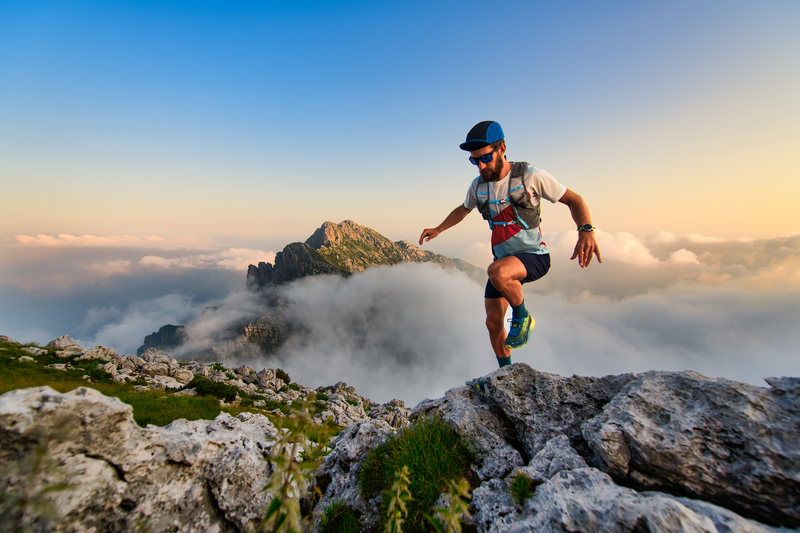
94% of researchers rate our articles as excellent or good
Learn more about the work of our research integrity team to safeguard the quality of each article we publish.
Find out more
ORIGINAL RESEARCH article
Front. Ecol. Evol. , 04 August 2022
Sec. Interdisciplinary Climate Studies
Volume 10 - 2022 | https://doi.org/10.3389/fevo.2022.941520
This article is part of the Research Topic Assessment of The Nexus among Energy, Climate and Environment in The Context of Carbon Neutrality View all 5 articles
China is the largest carbon emitter in the world; thus, reducing carbon emissions while maintaining economic growth has become an important issue. Within the context of carbon neutrality strategies, calculation of the carbon footprint and embodied carbon transfer can help policymakers formulate reasonable carbon reduction plans. The multi–regional input–output (MRIO) model can clarify carbon flow pathways between regions, and social network analysis (SNA) can comprehensively evaluate the different positions of individual sectors. Combining these two approaches, the specific characteristics of carbon emissions in complex production and trade relationships can be analyzed. China has become the world’s top total carbon emitter, and the Hanjiang River basin (HJRB) constitutes an important economic link between the developed and less developed regions of China. Studying carbon emissions in the HJRB can provide a reference for other, similar regions and is vital for the realization of China’s carbon emission reduction targets. This paper examines the carbon footprint and embodied carbon emission transfer among three provinces and 12 sectors in the HJRB during different periods and identifies the key industries in the carbon transfer process. The results indicate that (1) the total carbon footprint in the HJRB exhibits an increasing trend. Energy-based Shaanxi Province exhibits the highest growth rate of the carbon footprint, agriculture-based Henan Province shows a decreasing trend, and consumption-based Hubei Province displays the lowest carbon footprint intensity. (2) There are differences in the carbon emission coefficient and final consumption rate among various sectors; construction, metal processing and metal and non-metallic products, processing and manufacturing of petroleum, coking, nuclear fuel, chemical products, and other services are the sectors accounting for a high proportion of emissions. (3) The more obvious the supply relationship is, the higher the flow of embodied carbon emission transfer between sectors. (4) Energy-based regions transfer large amounts of fossil energy, electricity, steel and coal resources to developed regions and simultaneously assume more of the carbon reduction pressure imposed on developed regions. (5) The key industries within the embodied carbon emission transfer network notably control the carbon emissions of other industries and can provide breakthroughs to achieve challenging carbon emission reduction targets.
- This study proposes a new approach to characterizing complex production and trade of carbon emissions.
- Embodied carbon emission transfer leads to subtle transfers in carbon emission responsibilities originally shouldered by developed regions to less-developed regions.
- Focusing on direct and embodied carbon emissions is necessary to achieve more challenging carbon reduction targets.
Global warming is one of the most important environmental problems facing human society today. Carbon emission reduction to combat climate change has become a global consensus, and as of March 2022, more than 130 countries worldwide have committed to achieving carbon neutrality (Adams and Acheampong, 2019; Richardson, 2022). In December 2015, the success of the Climate Conference in Paris demonstrated the world’s determination to cooperate to protect the environment, and this conference resulted in concrete arrangements for countries worldwide to take action against climate change after 2020, representing a historic point in the global effort to tackle carbon reduction problems. It should be noted that carbon emission reduction is not only a regional environmental problem but also an environmental problem that can be transferred to other regions through industrial transfer and trade transactions. Suppose only a single region were considered in the formulation of a carbon emission reduction policy. In this case, the effect of carbon emission reduction among different regions may be reduced, resulting in the situation whereby carbon emissions are down in certain regions but increasing nationwide, which does not facilitate carbon emission reduction target achievement.
To efficiently achieve carbon emission reduction targets, specific carbon reduction responsibilities must be allocated and adjusted among different regions according to local conditions (Zhou and Wang, 2016; Gopalakrishnan et al., 2021). However, different regions exhibit varying economic levels, resource endowments and consumption patterns, and to meet their economic development needs, goods and services are frequently traded between regions, especially between developing regions containing abundant energy resources and developed regions (Wang et al., 2018c). Currently, the carbon accounting system based on the producer responsibility principle ignores the impact of regional trade (Choi, 2017), and a region can avoid a portion of its carbon emissions while importing products to meet local production and consumption demands (Lv et al., 2019). In other words, carbon emission responsibilities are transferred from one region to another with interregional trade (Li Y. L. et al., 2018). Therefore, a scientific carbon emission reduction policy must consider direct carbon emissions and embodied carbon emission transfer from the perspective of consumption. In contrast to direct emissions originating from energy combustion, embodied carbon emissions are hidden behind trade and flow from one region to another in response to regional trade. Thus, embodied carbon emissions are more difficult to account for than direct carbon emissions (Liu et al., 2015).
The carbon footprint is considered an indicator that can reasonably reflect carbon emissions (Chen G. et al., 2016; Moran et al., 2018). The carbon footprint method allows a more accurate assessment of consumption-based carbon emissions, including direct and embodied carbon emissions resulting from production processes to produce goods for final consumption. As an assessment indicator, the carbon footprint greatly expands the application scope of carbon emission policy formulation and research and provides theoretical support for carbon emission responsibility division, which has been widely considered worldwide (Yue et al., 2020; Isz et al., 2021).
There are three main carbon footprint accounting methods: the life cycle assessment (LCA) method, the methodology based on the calculation of emission coefficients for fossil fuels [the Intergovernmental Panel on Climate Change (IPCC) method], and the input–output analysis (IOA) method. Different methods rely on distinct calculation ideas with varying application conditions. The LCA method is a top-down calculation method that determines the total carbon emissions based on all the input and output data for a given product throughout its life cycle (including the entire process of raw material extraction, production and processing, storage and transportation, use and waste disposal). This method facilitates comprehensive analysis of carbon emissions in all segments, but the acquisition and organization processes of data are highly complex, resulting in a lack of operability (Fenner et al., 2018; Kavehei et al., 2018; Correa et al., 2019). The IPCC method is a detailed guide prepared by the IPCC, which can comprehensively consider greenhouse gas emissions attributed to the combustion of different fuels, with easy access to data and a simple calculation process, but this method is only applicable to study the carbon footprint of similarly closed silos but cannot obtain the embodied carbon emissions from a consumption perspective (Muthu et al., 2011; Zeng et al., 2021). The IOA method is a bottom-up calculation method that uses input–output tables to calculate the carbon footprint. Much previous literature has used the single–region input–output (SRIO) model, which assumes that production technologies are homogeneous but does not consider the intermediate products of inputs in the production process, resulting in notable deviation between the calculated results and reality (Sun et al., 2017; Wieland et al., 2020). With further IOA refinement, an increasing number of studies have used multi–regional input–output (MRIO) models to calculate the carbon footprint (Lin et al., 2017). The MRIO model reflects the relationship between initial, intermediate and total inputs, intermediate and final products and total output through an equilibrium equation, which can capture the relationship between the direct and embodied carbon emissions in each sector of the economic system and can also overcome the problem of duplication or omission of carbon emission calculations due to complex intersectoral production relationships (Sodersten et al., 2018), providing a practical method to quantify pollutant emissions and cross-regional transfer of resource consumption (Wang et al., 2018c; Cabernard and Pfister, 2021; Yuan et al., 2022).
The MRIO model can analyze the impact of a certain industry on other industries. However, this model cannot identify the importance of the considered sector among several industries nor describe the overall state of the industry network (Yuan et al., 2022). Social network analysis (SNA) can complement the MRIO model, which is a method for the evaluation of the interaction among different parts and elucidation of the corresponding structural state (Tabassum et al., 2018; Can and Alatas, 2019). The manufacture of products and services usually requires the cooperation of multiple sectors, so embodied carbon emission transfer can occur between different regions and between various sectors. The SNA method helps reveal the characteristics of the complex embodied carbon flows in cross-regional trade.
China is the largest primary energy consumer and carbon emitter in the world, facing pressure from countries worldwide to reduce carbon emissions (Zhang et al., 2011). With the rapid development of China’s economy, the extensive economic growth model has led to an exponential increase in resource and energy consumption, pollutant discharge and carbon emissions, posing very high environmental and climate risks to society (Cai et al., 2018). Although the growth rate of carbon emissions in China has declined due to aggressive environmental policies and slowing economic growth, China still poses a challenge to international carbon reduction targets because of its large economy and dependence on fossil fuels. The Hanjiang River is the longest tributary of the Yangtze River, with a total length of 1,577 km and a basin area of approximately 159,000 km2. Figure 1 shows that the Hanjiang River basin (HJRB) is an important economic link between Northwest and Central China. The mainstream flows through Shaanxi, Henan, and Hubei Provinces (Zhu et al., 2022). The study of carbon emissions in the HJRB is important for the realization of challenging carbon reduction targets in China.
This study calculated the carbon footprint in different provinces and constructed an embodied carbon emission transfer network, choosing the HJRB in China as an example. Compared to previous studies, the main contribution of this study includes the adopted methodology and data. Methodologically, we combined the MRIO model with the SNA method to identify core industries with a key carbon reduction position (Fu et al., 2017; Wen and Wang, 2019). Compared to previous methods, the proposed method can quantify the direct and indirect linkages between sectors, which can help identify sectors with key positions in complex production and consumption processes. This study can support decision-makers in the development of targeted carbon reduction policies. In terms of data, previous studies mainly used single-year data to calculate the carbon footprint, which cannot reflect temporal changes (Weinzettel et al., 2014; Wu and Liu, 2016; Lin et al., 2017; Yuan et al., 2022). This study combined MRIO tables for 3 years to analyze changes in the carbon footprint and embodied carbon emission transfer on temporal and spatial scales.
The carbon footprint is generally used to characterize the direct and indirect greenhouse gas emissions of a product or service over its life cycle (Matthews et al., 2008). The result is expressed in terms of its carbon dioxide equivalent to distinguish it from the general concept of carbon emissions, which uses the absolute mass of carbon dioxide as the unit of measurement. In terms of carbon footprint accounting, there is a more mainstream consensus that the carbon footprint refers to all greenhouse gas emissions from the final consumption of a product or service and its production process (Hertwich and Peters, 2009; Shi and Yin, 2021). Accounting for greenhouse gas emissions from a whole-industry perspective can provide a basis for finding key carbon emission sectors, optimizing the layout of industrial structure, and quantifying emission reduction responsibility. Therefore, carbon footprints have become an important indicator for evaluating sustainability (Cucek et al., 2012).
As an example of the application of economic principles in the environmental field (Lenzen et al., 2012; Kanemoto et al., 2014; Sun et al., 2020), the MRIO model is able to track information on the geospatial distribution of environmental impacts, thus providing a practical way to quantify the cross-regional transfer of waste emissions or resource consumption. Currently, the MRIO model has become the most widely used and effective carbon footprint accounting model (Dawkins et al., 2019). The MRIO model is a “top-down” analysis that reflects the relationship among initial inputs, intermediate inputs, total inputs and intermediate outputs, final outputs, and total outputs in a sector (Kucukvar et al., 2016). It allocates the final consumption of a product to the various stages of production and tracks the nodes that contribute the most to carbon emissions (Ali, 2017). Essentially, it converts the economic relationship among sectors or regions into the physical relationship of carbon emissions, whereby the exchange process of emissions is reflected and apportioned to each sector or region, thus clarifying the direct and indirect carbon emission relationships (Wiedmann, 2009). Carbon footprint accounting based on the MRIO model can be summarized into four steps: input–output table selection and processing, carbon footprint model construction, carbon footprint accounting, and result analysis. It is necessary to select input–output tables of appropriate scale and time according to the object and preprocess them, such as through sector aggregation, to reduce errors caused by factors such as differences in production technology and scale between sectors. The core of carbon footprint calculation with the MRIO model is the determination of the carbon intensity factor matrix, Leontief inverse matrix and final demand matrix. The carbon intensity factor matrix can be calculated from the energy consumption, energy carbon emission factor and sectoral output value from the statistical yearbook, and the Leontief inverse matrix and final demand matrix data are usually calculated from the input–output table.
The MRIO model has become the main method for carbon footprint accounting at the macro level (Sun et al., 2019), as it can reflect the direct and indirect carbon emission relationships among sectors within the economic system, overcome the problem of duplication or omission of calculation due to the complex production relationship among sectors, and reduce the uncertainty caused by system boundary delineation. However, there are some limitations in using the MRIO model to calculate carbon footprints. First, it takes considerable time and effort to compile input–output tables, which leads to a lag in using these tables to account for carbon footprints (Wiedmann et al., 2011; Malik et al., 2021). Second, there may be differences between the energy consumption data and the sectoral aggregation in the input–output table, leading to errors in the carbon footprint accounting results; moreover, sectoral aggregation also results in insufficient precision of the corresponding carbon emission factors (Heinonen et al., 2020). In addition, this method can only be applied at the sectoral or regional level, as there are large obstacles at the micro level (Hambÿe et al., 2018).
MRIO models are often combined with other methods to research carbon footprint issues, among which SNA has been widely adopted (Wei et al., 2017; Pompermayer Sesso et al., 2020). Production involves various complex relationships, and scholars have examined a series of characteristics behind these complex relationships in the attempt to abstract these relationships and explore their basic internal characteristics. Against this background, the concept of the SNA method has gradually been clarified, and its basic model has been established.
SNA is a method of applying graph theory and linear algebra to illustrate the structure of a network, defining the state of the system by analyzing the positions or roles of its participants and relationships (Zaw and Lim, 2017). An SNA system includes many objects and relationships; objects are treated as nodes in the network, relationships between objects form network connections, and a complex network system is built through these connections, which can be weighted or unweighted. SNA system networks can be classified into four types: undirected unweighted networks, undirected entitled networks, directed weighted networks, and directed unweighted networks.
The SNA method is a useful system modeling technique for complex problems (Maltseva and Batagelj, 2021). SNA has become one of the most widely used tools for visualizing the major intercountry/interindustry carbon emission flows along global production chains (Duan and Jiang, 2018) and has been applied to explore the drivers behind embodied energy flows (Shi et al., 2017; Chen B. et al., 2018; Gao et al., 2018), assess the contribution of supply chain clusters to global carbon emissions (Kagawa et al., 2015), and assess the impact of import/export trade on country carbon emissions (López et al., 2020).
Numerous studies have conducted industrial carbon transfer analyses, and while these studies have enriched our knowledge, some aspects of these studies need to be further addressed. Targeted policies on reducing carbon emissions must target the characteristics of carbon emissions across sectors to clarify their direct effects (Chen X. et al., 2016). Identifying key sectors based on province-specific industrial linkages allows for more precise control of sector-level carbon emissions. There are close and complex intersectoral production and carbon emission linkages between provinces, which vary in natural resources, energy consumption and industrial technology. Therefore, strategies to reduce carbon emissions need to vary from province to province. However, most previous studies have either selected key sectors at the national level (Kagawa et al., 2015; Shi et al., 2017; Chen B. et al., 2018; Duan and Jiang, 2018) or in individual provinces (Gao et al., 2018).
To address the above issues, key sectors in specific provinces were selected to determine the potential for carbon reduction in China based on the MRIO model and SNA method. The cross-regional transfer of carbon emissions is hidden in interregional trade flows, and the MRIO model is able to allocate the final consumption of products to various stages of production (Hubacek et al., 2014; Wiedmann and Lenzen, 2018). However, the MRIO model is unable to evaluate the spatial network characteristics of carbon footprints from the perspective of industrial structure (Serrano and Dietzenbacher, 2010). Therefore, combining the SNA method with the MRIO model is particularly important to research the spatial network characteristics of carbon emissions. The SNA method is used to construct an industrial carbon footprint network, and the analysis of network nodes is used to determine the position of each industry in the whole network, find the production link that contributes most to the carbon footprint, and provide a basis for industrial carbon reduction policies (Sun et al., 2020). In addition, the SNA method can study the interregional flow of embodied carbon emissions along with the trade of products and services, which can reveal the overall structural characteristics of the embodied carbon emission transfer network among regions and reflect the pattern of regional trade relations and carbon emissions.
The data used in this study include China’s MRIO tables (Zheng et al., 2021), provincial energy consumption, emission coefficients for the major energy sources, and other statistical information (population, GDP, etc.). The data are listed in Table 1.
Due to the different statistical methods between energy consumption data and MRIO tables, to maintain a consistent data quality for subsequent analysis, we referred to a previous method in the literature to merge the MRIO table containing 42 sectors into an MRIO table containing 12 sectors and divided these sectors into 3 major industries (Yuan et al., 2022). The merged results are summarized in Table 2.
This study used the MRIO model to calculate the carbon footprint and embodied carbon emission transfer. The MRIO model is suitable for multiregional environmental studies and can track trade relationships among different regions or sectors (Wiedmann et al., 2011; Martinez et al., 2018; Wang et al., 2018c; Cabernard and Pfister, 2021; Xu et al., 2022). In such an MRIO model, different regions are linked by interregional trade. The basic framework is provided in Table 3.
In region r of the MRIO model, production activity can be formulated in a balanced form as follows:
where denotes the total output of sector i in region r, which is the sum of the intermediate input and final consumption. More specifically, denotes the intermediate input originating from sector i in region r to sector j in region s, and denotes the final consumption, which refers to the amount of final product supplied by sector i in region r and consumed in region s. The direct consumption coefficient can be expressed as follows:
where denotes the amount of input provided by sector i in region r required to satisfy the needs of one unit of output of sector j in region s, is the total input stemming from sector j, and . Then, Equation (1) can be rewritten as:
The mathematical structure of the MRIO model comprises a set of (m × n) linear equations, in which n refers to the number of regions and m refers to the number of sectors. Then, Equation (3) can be extended in matrix form as follows:
which, with obvious definitions, exhibits the following form:
where X is the matrix of the total output, A is the matrix of the direct consumption coefficients , and Y is the matrix of the final consumption . Solving for X, we can obtain the following:
where (I−A)−1 is the Leontief inverse matrix, which can be expressed as L = (I−A)−1. This matrix represents the total amount of both the direct and indirect inputs to satisfy one unit of final consumption and exhibits the following matrix form:
The prerequisite for carbon footprint determination is the calculation of the total direct carbon emissions in each province. Based on carbon emission coefficient data published by the IPCC, the total direct carbon emissions in each province can be calculated based on energy consumption data (Sperow, 2020) as follows:
where Pa denotes the total direct carbon emissions in province a, n is the number of energy types, Ei, NCVi, CEFi, and COFi denote the energy consumption, average net calorific value, average carbonation rate and carbon oxidation rate, respectively, of different energy sources in province a, and their values can be obtained from guidelines for the preparation of provincial greenhouse gas inventories. The carbon emission coefficient for standard coal is the recommended value by the Energy Research Institute of the National Development and Reform Commission (Yu et al., 2014).
After direct carbon emission calculation, the direct carbon emission coefficient is multiplied by the Leontief inverse matrix L = (I−A)−1 and the final consumption Y to obtain the complete carbon emission coefficients for each sector in province a. The corresponding equations are as follows:
where C is the carbon footprint, ei, Pi, and Xi are the direct carbon emission coefficients, direct carbon emissions and total output, respectively, and E is the direct carbon emission coefficient matrix. Ad is the matrix of direct consumption coefficients (production activities to meet domestic needs), and Yd is the matrix of domestic final consumption.
It is important to note that this study focused only on carbon emissions generated within China and excluded carbon emissions contained in international imports of goods due to insufficient provincial information. On the one hand, international imports in the HJRB accounted for only 4.05% of the total production in 2017. On the other hand, previous literature has suggested that the impact of this component is relatively limited (Wang et al., 2018c).
The embodied carbon emission transfer calculated with the MRIO model must be combined with the direct carbon emissions. Therefore, an environmentally expanded input–output (EEIO) model was developed. The core of the EEIO model entails the construction of multilateral regional trade input–output relationships (Jiang et al., 2020), which can be formulated as follows.
where Lr denotes the total emission coefficients of the MRIO table, and E is a diagonal matrix comprising the direct carbon emission coefficients for each sector. Combining the Leontief inverse matrix L = (I−A)−1 and final consumption Y of the MRIO model, the embodied carbon emission transfer matrix CT can thus be formulated as:
where CTrs denotes the embodied carbon transfer from region r to region s. More specifically, the row vector denotes the scale of embodied carbon input from region r to region s, and the column vector denotes the scale of embodied carbon output from region s to region r.
The variation coefficient of carbon input/output is a statistic used to evaluate the degree of variation. The commonly used standard variation coefficient is denoted by CV, which is the ratio of the standard deviation to the mean and is expressed as follows:
The embodied carbon transfer network collects carbon flow relationships accompanying trade and industrial transfer (Lv et al., 2019). The industries in each province of the HJRB are the nodes of the embodied carbon emission transfer network, and the carbon relationships between industries are the network links. All the nodes and links constitute the embodied carbon emission transfer network, and the degree centrality, closeness centrality, and betweenness centrality are the main indicators used to measure the characteristics of this network.
All nodes and linkages form the embodied carbon emission transfer directed network matrix CT, and ctrs is an element of matrix CT, which denotes the carbon emission transfer from regions r and s. is the critical value of the average carbon flux between different regions. The CT matrix is converted to a binary matrix R and can thus be formulated as:
If ctrs is greater than or equal to the critical value, the corresponding element of the matrix R is assigned as 1. Otherwise, it is assigned as 0.
The overall structure of the network is generally characterized by the network density. Network density (Dn) is used to indicate the number and complexity of interregional carbon emission transfer network relationships. A higher network density indicates a greater impact on the carbon emission transfer relationship of each region.
where Dn is the network density, L is the actual number of carbon transfer relationships, N is the number of nodes in the carbon transfer network, and N×(N−1) is the maximum number of relationships that can be carried in the carbon transfer network.
The degree centrality is a measure of the importance of a given network node. The higher the degree centrality of a node, the more important the node is within the network (Liu et al., 2016). In other words, the industry with a high degree centrality is the center of the embodied carbon transfer network and strongly influences other industries. Within a directed network, the degree centrality can be divided into the in- and out-degree centralities, where the in-degree centrality represents the sum of all edges pointing to the node, which reflects the node ability to absorb internally, and the out-degree centrality represents the sum of all edges emanating from the node, which reflects the node ability to radiate externally (Wang et al., 2018b). These indicators can be calculated as follows:
where CDI is the in-degree centrality, Rji is the number of effective connections between nodes j and i, CDO is the out-degree centrality, Rij is the number of connections between nodes i and j, and n is the total number of nodes.
The closeness centrality indicates the importance of a certain node within the network from a distance perspective. If the distance between a node and other nodes is small, this suggests that the node is relatively important within the network (Meghanathan, 2018). Within the embodied carbon transfer network, a high closeness centrality of a node indicates that the distance between this node and other nodes is small. Similarly, if the closeness centrality of a node is low, this indicates that the node must follow a long path to connect with other nodes. The closeness centrality can be calculated as follows:
where Cci is the closeness centrality and l(i,j) is the shortest distance from node i to node j.
The betweenness centrality measures the interconnectivity of a node within the network and can reflect the extent to which the embodied carbon emission transfer between two other regions is indirectly connected to this region (Szczepanski et al., 2016). Within the embodied carbon emission transfer network, the higher the betweenness centrality of a node is, the more obvious the role of this node in bridging the carbon relationship to other nodes. The betweenness centrality can be calculated as follows:
where CB is the betweenness centrality, Rij denotes the number of shortest paths between nodes i and j, and Rij(ni) is the number of shortest paths between nodes i and j existing through node i.
Figure 2 shows the research design of this study, which combines the abovementioned methodologies and data inventories. First, we preprocess the input–output tables for 2012, 2015, and 2017 by combining the original 42 sectors into 12 sectors and three industries. Second, based on the IPCC emission coefficient method, each sector’s carbon emission intensity coefficients are calculated by using energy consumption data, energy emission coefficients and sectoral output value. Third, the MRIO model is applied to calculate the carbon footprint and embodied carbon transfer at the provincial and sectoral levels. Fourth, the SNA method is combined to construct a carbon emission transfer network among the three major industries in each province of the HJRB and identify the key sectors among them. Finally, we discuss the temporal and spatial variations in the carbon footprints of provinces and sectors in the HJRB, analyze the relationship of embodied carbon emission transfer, and propose policy recommendations for improving energy use efficiency and reducing embodied carbon emissions.
The total carbon footprint in the HJRB exhibits an increasing trend, while the carbon footprint intensity reveals a decreasing trend. As indicated in Table 4 and Figure 3, the total carbon footprint in the HJRB increased from 856.521 million tons (Mt) in 2012 to 879.14 Mt in 2017, with an average annual growth rate of 2.64%. Among the various provinces, the carbon footprint in Henan decreased instead of increased, but Henan remained the province with the highest total amount in the HJRB. The next fastest growing province is Shaanxi, whose carbon footprint was nearly four times the basin average. In addition, the carbon footprint in Hubei was the smallest in the HJRB. Contrary to the trend of the carbon footprint, the carbon footprint intensity decreased year by year in all provinces. The carbon footprint intensity is the carbon footprint contributed per unit of the gross domestic product (GDP), and this indicator is often used to measure the carbon contribution of the GDP (Dong et al., 2018). The total carbon footprint intensity in the HJRB reached 1.65 t in 2012, indicating that each 10,000 CNY of the GDP contributed 1.65 t to the carbon footprint, which decreased to 1.03 and 0.85 t in 2015 and 2017, respectively. It should be noted that Shaanxi surpassed Henan as the region with the highest carbon footprint intensity, in contrast to the carbon footprint ranking.
Table 4. Carbon footprint, carbon footprint per unit of the GDP, and per capita carbon footprint in the provinces of the HJRB in 2012, 2015, and 2017.
Figure 3. Carbon footprint, per capita carbon footprint, and carbon footprint per unit of the GDP in the provinces of the HJRB in 2012, 2015, and 2017.
The carbon footprint per capita in Henan indicated a decreasing trend, while that in the other provinces exhibited an increasing trend. The carbon footprint can be divided by the resident population to obtain the carbon footprint per capita, which can eliminate the effect of the population size on the carbon footprint (Solarin, 2019). The maximum-to-minimum carbon footprint per capita ratio in the HJRB approached 2.23, indicating a large difference between the different regions. The carbon footprint per capita in Henan decreased from 3.84 t in 2012 to 3.57 t in 2017, which is lower than the average level in the HJRB. The main reason is that Henan contains the largest resident population over the other provinces, accounting for approximately half of the total population of the HJRB. The carbon footprint in Henan decreased instead of increased, and the combination of a decreasing carbon footprint and increasing population resulted in Henan becoming the province with the smallest carbon footprint per capita.
The main reason for the above characteristics lies in the structural differences in industries between the different provinces. Shaanxi is a typical energy-based province, where coal, petroleum and chemical industries with high energy consumption levels and high carbon emission coefficients are relatively well developed, while light industry and services, with a greater contribution to the GDP, are developing more slowly. Therefore, energy-based regions, prone to high carbon emission coefficients and low GDP growth rates, and developing regions with a large total carbon footprint and high carbon footprint intensity, should constitute the main breakthrough point for future carbon reduction efforts. Henan is a typical agricultural and populous province, relying mainly on grain production and processing and other labor-intensive industries. The shares of non-fossil energy consumption and the resident population increased year by year, leading to a trend of year-to-year reduction in both the total carbon footprint and carbon footprint per capita. Hubei is a typical consumption-based province dominated by industrial manufacturing and service industries, with lower carbon emission coefficients and less fossil energy consumption, but this province notably contributes to the GDP growth rate. Therefore, the carbon footprint intensity in consumption-based regions is lower than that in energy- and agriculture-based regions.
Metal processing and metal and non-metallic products (S6), processing and manufacturing of petroleum, coking, nuclear fuel and chemical products (S5), other services (S12) and construction (S9) are the sectors with a large carbon footprint. An uneven distribution of resources and heterogeneity of the industrial structure lead to significant differences in the carbon footprint between different sectors (Wang et al., 2020). As indicated in Table 2, we reconstructed the original 42 sectors of the MRIO table into 12 sectors and denoted these sectors as S1–S12, and Figure 4 shows the sectoral composition characteristics of the carbon footprint in different years. In 2012 and 2015, the top 4 carbon footprints among the 12 sectors occurred in S9 (50.6%), S6 (40.9%), S5 (44.0%), and S12 (24.7%). In 2017, S6 jumped to the top with a high share of 58%, while the other sectors did not change significantly.
Figure 4. Sectoral composition of the carbon footprint in the provinces of the HJRB in 2012, 2015, and 2017.
Metal processing and metal and non-metallic products (S6) and processing and manufacturing of petroleum, coking, nuclear fuel and chemical products (S5) are supply sectors in the upper part of the production chain and achieve high carbon emission coefficients due to their high energy consumption and low production values, resulting in a large carbon footprint (Ma et al., 2019). The carbon footprint share of S5 in Shaanxi was much higher than that of the other provinces because Shaanxi is an energy-based province with abundant coal, oil, and natural gas resources, which provide energy and raw materials for other sectors (Lu et al., 2010). In addition, the carbon footprint share of S6 in Hubei was significantly higher than that of the other provinces. Hubei has a long history in the steel processing industry. A large number of steel products are used in the construction of buildings, machinery, vehicles, railroads and infrastructure, which require the consumption of large amounts of energy resources.
Although the other services (S12) sector attains a low carbon emission coefficient, its high final consumption rate results in a large carbon footprint. Since the service industry is mostly concentrated in less polluting industries, such as the restaurant, tourism and education sectors, its impact on the environment is easily overlooked. In fact, the service industry is not an emission-free sector. The energy consumption structure of the service industry mainly includes electricity, coal and natural gas, and the industrial scale is expanding globally (Yu et al., 2022). This indicates that the service sector has become a major sector of energy consumption and carbon emissions, and more attention should be given to energy restructuring and energy saving in the service sector in the future.
The carbon footprint in the construction (S9) sector increased with increasing urbanization. Urbanization plays an important role in the global carbon emissions process, especially in intercity transportation infrastructure such as high-speed railways and subways (Du et al., 2018). The demand for urbanization has led to the rapid development of various industries, such as highways, urban transportation and large urban buildings. The upstream sectors of these industries include carbon-intensive industries such as petrochemicals, steel, metals and chemicals. In addition, the current construction process is characterized by inefficient energy use and disordered construction methods, and effective measures are needed to obtain a balance between infrastructure expansion and environmental sustainability.
The embodied carbon emission transfer paths between sectors reflect the flow relationships in the supply chain. Figure 5 shows the embodied intersectoral carbon emission transfer within each province in different years. S2–S5 and S2–S8 were the two pathways with the highest embodied carbon transfer flow in Shaanxi. As an energy-based region, Shaanxi contains abundant fossil energy resources, whose extraction requires large amounts of electricity, natural gas and water, and the extracted coal, oil, and natural gas are heavily invested in the production of local chemical products. The embodied carbon emission transfer was higher under S1–S4 and S6–S9 in Henan. As an agriculture-based region, the agriculture, forestry, animal husbandry, and fishery industry in Henan provides raw materials for the production of grain, tobacco, and other agricultural byproducts. In addition, the rapid urbanization of Henan is an important reason for the large carbon footprint in the construction industry, which uses a large number of raw materials, such as metal products. In Hubei, the embodied carbon emission transfer flows of S12–S9 and S12–S10 were highly significant. As a consumption-based region, industries such as commerce, education, culture, and tourism are growing rapidly. Their upstream and downstream industries include a variety of sectors, such as construction, transportation, warehousing and accommodation and catering, which have become a major source of incremental carbon emissions (Mingxing et al., 2014; Li K. et al., 2018).
Figure 5. Carbon emission transfer between sectors in (A) Shaanxi, (B) Henan, and (C) Hubei of the HJRB in 2012, 2015, and 2017 (million tons).
The above results demonstrate that the implementation of efficient carbon reduction policies requires the full manifestation of intersectoral linkage effects. The supply relationships of raw and auxiliary materials, products, and byproducts between sectors with high carbon transfer flows are very obvious, and carbon emission reduction in sectors with a high embodied carbon output could reduce carbon emissions in other related sectors. For example, the implementation of carbon reduction measures in the agriculture, forestry, animal husbandry, and fishery industries can simultaneously reduce carbon emissions originating from the food processing industry.
The embodied carbon emission transfer paths between provinces reflect the flow relationships in the interprovincial trade chain. Figure 6 shows the interprovincial embodied carbon emission transfer in the HJRB in different years, and AS1–AS12, BS1–BS12, and CS2–CS12 represent the 12 sectors in Shaanxi, Henan, and Hubei, respectively. The total interprovincial embodied carbon emission transfer increased from 18.40 Mt in 2012 to 50.92 Mt in 2017, indicating that the flow of goods and services between provinces frequently occurred. The embodied carbon emission transfer between Shaanxi and Hubei was substantial and rapidly increased over time, indicating a close trade link between these provinces. As a consumption-based region with a focus on industrial manufacturing and service industries, there exist no abundant energy resources suitable for extraction, so this region must import large amounts of electricity, steel, coal and other resources from energy-intensive regions to sustain its social development and normal economic operations.
Figure 6. Embodied carbon emission transfer between the three provinces of the HJRB in (A) 2012, (B) 2015, and (C) 2017 (million tons).
The embodied carbon emission transfer from less developed to developed regions suggests that the pressure of carbon emission reduction is transferred from developed regions to other regions. Shaanxi remained the dominant province in terms of the embodied carbon emission output, exporting a large amount of heavy industrial products (e.g., steel and cement) and energy products (e.g., oil, coal, and electricity) to other regions, thus indicating that energy-based provinces play a pivotal role in regional economic development. The carbon emission reduction pressure is positively proportional to the output of embodied carbon emissions, which demonstrates that energy-based regions with the highest embodied carbon output bear a larger share of the carbon pressure than that borne by agricultural and consumption-based regions. Therefore, optimization of the energy consumption structure in regions with the highest embodied carbon emission output and carbon emission reduction can play a positive role in the carbon emission reduction efforts of the entire region.
Given today’s increasingly pressing carbon emissions problems, it is necessary to examine the economic structure in terms of carbon emissions. As indicated in Table 2, the 12 sectors in each province were combined into 3 major industries, where S1 indicates the primary industry, denoted as P, S2–S9 indicate the secondary industry, denoted as S, and S10–S12 indicate the tertiary industry, denoted as T. There are 9 nodes in the embodied carbon emission transfer network after sector merger, and A, B, and C represent Shaanxi, Henan, and Hubei, respectively. For example, AP denotes the primary industry in Shaanxi Province. To clarify the intersectoral trade relationship, we ignored carbon emission transfer between the three major industries in each province, recorded as 0. However, if the embodied carbon emission transfer between two industries in different provinces was less than 5% of the total amount, the effect could be considered negligible and was also recorded as 0. We use Ucinet, Gephi, and Visio to calculate centrality, create and embellish carbon transfer networks.
Analysis of the structural characteristics of carbon emission transfer networks can help identify the key industries influencing embodied carbon emission transfer across regions. As shown in Figure 7, the embodied carbon emission transfer networks in 2012, 2015, and 2017 showed increasing correlation, with a more stable network shape and good accessibility between nodes in the network. From 2012 to 2017, the carbon linkage between AP and BS became increasingly tight, while BS and CS became increasingly detached. The carbon linkage between AP and BP showed a change from tight to distant, while the change between AS and BS showed the opposite trend. The network tended to diversify in direction and tighten in connection, and most nodes moved closer to the center through their connection with the central nodes. However, the CP, BP, and CT industries had the lowest degree centrality in 2012, 2015, and 2017, indicating that these industries take long paths to connect to other industries and are vulnerable to influence by other industries. In other words, agriculture and the service industry in Hubei and agriculture in Henan occupied the edge of the embodied carbon emission transfer network. Overall, the embodied carbon emission transfer network showed a clear “core-edge” pattern.
Figure 7. Carbon emission transfer network among three major industries in the three provinces of the HJRB in (A) 2012, (B) 2015, and (C) 2017.
The central node had high point degree centrality, high influence on the whole network, and the core position in the network. Both BS and AS yielded a more significant betweenness centrality than that of the other industries in 2012, 2015, and 2017, indicating that the secondary industries in Henan and Shaanxi strongly control other industries as they had the highest linkage as an absolute measure among all industries. This result indicates that the carbon emissions in these industry clusters not only increase by supplying products to downstream industries but also induce more carbon emissions in similar industries than in other provinces. If these industries are affected, it could disrupt the carbon emission linkages between their upstream and downstream industries. However, the ability to control these industries can also be used to reduce carbon emission levels in other industries and contribute to overall carbon emission reduction in the HJRB.
The secondary industries in Henan and Shaanxi comprise the core of the embodied carbon emission transfer network, combining the ability to control outward and absorb inward. They occupied the dominant and key position in the whole network and had very strong control over other nodes. Most industrial sectors establish carbon relationships with other industries through them. The above results suggest that the control of these core industries over peripheral industries could be fully utilized to reduce carbon emissions and ultimately to reduce carbon emissions in the entire region.
In this study, we combined the MRIO model and SNA method and selected the HJRB as a case site to quantify the carbon footprint and embodied carbon emission transfer between the different provinces in 2012, 2015, and 2017. The following main findings were obtained:
Traditional urbanization and industrialization are marked by energy consumption, such as the consumption of coal, oil, and natural gas. In addition to their own emissions, these sectors contribute to increases in the carbon emissions in coal mining and dressing through interindustry linkages. Thus, the carbon footprint in energy-based regions increases at a significantly higher rate than that in agricultural and consumption-based regions (Yang and Huang, 2019). However, energy-based regions usually also belong to less developed regions with scattered industrial structures and poorly developed high technology, and most extracted energy products are transferred to developed regions. In other words, energy-based regions emit more carbon. However, manufactured energy products are consumed in developed regions upon trade, resulting in the transfer of the carbon emission reduction responsibility originally borne by developed regions to less developed regions via trade (Chen W. et al., 2018). Therefore, policy-makers must give more attention to the rationality and operability of policies when designing carbon emission reduction policies (Wang et al., 2011). It is necessary to consider the economic development status, consumption capacity of residents, and urban development potential in different regions and the embodied carbon emission transfer due to trade flows (Wang et al., 2018c).
In recent years, many regions have implemented actions to reduce carbon emissions in response to climate change. These actions have achieved the expected results, according to the decreasing trend of the carbon footprint intensity year by year. The carbon footprint intensity reflects the contribution of carbon emissions per unit of the GDP, and a decrease in the carbon footprint intensity indicates that the GDP is growing faster than the carbon footprint, demonstrating that these regions are implementing effective efforts to reduce carbon emissions while maintaining economic growth. This finding is also consistent with that in previous studies (Tian et al., 2014). In addition, the carbon footprint is largely related to production activities and residential consumption (Liu et al., 2022), and considering that the HJRB is still undergoing rapid urbanization, the total carbon footprint is expected to continue to increase in the future (Zhang Y. et al., 2020). Therefore, carbon emission reduction can be promoted from both production (e.g., encouraging factories to use clean raw materials) and consumption perspectives (e.g., enhancing the decarbonization of residential consumption activities).
In contrast to energy- and agriculture-based regions, which focus on sectors with a large carbon footprint, such as industry and agriculture, respectively, and occur on the supply side of the trade relationship, consumption-based regions rely more on sectors with a small carbon footprint, such as finance, education, culture, tourism, and high technology (Sun et al., 2021). These service sectors do not directly consume large amounts of fossil energy resources. However, their primary upstream industries include high-carbon emission industries such as electricity, construction, and transportation, leading to an increasingly obvious environmental impact on the service sector, which has become an important part of global carbon emissions.
Traditional industrial sectors, such as the mineral processing, fossil fuel and construction industries, are the main sources of carbon emissions. The construction sector is the largest carbon emissions driver in the HJRB. However, a side-by-side comparison among provinces revealed that this was not the case for all individual provinces. For example, the carbon footprint of the construction sector in Hubei was significantly higher than for other provinces in the HJRB. Thus, the construction sector is heterogeneous in different provinces, and it is inappropriate to adopt the same carbon reduction strategy in all provinces. The construction sector contributes significantly to carbon emissions because of its long supply chain and high demand for construction materials, building finishes, construction equipment and other construction-related products. Construction is a key sector in the HJRB, and thus, modernization and quality improvement are crucial and require a transition from material and capital-driven industries to technology-driven industries and from sloppy growth to intensive growth.
These industries should be encouraged to use cleaner energy and explore sustainable development models to achieve carbon reduction targets. However, it cannot be ignored that the restriction of the development of energy-intensive industries does not fundamentally solve the problem of carbon emissions and may even affect local social and economic development (Adams and Acheampong, 2019; Zhang W. et al., 2020). In addition, due to the industrial and trade linkages between different regions, carbon emission reduction measures implemented in one sector within a specific region may significantly impact other sectors in other regions (Wen and Wang, 2019). Therefore, further analysis of carbon relationships between different sectors is needed to clarify the flow and distribution of carbon emissions among sectors. An environmentally extended key sector analysis in the context of carbon emissions can help identify the sectors where mitigation policies are likely to be most effective.
Identifying key sectors and provinces is the basis for developing effective policies. In the case study in this paper, to analyze the carbon relationships between different sectors, we constructed an embodied carbon emission transfer network, which exhibits a more obvious and stable association structure. The results indicate that the secondary industries in Henan and Shaanxi notably control other industries. These findings can help policymakers better understand the position and role of each sector in economic development and identify key industries with significant potential for carbon reduction to facilitate more targeted carbon reduction programs.
Unlike previous studies, we combine the MRIO model and the SNA method to identify key provinces and sectors in the HJRB that deserve more attention with regard to carbon reduction targets and economic development. In addition, by combining intersector production and carbon emission linkages, our approach quantifies direct and indirect cross-sectoral linkages more clearly than previous approaches. Moreover, the results generated by SNA elucidate the relationship between economic structure and carbon emissions to help researchers better understand the role of each sector and province in the HJRB in carbon emissions and economic development and identify key sectors and provinces for emissions reduction. This information can help policymakers develop more targeted strategies to ultimately achieve the economic transformation and carbon reduction goals in the HJRB.
Based on the above analysis, to achieve more challenging carbon reduction targets, this paper proposes the following policy recommendations:
First, to achieve long-term overall carbon reduction in the HJRB, it is necessary for future policies to focus on both production and consumption. On the production side, to curb the growth in energy consumption at the source, the government should encourage key sectors located upstream in the supply chain to develop low-carbon production technologies, use cleaner raw materials and intermediate products, and provide low-carbon products and services to downstream sectors. On the consumption side, it is necessary to cultivate awareness of low-carbon consumption among key sectors and consumers located downstream of the supply chain and reduce the final consumption of high-carbon products (Wang et al., 2018a). For example, suppliers or consumers of products and services should prioritize clean partners and encourage the industry to move toward low-carbon production from the consumer side.
Second, policymakers should not ignore the intersectoral linkages between provinces. Multiregional collaboration is indispensable for the achievement of ambitious carbon reduction targets, but the difference in technology level between regions is the main obstacle to carbon reduction (Suk et al., 2016). As previously mentioned, due to regional differences in the production and consumption of energy products, less developed regions bear more of the carbon emission reduction pressure than that borne by developed regions. Therefore, developed regions should invest more effort in the development of low-carbon technologies and clean energy and proactively support less developed regions in improving their technology to replace energy-intensive technologies. To reduce the additional costs associated with technological upgrading, tax subsidies should be employed to encourage less-developed regions to use clean energy in their products as much as possible (Mi et al., 2016; Wang et al., 2019). In addition, policy instruments should be used to raise the market access threshold to limit the entry of high-carbon emission industries into the market (Xu et al., 2017).
Third, as different resource endowments lead to the movement of resources and products and the consequent carbon emission transfer between provinces, there is a need to implement targeted carbon reduction policies in key provinces. For provinces that import carbon-intensive products from other provinces to meet their demand, the demand for carbon-intensive products can be limited administratively, and the consumption of cleaner products can be encouraged through taxes and subsidies (Fang and Chen, 2018; Wen and Wang, 2019). Restructuring a province’s consumption can reduce its dependence on fossil fuels, which can be achieved by buying more products from provinces with low-carbon linkages and encouraging suppliers to reduce their carbon emissions (Feng et al., 2014). For provinces that export products containing significant carbon emissions to other provinces, their demand patterns need to be optimized to reduce carbon emissions and transfers. Policies such as a carbon tax could be implemented for these provinces, depending on the actual situation, to encourage them to produce less carbon-intensive products. In addition, it is possible to reduce direct emissions from the production process by providing high-value-added, low-carbon emission products through technological advances and adjusting the product production structure (Huang et al., 2015).
Finally, policymakers need to develop targeted sectoral carbon reduction policies because these key sectors can spread carbon reduction trends to other sectors. The most common key carbon reduction sector is the electricity production and supply sector due to the huge demand for energy, particularly in Shaanxi Province. As a fundamental industry in China, the electricity production and supply sector has a very large carbon footprint, so it is important to take measures to manage it. Other industries, such as the mineral processing, fossil fuel and construction industries, have large carbon outputs, which are always associated with high energy consumption and emission intensity. Thus, the emission intensity of these industries should be reduced through technical means, such as improving power generation technology, energy extraction technology, and adjusting the structure of energy consumption. These measures will enable non-conventional power generation technologies such as thermal, hydro, wind, and nuclear power to replace coal (Wen and Wang, 2019).
There are certain limitations in this study. First, the input–output table provides data for 42 sectors, which we combined into 12 sectors based on intersectoral relationships to facilitate calculation and analysis. In addition, there may be differences between the energy consumption data and the sectoral aggregation in the input–output table, leading to some errors in the carbon footprint accounting results. Sectoral aggregation also results in deficient precision of the corresponding carbon emission coefficients.
Second, carbon footprint accounting with input–output tables reflects the relationship between economic activities and environmental emissions in a given year. However, the long time required to prepare input–output tables and their slow updating have led to some studies having to use input–output data from previous years, resulting in errors in the calculation results. The data used in this study were published in 2012, 2015, and 2017 and may not reflect the current situation. The 2017 MRIO table was published in July 2020 and contains the most recent data currently available.
Third, because input–output tables use intersectoral production relationships to reflect economic activities at the regional scale, carbon emissions can only be attributed to the sectoral level when accounting for carbon footprints. Compared with LCA, the MRIO model has larger errors at the micro level, such as organization and product, and it is difficult to accurately account for the carbon emissions of a specific link. Therefore, carbon footprint studies based on the MRIO model are mainly restricted to the macro level, such as trade, industry, and region.
In general, although the MRIO model has greater challenges in data acquisition, model construction, and department aggregation, its advantages cannot be ignored. The MRIO model reflects the complex production relationship among sectors, and this approach to carbon footprint accounting can distinguish direct and indirect carbon emissions, avoiding the errors that exist in the LCA method. In addition, once the input–output table is constructed, the accounting results can be obtained more quickly without spending too many resources, which has better economic benefits.
Input–output tables are the basis of the MRIO model, and in future work, we can construct input–output tables with more detailed and reliable sectoral classification, closer environmental impact, and more regional coverage. In addition, we can use MRIO models to study the impact of social, economic and natural factors on carbon emissions and the greenhouse effect, which is important for the development of environmental and energy policies. Especially in the context of the Paris Agreement, the prediction of the carbon footprint under different policy and technology scenarios may affect the future trends of the industrial structure and demand structure.
Although the carbon footprint scenario analysis based on the MRIO model still has some shortcomings (e.g., the assumption of a linear relationship between inputs and outputs), the method still has good explanatory power for changes in the carbon footprint due to long-term discontinuous changes in production structure. Carbon footprint scenario analysis has greater potential to predict sectoral technology levels, intersectoral production structure relationships, demand structures, energy quality, and technological changes.
This paper proposed a method based on the MRIO model and SNA method and analyzed the carbon footprint and embodied carbon emission transfer during different periods with the HJRB as a case study. The main findings are as follows:
The total carbon footprint in the HJRB exhibits an increasing trend, and the different provinces reveal significant differences depending on the industrial structure. The carbon emission coefficient and final consumption rate are important factors influencing the carbon footprint in a given sector. The embodied carbon emission transfer pathways across sectors and regions reflect intersectoral linkage effects and interregional trade relationships, respectively. The embodied carbon transfer network reveals key industries with notable control over carbon emissions stemming from other industries.
To achieve more challenging carbon reduction targets, rational and actionable policies based on an analysis of carbon relationships across regions and sectors are needed from multiple perspectives, including production, consumption, regional cooperation, and financial support.
The case study in this paper demonstrated that the MRIO model can determine the carbon footprint and embodied carbon emission transfer in complex regional trade relationships. The SNA method can portray the interrelationships among different industries through visual network mapping and can identify key sectors. Compared to previous methods, this method considers regional trade and sectoral production. Additionally, this approach reflects the relationship between trade chains (production and consumption) and supply chains (supply and demand side). This study could provide a theoretical approach for policymakers in the development of more targeted carbon reduction programs and a case reference for other similar regions in the assessment of carbon relationships across regions and sectors.
The original contributions presented in this study are included in the article/supplementary material, further inquiries can be directed to the corresponding author/s.
KZ: methodology, visualization, formal analysis, data curation, and writing–original draft. QL, XX, and YZ: data curation. MW: validation and resources. HL: conceptualization, funding acquisition, supervision, and writing–review and editing. All authors contributed to the article and approved the submitted version.
This research was supported by the National Natural Science Foundation of China (NO. 41971402).
The authors declare that the research was conducted in the absence of any commercial or financial relationships that could be construed as a potential conflict of interest.
All claims expressed in this article are solely those of the authors and do not necessarily represent those of their affiliated organizations, or those of the publisher, the editors and the reviewers. Any product that may be evaluated in this article, or claim that may be made by its manufacturer, is not guaranteed or endorsed by the publisher.
CF, Carbon Footprint; CFPGDP, Carbon Footprint Per Unit of Gross Domestic Product; CEADs, Carbon Emission Accounts Datasets; EEIO, Environmentally Expanded Input–Output, GDP, Gross Domestic Product; HJRB, Hanjiang River Basin; IPCC, Intergovernmental Panel on Climate Change; IOA, Input–Output Analysis; LCA, Life Cycle Assessment; MRIO, Multi–Regional Input–Output; Mt, Million Tons; NBSC, National Bureau of Statistics of China; PCF, Per Capita Carbon Footprint; SNA, Social Network Analysis; SRIO, Single–Region Input–Output.
Adams, S., and Acheampong, A. O. (2019). Reducing carbon emissions: the role of renewable energy and democracy. J. Clean. Prod. 240:118245. doi: 10.1016/j.jclepro.2019.118245
Ali, Y. (2017). Carbon, water and land use accounting: consumption vs production perspectives. Renew. Sust. Energy Rev. 67, 921–934. doi: 10.1016/j.rser.2016.09.022
Cabernard, L., and Pfister, S. (2021). A highly resolved MRIO database for analyzing environmental footprints and Green Economy Progress. Sci. Total Environ. 755:142587. doi: 10.1016/j.scitotenv.2020.142587
Cai, B., Wang, X., Huang, G., Wang, J., Cao, D., Baetz, B. W., et al. (2018). Spatiotemporal changes of China’s carbon emissions. Geophys. Res. Lett. 45, 8536–8546. doi: 10.1029/2018GL079564
Can, U., and Alatas, B. (2019). A new direction in social network analysis: online social network analysis problems and applications. Physica A 535:122372. doi: 10.1016/j.physa.2019.122372
Chen, B., Li, J. S., Wu, X. F., Han, M. Y., Zeng, L., Li, Z., et al. (2018). Global energy flows embodied in international trade: a combination of environmentally extended input–output analysis and complex network analysis. Appl. Energy 210, 98–107. doi: 10.1016/j.apenergy.2017.10.113
Chen, W., Shen, Y., and Wang, Y. (2018). Evaluation of economic transformation and upgrading of resource-based cities in Shaanxi province based on an improved TOPSIS method. Sustain. Cities Soc. 37, 232–240. doi: 10.1016/j.scs.2017.11.019
Chen, G., Wiedmann, T., Wang, Y., and Hadjikakou, M. (2016). Transnational city carbon footprint networks - exploring carbon links between Australian and Chinese cities. Appl. Energy 184, 1082–1092. doi: 10.1016/j.apenergy.2016.08.053
Chen, X., Chan, C. K., and Lee, Y. C. E. (2016). Responsible production policies with substitution and carbon emissions trading. J. Clean. Prod. 134, 642–651. doi: 10.1016/j.jclepro.2015.10.083
Choi, T. (2017). Environmental impact of voluntary extended producer responsibility: the case of carpet recycling. Resour. Conserv. Recycl. 127, 76–84. doi: 10.1016/j.resconrec.2017.08.020
Correa, J. P., Montalvo-Navarrete, J. M., and Hidalgo-Salazar, M. A. (2019). Carbon footprint considerations for biocomposite materials for sustainable products: a review. J. Clean. Prod. 208, 785–794. doi: 10.1016/j.jclepro.2018.10.099
Cucek, L., Klemes, J. J., and Kravanja, Z. (2012). A review of footprint analysis tools for monitoring impacts on sustainability. J. Clean. Prod. 34, 9–20. doi: 10.1016/j.jclepro.2012.02.036
Dawkins, E., Moran, D., Palm, V., Wood, R., and Björk, I. (2019). The Swedish footprint: a multi-model comparison. J. Clean. Prod. 209, 1578–1592. doi: 10.1016/j.jclepro.2018.11.023
Dong, F., Yu, B., Hadachin, T., Dai, Y., Wang, Y., Zhang, S., et al. (2018). Drivers of carbon emission intensity change in China. Resour. Conserv. Recycl. 129, 187–201. doi: 10.1016/j.resconrec.2017.10.035
Du, Q., Lu, X., Li, Y., Wu, M., Bai, L., and Yu, M. (2018). Carbon emissions in China’s construction industry: calculations, factors and regions. Int. J. Environ. Res. Public Health 15:1220. doi: 10.3390/ijerph15061220
Duan, Y., and Jiang, X. (2018). Visualizing the change of embodied CO2 emissions along global production chains. J. Clean. Prod. 194, 499–514. doi: 10.1016/j.jclepro.2018.05.133
Fang, D., and Chen, B. (2018). Linkage analysis for water-carbon nexus in China. Appl. Energy 225, 682–695. doi: 10.1016/j.apenergy.2018.05.058
Feng, K., Hubacek, K., Sun, L., and Liu, Z. (2014). Consumption-based CO2 accounting of China’s megacities: the case of Beijing, Tianjin, Shanghai and Chongqing. Ecol. Indic. 47, 26–31. doi: 10.1016/j.ecolind.2014.04.045
Fenner, A. E., Kibert, C. J., Woo, J., Morque, S., Razkenari, M., Hakim, H., et al. (2018). The carbon footprint of buildings: a review of methodologies and applications. Renew. Sustain. Energy Rev. 94, 1142–1152. doi: 10.1016/j.rser.2018.07.012
Fu, X., Lahr, M., Yaxiong, Z., and Meng, B. (2017). Actions on climate change, reducing carbon emissions in China via optimal interregional industry shifts. Energy Policy 102, 616–638. doi: 10.1016/j.enpol.2016.10.038
Gao, C., Su, B., Sun, M., Zhang, X., and Zhang, Z. (2018). Interprovincial transfer of embodied primary energy in China: a complex network approach. Appl. Energy 215, 792–807. doi: 10.1016/j.apenergy.2018.02.075
Gopalakrishnan, S., Granot, D., Granot, F., Sosic, G., and Cui, H. (2021). Incentives and emission responsibility allocation in supply chains. Manag. Sci. 67, 4172–4190. doi: 10.1287/mnsc.2020.3724
Hambÿe, C., Hertveldt, B., and Michel, B. (2018). Does consistency with detailed national data matter for calculating carbon footprints with global multi-regional input–output tables? A comparative analysis for Belgium based on a structural decomposition. Econ. Struct. 7:11. doi: 10.1186/s40008-018-0110-6
Heinonen, J., Ottelin, J., Ala-Mantila, S., Wiedmann, T., Clarke, J., and Junnila, S. (2020). Spatial consumption-based carbon footprint assessments - a review of recent developments in the field. J. Clean. Prod. 256:120335. doi: 10.1016/j.jclepro.2020.120335
Hertwich, E. G., and Peters, G. P. (2009). Carbon footprint of nations: a global, trade-linked analysis. Environ. Sci. Technol. 43, 6414–6420. doi: 10.1021/es803496a
Huang, G., Ouyang, X., and Yao, X. (2015). Dynamics of China’s regional carbon emissions under gradient economic development mode. Ecol. Indic. 51, 197–204. doi: 10.1016/j.ecolind.2014.07.040
Hubacek, K., Feng, K., Minx, J. C., Pfister, S., and Zhou, N. (2014). Teleconnecting consumption to environmental impacts at multiple spatial scales research frontiers in environmental footprinting. J. Ind. Ecol. 18, 7–9. doi: 10.1111/jiec.12082
Isz, A., Aab, C., Mmad, E., and Bd, B. (2021). Magnitudes of households’ carbon footprint in Iskandar Malaysia: policy implications for sustainable development. J. Clean. Prod. 315:128042. doi: 10.1016/j.jclepro.2021.128042
Jiang, M., Liu, L., Behrens, P., Wang, T., Tang, Z., Chen, D., et al. (2020). Improving subnational input-output analyses using regional trade data: a case-study and comparison. Environ. Sci. Technol. 54, 12732–12741. doi: 10.1021/acs.est.0c04728
Kagawa, S., Suh, S., Hubacek, K., Wiedmann, T., Nansai, K., and Minx, J. (2015). CO2 emission clusters within global supply chain networks: implications for climate change mitigation. Glob. Environ. Change 35, 486–496. doi: 10.1016/j.gloenvcha.2015.04.003
Kanemoto, K., Moran, D., Lenzen, M., and Geschke, A. (2014). International trade undermines national emission reduction targets: new evidence from air pollution. Glob. Environ. Change 24, 52–59. doi: 10.1016/j.gloenvcha.2013.09.008
Kavehei, E., Jenkins, G. A., Adame, M. F., and Lemckert, C. (2018). Carbon sequestration potential for mitigating the carbon footprint of green stormwater infrastructure. Renew. Sustain. Energy Rev. 94, 1179–1191. doi: 10.1016/j.rser.2018.07.002
Kucukvar, M., Cansev, B., Egilmez, G., Onat, N. C., and Samadi, H. (2016). Energy-climate-manufacturing nexus: new insights from the regional and global supply chains of manufacturing industries. Appl. Energy 184, 889–904. doi: 10.1016/j.apenergy.2016.03.068
Lenzen, M., Kanemoto, K., Moran, D., and Geschke, A. (2012). Mapping the structure of the world economy. Environ. Sci. Technol. 46, 8374–8381. doi: 10.1021/es300171x
Li, K., Fang, L., and He, L. (2018). How urbanization affects China’s energy efficiency: a spatial econometric analysis. J. Clean. Prod. 200, 1130–1141. doi: 10.1016/j.jclepro.2018.07.234
Li, Y. L., Chen, B., Han, M. Y., Dunford, M., Liu, W., and Li, Z. (2018). Tracking carbon transfers embodied in Chinese municipalities’ domestic and foreign trade. J. Clean. Prod. 192, 950–960. doi: 10.1016/j.jclepro.2018.04.230
Lin, J., Hu, Y., Zhao, X., Shi, L., and Kang, J. (2017). Developing a city-centric global multiregional input-output model (CCG-MRIO) to evaluate urban carbon footprints. Energy Policy 108, 460–466. doi: 10.1016/j.enpol.2017.06.008
Liu, H., Liu, W., Fan, X., and Liu, Z. (2015). Carbon emissions embodied in value added chains in China. J. Clean. Prod. 103, 362–370. doi: 10.1016/j.jclepro.2014.09.077
Liu, Y., Jiang, Y., Liu, H., Li, B., and Yuan, J. (2022). Driving factors of carbon emissions in China’s municipalities: a LMDI approach. Environ. Sci. Pollut. Res. 29, 21789–21802. doi: 10.1007/s11356-021-17277-w
Liu, Y., Wei, B., Du, Y., Xiao, F., and Deng, Y. (2016). Identifying influential spreaders by weight degree centrality in complex networks. Chaos Solitons Fractals 86, 1–7. doi: 10.1016/j.chaos.2016.01.030
López, L. A., Arce, G., and Jiang, X. (2020). Mapping China’s flows of emissions in the world’s carbon footprint: a network approach of production layers. Energy Econ. 87:104739. doi: 10.1016/j.eneco.2020.104739
Lu, C., Zhang, X., and He, J. (2010). A CGE analysis to study the impacts of energy investment on economic growth and carbon dioxide emission: a case of Shaanxi Province in western China. Energy 35, 4319–4327. doi: 10.1016/j.energy.2009.04.007
Lv, K., Feng, X., Kelly, S., Zhu, L., and Deng, M. (2019). A study on embodied carbon transfer at the provincial level of China from a social network perspective. J. Clean. Prod. 225, 1089–1104. doi: 10.1016/j.jclepro.2019.03.233
Ma, X., Wang, C., Dong, B., Gu, G., Chen, R., Li, Y., et al. (2019). Carbon emissions from energy consumption in China: its measurement and driving factors. Sci. Total Environ. 648, 1411–1420. doi: 10.1016/j.scitotenv.2018.08.183
Malik, A., Egan, M., du Plessis, M., and Lenzen, M. (2021). Managing sustainability using financial accounting data: the value of input-output analysis. J. Clean. Prod. 293:126128. doi: 10.1016/j.jclepro.2021.126128
Maltseva, D., and Batagelj, V. (2021). Journals publishing social network analysis. Scientometrics 126, 3593–3620. doi: 10.1007/s11192-021-03889-z
Martinez, S., del Mar Delgado, M., Martinez Marin, R., and Alvarez, S. (2018). The environmental footprint of the end-of-life phase of a dam through a hybrid-MRIO analysis. Build. Environ. 146, 143–151. doi: 10.1016/j.buildenv.2018.09.049
Matthews, H. S., Hendrickson, C. T., and Weber, C. L. (2008). The importance of carbon footprint estimation boundaries. Environ. Sci. Technol. 42, 5839–5842. doi: 10.1021/es703112w
Meghanathan, N. (2018). delta-Space for real-world networks: a correlation analysis of decay centrality vs. degree centrality and closeness centrality. J. King Saud Univ. Comput. Inf. Sci. 30, 391–403. doi: 10.1016/j.jksuci.2017.04.006
Mi, Z., Zhang, Y., Guan, D., Shan, Y., Liu, Z., Cong, R., et al. (2016). Consumption-based emission accounting for Chinese cities. Appl. Energy 184, 1073–1081. doi: 10.1016/j.apenergy.2016.06.094
Mingxing, C., Yongbin, H., Zhipeng, T., Dadao, L., Hui, L., and Li, M. (2014). The provincial pattern of the relationship between urbanization and economic development in China. J. Geogr. Sci. 24, 33–45. doi: 10.1007/s11442-014-1071-9
Moran, D., Kanemoto, K., Jiborn, M., Wood, R., Tobben, J., and Seto, K. C. (2018). Carbon footprints of 13 000 cities. Environ. Res. Lett. 13:064041. doi: 10.1088/1748-9326/aac72a
Muthu, S. S., Li, Y., Hu, J. Y., and Mok, P. Y. (2011). Carbon footprint of shopping (grocery) bags in China, Hong Kong and India. Atmos. Environ. 45, 469–475. doi: 10.1016/j.atmosenv.2010.09.054
Pompermayer Sesso, P., Amâncio-Vieira, S. F., Zapparoli, I. D., and Sesso Filho, U. A. (2020). Structural decomposition of variations of carbon dioxide emissions for the United States, the European Union and BRIC. J. Clean. Prod. 252:119761. doi: 10.1016/j.jclepro.2019.119761
Richardson, M. T. (2022). Prospects for detecting accelerated global warming. Geophys. Res. Lett. 49:e2021GL095782. doi: 10.1029/2021GL095782
Serrano, M., and Dietzenbacher, E. (2010). Responsibility and trade emission balances: an evaluation of approaches. Ecol. Econ. 69, 2224–2232. doi: 10.1016/j.ecolecon.2010.06.008
Shi, J., Li, H., Guan, J., Sun, X., Guan, Q., and Liu, X. (2017). Evolutionary features of global embodied energy flow between sectors: a complex network approach. Energy 140, 395–405. doi: 10.1016/j.energy.2017.08.124
Shi, S., and Yin, J. (2021). Global research on carbon footprint: a scientometric review. Environ. Impact Assess. Rev. 89:106571. doi: 10.1016/j.eiar.2021.106571
Sodersten, C.-J. H., Wood, R., and Hertwich, E. G. (2018). Endogenizing capital in MRIO models: the implications for consumption-based accounting. Environ. Sci. Technol. 52, 13250–13259. doi: 10.1021/acs.est.8b02791
Solarin, S. A. (2019). Convergence in CO(2)emissions, carbon footprint and ecological footprint: evidence from OECD countries. Environ. Sci. Pollut. Res. 26, 6167–6181. doi: 10.1007/s11356-018-3993-8
Sperow, M. (2020). Updated potential soil carbon sequestration rates on US agricultural land based on the 2019 IPCC guidelines. Soil Tillage Res. 204:104719. doi: 10.1016/j.still.2020.104719
Suk, S., Lee, S.-Y., and Jeong, Y. S. (2016). A survey on the impediments to low carbon technology investment of the petrochemical industry in Korea. J. Clean. Prod. 133, 576–588. doi: 10.1016/j.jclepro.2016.05.132
Sun, C., Ding, D., and Yang, M. (2017). Estimating the complete CO2 emissions and the carbon intensity in India: from the carbon transfer perspective. Energy Policy 109, 418–427. doi: 10.1016/j.enpol.2017.07.022
Sun, L., Qin, L., Taghizadeh-Hesary, F., Zhang, J., Mohsin, M., and Chaudhry, I. S. (2020). Analyzing carbon emission transfer network structure among provinces in China: new evidence from social network analysis. Environ. Sci. Pollut. Res. 27, 23281–23300. doi: 10.1007/s11356-020-08911-0
Sun, Y., Qian, L., and Liu, Z. (2021). The carbon emissions level of China’s service industry: an analysis of characteristics and influencing factors. Environ. Dev. Sustain. doi: 10.1007/s10668-021-02001-y [Epub ahead of print].
Sun, Z., Tukker, A., and Behrens, P. (2019). Going global to local: connecting top-down accounting and local impacts, a methodological review of spatially explicit input-output approaches. Environ. Sci. Technol. 53, 1048–1062. doi: 10.1021/acs.est.8b03148
Szczepanski, P. L., Michalak, T. P., and Rahwan, T. (2016). Efficient algorithms for game-theoretic betweenness centrality. Artif. Intell. 231, 39–63. doi: 10.1016/j.artint.2015.11.001
Tabassum, S., Pereira, F. S. F., Fernandes, S., and Gama, J. (2018). Social network analysis: an overview. Wiley Interdiscip. Rev. Data Min. Knowl. Discov. 8:e1256. doi: 10.1002/widm.1256
Tian, X., Chang, M., Lin, C., and Tanikawa, H. (2014). China’s carbon footprint: a regional perspective on the effect of transitions in consumption and production patterns. Appl. Energy 123, 19–28. doi: 10.1016/j.apenergy.2014.02.016
Wang, C., Engels, A., and Wang, Z. (2018a). Overview of research on China’s transition to low-carbon development: the role of cities, technologies, industries and the energy system. Renew. Sustain. Energy Rev. 81, 1350–1364. doi: 10.1016/j.rser.2017.05.099
Wang, J., Li, C., and Xia, C. (2018b). Improved centrality indicators to characterize the nodal spreading capability in complex networks. Appl. Math. Comput. 334, 388–400. doi: 10.1016/j.amc.2018.04.028
Wang, Z., Yang, Y., and Wang, B. (2018c). Carbon footprints and embodied CO2 transfers among provinces in China. Renew. Sustain. Energy Rev. 82, 1068–1078. doi: 10.1016/j.rser.2017.09.057
Wang, M., Li, Y., Li, M., Shi, W., and Quan, S. (2019). Will carbon tax affect the strategy and performance of low-carbon technology sharing between enterprises? J. Clean. Prod. 210, 724–737. doi: 10.1016/j.jclepro.2018.10.321
Wang, R., Liu, W., Xiao, L., Liu, J., and Kao, W. (2011). Path towards achieving of China’s 2020 carbon emission reduction target-a discussion of low-carbon energy policies at province level. Energy Policy 39, 2740–2747. doi: 10.1016/j.enpol.2011.02.043
Wang, Y., Zhang, D., Ji, Q., and Shi, X. (2020). Regional renewable energy development in China: a multidimensional assessment. Renew. Sustain. Energy Rev. 124:109797. doi: 10.1016/j.rser.2020.109797
Wei, J., Huang, K., Yang, S., Li, Y., Hu, T., and Zhang, Y. (2017). Driving forces analysis of energy-related carbon dioxide (CO2) emissions in Beijing: an input–output structural decomposition analysis. J. Clean. Prod. 163, 58–68. doi: 10.1016/j.jclepro.2016.05.086
Weinzettel, J., Steen-Olsen, K., Hertwich, E. G., Borucke, M., and Galli, A. (2014). Ecological footprint of nations: comparison of process analysis, and standard and hybrid multiregional input-output analysis. Ecol. Econ. 101, 115–126. doi: 10.1016/j.ecolecon.2014.02.020
Wen, W., and Wang, Q. (2019). Identification of key sectors and key provinces at the view of CO2 reduction and economic growth in China: linkage analyses based on the MRIO model. Ecol. Indic. 96, 1–15. doi: 10.1016/j.ecolind.2018.08.036
Wiedmann, T. (2009). A review of recent multi-region input-output models used for consumption-based emission and resource accounting. Ecol. Econ. 69, 211–222. doi: 10.1016/j.ecolecon.2009.08.026
Wiedmann, T., and Lenzen, M. (2018). Environmental and social footprints of international trade. Nat. Geosci. 11, 314–321. doi: 10.1038/s41561-018-0113-9
Wiedmann, T., Wilting, H. C., Lenzen, M., Lutter, S., and Palm, V. (2011). Quo Vadis MRIO? Methodological, data and institutional requirements for multi-region input-output analysis. Ecol. Econ. 70, 1937–1945. doi: 10.1016/j.ecolecon.2011.06.014
Wieland, H., Giljum, S., Eisenmenger, N., Wiedenhofer, D., Bruckner, M., Schaffartzik, A., et al. (2020). Supply versus use designs of environmental extensions in input-output analysis: conceptual and empirical implications for the case of energy. J. Ind. Ecol. 24, 548–563. doi: 10.1111/jiec.12975
Wu, D., and Liu, J. (2016). Multi-Regional Input-Output (MRIO) study of the provincial ecological footprints and domestic embodied footprints traded among China’s 30 provinces. Sustainability 8:1345. doi: 10.3390/su8121345
Xu, J., Zhang, M., Zhou, M., and Li, H. (2017). An empirical study on the dynamic effect of regional industrial carbon transfer in China. Ecol. Indic. 73, 1–10. doi: 10.1016/j.ecolind.2016.09.002
Xu, W.-H., Xie, Y.-L., Ji, L., Cai, Y.-P., Yang, Z.-F., and Xia, D.-H. (2022). Spatial-temporal evolution and driving forces of provincial carbon footprints in China: an integrated EE-MRIO and WA-SDA approach. Ecol. Eng. 176:106543. doi: 10.1016/j.ecoleng.2022.106543
Yang, Y., and Huang, P. (2019). Has the level of green development in the Northwestern provinces of China truly improved? A case study of Shaanxi. Sustain. Cities Soc. 51:101779. doi: 10.1016/j.scs.2019.101779
Yu, J., Yu, Y., and Jiang, T. (2022). Structural factors influencing energy carbon emissions in China’s service industry: an input–output perspective. Environ. Sci. Pollut. Res. 29, 49361–49372. doi: 10.1007/s11356-022-19287-8
Yu, S., Wei, Y.-M., Guo, H., and Ding, L. (2014). Carbon emission coefficient measurement of the coal-to-power energy chain in China. Appl. Energy 114, 290–300. doi: 10.1016/j.apenergy.2013.09.062
Yuan, X., Sheng, X., Chen, L., Tang, Y., Li, Y., Jia, Y., et al. (2022). Carbon footprint and embodied carbon transfer at the provincial level of the Yellow River Basin. Sci. Total Environ. 803:149993. doi: 10.1016/j.scitotenv.2021.149993
Yue, T., Liu, H., Long, R., Chen, H., Gan, X., and Liu, J. (2020). Research trends and hotspots related to global carbon footprint based on bibliometric analysis: 2007-2018. Environ. Sci. Pollut. Res. 27, 17671–17691. doi: 10.1007/s11356-020-08158-9
Zaw, T. N., and Lim, S. (2017). The military’s role in disaster management and response during the 2015 Myanmar floods: a social network approach. Int. J. Disaster Risk Reduct. 25, 1–21. doi: 10.1016/j.ijdrr.2017.06.023
Zeng, J., Zhang, R., Tang, J., Liang, J., Li, J., Zeng, Y., et al. (2021). Ecological sustainability assessment of the carbon footprint in Fujian Province, southeast China. Front. Earth Sci. 15, 12–22. doi: 10.1007/s11707-020-0815-3
Zhang, W., Li, J., Li, G., and Guo, S. (2020). Emission reduction effect and carbon market efficiency of carbon emissions trading policy in China. Energy 196:117117. doi: 10.1016/j.energy.2020.117117
Zhang, Y., Liu, Y., Wang, Y., Liu, D., Xia, C., Wang, Z., et al. (2020). Urban expansion simulation towards low-carbon development: a case study of Wuhan, China. Sustain. Cities Soc. 63:102455. doi: 10.1016/j.scs.2020.102455
Zhang, Y., Zhang, J., Yang, Z., and Li, S. (2011). Regional differences in the factors that influence China’s energy-related carbon emissions, and potential mitigation strategies. Energy Policy 39, 7712–7718. doi: 10.1016/j.enpol.2011.09.015
Zheng, H., Bai, Y., Wei, W., Meng, J., Zhang, Z., Song, M., et al. (2021). Chinese provincial multi-regional input-output database for 2012, 2015, and 2017. Sci. Data 8:244. doi: 10.1038/s41597-021-01023-5
Zhou, P., and Wang, M. (2016). Carbon dioxide emissions allocation: a review. Ecol. Econ. 125, 47–59. doi: 10.1016/j.ecolecon.2016.03.001
Keywords: carbon neutrality, carbon reduction, carbon footprint, embodied carbon emission transfer, MRIO model, SNA method, Hanjiang River basin
Citation: Zhu K, Liu Q, Xiong X, Zhang Y, Wang M and Liu H (2022) Carbon footprint and embodied carbon emission transfer network obtained using the multi–regional input–output model and social network analysis method: A case of the Hanjiang River basin, China. Front. Ecol. Evol. 10:941520. doi: 10.3389/fevo.2022.941520
Received: 11 May 2022; Accepted: 11 July 2022;
Published: 04 August 2022.
Edited by:
Shaohui Zhang, Beihang University, ChinaReviewed by:
Murat Kucukvar, Qatar University, QatarCopyright © 2022 Zhu, Liu, Xiong, Zhang, Wang and Liu. This is an open-access article distributed under the terms of the Creative Commons Attribution License (CC BY). The use, distribution or reproduction in other forums is permitted, provided the original author(s) and the copyright owner(s) are credited and that the original publication in this journal is cited, in accordance with accepted academic practice. No use, distribution or reproduction is permitted which does not comply with these terms.
*Correspondence: Min Wang, d2FuZ21pbjIzMTFAMTYzLmNvbQ==; Hai Liu, bGl1aGFpMTExOTFAMTYzLmNvbQ==
Disclaimer: All claims expressed in this article are solely those of the authors and do not necessarily represent those of their affiliated organizations, or those of the publisher, the editors and the reviewers. Any product that may be evaluated in this article or claim that may be made by its manufacturer is not guaranteed or endorsed by the publisher.
Research integrity at Frontiers
Learn more about the work of our research integrity team to safeguard the quality of each article we publish.