. q represents the determinant power and p represents the correlation coefficient.
The results also showed that the driving factors had different impacts on each landscape pattern metric. In particular, the explanatory power of CONTAG was relatively low. At the county scale, the average explanatory power of the eight driving factors to the change in CONTAG was only 3.96% (1999–2020), and the same situation also appeared in AEP, LEG, EFG, and ERL. This was mainly due to the relatively low p-values in this region for the factors we selected; overall, it passed the significance test. In addition, the smaller change in CONTAG compared with other metrics was also one of the reasons why the explanatory power of this factor was not obvious. Moreover, from the analysis of different periods, the overall explanatory power of the driving factors kept increasing. At the county scale, the average explanatory power of driving factors increased by 13.27 and 16.16% during 2006–2013 and 2013–2020, respectively. This situation was also common in most EFRs. Combined with the spatial computing method adopted by geographical detectors used to analyze this trend, it can be verified that the degree of human development is expanding. This also shows that these factors that we selected will still apply in future studies.
The strength of the interaction between the driving factors was calculated by the interaction detector at the county scale (Figure 8). Except for CONTAG, the interaction between drivers was mainly a bilinear enhancement. The results also show that there were great differences in the intensity and type of interaction among EFRs (Supplementary Section 2). Only EFG and ERL were consistent with the county scale, and the interaction between factors was mainly bilinear enhancement, while the remaining four EFRs were predominantly non-linear enhancement. However, in EOA and KEA, the interaction of population density, light intensity, and GDP was mainly a bilinear enhancement. In AEP and LEG, the interaction of driving factors was mainly a non-linear enhancement, including the CONTAG index, which was different from that at the county scale. Therefore, in the complex natural-socioeconomic system in mountainous areas, the interaction of a series of factors, such as land development, population, and natural conditions, was the main reason for the change in landscape pattern.
Discussion
The Microspatials and Macrospatial Scale Dependence of Landscape Patterns
Our study confirmed that scale dependence exists at both the macroscale and the microscale levels, which is consistent with some previous research reports (Luo et al., 2020; Xu et al., 2020). First, at the microscale, we found that the original resolution of the land use data was not the best granularity for landscape pattern analysis (Figure 4). In this study, the resolution was changed from 15 × 15 m to 30 × 30 m, and the size of the moving window was determined to be 500 m through the semivariogram mode. The overall scale of this study was the county scale; therefore, the grain size and extent were smaller than those in large-scale studies. For example, the extent determined by Li et al. (2021) in the analysis of the main river basins on the Qinghai Tibet Plateau was 9 km. The optimal scale for analysis was influenced by the overall scale, data accuracy, and regional characteristics of the research region (Ding et al., 2016). Second, at the macroscale level, we found that using EFRs as a research scale can better explain landscape pattern evolution and its driving forces than using the county scale alone (Figures 6–8 and Supplementary Figures 1–6). This suggests that improving the overall research scale to be more detailed can better guide practice, the conclusion of which is consistent with those of several previous studies. However, most studies are based on administrative boundaries, river basins, or their buffer zones as the basis of scale segmentation (Xu et al., 2020, 2022; Nie et al., 2021). Ecological governance policies at a small scale require a comprehensive consideration of many factors, such as urban planning, the natural environment, and ecological protection planning (Mallinis et al., 2014; Luo et al., 2020). Therefore, it is necessary to consider both natural and managerial attributes for policies to be implemented at an operational scale.
In addition, scale dependence has different effects on each landscape pattern metric. According to Figures 7, 8 and Supplementary Figures 2–6, we observed the different features of landscape pattern metrics at the county scale and the EFR scale. Moreover, PD, ND, and ED show a single downward trend with the change in grain size, which was different from the dynamic patterns of the other metrics (Figure 4). Most previous studies focusing on the scale effect of landscape patterns considered dividing the study area using regular shapes rather than using a more scientific basis. Although some studies combined the buffer zone and watershed (Xu et al., 2020; Zhong et al., 2022), a few studies combined regional ecology and development planning. This study not only emphasizes the scale effect of landscape patterns on the microscale but also divides the study area by using EFRs on the macro scale.
Temporal Dependence of Landscape Pattern Evolution and Its Driving Forces
For the study of landscape pattern evolution with long time series, if the analysis focuses only on the changes between the first and last years, the process of landscape evolution will be neglected. This study divided the span of research time into three stages: 1999–2006, 2006–2013, and 2013–2020. The results showed that the characteristics of landscape pattern evolution and its driving forces were different in each period. In the first two time intervals, the six landscape pattern metrics increased by 14.03 and 17.67% on average at the county scale, respectively, but decreased by 3.81% in the third time interval. Among the EFRs, except for the EOA, the explanatory power of the driving factors increased overall during 1999–2020. However, this was not the case for population density. Among the 42 q-values calculated for population density, 57.14% of the maximum value was in the second period. The dominant drivers also changed over time. For example, in LEG, GDP was not the main driving factor in the first stage but had a more significant explanatory power in the later period.
The importance of the spatial scale effect of landscape patterns to the process of analysis has been proven in previous studies (Fan et al., 2017; Xu et al., 2020). However, few studies focused on both spatial and temporal scale effects. Although some studies analyzed land use change trajectory, driving forces were not considered (Wuyun et al., 2021). Studies on temporal scale dependence mainly focused on forest research, and research results could obtain the driving factors in different periods and effectively guide the implementation of afforestation plans (Yackulic et al., 2011; Calaboni et al., 2018; Luo et al., 2020). This study focused on the temporal and spatial dependence of landscape pattern changes and their driving forces. In addition, both microspatial and macrospatial scale effects were considered.
In addition to the eight driving factors quantified by us, the policy factor was also an important driving force of landscape pattern changes in Anji County. In the first time interval (1999–2006), Anji County began to implement the grain-for-green plan in 1999, which promoted more cultivated land to woodland, and the impact of this plan continues today. The “Two Mountains Theory” proposed in 2005 had an important impact on the changes in landscape patterns in the second and third stages, which emphasized ecological governance and sustainable development. Under the guidance of this development concept, the implementation of major ecological governance and industrial structure optimization has effectively reduced the damage to the environment caused by regional construction. The achievement transformation of this development concept can be reflected in the analysis of landscape pattern metrics from 2013 to 2020 (Figures 5, 6), which also indicates that there is a certain time delay in the representation of the effect of environmental governance on landscape pattern metrics. In 2012 and 2018, Anji County took the lead in implementing the strategies of rural construction and rural revitalization in China and made a long-term plan for the county's rural industries, environmental governance, and construction. These policies are an important driving factor in past and future landscape changes. They will promote the rationality of land use structure and function in the whole region and are of great significance to the sustainable use of resources (Long et al., 2019). Although these policies are difficult to spatially grade and quantify at the county scale, they also affect landscape patterns through natural and human activities to a certain extent. For example, the main impact of the “grain for green” plan was on land with a slope >25° (Zhou et al., 2020).
Zoning Management Based on Environmental Functional Regions
Through quantitative analysis on different temporal and spatial scales, this study revealed the complex process of landscape pattern evolution and its driving factors. According to fragmentation, aggregation, and diversity, important landscape metrics were selected for analysis. In addition, the county scale was divided according to the boundaries of the EFRs, and zoning studies were conducted. We divided the EFRs into three categories according to the intensity of human activities and provided suggestions on protection, planning, and construction, as follows:
(1) AEP and LEG (high-intensity human activities): These two regions help to focus on protecting the living environment and improving land use intensification and to pilot a new model of land development. These two regions are densely populated and are important areas for the output and input of ecological services, respectively. From the analysis of landscape metrics, the fragmentation degree of AEP gradually increased; therefore, it is necessary to improve agricultural intensification, land consolidation, and production efficiency to balance ecological land use and food yield. Both patch numbers and diversity indices are increasing in LEG, indicating a growing tension between urban expansion and the compact mountain space of Anji County. The integrated development of low-slope hills piloted in many regions can effectively balance this contradiction (Peng et al., 2018). The potential area of low-slope hills for agriculture and construction development in Anji County was 6,500 hm2, accounting for 15.78% of the total amount of existing cultivated and construction land. Therefore, the implementation of this strategy not only can protect the cultivated land in plain areas but also can provide space for urban expansion.
(2) EOA and KEA (moderate-intensity human activities): These two regions coordinate the development intensity and the environmental carrying capacity. These areas still have a certain environmental carrying capacity, which can carry out appropriate high-quality industrial development or urban construction. In addition, the overall landscape ecological pattern has improved under the strategy of industrial structure optimization and adjustment in recent years. However, some measures still need to be taken to solve the problem of aggregation between landscapes. The impact of construction land aggregation on ecological functions can be reduced by enhancing connectivity between ecological patches, the complexity of patch boundaries, or supplementing stepping stones between construction land areas (Shi et al., 2017; Liu W. et al., 2021). The driving force analysis of these regions shows that both natural and artificial factors are important. Therefore, urban expansion and construction should continue to coordinate the environmental capacity and take the tertiary industry as the main new development project. In addition, unorganized development can be prevented by formulating policies on optimizing the type of industrial development and controlling the boundary between ecological protection and construction intensity.
(3) EFG and ERL (low-intensity human activity): These two regions focus on elastic protection. These areas have the lowest development intensity and the highest degree of protection. Woodland and cultivated land are dominant in these regions. Under the ecological protection policy of Anji County, the landscape pattern gradually improved overall, but the fragmentation of patches was still increasing in EFG. Since EFG accounts for 64.67% of the total area of the county and is not a no-construction zone, appropriate development activities are inevitable. However, as EFG is an important ecological functional guaranteed area, environmentally friendly projects should be selected for development and construction. In addition, owing to the relatively low intensity of human activities, natural factors were the main driving factors in these regions. Therefore, the monitoring of temperature, precipitation, and geological disasters is particularly important. In addition, owing to the large area, the main problems were financial and policy issues. Ecological protection policies usually put great economic pressure on producers. For example, the cost of forest farm protection and management is usually borne by producers rather than the government. Therefore, ecological protection should establish a more flexible financial system that can be supported by the government and private enterprises to ensure the wider implementation of ecological governance policies.
Limitations and Prospects
This study analyzed landscape pattern evolution and its driving forces at an optimal grain size and extent, coupled with multiple spatial and temporal scales. Based on this method, the microscale and macroscale effects can be considered simultaneously; therefore, the scientificity and operability of the research are significantly improved. Theoretically, this method can also be applied to other types of landscape spatial analysis, such as ecological sensitivity assessment, landscape vulnerability analysis, and dynamic evolution of ecological risks. In terms of combining with urban planning, the environmental functional regions used in this study can also be replaced by other planning, such as urban green space system planning, ecological protection area planning, and the spatial planning of national land. Moreover, our research method is also suitable for large-scale research, but it is necessary to find a more manageable scale to combine with the overall research scale.
However, our research still has some uncertainties and limitations. First, we analyzed only part of the landscape metrics in a single year to determine the optimal grain size and extent. If we analyzed more metrics in multiple years, this may cause some uncertainty. Therefore, the method to determine the optimal scale still needs further evaluation. Second, different time intervals may cause uncertainty in the analysis of LUCCs and landscape pattern changes (Wang et al., 2016). Future research can set different time intervals to find possible new features. In addition, we considered the most natural and artificial driving factors, but there may be information redundancy between these factors, which is difficult to remove with the geographic detector. Therefore, combining spatial principal component analysis (SPCA) may be a potential solution to this problem, but more factor data must be selected for spatial analysis (Dai et al., 2021). Finally, we used the resampling method to unify the resolution of different data, which may also introduce some uncertainty into the results.
Conclusion
Microscale and macroscale effects play an important role in the study of the spatiotemporal evolution of landscape patterns. Research on the microscale can increase the accuracy and reliability of the analysis, while segmentation on the macroscale can increase the implementation of policies guided by the research. In addition, evolutionary processes can be better understood by focusing on the heterogeneity of landscape patterns at the temporal scale. First, we analyzed LUCCs at multiple scales by using the transfer matrix and the transfer trajectory of land use to master their detailed process. Second, we considered grain size and extent effects at the micro-level in this study. Third, the study area was divided by using environmental functional regions (EFRs) at the macro scale. Then, the study period was divided into three time intervals to examine temporal scale effects. In addition, EFRs were divided into three categories that were based on the intensity of human activities. In addition, the corresponding land planning and ecological governance strategies were given according to the landscape pattern characteristics and future developmental needs in different types of regions. The main conclusions are as follows:
(1) Land use transfer in the past 20 years mainly occurred between woodland and cultivated land at the county scale, but this was not always the case in the EFRs. Conversion of cultivated land to construction land was the most dominant type in LEG, EOA, AEP, and KEA.
(2) The optimal grain size (30 × 30 m) and extent (500 m) for landscape pattern research were obtained by analyzing the fluctuation of landscape metrics and semivariogram models.
(3) At the county scale, in the first two time intervals (1999–2006 and 2006–2013), except for CONTAG, the landscape pattern was gradually fragmented and homogenized. In the third time interval (2013–2020), the landscape metrics showed that the ecological environment was significantly improved, except for the CONTAG metric. This shows that the environmental characterization of the CONTAG metric had a time lag. In addition, except for AEP and LEG, the degree and speed of landscape damage decreased by 2020, and the turning point was in the second period (2006–2013).
(4) According to detection by the geographical detector, elevation (DEM) and GDP were the most critical driving factors at the county scale and in LEG, EFG, and ERL. Population density and temperature were the main drivers in AEP, KEA, and EOA.
(5) EFRs were divided into three groups according to the intensity of human activities. In AEP and LEG, with high-intensity human interference, the landscape pattern was gradually fragmented; therefore, the degree of land use intensification should be enhanced and low-slope hills should be developed in pilot projects. In EOA and KEA, with a moderate intensity of human activities, attention should be given to reducing environmental damage caused by cluster development and optimizing industrial structure. In EFG and ERL with low human activity intensity, it is necessary to focus on monitoring the natural driving factors and improving the implementation quality of ecological governance by combining land planning and policymaking.
Data Availability Statement
The original contributions presented in the study are included in the article/Supplementary Material, further inquiries can be directed to the corresponding author/s.
Author Contributions
WN and FY designed the study and methodology. BX and BL extracted and compiled the data. ZB analyzed the data with the help of RW, YS, and WL. All authors contributed to the article and approved the submitted version.
Funding
This research was financially supported by the Zhejiang Province Key Research and Development Project under Grant No. 2019C02023 and the Zhejiang Provincial Natural Science Foundation of China under Grant No. LY19C160007.
Conflict of Interest
The authors declare that the research was conducted in the absence of any commercial or financial relationships that could be construed as a potential conflict of interest.
Publisher's Note
All claims expressed in this article are solely those of the authors and do not necessarily represent those of their affiliated organizations, or those of the publisher, the editors and the reviewers. Any product that may be evaluated in this article, or claim that may be made by its manufacturer, is not guaranteed or endorsed by the publisher.
Supplementary Material
The Supplementary Material for this article can be found online at: https://www.frontiersin.org/articles/10.3389/fevo.2022.954232/full#supplementary-material
References
Abadie, J., Dupouey, J.-L., Avon, C., Rochel, X., Tatoni, T., and Bergès, L. (2018). Forest recovery since 1860 in a Mediterranean region: drivers and implications for land use and land cover spatial distribution. Landsc. Ecol. 33, 289–305. doi: 10.1007/s10980-017-0601-0
Abrantes, P., Fontes, I., Gomes, E., and Rocha, J. (2016). Compliance of land cover changes with municipal land use planning: evidence from the Lisbon metropolitan region (1990–2007). Land Use Pol. 51, 120–134. doi: 10.1016/j.landusepol.2015.10.023
Amuti, T., and Luo, G. (2014). Analysis of land cover change and its driving forces in a desert oasis landscape of Xinjiang, Northwest China. Solid Earth 5, 1071–1085. doi: 10.5194/se-5-1071-2014
Asgarian, A., Amiri, B. J., and Sakieh, Y. (2015). Assessing the effect of green cover spatial patterns on urban land surface temperature using landscape metrics approach. Urban Ecosyst. 18, 209–222. doi: 10.1007/s11252-014-0387-7
Calaboni, A., Tambosi, L. R., Igari, A. T., Farinaci, J. S., Metzger, J. P., and Uriarte, M. (2018). The forest transition in São Paulo, Brazil. Ecol. Soc. 23, 230407. doi: 10.5751/ES-10270-230407
Chefaoui, R. M. (2014). Landscape metrics as indicators of coastal morphology: a multi-scale approach. Ecol. Indic. 45, 139–147. doi: 10.1016/j.ecolind.2014.04.004
Chen, X., Pan, J.-J., Wang, W.-Y., and Li, B.-Y. (2016). Landscape patterns characteristics of soil heavy metal pollution in a town of Southern Jiangsu. Environ. Sci. 37, 2313–2321. doi: 10.13227/j.hjkx.2016.06.040
Chi, Y., Zhang, Z., Gao, J., Xie, Z., Zhao, M., and Wang, E. (2019). Evaluating landscape ecological sensitivity of an estuarine island based on landscape pattern across temporal and spatial scales. Ecol. Indic. 101, 221–237. doi: 10.1016/j.ecolind.2019.01.012
Dadashpoor, H., Azizi, P., and Moghadasi, M. (2019). Land use change, urbanization, and change in landscape pattern in a metropolitan area. Sci. Total Environ. 655, 707–719. doi: 10.1016/j.scitotenv.2018.11.267
Dai, L., Liu, Y., and Luo, X. (2021). Integrating the MCR and DOI models to construct an ecological security network for the urban agglomeration around Poyang Lake, China. Sci. Total Environ. 754:141868. doi: 10.1016/j.scitotenv.2020.141868
Ding, J., Jiang, Y., Liu, Q., Hou, Z., Liao, J., Fu, L., et al. (2016). Influences of the land use pattern on water quality in low-order streams of the Dongjiang River basin, China: a multi-scale analysis. Sci. Total Environ. 551, 205–216. doi: 10.1016/j.scitotenv.2016.01.162
Du, C., Jia, L., and Lin, L. (2020). In-site landscape study in mountainous cities: origin, progress, and opportunities. Chin. Landsc. Archit. 36, 6–12. doi: 10.19775/j.cla.2020.12.0006
Enaruvbe, G., and Atafo, O. (2019). Land cover transition and fragmentation of River Ogba catchment in Benin City, Nigeria. Sustain. Cities Soc. 45, 70–78. doi: 10.1016/j.scs.2018.11.022
Fan, M., Wang, Q., Mi, K., and Peng, Y. (2017). Scale-dependent effects of landscape pattern on plant diversity in Hunshandak Sandland. Biodivers. Conserv. 26, 2169–2185. doi: 10.1007/s10531-017-1351-7
Fan, Q., and Ding, S. (2016). Landscape pattern changes at a county scale: a case study in Fengqiu, Henan Province, China from 1990 to 2013. Catena. 137, 152–160. doi: 10.1016/j.catena.2015.09.012
Feng, Y., Liu, Y., and Tong, X. (2018). Spatiotemporal variation of landscape patterns and their spatial determinants in Shanghai, China. Ecol. Indic. 87, 22–32. doi: 10.1016/j.ecolind.2017.12.034
Feng, Z., Yang, Y., Zhang, Y., Zhang, P., and Li, Y. (2005). Grain-for-green policy and its impacts on grain supply in West China. Land Use Pol. 22, 301–312. doi: 10.1016/j.landusepol.2004.05.004
Frondoni, R., Mollo, B., and Capotorti, G. (2011). A landscape analysis of land cover change in the Municipality of Rome (Italy): spatio-temporal characteristics and ecological implications of land cover transitions from 1954 to 2001. Landsc. Urban Plann. 100, 117–128. doi: 10.1016/j.landurbplan.2010.12.002
Fu, B., Liang, D., and Lu, N. (2011). Landscape ecology: Coupling of pattern, process, and scale. Chin. Geogr. Sci. 21:385. doi: 10.1007/s11769-011-0480-2
Gao, C., Zhou, P., Jia, P., Liu, Z., Wei, L., and Tian, H. (2016). Spatial driving forces of dominant land use/land cover transformations in the Dongjiang River watershed, Southern China. Environ. Monit. Assess. 188, 84. doi: 10.1007/s10661-015-5088-z
Gao, J., Liu, Y., and Chen, J. (2020). China's initiatives towards rural land system reform. Land Use Pol. 94, 104567. doi: 10.1016/j.landusepol.2020.104567
Group, G. D. R. (2017). The “Anji Code” of Ecological Civilization Construction, Guangming Daily. Beijing: Guangming Daily.
Hebin, W., Wen, J. W, and Ming, J. (2022). Impacts of future climate and land use/cover changes on water-related ecosystem services in Changbai mountains, Northeast China. Front. Ecol. Evol. 10, 206. doi: 10.3389/fevo.2022.854497
Herold, M., Scepan, J., and Clarke, K. C. (2002). The use of remote sensing and landscape metrics to describe structures and changes in urban land uses. Environ. Plan. A. 34, 1443–1458. doi: 10.1068/a3496
Hijmans, R. J., Cameron, S. E., Parra, J. L., Jones, P. G., and Jarvis, A. (2005). Very high resolution interpolated climate surfaces for global land areas. Int. J. Climatol. 25, 1965–1978. doi: 10.1002/joc.1276
Hoyos, L. E., Cabido, M. R., and Cingolani, A. M. (2018). A multivariate approach to study drivers of land-cover changes through remote sensing in the Dry Chaco of Argentina. ISPRS Int. J. Geo-Inf. 7, 170. doi: 10.3390/ijgi7050170
Hu, R., Du, S., and Li, P. (2021). Analysis of landscape fragmentation and driving forces in semi-arid ecologically fragile regions based on the moving window method. J. Agri. Resour. Environ. 38, 502–511. doi: 10.13254/j.jarc.2020.0490
Jia, L. Y., Ma, Q., Du, C. L., Hu, G., and Shang, C. W. (2020). Rapid urbanization in a mountainous landscape: patterns, drivers, and planning implications. Landsc. Ecol. 35, 2449–2469. doi: 10.1007/s10980-020-01056-y
Jiao, M., Hu, M., and Xia, B. (2019). Spatiotemporal dynamic simulation of land-use and landscape-pattern in the Pearl River Delta, China. Sustain. Cities Soc. 49, 101581. doi: 10.1016/j.scs.2019.101581
Kienast, F., Frick, J., van Strien, M. J., and Hunziker, M. (2015). The Swiss Landscape Monitoring Program–a comprehensive indicator set to measure landscape change. Ecol. Model. 295, 136–150. doi: 10.1016/j.ecolmodel.2014.08.008
Li, P., Zuo, D., Xu, Z., Zhang, R., Han, Y., Sun, W., et al. (2021). Dynamic changes of land use/cover and landscape pattern in a typical alpine river basin of the Qinghai-Tibet Plateau, China. Land Degrad. Dev. 32, 4327–4339. doi: 10.1002/ldr.4039
Li, Y., Sun, X., Zhu, X., and Cao, H. (2010). An early warning method of landscape ecological security in rapid urbanizing coastal areas and its application in Xiamen, China. Ecol. Model. 221, 2251–2260. doi: 10.1016/j.ecolmodel.2010.04.016
Liu, M., Hu, Y., Zhang, W., Zhu, J., Chen, H., and Xi, F. (2011). Application of land-use change model in guiding regional planning: a case study in Hun-Taizi River watershed, Northeast China. Chin. Geogr. Sci. 21, 609–618. doi: 10.1007/s11769-011-0497-6
Liu, Q. H., Liu, Y. X., Peng, J., Zhang, T., Li, Y. R., and Hu, Y. N. (2018). Linking GRNN and neighborhood selection algorithm to assess land suitability in low-slope hilly areas. Ecol. Indic. 93, 581–590. doi: 10.1016/j.ecolind.2018.05.008
Liu, W., Shi, C., Ma, Y., Li, H., and Ma, X. (2021). Land use and land cover change-induced changes of sediment connectivity and their effects on sediment yield in a catchment on the Loess Plateau in China. CATENA 207, 105688. doi: 10.1016/j.catena.2021.105688
Liu, Y., Fu, B., Wang, S., and Zhao, W. (2018). Global ecological regionalization: from biogeography to ecosystem services. Curr. Opin. Environ. Sustain. 33, 1–8. doi: 10.1016/j.cosust.2018.02.002
Liu, Y., Liu, S., Sun, Y., Wang, F., and Li, M. (2021). Driving forces of cultivated land evolution in agro-pastoral areas on the Qinghai-Tibet Plateau based on ecological niche theory. J. Cleaner Prod. 313, 127899. doi: 10.1016/j.jclepro.2021.127899
Long, H., Zhang, Y., and Tu, S. (2019). Rural vitalization in China: a perspective of land consolidation. J. Geog. Sci. 29, 517–530. doi: 10.1007/s11442-019-1599-9
Luo, Y., Lu, Y., Liu, L., Liang, H., Li, T., and Ren, Y. (2020). Spatiotemporal scale and integrative methods matter for quantifying the driving forces of land cover change. Sci. Total Environ. 739, 139622. doi: 10.1016/j.scitotenv.2020.139622
Ma, L., Bo, J., Li, X., Fang, F., and Cheng, W. (2019). Identifying key landscape pattern indices influencing the ecological security of inland river basin: the middle and lower reaches of Shule River Basin as an example. Sci. Total Environ. 674, 424–438. doi: 10.1016/j.scitotenv.2019.04.107
Mallinis, G., Koutsias, N., and Arianoutsou, M. (2014). Monitoring land use/land cover transformations from 1945 to 2007 in two peri-urban mountainous areas of Athens metropolitan area, Greece. Sci. Total Environ. 490, 262–278. doi: 10.1016/j.scitotenv.2014.04.129
Meng-wen, W., Wei, Q., Peng-tao, W., and Zhuo-ran, W. (2020). Relationship of landscape pattern and non-point source pollution in mountainous area of Eastern Shandong province. J. Nat. Resou. 35, 12003007. doi: 10.31497/zrzyxb.20201215
Miguet, P., Jackson, H. B., Jackson, N. D., Martin, A. E., and Fahrig, L. (2016). What determines the spatial extent of landscape effects on species? Landsc. Ecol. 31, 1177–1194. doi: 10.1007/s10980-015-0314-1
Moraga, A. D., Martin, A. E., and Fahrig, L. (2019). The scale of effect of landscape context varies with the species' response variable measured. Landsc. Ecol. 34, 703–715. doi: 10.1007/s10980-019-00808-9
Nahuelhual, L., Carmona, A., Aguayo, M., and Echeverria, C. (2014). Land use change and ecosystem services provision: a case study of recreation and ecotourism opportunities in southern Chile. Landsc. Ecol. 29, 329–344. doi: 10.1007/s10980-013-9958-x
Nie, W., Shi, Y., Siaw, M. J., Yang, F., Wu, R., Wu, X., et al. (2021). Constructing and optimizing ecological network at county and town Scale: the case of Anji County, China. Ecol. Indic. 132, 108294. doi: 10.1016/j.ecolind.2021.108294
Peng, J., Liu, Y., Liu, Z., and Yang, Y. (2017). Mapping spatial non-stationarity of human-natural factors associated with agricultural landscape multifunctionality in Beijing–Tianjin–Hebei region, China. Agric. Ecosyst. Environ. 246, 221–233. doi: 10.1016/j.agee.2017.06.007
Peng, J., Yang, Y., Liu, Y., Hu, Y., Du, Y., Meersmans, J., et al. (2018). Linking ecosystem services and circuit theory to identify ecological security patterns. Sci. Total Environ. 644, 781–790. doi: 10.1016/j.scitotenv.2018.06.292
Petersen, M., Bergmann, C., Roden, P., and Nüsser, M. J. L. D. (2021). Contextualizing land-use and land-cover change with local knowledge: a case study from Pokot Central, Kenya. Land Degrad. Dev. 32, 2992–3007. doi: 10.1002/ldr.3961
Qiao, J., and Li, X. (2006). Impact of LULC on human-land systems: a micro-study of three villages in Gongyi City, Henan Province. Res. Soil. Water. Conserv. 13, 91–94. (In chinese).
Quintero-Gallego, M. E., Quintero-Angel, M., and Vila-Ortega, J. J. (2018). Exploring land use/land cover change and drivers in Andean mountains in Colombia: a case in rural Quindío. Sci. Total Environ. 634, 1288–1299. doi: 10.1016/j.scitotenv.2018.03.359
Saarikoski, H., Primmer, E., Saarela, S.-R., Antunes, P., Aszalós, R., Bar,ó, F., et al. (2018). Institutional challenges in putting ecosystem service knowledge in practice. Ecosyst. Serv. 29, 579–598. doi: 10.1016/j.ecoser.2017.07.019
Shi, P., Zhang, Y., Li, Z., Li, P., and Xu, G. (2017). Influence of land use and land cover patterns on seasonal water quality at multi-spatial scales. Catena 151, 182–190. doi: 10.1016/j.catena.2016.12.017
Simonit, S., and Perrings, C. (2013). Bundling ecosystem services in the Panama Canal watershed. Proc. Natl. Acad. Sci. U. S. A. 110, 9326–9331. doi: 10.1073/pnas.1112242110
Sterling, S. M., Ducharne, A., and Polcher, J. (2013). The impact of global land-cover change on the terrestrial water cycle. Nat. Clim. Change. 3, 385–390. doi: 10.1038/nclimate1690
Trangmar, B. B., Yost, R. S., and Uehara, G. (1986). Application of geostatistics to spatial studies of soil properties. Adv. Agron. 38, 45–94. doi: 10.1016/S0065-2113(08)60673-2
Wang, J.-F., Zhang, T.-L., and Fu, B.-J. (2016). A measure of spatial stratified heterogeneity. Ecol. Indic. 67, 250–256. doi: 10.1016/j.ecolind.2016.02.052
Wang, J. F., Li, X. H., Christakos, G., Liao, Y. L., Zhang, T., Gu, X., et al. (2010). Geographical detectors-based health risk assessment and its application in the neural tube defects study of the Heshun Region, China. J. Geogr. Inf. Sci. 24, 107–127. doi: 10.1080/13658810802443457
Wang, L.-J., Ma, S., Zhao, Y.-G., and Zhang, J.-C. (2021). Ecological restoration projects did not increase the value of all ecosystem services in Northeast China. For. Ecol. Manag. 495:119340. doi: 10.1016/j.foreco.2021.119340
Wu, J., and Hobbs, R. (2002). Key issues and research priorities in landscape ecology: an idiosyncratic synthesis. Landsc. Ecol. 17, 355–365. doi: 10.1023/A:1020561630963
Wuyun, D., Sun, L., Chen, Z., Hou, A., Crusiol, L. G. T., Yu, L., et al. (2021). The spatiotemporal change of cropland and its impact on vegetation dynamics in the farming-pastoral ecotone of northern China. Sci. Total Environ. 805, 150286. doi: 10.1016/j.scitotenv.2021.150286
Xie, G., Zhang, C., Zhang, L., Su, D., Cao, S.-y., Leng, Y.-F., et al. (2012). China's county-scale ecological regionalization. J. Nat. Resou. 27, 154–162. doi: 10.11849/zrzyxb.2012.01.016
Xu, S., Li, S.-L., Zhong, J., and Li, C. (2020). Spatial scale effects of the variable relationships between landscape pattern and water quality: example from an agricultural karst river basin, Southwestern China. Agric. Ecosyst. Environ. 300:106999. doi: 10.1016/j.agee.2020.106999
Xu, W., Wang, J., Zhang, M., and Li, S. (2021). Construction of landscape ecological network based on landscape ecological risk assessment in a large-scale opencast coal mine area. J. Cleaner Prod. 286, 125523. doi: 10.1016/j.jclepro.2020.125523
Xu, Z., Peng, J., Dong, J., Liu, Y., Liu, Q., Lyu, D., et al. (2022). Spatial correlation between the changes of ecosystem service supply and demand: an ecological zoning approach. Landsc. Urban Plann. 217, 104258. doi: 10.1016/j.landurbplan.2021.104258
Yackulic, C. B., Fagan, M., Jain, M., Jina, A., Lim, Y., Marlier, M., et al. (2011). Biophysical and socioeconomic factors associated with forest transitions at multiple spatial and temporal scales. Ecol. Soc. 16, 160315. doi: 10.5751/ES-04275-160315
Ying, B., and Fang, Y. (2017). The classification of China mountain areas and its spatial distribution characteristics. J. Guizhou Norm. Univ. 35, 7–14. doi: 10.16614/j.cnki.issn1004-5570.2017.05.002
Zhang, L., Zhao, Y., Yin, S., Fang, S., Liu, X., and Pu, M. (2014). Gradient analysis of dry valley of Minjiang River landscape pattern, based on moving window method. Acta Ecol. Sin. 34, 3276–3284. doi: 10.5846/stxb201310312639
Zhang, M., Wang, J. M., Li, S. J., Feng, D. R., and Cao, E. (2020). Dynamic changes in landscape pattern in a large-scale opencast coal mine area from 1986 to 2015: a complex network approach. Catena 194, 104738. doi: 10.1016/j.catena.2020.104738
Zhang, Q., Chen, C., Wang, J., Yang, D., Zhang, Y., Wang, Z., et al. (2020). The spatial granularity effect, changing landscape patterns, and suitable landscape metrics in the Three Gorges Reservoir Area, 1995–2015. Ecol. Indic. 114, 106259. doi: 10.1016/j.ecolind.2020.106259
Zhang, X., Luo, S., and Zhang, J. (2021b). “Anji Case, Zhejiang Experience, and China Approach of Rural Revitalization,” in 2021 6th International Conference on Social Sciences and Economic Development (ICSSED 2021). (Paris: Atlantis Press), 827–830. doi: 10.2991/assehr.k.210407.157
Zhang, X., Wang, G., Xue, B., Zhang, M., and Tan, Z. (2021a). Dynamic landscapes and the driving forces in the Yellow River Delta wetland region in the past four decades. Sci. Total Environ. 787, 147644. doi: 10.1016/j.scitotenv.2021.147644
Zhong, X., Xu, Q., Yi, J., and Jin, L. (2022). Study on the threshold relationship between landscape pattern and water quality considering spatial scale effect—a case study of Dianchi Lake Basin in China. Environ. Sci. Pollut. Res. 2022, 1–16. doi: 10.1007/s11356-022-18970-0
Zhou, Y., Li, X., and Liu, Y. (2020). Land use change and driving factors in rural China during the period 1995-2015. Land Use Pol. 99, 105048. doi: 10.1016/j.landusepol.2020.105048
Keywords: land use/cover change, landscape pattern evolution, spatiotemporal characteristics, driving forces, environmental function regions, granularity and extent
Citation: Nie W, Yang F, Xu B, Bao Z, Shi Y, Liu B, Wu R and Lin W (2022) Spatiotemporal Evolution of Landscape Patterns and Their Driving Forces Under Optimal Granularity and the Extent at the County and the Environmental Functional Regional Scales. Front. Ecol. Evol. 10:954232. doi: 10.3389/fevo.2022.954232
Received: 27 May 2022; Accepted: 20 June 2022;
Published: 18 July 2022.
Edited by:
Changchun Huang, Nanjing Normal University, ChinaReviewed by:
Hongfei Yang, Anhui Normal University, ChinaChen Lin, Nanjing Institute of Geography and Limnology (CAS), China
Copyright © 2022 Nie, Yang, Xu, Bao, Shi, Liu, Wu and Lin. This is an open-access article distributed under the terms of the Creative Commons Attribution License (CC BY). The use, distribution or reproduction in other forums is permitted, provided the original author(s) and the copyright owner(s) are credited and that the original publication in this journal is cited, in accordance with accepted academic practice. No use, distribution or reproduction is permitted which does not comply with these terms.
*Correspondence: Fan Yang, eWYxOTg0MzYxMUAxNjMuY29t
†These authors have contributed equally to this work and share first authorship