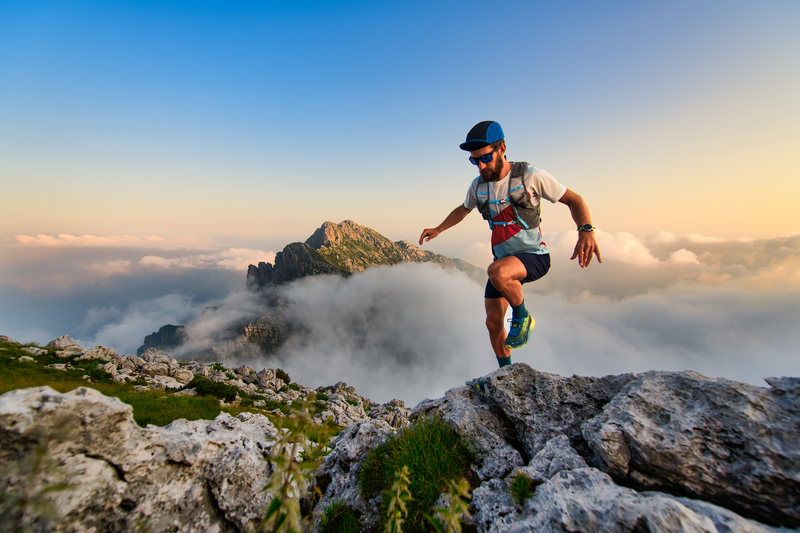
94% of researchers rate our articles as excellent or good
Learn more about the work of our research integrity team to safeguard the quality of each article we publish.
Find out more
ORIGINAL RESEARCH article
Front. Earth Sci. , 20 January 2025
Sec. Geohazards and Georisks
Volume 13 - 2025 | https://doi.org/10.3389/feart.2025.1531615
The northwest part of Hubei Province, China, is characterized by steep topography, complex geological structures, and intense precipitation, providing ideal natural conditions for landslide disasters. To address the lack of integration of synthetic aperture radar interferometry (InSAR) and geological data for the identification of latent landslide hazards, in this study, we incorporated InSAR technology and geological data to identify potential landslides in Fang County, northwest Hubei Province. With the aid of 10 ALOS-2 data scenes and high-precision digital elevation models of the study area, a displacement rate map with a maximum value of −70.6 mm/a was extracted. Then, according to the displacement rate and optical images, the suspected latent landslide area was delineated, and a comprehensive analysis of the slope map and fault and watershed buffer zone map was performed to obtain the final results. Compared to the existing latent landslide recognition method, the proposed method integrating InSAR and geological data can eliminate areas where landslides are geologically unlikely to occur, thereby enhancing the efficiency and accuracy of latent landslide hazard identification. The results were verified using geological and optical image features, which confirmed its effectiveness for identifying latent landslide hazards. The results of this research can contribute to the prediction and early warning of landslides and guide field investigations of geological disasters.
Landslides, as one of the deadliest geological disasters, rank second only to earthquakes in causing substantial economic and human losses annually (Bekaert et al., 2020; Gelete et al., 2024; Dong et al., 2019; Strozzi et al., 2018). Consequently, early and continuous monitoring of latent landslides over vast areas is paramount for disaster prevention and mitigation strategies in numerous countries, including China (Zhao et al., 2010). China, in particular, experiences frequent landslide disasters (Huang, 2007; Li et al., 2016; Tang et al., 2024), with western Hubei Province being a hotspot due to its dense forests and challenging accessibility (Lu et al., 2018; Shen et al., 2020; Sheng et al., 2024). Furthermore, the complex terrain and persistent cloud cover during the rainy season (Li et al., 2022; Ma et al., 2010) hinder the effectiveness of traditional remote sensing methods such as GPS, optical imaging, and UAV imagery.
Synthetic aperture radar interferometry (InSAR) is a groundbreaking Earth observation technology that emerged at the end of the 20th century. With its capability for all-day, all-weather, cloud-penetrating observations, InSAR provides accurate surface deformation measurements at centimeter resolution, revolutionizing the early identification and monitoring of landslides (Colesanti and Wasowski, 2006; Soltanieh and Macciotta, 2022; Tralli et al., 2005; Xiong et al., 2023). Differential InSAR (D-InSAR) has been applied to monitor small-scale landslide deformation (Achache et al., 1996; Fruneau et al., 1996). However, D-InSAR has limitations, including its ability to only detect relative deformation between two SAR images and susceptibility to temporal or spatial decorrelation and atmospheric delays (Zhu W. et al., 2019). To address these limitations, scholars have developed advanced time-series InSAR methods, such as Permanent Scatterer InSAR (PS-InSAR) (Ferretti et al., 1999), Small Baseline Subsets InSAR (SBAS-InSAR) (Berardino et al., 2002), stacking (Sandwell and Price, 1998), and SqueeSAR (Ferretti et al., 2011). These methods leverage multi-temporal radar data from repeat-pass observations, focusing on extracting and analyzing the phase signals of stable scatterers to invert time-series deformation data with millimeter-level accuracy. These methods have proven highly reliable in monitoring known sliding slopes (Dai et al., 2016; Dong et al., 2018; Liu et al., 2013; Sun et al., 2015; Tomás et al., 2016).
In terms of latent landslide hazard screening over large areas, InSAR has shown promising results. Zhao et al. (2012) were the first to identify more than 50 active landslides over an area of approximately 40 km2 on the west coast of the US using InSAR. Subsequent studies have successfully applied InSAR for similar purposes (Bekaert et al., 2020; Lian et al., 2024; Strozzi et al., 2018; Yao et al., 2022). These studies primarily rely on deformation rate maps extracted from InSAR data to identify potential landslide hazards. However, it is important to note that landslides are influenced by a multitude of factors beyond deformation rate, including slope angle, precipitation, fault layer activity, vegetation cover, and human activities (Arrara et al., 2010; Bui et al., 2016; Gheshlaghi and Feizizadeh, 2021; Li et al., 2023; Westen et al., 2008; Zhang et al., 2020). Therefore, relying solely on InSAR results for landslide identification may lead to false positives, for example, ground deformation in a flat zone is unlikely to develop into a landslide.
To address the research gap in integrating InSAR data with geological information for the identification of latent landslide hazards, this study aims to combine InSAR and geological data to identify potential landslides in Fang County, northwest Hubei Province, China. Firstly, InSAR analysis is conducted to extract the deformation rate map of the study area. By comparing this map with high-resolution optical images, unstable areas are delineated and defined as suspected landslide hazard areas. Next, a slope map and a watershed map of the survey area are generated using a high-precision digital elevation model (DEM), while a fault distribution map is extracted from the geological map. These maps are then integrated to refine the preliminary results of suspected landslide areas by eliminating unlikely landslide occurrence zones. The final latent landslide hazard results are obtained through this integrated analysis. Furthermore, optical images and geological maps are utilized to verify the identified latent landslides, ensuring the reliability of the proposed approach.
Fang County lies in the northwest of Hubei Province. The terrain is high in the west but low in the east, and steep in the south but gentle in the north, with a flat river valley in the middle area. The study area is dominated by high mountains with an altitude of 400–1,500 m. The Qingfeng fault traverses the middle of Fang County (Yin et al., 2019), forming a long and narrow fault basin (Li et al., 2014). The average annual precipitation is 914 mm, and the rainy season lasts for 100–140 days. The river system in Fang County is well-developed with a total length of 2,612 km.
The study area has a steep terrain, numerous fault structures, a well-developed water system, and intense anthropologic activities due to ongoing development, resulting in a natural propensity for landslide disasters and many previously recorded landslide events. In addition, Fang County has a large population. If a landslide occurred in a human settlement, the consequences would be unimaginable. Therefore, it is necessary to continuously monitor landslide hazard risk to ensure successful early warnings.
In this study, 10 ascending scenes of ALOS-2 fine-mode dual polarization (FDB) data from July 2015 to March 2019 were used for the InSAR analysis. Each scene has a resolution of 10 m and covers an area of 70 × 70 km2. The long wavelength of the L-band (23.8 cm) can effectively penetrate through canopy and acquire more accurate ground deformation (Abe et al., 2020; Zhu J. et al., 2019). The data coverage area is shown in Figure 1.
As a DEM is required to remove the topographic phase during InSAR processing, its accuracy has a direct and pivotal impact on the accuracy of InSAR deformation inversion results (Zhang et al., 2019; Zhu J. et al., 2019). Here, we introduced high-precision DEM data generated from the Ziyuan-3 satellite, which is the first civil high-resolution multispectral satellite with stereoimaging capability in China (Jiang et al., 2014; Wang et al., 2014). The overall elevation accuracy of the Ziyuan-3 DEM of Hubei Province is 2.3 m with a 5 m resolution (Zhou et al., 2018). Figure 2 shows a comparison between the Ziyuan-3 DEM and the commonly used the Shuttle Radar Topography Mission (SRTM) 30 m DEM, where the details of houses, rivers, and mountains are effectively preserved in the Ziyuan-3 DEM. The SRTM was measured by NASA using the space shuttle in the early 2000s (Koch and Heipke, 2002; Sun et al., 2003) and has not been updated since, except for hole filling. In the past 20 years, changes in the topography are inevitable, particularly in landslide-prone mountainous areas. Therefore, the use of more recent high-precision Ziyuan-3 DEM data can greatly reduce the impact of topographic errors (Lazecký et al., 2015).
Considering the limited amount of L-band data, the SBAS-InSAR method was used to extract the deformation as it requires less data. Firstly, an appropriate threshold was set for the temporal-spatial baseline to generate the differential interferogram, and the topographic phase was removed using Ziyuan-3 high-precision DEM data. To ensure alignment with the resolution of the SAR data and mitigate any uncertainty, the Ziyuan-3 DEM was resampled to a 10-meter resolution. Then, phase unwrapping is conducted with the minimum cost flow method. At this time, the corresponding unwrapping phase at any pixel in the
where
Here,
Due to the rank deficiency of matrix B, the singular value decomposition (SVD) method is employed to derive the minimum norm solution for the deformation rate. Subsequently, the time series deformation is obtained by integrating the velocity over each respective period.
To improve the accuracy and reliability of the results, it is necessary to estimate and eliminate these errors effectively. By introducing Ziyuan-3 DEM and constructing the linear relationship model between the elevation error and baseline, the topographic error was estimated by the least square method. According to the different characteristics of nonlinear deformation and the atmospheric delay and noise in the space and time domains, the atmospheric delay phase was separated by temporal-spatial filtering, whereas noise could be addressed using multi-looking and filtering approaches. After removing the above-mentioned phase error components, the deformation rate along the line-of-sight was obtained by singular value decomposition.
A schematic diagram of the proposed method for recognizing latent landslide hazards, which integrates both InSAR and geological data, is depicted in Figure 3. Initially, single-look complex data, after being registered, along with an external high-precision DEM, are employed to implement the SBAS-InSAR process, resulting in a deformation rate map of the study area. This data reveals the dynamic changes of the ground surface during the data acquisition period and serves as a direct indicator for identifying landslides, as latent landslides often precede large-scale movements with a creep process, characterized by slow deformation, which can be effectively captured by InSAR. This step harnesses InSAR’s capability to detect surface deformation. Subsequently, the slope map and watershed buffer zone are extracted using the same high-precision DEM data, providing insights into topographical features that may contribute to landslide risk. Additionally, the fault buffer zone is extracted from the geological map, adding crucial geological information about fault lines that could impact slope stability. Within the study area, slope, watershed, and fault activity are significant factors contributing to potential landslides (Wang et al., 2025). The latent landslide hazards are then identified by integrating all these data types, emphasizing areas where deformation, slope angle, proximity to watersheds, and fault lines converge, thereby indicating potential landslide hazards.
The deformation rate map of the study area extracted by SBAS-InSAR is shown in Figure 4. Overall, the study area is relatively stable, but exhibits small-scale severe deformation. The overall coherence is better in the central human settlement, which has better InSAR coverage, and the relatively small deformation rate indicates that this area is stable. However, many null values are interspersed in the northern and southern mountainous areas, which indicate the influence of decorrelation as well as the shadowing effect of steep terrain, with the latter as the main reason for such values. In complex mountainous terrains within the study area, the propagation path of radar signals is affected by topographic relief, leading to occlusion or distance compression. This results in multipath effects or shadow areas in radar signals, manifesting as voids in InSAR results. Meanwhile, due to the missing data in these areas, we will refer to the pixel values surrounding them for subsequent processing.
This deformation rate map of the study area is considered as a benchmark for subsequent identification of latent landslide hazards. To date, many studies have analyzed and discussed landslide factors in northwest Hubei, which predominantly include precipitation, vegetation coverage, slope rate, distance from the watershed, and geological faults (Fu et al., 2019; Long et al., 2020; Tang et al., 2020). The precipitation pattern in northwest Hubei is approximately constant each year, featuring a rainy season from April to September, and the precipitation variation within the study area is minimal (less than 100 mm). The vegetation coverage pattern is also constant; except for the central area with intense human activities, the southern and northern parts are largely covered with vegetation. Therefore, we focus on the slope rate, watershed location, and presence of faults, which are closely related to the occurrence of geological disasters. The slope rate of the study area is generated by the Ziyuan-3 DEM, and the results are shown in Figure 5A. We also use the Ziyuan-3 DEM to generate the watershed map of the study area after determining the threshold value of watershed extraction to delineate the most accurate watershed impact range. Here, we use local data to calculate the threshold using a formula described in previous literature (Sun et al., 2013). The extracted watershed data are shown in Figure 5B.
Fault data were obtained from a 1:500,000 geological map of Hubei Province archived on the GeoCloud website (http://geocloud.cgs.gov.cn/), as shown in Figure 6A. Regarding the impact of watersheds and faults on landslide hazards, the most direct impact will occur in the area within the respective buffer zones. Upon examining previous studies on the impacts of watersheds and faults on landslides in Hubei Province (Fu et al., 2019; Long et al., 2020; Tang et al., 2020), it was found that the impact of these factorial layers on landslide occurrence does not exceed 1,000 m. Therefore, the range of the watershed and fault buffer zones is conservatively set to 1,000 m, and the influence range of watersheds and faults is obtained, as shown in Figure 6B.
After all data are prepared, latent landslides are identified in the study area. First, the deformation rate map obtained by InSAR is used with optical images to circle suspected latent landslides (as shown in Figure 7). Then, these results are refined using the fault and watershed buffer zones and the slope rate map to exclude the following types of suspected landslides: 1) those with a slope rate of less than 20° and 2) those outside the buffer zones. Thus, the final recognition results are determined; the specific process is shown in Figure 8. After interpretation of the deformation rate map of the study area obtained by InSAR, a total of 44 suspected latent landslide hazard areas are identified. After a comprehensive analysis with the various geological maps, 32 are defined as latent landslide hazards. Due to the limitations of image size, Figure 9 shows the deformation rate map of each identified area superimposed onto a 1-m resolution optical image from the GF-2 satellite for a more detailed display. Below each sub-image is the center coordinate and the category, where “1” denotes a latent landslide, “2” is a suspected landslide area excluded due to a small slope rate, and “3” is a suspected landslide area excluded for being outside the watershed/fault buffer zones. Finally, to verify the accuracy of the latent landslide identification results, we combined the optical images and geological data to analyze why these areas may be prone to landslides.
In terms of their geology, the unstable areas are predominantly distributed in the northwest and northeast parts of the study area. The northwest areas (No. 1, 3, 4, 5, and 6) are located on Silurian and Sinian strata. Silurian strata are predominantly a combination of siltstone and sandstone, characterized by an inclined bedding structure and a soft rock texture. These properties, combined with the geological faulting, contribute to the instability of the area. Meanwhile, Sinian strata are predominantly composed of volcanic rocks and clastic rocks, interbedded with soft and hard rocks. The direct contact between these two sets of strata along faults, coupled with numerous secondary faults within the layers, suggests a strong tectonic stress influence in the region. Furthermore, the erosion of the well-developed water system indicates a heightened susceptibility to landslides. The northeast areas (No. 8, 9, 10, 35, 36, and 38) predominantly occur in the Mesoproterozoic Wudang group, which is divided into three lithofacies: the upper and lower metapyroclastic rock formation and sedimentary rocks with a lithologic association of schist, gneiss, metasandstone, metatuff, and other lithologies. The soft rock and well-developed faults, along with intense tectonic activity, make this region prone to landslides. Additionally, there are also a few unstable areas (No. 25, 26, 27, 28) in the south of the study area, located within Cambrian strata. The exposed lithology is predominantly slate and limestone, which possess a relatively hard rock texture. However, the dense watershed and steep slopes, as well as the strong erosional forces of the terrain, exacerbate the landslide hazard in this region.
According to the optical images, there are a few deformation areas (No. 2 and 5) caused by infrastructure construction in the study area, as well as some open-pit mines (No. 1, 8, and 33) leading to mining-induced subsidence. However, construction and open-pit mining areas are generally located in the region with gentle terrain and hence, unlikely to generate landslides. Nevertheless, construction and mining activities have a large impact on the ground surface, which requires continuous monitoring of these areas and their surroundings. There are many abandoned underground mines in the south of Fang County, which are identified as potential landslide areas (No. 20, 21, and 38). Although mining areas are backfilled before they are abandoned, subsidence is inevitable under the action of gravity. In addition, these underground mines are covered with steep slopes; thus, subsidence would contribute to the generation of landslides. In addition, the identified hazard areas include some terraced fields (No. 3, 4, 9, 12, 13, 17, 24, and 32). Because of large topographic fluctuations, there are few farmland areas available for cultivation in Fang County. As such, terraced fields are considered more suitable for local crop cultivation, as the root system of crops can fix water and soil. However, the relatively large gradient of terraced fields indicates that irrigation will remove soil and water and increases the risk of landslides; therefore, special research attention is required in these areas.
To validate the effectiveness of the method proposed in this paper, we utilized the commonly used Random Forest method to extract and map the landslide susceptibility of the study area. The selected factors were the same as the geological factors used in our method, including slope rate, distance to watershed and faults. The resulting landslide susceptibility map is shown in Figure 10. Since only three factors were used for mapping, it can be observed that the areas with very high and above susceptibility are closely concentrated around steep mountain slopes and water bodies. Moreover, the landslide susceptibility map provides a raster result of the susceptibility level across the entire study area, while our method yields specific landslide locations, which are more targeted and can significantly improve the efficiency of subsequent field survey. Quantitatively speaking, all 32 potential landslides identified using our method are located within the very high and extremely high susceptibility zones obtained from the Random Forest results, which further supports the effectiveness of our method.
It should be noted that our research focuses on the recognition and extraction of latent landslide areas rather than existing landslides because once landslides occur, decorrelation will appear on the interferograms, resulting in null values on the deformation rate map. Moreover, while optical images can readily distinguish surface differences in landslides that have already occurred, they are less effective in identifying latent landslides. Consequently, this study places a particular emphasis on recognizing latent landslide hazards. Smart measurement technologies, however, offer significant advantages in this regard. They provide real-time data, enhance the accuracy and precision of measurements, and enable the implementation of early warning systems, ultimately improving safety and response times in landslide-prone areas (Fang et al., 2024a; Fang et al., 2024b). These technological advancements serve as a valuable supplement to the data collected by landslide disaster management departments, offering scientific support for future disaster prevention strategies and decision-making processes.
Time-series InSAR is an invaluable method for remotely identifying and monitoring landslide disasters, which pose significant threats to human life and property. In this study, we employed the InSAR technique in conjunction with geological data to identify latent landslide hazards. By utilizing 10 scenes of ALOS-2 data of Fang County, we generated a deformation rate map of the study area. Our analysis revealed that the basin located in the central part of the study area exhibited relative stability, whereas localized subsidence was observed in the northern and southern regions, with a maximum subsidence rate of −70.6 mm/year. By overlaying the deformation rate map with optical images, we delineated suspected latent landslide areas. Furthermore, we created a slope map and watershed buffer map using high-precision DEM data, and extracted a fault buffer map from archived geological maps. These maps were then integrated to analyze the suspected landslide areas and identify the final potential landslide hazard zones, excluding those areas that are not geologically susceptible to landslides. Our results demonstrated that the integration of InSAR and geological data can enhance the efficiency and accuracy of the result. Verification through geological data and optical images confirmed the effectiveness of our proposed method in identifying latent landslides.
In this research, we only use ascending SAR data, which leads to many null pixels in InSAR results, as certain regions may be obscured by topographic features or other obstacles when viewed from this single angle. Joint processing of both ascending and descending SAR data will be adopted in a follow-up study. Due to the different observation geometries of ascending and descending SAR data, they capture different components of ground deformation. By combining these two types of data, we can improve the coverage of InSAR results and obtain a more comprehensive understanding of the deformation patterns and their underlying causes. Meanwhile, longer time-series data will enable us to accurately track the long-term evolution of landslides, which is also the work we plan to carry out in our future study. Meanwhile, we will also strive to establish closer cooperation with the local authorities to obtain real field survey data on the scope of landslides, in order to better validate our results. These efforts will enable a more comprehensive identification and extraction of latent landslide hazards in the study area.
The raw data supporting the conclusions of this article will be made available by the authors, without undue reservation.
SW: Writing–original draft, Writing–review and editing. QF: Investigation, Resources, Writing–review and editing. HL: Writing–review and editing.
The author(s) declare that financial support was received for the research, authorship, and/or publication of this article. This work was supported by the PhD Special Project [grant number 231384] and Youth Project Cultivation Fund [grant number 2025PY001] of Nanyang Normal University, and the Henan Provincial Science and Technology Research Project [grant number 242102320235], and Science and Technology Plan Projects of Nanyang [grant number 24JCQY009, 24KJGG013].
The authors wish to thank the High Resolution Earth Observation System Hubei Data and Application Network for providing ALOS-2 SAR data and Ziyuan-3 DEM data, as wll as NASA for SRTM DEM data. The authors also wish to thank Editage (www.editage.com) for English language editing.
The authors declare that the research was conducted in the absence of any commercial or financial relationships that could be construed as a potential conflict of interest.
The author(s) declare that no Generative AI was used in the creation of this manuscript.
All claims expressed in this article are solely those of the authors and do not necessarily represent those of their affiliated organizations, or those of the publisher, the editors and the reviewers. Any product that may be evaluated in this article, or claim that may be made by its manufacturer, is not guaranteed or endorsed by the publisher.
Abe, T., Iwahana, G., Efremov, P. V., Desyatkin, A. R., Kawamura, T., Fedorov, A., et al. (2020). Surface displacement revealed by L-band InSAR analysis in the Mayya area, Central Yakutia, underlain by continuous permafrost. Earth Planets Space 72 (1), 138–216. doi:10.1186/s40623-020-01266-3
Achache, J., Fruneau, B., and Delacourt, C. (1996). “Applicability of SAR interferometry for monitoring of landslides. ERS Applications,” in Proceedings of the second international workshop held 6-8 december, 1995 in london. Editor T. D. Guyenne (London, England: European Space Agency), 165. ESA SP-383.
Arrara, A., Cardinali, M., Detti, R., Guzzetti, F., Pasqui, V., and Reichenbach, P. (2010). GIS techniques and statistical models in evaluating landslide hazard. Earth Surf. Process. Landf. 16 (5), 427–445. doi:10.1002/esp.3290160505
Bekaert, D. P. S., Handwerger, A. L., Agram, P., and Kirschbaum, D. B. (2020). InSAR-based detection method for mapping and monitoring slow-moving landslides in remote regions with steep and mountainous terrain: an application to Nepal. Remote Sens. Environ. 249 (1), 111983. doi:10.1016/j.rse.2020.111983
Berardino, P., Fornaro, G., Lanari, R., and Sansosti, E. (2002). A new algorithm for surface deformation monitoring based on small baseline differential SAR interferograms. IEEE Trans. Geosci. Remote Sens. 40 (11), 2375–2383. doi:10.1109/tgrs.2002.803792
Bui, D. T., Tran, A. T., Klempe, H., Pradhan, B., and Revhaug, I. (2016). Spatial prediction models for shallow landslide hazards: a comparative assessment of the efficacy of support vector machines, artificial neural networks, kernel logistic regression, and logistic model tree. Landslides 13 (2), 361–378. doi:10.1007/s10346-015-0557-6
Colesanti, C., and Wasowski, J. (2006). Investigating landslides with space-borne synthetic aperture radar (SAR) interferometry. Eng. Geol. 88 (3–4), 173–199. doi:10.1016/j.enggeo.2006.09.013
Dai, K., Li, Z., Tomas, R., Liu, G., Yu, B., Wang, X., et al. (2016). Monitoring activity at the Daguangbao mega-landslide (China) using Sentinel-1 TOPS time series interferometry. Remote Sens. Environ. 186, 501–513. doi:10.1016/j.rse.2016.09.009
Dong, J., Zhang, L., Liao, M., and Gong, J. (2019). Improved correction of seasonal tropospheric delay in InSAR observations for landslide deformation monitoring. Remote Sens. Environ. 233, 111370. doi:10.1016/j.rse.2019.111370
Dong, J., Zhang, L., Tang, M., Liao, M., Xu, Q., Gong, J., et al. (2018). Mapping landslide surface displacements with time series SAR interferometry by combining persistent and distributed scatterers: a case study of Jiaju landslide in Danba, China. Remote Sens. Environ. 205, 180–198. doi:10.1016/j.rse.2017.11.022
Fang, K., Dong, A., Tang, H., An, P., Wang, Q., Jia, S., et al. (2024a). Development of an easy-assembly and low-cost multismartphone photogrammetric monitoring system for rock slope hazards. Int. J. Rock Mech. Min. Sci. 174, 105655. Article 105655. doi:10.1016/j.ijrmms.2024.105655
Fang, K., Jia, S., Tang, H., Zhou, R., Kong, Z., Fu, Y., et al. (2024b). Arching effect in slopes under excavation: classification and features. Eng. Geol. 337, 107563. Article 107563. doi:10.1016/j.enggeo.2024.107563
Ferretti, A., Fumagalli, A., Novali, F., Prati, C., Rocca, F., and Rucci, A. (2011). A new algorithm for processing interferometric data-stacks: SqueeSAR. IEEE Trans. Geosci. Remote Sens. 49 (9), 3460–3470. doi:10.1109/tgrs.2011.2124465
Ferretti, A., Prati, C., and Rocca, F. (1999). Permanent scatterers in SAR interferometry. IEEE 1999 International. Geoscience Remote Sens. Symposium 3, 1528–1530.
Fruneau, B., Achache, J., and Delacourt, C. (1996). Observation and modelling of the Saint-Étienne-de-Tinée landslide using SAR interferometry. Tectonophysics 265 (3–4), 181–190. doi:10.1016/s0040-1951(96)00047-9
Fu, S., Chen, L., Woldai, T., Yin, K., Gui, L., Li, D., et al. (2019). Community-based landslide hazard probability and risk assessment: a case in west Hubei, China. Nat. Hazards Earth Syst. Sci., 1–31. doi:10.5194/nhess-2019-259
Gelete, T. B., Pasala, P., Abay, N. G., Woldemariam, G. W., Yasin, K. H., Kebede, E., et al. (2024). Integrated machine learning and geospatial analysis enhanced gully erosion susceptibility modeling in the Erer watershed in Eastern Ethiopia. Front. Environ. Sci. 12, 1410741. doi:10.3389/fenvs.2024.1410741
Gheshlaghi, H. A., and Feizizadeh, B. (2021). GIS-based ensemble modelling of fuzzy system and bivariate statistics as a tool to improve the accuracy of landslide susceptibility mapping. Nat. Hazards 107 (2), 1981–2014. doi:10.1007/s11069-021-04673-1
Huang, R. (2007). Large-scale landslides and their sliding mechanisms in China since the 20th century. Chin. J. Rock Mech. Eng. 26 (31), 433–454.
Jiang, Y. H., Guo, Z., Tang, X. M., Li, D., Huang, W. C., and Pan, H. B. (2014). Geometric calibration and accuracy assessment of ZiYuan-3 multispectral images. IEEE Trans. Geosci. Remote Sens. 52 (7), 41614172. doi:10.1109/TGRS.2013.2280134
Koch, A., and Heipke, C. (2002). “Quality assessment of digital surface models derived from the Shuttle Radar Topography Mission (SRTM),” in Igarss 2001. Scanning the present and resolving the future. Proceedings. IEEE 2001 international geoscience and remote sensing symposium (cat. No.01CH37217). doi:10.1109/IGARSS.2001.978187
Lazecký, M., Bakoň, M., and Hlaváčová, I. (2015). “Effect of DEM inaccuracy on precision of satellite InSAR results,” in Surface models for geosciences. Lecture notes in geoinformation and cartography. Editors K. Růžičková, and T. Inspektor (Cham: Springer). doi:10.1007/978-3-319-18407-4_14
Li, H., He, Y., Xu, Q., Deng, J., Li, W., and Wei, Y. (2022). Detection and segmentation of loess landslides via satellite images: a two-phase framework. Landslides 19, 673–686. doi:10.1007/s10346-021-01789-0
Li, H., He, Y., Xu, Q., Deng, J., Li, W., Wei, Y., et al. (2023). Sematic segmentation of loess landslides with STAPLE mask and fully connected conditional random field. Landslides 20, 367–380. doi:10.1007/s10346-022-01983-8
Li, W., Liu, S., Qian, T., Dou, G., and Gao, T. (2014). Analysis of structural deformation in the north dabashan thrust belt, south qinling, Central China. Int. Geol. Rev. 56 (10), 1276–1294. doi:10.1080/00206814.2014.935966
Li, W. Y., Liu, C., Hong, Y., Zhang, X. H., Wan, Z. M., Saharia, M., et al. (2016). A public Cloud-based China’s Landslide Inventory Database (CsLID): development, zone, and spatiotemporal analysis for significant historical events, 1949-2011. J. Mt. Sci. 13, 1275–1285. doi:10.1007/s11629-015-3659-7
Lian, B., Wang, D., Wang, X., and Tan, W. (2024). Early identification and dynamic stability evaluation of high-locality landslides in yezhi site area, China by the InSAR method. Land 13 (5), 569. doi:10.3390/land13050569
Liu, P., Li, Z., Hoey, T., Kincal, C., Zhang, J., Zeng, Q., et al. (2013). Using advanced InSAR time series techniques to monitor landslide movements in Badong of the Three Gorges Region, China. Int. J. Appl. Earth Obs. Geoinf. 21, 253–264. doi:10.1016/j.jag.2011.10.010
Long, J., Liu, Y., Li, C., Fu, Z., and Zhang, H. (2020). A novel model for regional susceptibility mapping of rainfall-reservoir induced landslides in Jurassic slide-prone strata of western Hubei Province, Three Gorges Reservoir area. Stoch. Environ. Res. Risk Assess. 35, 1403–1426. doi:10.1007/s00477-020-01892-z
Lu, W., Yan, E., Wang, J., Li, Q., Zhu, Z., et al. (2018). Landslide pregnancy pattern of central area in northwest Hubei, China. J. Geol. Hazard Control. 29 (01), 60–66+71.
Ma, Y., Wei, G., Zhu, Z., Zhang, L., and Li, P. (2010). Cloud amount and aerosol characteristic research in the atmosphere over Hubei province, China. IEEE Trans. Geosci. Remote Sens. 3, III–631. doi:10.1109/IGARSS.2009.5417839
Sandwell, D. T., and Price, E. J. (1998). Phase gradient approach to stacking interferograms. J. Geophys. Res. 103 (B12), 30183–30204. doi:10.1029/1998jb900008
Shen, D., Shi, Z., Peng, M., Zhang, L., and Zhu, Y. (2020). Preliminary analysis of a rainfall-induced landslide hazard chain in Enshi city, Hubei province, China in july 2020. Landslides 18 (6), 509–512. doi:10.1007/s10346-020-01553-w
Sheng, Y., Zhang, B., Xu, G., Cheng, Z., Shi, Z., and Li, Y. (2024). Mobility forecast of gravel-silty clay landslide using ring shear test and discrete element method in Shaziba, southwestern Hubei Province, China. Bull. Eng. Geol. Environ. 83, 488. doi:10.1007/s10064-024-03983-5
Soltanieh, A., and Macciotta, R. (2022). Updated understanding of the thompson river valley landslides kinematics using satellite InSAR. Geosciences 12 (10), 359. doi:10.3390/geosciences12100359
Strozzi, T., Klimeš, J., Frey, H., Caduff, R., Huggel, C., Wegmüller, U., et al. (2018). Satellite SAR interferometry for the improved assessment of the state of activity of landslides: a case study from the Cordilleras of Peru. Remote Sens. Environ. 217, 111–125. doi:10.1016/j.rse.2018.08.014
Sun, A. L., Yu, Z. B., Yang, C. G., and Gu, H. (2013). Impact factors of contribution area threshold in extracting drainage network for rivers in China. J. Hydraul. Eng. 44 (8), 901–908.
Sun, G., Ranson, K. J., Kharuk, V. I., and Kovacs, K. (2003). Validation of surface height from shuttle radar topography mission using shuttle laser altimeter. Remote Sens. Environ. 88 (4), 401–411. doi:10.1016/j.rse.2003.09.001
Sun, Q., Zhang, L., Ding, X. L., Hu, J., Li, Z. W., and Zhu, J. J. (2015). Slope deformation prior to Zhouqu, China landslide from InSAR time series analysis. Remote Sens. Environ. 156, 45–57. doi:10.1016/j.rse.2014.09.029
Tang, B., Ren, H., Qiu, J.-a., Miao, C., and Chen, Y. (2024). Evaluation of geo-hazard risks in the pearl river delta based on geographic information system and weighted informativeness approach. Front. Environ. Sci. 12, 1406386. doi:10.3389/fenvs.2024.1406386
Tang, R. X., Kulatilake, P. H. S. W., Yan, E. C., and Cai, J. S. (2020). Evaluating landslide susceptibility based on cluster analysis, probabilistic methods, and artificial neural networks. Bull. Eng. Geol. Environ. 79 (1), 2235–2254. doi:10.1007/s10064-019-01684-y
Tomás, R., Li, Z., Lopez-Sanchez, J. M., Liu, P., and Singleton, A. (2016). Using wavelet tools to analyse seasonal variations from InSAR time-series data: a case study of the Huangtupo landslide. Landslides 13 (3), 437–450. doi:10.1007/s10346-015-0589-y
Tralli, D. M., Blom, R. G., Zlotnicki, V., Donnellan, A., and Evans, D. L. (2005). Satellite remote sensing of earthquake, volcano, flood, landslide and coastal inundation hazards. ISPRS J. Photogramm. Remote Sens. 59 (4), 185–198. doi:10.1016/j.isprsjprs.2005.02.002
Wang, B., Zuo, Q., Deng, M., Yi, Q., Ruan, D., and Liang, Z. (2025). Study on the deformation mechanism of chair-like bedding rock landslides under the coupling effect of geological and hydrological factors. Eng. Geol. 344, 107832. doi:10.1016/j.enggeo.2024.107832
Wang, T., Zhang, G., Li, D., Tang, X., Yong-hua, J., Pan, H., et al. (2014). Geometric accuracy validation for ZY-3 satellite imagery. IEEE Geosci. Remote Sens. Lett. 11 (6), 1168–1171. doi:10.1109/LGRS.2013.2288918
Westen, C. V., Castellanos, E., and Kuriakose, S. L. (2008). Spatial data for landslide susceptibility, hazard, and vulnerability assessment: an overview. Eng. Geol. 102 (3–4), 112–131. doi:10.1016/j.enggeo.2008.03.010
Xiong, Z., Zhang, M., Ma, J., Xing, G., Feng, G., and An, Q. (2023). InSAR-based landslide detection method with the assistance of C-index. Landslides 20, 2709–2723. doi:10.1007/s10346-023-02120-9
Yao, J., Yao, X., and Liu, X. (2022). Landslide detection and mapping based on SBAS-InSAR and PS-InSAR: a case study in gongjue county, tibet, China. Remote Sens. 14 (19), 4728. doi:10.3390/rs14194728
Yin, X., Yan, E. C., and Feng, B. (2019). Deformation and failure response pattern of schistosed surrounding rock to its anisotropic properties. Geotech. Geol. Eng. 37 (6), 5131–5146. doi:10.1007/s10706-019-00967-1
Zhang, F., Zhu, Y., and Zhao, X. (2020). Spatial distribution and identification of hidden danger points of landslides based on geographical factors. Geomatics Inf. Sci. Wuhan Univ. 45 (8), 1233–1244. doi:10.13203/j.whu-gis20200126
Zhang, L., Jia, H., Lu, Z., Liang, H., Ding, X., and Li, X. (2019). Minimizing height effects in MTInSAR for deformation detection over built areas. IEEE Trans. Geosci. Remote Sens. 57 (11), 9167–9176. doi:10.1109/tgrs.2019.2925115
Zhao, C. Y., Zhong, L., Zhang, Q., and de la Fuente, J. (2012). Large-area landslide detection and monitoring with ALOS/PALSAR imagery data over Northern California and Southern Oregon, USA. Remote Sens. Environ. 124, 348–359. doi:10.1016/j.rse.2012.05.025
Zhao, W., Yuan, T., Lun, W., and Liu, Y. (2010). “Human impact index in landslide susceptibility mapping,” in 2010 18th International Conference on Geoinformatics, Beijing, China, 18-20 June 2010, 1–6. doi:10.1109/geoinformatics.2010.5567817
Zhou, P., Tang, X., Guo, L., Wang, X., and Fan, W. (2018). DEM generation using Ziyuan-3 mapping satellite imagery without ground control points. Int. J. Remote Sens. 39 (19–20), 6213–6233. doi:10.1080/01431161.2018.1456702
Zhu, J., Yang, Z., and Li, Z. (2019). Recent progress in retrieving and predicting mining-induced 3D displacements using InSAR. Acta Geod. Cartogr. Sin. 48 (2), 135–144. doi:10.11947/j.AGCS.2019.20180188
Keywords: landslide, InSAR, geological data, hazard recognition, Fang County
Citation: Wang S, Fan Q and Li H (2025) Latent landslide hazard recognition in Fang County using synthetic aperture radar interferometry and geological data. Front. Earth Sci. 13:1531615. doi: 10.3389/feart.2025.1531615
Received: 21 November 2024; Accepted: 02 January 2025;
Published: 20 January 2025.
Edited by:
Chong Xu, Ministry of Emergency Management, ChinaReviewed by:
Xiaoyi Shao, China Earthquake Administration, ChinaCopyright © 2025 Wang, Fan and Li. This is an open-access article distributed under the terms of the Creative Commons Attribution License (CC BY). The use, distribution or reproduction in other forums is permitted, provided the original author(s) and the copyright owner(s) are credited and that the original publication in this journal is cited, in accordance with accepted academic practice. No use, distribution or reproduction is permitted which does not comply with these terms.
*Correspondence: Shunyao Wang, c2h1bnlhb3dhbmdAbnludS5lZHUuY24=
Disclaimer: All claims expressed in this article are solely those of the authors and do not necessarily represent those of their affiliated organizations, or those of the publisher, the editors and the reviewers. Any product that may be evaluated in this article or claim that may be made by its manufacturer is not guaranteed or endorsed by the publisher.
Research integrity at Frontiers
Learn more about the work of our research integrity team to safeguard the quality of each article we publish.