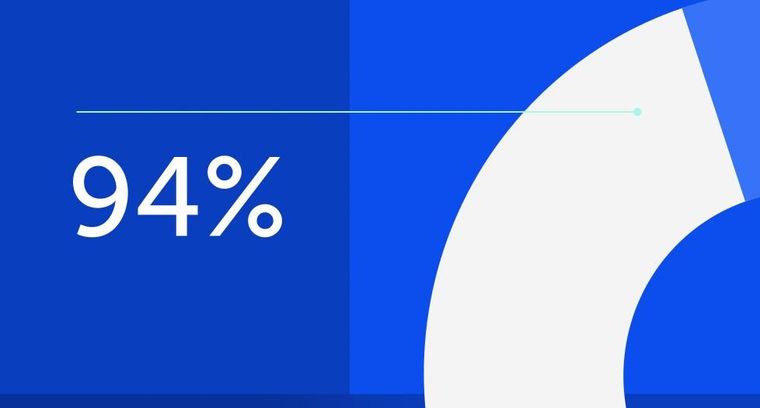
94% of researchers rate our articles as excellent or good
Learn more about the work of our research integrity team to safeguard the quality of each article we publish.
Find out more
EDITORIAL article
Front. Earth Sci., 06 December 2024
Sec. Geohazards and Georisks
Volume 12 - 2024 | https://doi.org/10.3389/feart.2024.1480635
This article is part of the Research TopicAdvances and Applications in Modeling, Assessment, and Mitigation of Landslide DisastersView all 7 articles
Editorial on the Research Topic
Advances and applications in modeling, assessment, and mitigation of landslide disasters
In recent decades, natural hazards and anthropogenic-induced disasters such as earthquakes, landslides, rockfalls, debris flows, rainstorms, floods, tunnel collapses, dam failures, and forest fires have posed major challenges. These events necessitate efforts to mitigate risks and safeguard structures, infrastructure, economic activities, and human lives, especially in mountainous areas (Cutter et al., 2015; Zou et al., 2018; Klein et al., 2019; Adler et al., 2020; Alcántara-Ayala and Geertsema, 2022).
Landslides are among the most common and destructive natural disasters worldwide, threatening human lives, properties, and infrastructure safety (Chen and Song, 2023; Chen D. et al., 2024; Liu et al., 2024; Zhang Q. et al., 2024; CRED, 2023; Kennedy et al., 2015; Froude and Petley, 2018; Fidan et al., 2024). They are characterized by intricate formation mechanisms, nonlinear deformation, and uncertainty, necessitating multidisciplinary approaches for their analysis and prediction.
Scientific research has focused on enhancing understanding and developing technologies for effective risk mitigation against landslides (Carrión-Mero et al., 2021). Advanced monitoring tools like InSAR (Interferometric Synthetic Aperture Radar) (Scaioni et al., 2014; Xu et al., 2023), UAV (Unmanned Aerial Vehicles) (Sun et al., 2024; Zhang Z. et al., 2024), Fiber Optics (Ivanov et al., 2021; Brezzi et al., 2023), Beidou (Huang et al., 2023), and MEMS (Micro-Electro-Mechanical System) have been employed (Barzegar et al., 2023).
Yang et al. used multi-temporal InSAR techniques combined with geospatial statistical analysis to study the Muyuba landslide in China. Findings revealed continuous subsidence largely influenced by drainage networks, rock strata orientation, and reservoir water level variations, linking anthropogenic activities with increased landslide risk.
Wang et al. applied wavelet transform and ARIMA models for landslide displacement prediction. The ARIMA model demonstrated high accuracy, with a root mean square error (RMSE) of 4.52 mm, confirming its effectiveness in specific conditions. This model’s applicability was further validated in practical scenarios.
Numerical methods such as CDEM continuum-based discrete element method) (Li et al., 2024), 3D-DDA (three-dimensional discontinuous deformation analysis) (Wang et al., 2021; Ma and Liu, 2022), NMM (numerical manifold method) (Cao et al., 2024; Zhang and Li, 2024), SPH (smoothed particle hydrodynamics) (Dai et al., 2014; Mahallem et al., 2022; Chen W. et al., 2024), MPM (material point method) (Conte et al., 2019; Yamaguchi et al., 2023), and LBM (Lattice Boltzmann Method) (Krüger et al., 2016), provide deeper insights into landslide dynamics. Research contributions highlight the integration of machine learning and artificial intelligence to predict soil evaporation rates using models like KNORA (Kainthura and Sharma, 2022; Tehrani et al., 2022; Youssef et al., 2023; Zhang and Wang, 2024).
Priyanka et al. utilized machine learning to predict soil evaporation, employing a novel feature selection technique to enhance accuracy. Their findings underscore the superiority of certain ML models in predicting environmental phenomena.
Laboratory techniques have been refined to better understand influential factors in gravitational phenomena (Hemeda, 2018; Menon and Kolathayar, 2024). Guo et al. focused on soil stability through wet and dry compaction tests, shedding light on risks associated with field compaction variations.
Zhao et al. analyzed water inrush disasters in coal seam mining through numerical simulations. Findings emphasized the role of mining stress and confined water in crack propagation along hidden faults, offering insights for preventing water inrush incidents.
Effective defense structure design significantly reduces landslide impact on infrastructure (Luo et al., 2023). Barbini et al. proposed methods to control sediment volume in debris flows through deposition areas and retention basins, confirmed through hydraulic modeling.
Research on landslide dynamics continues to evolve, with interdisciplinary efforts advancing our understanding of geological disaster science. This ongoing pursuit provides a scientific foundation for forecasting and mitigating geological hazards.
We express gratitude to all contributors to this Research Topic, advancing the modeling, assessment, and mitigation of landslide disasters. This compilation serves as a valuable resource, inspiring further studies in this critical field.
ZC: Writing–original draft, Writing–review and editing. DT: Writing–review and editing, Writing–original draft. OG: Writing–review and editing. DS: Writing–review and editing. MJ: Writing–review and editing. EP: Writing–review and editing. TO: Writing–review and editing.
The author(s) declare that financial support was received for the research, authorship, and/or publication of this article. This work was funded by the National Natural Science Foundation of China (52208359), the Natural Science Foundation of Sichuan Province (2024NSFSC0925), the National Natural Science Foundation of China (52109125), and the Fundamental Research Funds for the Central Universities (2023ZYGXZRx2tjD2231010).
The authors declare that the research was conducted in the absence of any commercial or financial relationships that could be construed as a potential conflict of interest.
All claims expressed in this article are solely those of the authors and do not necessarily represent those of their affiliated organizations, or those of the publisher, the editors and the reviewers. Any product that may be evaluated in this article, or claim that may be made by its manufacturer, is not guaranteed or endorsed by the publisher.
Adler, C., Balsiger, J., Grêt-Regamey, A., Heinimann, A., Huggel, C., Weingartner, R., et al. (2020). Making connections for our changing mountains: future directions for the mountain research initiative (MRI). Mt. Res. Dev. 40 (1), 1–6. doi:10.1659/mrd-journal-d-20-00045.1
Alcántara-Ayala, I., and Geertsema, M. (2022). “Construction of disaster risk in mountain systems and its integrated management,” in Montology palimpsest. Montology. Editor F. O. Sarmiento (Cham: Springer), 1, 335–355. doi:10.1007/978-3-031-13298-8_19
Barzegar, M., Tadich, P., Sainsbury, B.-A., Blanks, S., and Timms, W. (2023). Meas. Sci. Technol. 34, 025111. doi:10.1088/1361-6501/aca0b3
Brezzi, L., Damiano, E., Schenato, L., De Cristofaro, M., Netti, N., Olivares, L., et al. (2023). “Distributed fiber-optic sensors for monitoring slow landslides and anchors for their stabilization,” in Geotechnical engineering in the digital and technological innovation era. CNRIG 2023. Editors A. Ferrari, M. Rosone, M. Ziccarelli, and G. Gottardi (Cham: Springer Series in Geomechanics and Geoengineering. Springer). doi:10.1007/978-3-031-34761-0_19
Cao, X., Lin, S., Liang, Z., Guo, H., and Zheng, H. (2024). Meshless numerical manifold method with novel subspace tracking and CSS locating techniques for slope stability analysis. Comput. Geotechnics 166, 106025. doi:10.1016/j.compgeo.2023.106025
Carrión-Mero, P., Montalván-Burbano, N., Morante-Carballo, F., Quesada-Román, A., and Apolo-Masache, B. (2021). Worldwide research trends in landslide science. Int. J. Environ. Res. Public Health 18, 9445. doi:10.3390/ijerph18189445
Chen, D., Huang, W., and Liang, C. (2024). A three-dimensional smoothed particle hydrodynamics analysis of multiple retrogressive landslides in sensitive soil. Comput. Geotechnics 170, 106284. doi:10.1016/j.compgeo.2024.106284
Chen, W., Chen, Z., Song, D., He, H., Li, H., and Zhu, Y. (2024). Landslide detection using the unsupervised domain-adaptive image segmentation method. Land 13 (7), 928. doi:10.3390/land13070928
Chen, Z., and Song, D. (2023). Modeling landslide susceptibility based on convolutional neural network coupling with metaheuristic optimization algorithms. Int. J. Digital Earth 16 (1), 3384–3416. doi:10.1080/17538947.2023.2249863
Conte, E., Pugliese, L., and Troncone, A. (2019). Post-failure stage simulation of a landslide using the material point method. Eng. Geol. 253, 149–159. doi:10.1016/J.ENGGEO.2019.03.006
CRED (2023). 2023 disasters in numbers: a significant year of disaster impact. Brussels, Belgium: Université Catholique de Louvain. Available at: https://files.emdat.be/reports/2023_EMDAT_report.pdf (Accesed August 9, 2024).
Cutter, S. L., Ismail-Zadeh, A., Alcántara-Ayala, I., Altan, O., Baker, D. N., Briceño, S., et al. (2015). Global risks: pool knowledge to stem losses from disasters. Nature 522 (7556), 277–279. doi:10.1038/522277a
Dai, Z., Huang, Y., Cheng, H., and Xu, Q. (2014). 3D numerical modeling using smoothed particle hydrodynamics of flow-like landslide propagation triggered by the 2008 Wenchuan earthquake. Eng. Geol. 180, 21–33. doi:10.1016/j.enggeo.2014.03.018
Fidan, S., Tanyaş, H., Akbaş, A., Lombardo, L., Petley, D. N., and Görüm, T. (2024). Understanding fatal landslides at global scales: a summary of topographic, climatic, and anthropogenic perspectives. Nat. Hazards 120, 6437–6455. doi:10.1007/s11069-024-06487-3
Froude, M. J., and Petley, D. N. (2018). Global fatal landslide occurrence from 2004 to 2016. Nat. Hazards Earth Syst. Sci. 18, 2161–2181. doi:10.5194/nhess-18-2161-2018
Hemeda, S. (2018). Engineering failure analysis and design of support system for ancient Egyptian monuments in Valley of the Kings, Luxor, Egypt. Geoenviron Disasters 5, 12. doi:10.1186/s40677-018-0100-x
Huang, G., Du, S., and Wang, D. (2023). GNSS techniques for real-time monitoring of landslides: a review. Satell. Navig. 4, 5. doi:10.1186/s43020-023-00095-5
Ivanov, V., Longoni, L., Ferrario, M., Brunero, M., Arosio, D., and Papini, M. (2021). Applicability of an interferometric optical fibre sensor for shallow landslide monitoring – experimental tests. Eng. Geol. 288, 106128. doi:10.1016/j.enggeo.2021.106128
Kainthura, P., and Sharma, N. (2022). Hybrid machine learning approach for landslide prediction, Uttarakhand, India. Sci. Rep. 12, 20101. doi:10.1038/s41598-022-22814-9
Kennedy, I. T. R., Petley, D. N., Williams, R., and Murray, V. (2015). A systematic review of the health impacts of mass earth movements (landslides). PLOS Curr. Disasters 7. doi:10.1371/currents.dis.1d49e84c8bbe678b0e70cf7fc35d0b77
Klein, J. A., Tucker, C. M., Steger, C. E., Nolin, A., Reid, R., Hopping, K. A., et al. (2019). An integrated community and ecosystem-based approach to disaster risk reduction in mountain systems. Environ. Sci. & Policy 94, 143–152. doi:10.1016/j.envsci.2018.12.034
Krüger, T., Kusumaatmaja, H., Kuzmin, A., Shardt, O., Silva, G., and Viggen, E. M. (2016). The lattice Boltzmann method. Edition 1. Cham: Springer, 694. doi:10.1007/978-3-319-44649-3
Li, Y., Yang, X., Hu, X., Wan, L., and Ma, E. (2024). Mechanisms of rainfall-induced landslides and interception dynamic response: a case study of the Ni changgou landslide in Shimian, China. Sci. Rep. 14, 1567. doi:10.1038/s41598-024-51419-7
Liu, S., Du, J., Yin, K., Zhou, C., Huang, C., Jiang, J., et al. (2024). Regional early warning model for rainfall induced landslide based on slope unit in Chongqing, China. Eng. Geol. 333, 107464. doi:10.1016/j.enggeo.2024.107464
Luo, H. Y., Zhang, L. M., Zhang, L. L., He, J., and Yin, K. S. (2023). Vulnerability of buildings to landslides: the state of the art and future needs. Earth-Science Rev. 238, 104329. doi:10.1016/j.earscirev.2023.104329
Ma, K., and Liu, G. (2022). Three-dimensional discontinuous deformation analysis of failure mechanisms and movement characteristics of slope rockfalls. Rock Mech. Rock Eng. 55, 275–296. doi:10.1007/s00603-021-02656-z
Mahallem, A., Roudane, M., Krimi, A., and Gouri, S. A. (2022). Smoothed Particle Hydrodynamics for modelling landslide–water interaction problems. Landslides 19, 1249–1263. doi:10.1007/s10346-021-01807-1
Menon, V., and Kolathayar, S. (2024). “Review of experimental studies on rainfall-induced landslide using the laboratory flume technique,” in Proceedings of the Indian geotechnical conference 2022 volume 6. IGC 2022. Lecture notes in civil engineering. Editors B. T. Jose, D. K. Sahoo, T. Oommen, K. Muthukkumaran, S. Chandrakaran, and T. G. Santhosh Kumar (Singapore: Springer), 484, 27–33. doi:10.1007/978-981-97-3393-4_3
Scaioni, M., Longoni, L., Melillo, V., and Papini, M. (2014). Remote sensing for landslide investigations: an overview of recent achievements and perspectives. Remote Sens. 6, 9600–9652. doi:10.3390/rs6109600
Sun, J., Song, L., Yuan, G., and Zhang, H. (2024). Unmanned aerial Vehicles (UAVs) in landslide investigation and monitoring: a review. Drones 8, 30. doi:10.3390/drones8010030
Tehrani, F. S., Calvello, M., Liu, Z., Zhang, L., and Lacasse, S. (2022). Machine learning and landslide studies: recent advances and applications. Nat. Hazards 114, 1197–1245. doi:10.1007/s11069-022-05423-7
Wang, X., Wu, W., Zhu, H., Liu, F., Zhang, H., and Lin, J. S. (2021). Three-dimensional discontinuous deformation analysis with explicit contact formulation and block-wise multicore CPU acceleration. Comput. Geotechnics 139, 104410. doi:10.1016/j.compgeo.2021.104410
Xu, Q., Dong, X., Zhu, X., Deng, B., and Dai, K. (2023). Landslide collaborative observation technology based on real scene 3D view from Space-Air-Ground-Interior perspective[J]. Journal of Engineering Geology 31 (3), 706–717. doi:10.13544/j.cnki.jeg.2023-0005
Yamaguchi, Y., Makinoshima, F., and Oishi, Y. (2023). Simulating the entire rainfall-induced landslide process using the material point method for unsaturated soil with implicit and explicit formulations. Landslides 20, 1617–1638. doi:10.1007/s10346-023-02052-4
Youssef, K., Shao, K., Moon, S., and Bouchard, L. S. (2023). Landslide susceptibility modeling by interpretable neural network. Commun. Earth Environ. 4, 162. doi:10.1038/s43247-023-00806-5
Zhang, Q., Bai, Z., Huang, G., Kong, J., Du, Y., Wang, D., et al. (2024). Innovative landslide disaster monitoring: unmanned aerial vehicle-deployed GNSS technology. Geomatics, Nat. Hazards Risk 15 (1). doi:10.1080/19475705.2024.2366374
Zhang, Q., and Wang, T. D. (2024). Deep learning for exploring landslides with remote sensing and geo-environmental data: frameworks, progress, challenges, and opportunities. Remote Sens. 16, 1344. doi:10.3390/rs16081344
Zhang, R., and Li, J. (2024). Buckling failure analysis and numerical manifold method simulation for high and steep slope: a case study. Geohazard Mech. 2 (2), 143–152. doi:10.1016/j.ghm.2024.04.001
Zhang, Z., Liu, M., Tan, Y. J., Walter, F., He, S., Chmiel, M., et al. (2024). Landslide hazard cascades can trigger earthquakes. Nat. Commun. 15 (1), 2878. doi:10.1038/s41467-024-47130-w
Keywords: landslides, laboratory experiments, computational geosciences, numerical simulation, stability analysis, spatial distribution, monitoring method, disaster mitigation
Citation: Chen Z, Tiranti D, Ghorbanzadeh O, Song D, Juliev M, Pitman EB and Oommen T (2024) Editorial: Advances and applications in modeling, assessment, and mitigation of landslide disasters. Front. Earth Sci. 12:1480635. doi: 10.3389/feart.2024.1480635
Received: 14 August 2024; Accepted: 27 November 2024;
Published: 06 December 2024.
Edited and reviewed by:
Gordon Woo, Risk Management Solutions, United KingdomCopyright © 2024 Chen, Tiranti, Ghorbanzadeh, Song, Juliev, Pitman and Oommen. This is an open-access article distributed under the terms of the Creative Commons Attribution License (CC BY). The use, distribution or reproduction in other forums is permitted, provided the original author(s) and the copyright owner(s) are credited and that the original publication in this journal is cited, in accordance with accepted academic practice. No use, distribution or reproduction is permitted which does not comply with these terms.
*Correspondence: Zhuo Chen, MTM4ODI1MzUwMDlAMTYzLmNvbQ==
Disclaimer: All claims expressed in this article are solely those of the authors and do not necessarily represent those of their affiliated organizations, or those of the publisher, the editors and the reviewers. Any product that may be evaluated in this article or claim that may be made by its manufacturer is not guaranteed or endorsed by the publisher.
Research integrity at Frontiers
Learn more about the work of our research integrity team to safeguard the quality of each article we publish.