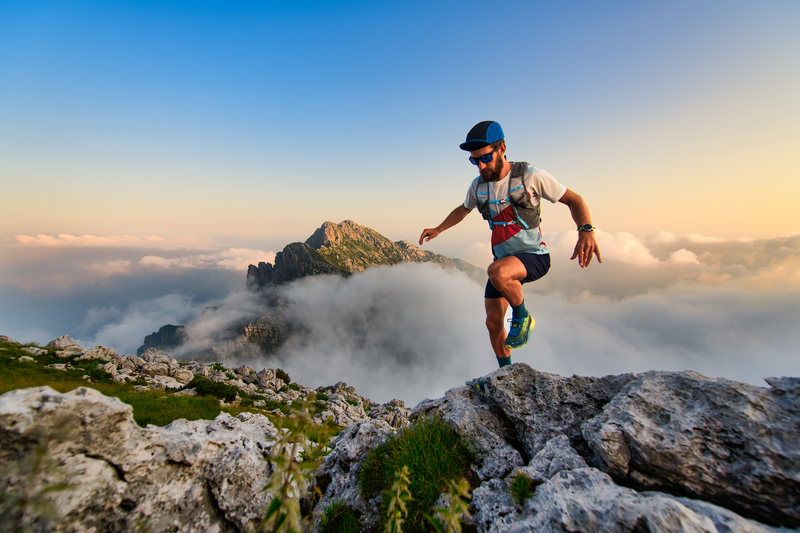
94% of researchers rate our articles as excellent or good
Learn more about the work of our research integrity team to safeguard the quality of each article we publish.
Find out more
ORIGINAL RESEARCH article
Front. Earth Sci.
Sec. Geohazards and Georisks
Volume 13 - 2025 | doi: 10.3389/feart.2025.1501498
This article is part of the Research Topic Prevention, Mitigation, and Relief of Compound and Chained Natural Hazards Volume II View all 14 articles
The final, formatted version of the article will be published soon.
You have multiple emails registered with Frontiers:
Please enter your email address:
If you already have an account, please login
You don't have a Frontiers account ? You can register here
Landslide relic inventories serve as essential data for geological disaster investigations and risk assessments. Using a previously developed landslide relic inventory for Minhe County, Qinghai Province, this study employs landslide number density (LND) and landslide area percentage (LAP) to thoroughly investigate the spatial distribution characteristics of landslides in the region. Utilizing a GIS platform, we selected ten factors for in-depth analysis, including elevation, slope aspect, slope gradient, relief degree of land surface, distance to faults, lithology, land use type, distance to rivers, rainfall, and NDVI. The results show that at least 5,517 landslide relics have developed in Minhe County, with a total landslide coverage area of 434.43 km 2 . These landslides are mainly distributed in regions with elevations of 2000-2100 meters, slope gradients of 15°-25°, Neogene strata, grassland, and within 0-2 km of rivers. Both slope and aspect are the most significant factors influencing the landslide relics in Minhe County. The findings of this study contribute to a better understanding of the development characteristics and spatial distribution of landslides in the Huangshui River Basin and provide valuable data support for future landslide assessments and disaster prevention efforts.
Keywords: Huangshui River Basin, Upper Yellow River, landslide inventory, Google Earth, Visual interpretation, spatial analysis
Received: 25 Sep 2024; Accepted: 05 Mar 2025.
Copyright: © 2025 Wang and Xu. This is an open-access article distributed under the terms of the Creative Commons Attribution License (CC BY). The use, distribution or reproduction in other forums is permitted, provided the original author(s) or licensor are credited and that the original publication in this journal is cited, in accordance with accepted academic practice. No use, distribution or reproduction is permitted which does not comply with these terms.
* Correspondence:
Chong Xu, National Institute of Natural Hazards, Ministry of Emergency Management (China), Beijing, China
Disclaimer: All claims expressed in this article are solely those of the authors and do not necessarily represent those of their affiliated organizations, or those of the publisher, the editors and the reviewers. Any product that may be evaluated in this article or claim that may be made by its manufacturer is not guaranteed or endorsed by the publisher.
Research integrity at Frontiers
Learn more about the work of our research integrity team to safeguard the quality of each article we publish.