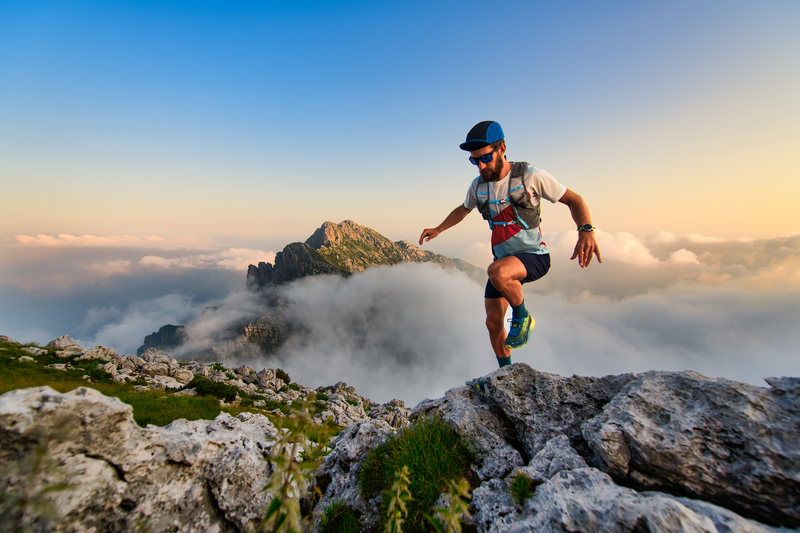
94% of researchers rate our articles as excellent or good
Learn more about the work of our research integrity team to safeguard the quality of each article we publish.
Find out more
ORIGINAL RESEARCH article
Front. Earth Sci.
Sec. Economic Geology
Volume 13 - 2025 | doi: 10.3389/feart.2025.1552058
This article is part of the Research Topic Applications of Artificial Intelligence in Geoenergy View all 3 articles
The final, formatted version of the article will be published soon.
You have multiple emails registered with Frontiers:
Please enter your email address:
If you already have an account, please login
You don't have a Frontiers account ? You can register here
Unlike conventional sandstone reservoirs, which store hydrocarbons in sandstone pores, faultcontrolled tight sandstone reservoirs are unconventional, primarily storing oil or/and gas in fault zones. While these reservoirs have significant reserves, their highly heterogeneous fault zones structures, including fault core and damage zone, pose challenges for geological modeling and precise development. Traditional two-point geostatistics (TPG) struggle to reproduce strike-slip fault zones patterns, and object-based methods have difficulty statistically quantifying their structural parameters. Deterministic methods, truncated by seismic data threshold, often misalign with well data, reducing accuracy in representing fault zone details. To overcome these challenges, we propose a new modeling workflow for fault-controlled tight sandstone reservoirs based on multi-sources information-constrained multiple-point geostatistics (MPG). First, a deep neural network (DNNs) is used to correlate conventional logging curves with fracture density (FD) to obtain well-interpreted facies data. Next, inter-well factors like brittleness index, shale content, and fault proximity are used to construct four single-sources probability bodies. These are combined into a multi-source probability body using the Permanence of Ratios (PR) method, which effectively integrates the contributions of different sources for greater constraint. Finally, the multiple-point geostatistical direct sampling (DS) algorithm generates a three-dimensional (3-D) geological model that captures the reservoir's geological features while satisfying the multi-source information constraints. The results shows that the proposed method effectively reduces model uncertainty and improves spatial prediction of the reservoir, achieving over 85% accuracy when compared with field production data. This workflow offers a promising approach for fine-scale modeling of fault-controlled tight sandstone reservoirs, with broad potential for similar reservoir development and management.
Keywords: Fault-controlled Tight Sandstone Reservoir, Geological modeling, Permanence of Ratios Probabilistic Fusion, Multiple-point geostatistics, Fracture
Received: 27 Dec 2024; Accepted: 13 Feb 2025.
Copyright: © 2025 Zhang, Hou, Long, Liang and Chen. This is an open-access article distributed under the terms of the Creative Commons Attribution License (CC BY). The use, distribution or reproduction in other forums is permitted, provided the original author(s) or licensor are credited and that the original publication in this journal is cited, in accordance with accepted academic practice. No use, distribution or reproduction is permitted which does not comply with these terms.
* Correspondence:
Jiagen Hou, China University of Petroleum, Beijing, Beijing, 102200, Beijing, China
Disclaimer: All claims expressed in this article are solely those of the authors and do not necessarily represent those of their affiliated organizations, or those of the publisher, the editors and the reviewers. Any product that may be evaluated in this article or claim that may be made by its manufacturer is not guaranteed or endorsed by the publisher.
Research integrity at Frontiers
Learn more about the work of our research integrity team to safeguard the quality of each article we publish.