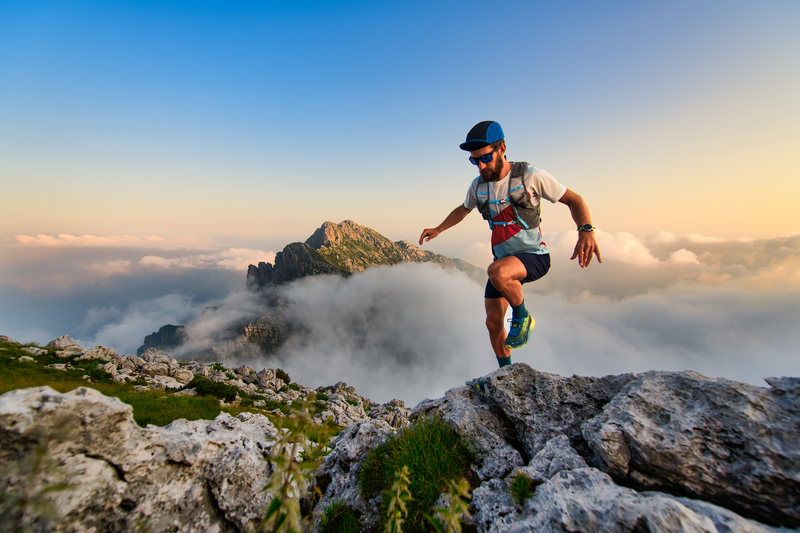
94% of researchers rate our articles as excellent or good
Learn more about the work of our research integrity team to safeguard the quality of each article we publish.
Find out more
ORIGINAL RESEARCH article
Front. Earth Sci.
Sec. Economic Geology
Volume 13 - 2025 | doi: 10.3389/feart.2025.1538908
This article is part of the Research Topic Exploration, Development, and Protection of Earth’s Resources and Environment: Methods, Techniques, Applications, Prospects, Insights, and Problems View all 36 articles
The final, formatted version of the article will be published soon.
You have multiple emails registered with Frontiers:
Please enter your email address:
If you already have an account, please login
You don't have a Frontiers account ? You can register here
Manganese is a strategic mineral resource primarily utilized in iron and steel metallurgy. As the world's largest consumer of manganese, China's future demand for this resource is crucial for its economic and social development and industrial planning. This paper focuses on manganese demand in China's iron and steel sector, establishing a combined grey prediction model and support vector regression model (GM-SVR) to forecast China's crude steel output from 2024 to 2035. Based on this output, we estimate manganese resource demand from 2024 to 2035 using the steel-to-manganese ratio. We employ qualitative and quantitative analyses to project manganese resource needs for this period by applying the "S" shape law and sectoral demand forecasting methods. These three methods indicate a gradual decrease in China's manganese demand, with projections of 12.86 million tons in 2025, 11.76 million tons in 2030, and 10.64 million tons in 2035. Despite the yearly decline in demand and tightening environmental policies, the overall need for manganese in China remains substantial. Therefore, increasing investment in manganese ore exploration is essential, as well as enhancing research and development in application technologies, optimizing the structure of manganese-related industries, and improving green growth and resource management.
Keywords: manganese resources, "S" shaped law, grey support vector machine model, Crude steel production, sectoral demand forecasting method
Received: 03 Dec 2024; Accepted: 05 Mar 2025.
Copyright: © 2025 Zhao, Han, Feng, Liu, Liu, Liu, Du and Zhu. This is an open-access article distributed under the terms of the Creative Commons Attribution License (CC BY). The use, distribution or reproduction in other forums is permitted, provided the original author(s) or licensor are credited and that the original publication in this journal is cited, in accordance with accepted academic practice. No use, distribution or reproduction is permitted which does not comply with these terms.
* Correspondence:
Hongye Feng, Institute of Mineral Resources, Chinese Academy of Geological Sciences, Beijing, 100037, China
Disclaimer: All claims expressed in this article are solely those of the authors and do not necessarily represent those of their affiliated organizations, or those of the publisher, the editors and the reviewers. Any product that may be evaluated in this article or claim that may be made by its manufacturer is not guaranteed or endorsed by the publisher.
Research integrity at Frontiers
Learn more about the work of our research integrity team to safeguard the quality of each article we publish.